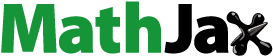
Abstract
A nonlinear ecoepidemic model of new type is introduced here, in that it contains genetically distinguishable subpopulations. Further, in the system a predator is present, that hunts these two disease-affected genotypes. Under the assumptions of the model, the disease cannot endemically survive in the predator-free environment. The healthy prey can thrive in the absence of the predators, but this is in line with previous results and does not appear to be due to the effects of the epidemics. On the other hand, the disease affects the stability of the purely demographic equilibria.
AMS subject classifications:
Public Interest Statement
Evolutionary adaptation in population genetics is observed in the peppered moth, Biston betularia, possessing melanic and non-melanic morphs. As a mimetic feature against predators, 200 years ago, it was light colored like trees trunks. But in the following century industrial pollution darkened the trees; the melanic moth, carbonaria, thrived instead. Parasites respond differently to host genotypes. Experiments with Plodia interpuncella and the granulosis virus, report an increased viral resistance in the virus-affected subpopulation. A predator–prey mathematical model is introduced, where the prey are genetically distinguishable and one genetic subpopulation suffers an epidemic. Specifically, the prey is Drosophila suzukii, one of its genotype being affected by nematodes; its predators are frogs, but also the commercially available Orius majusculus and Orius laevigatus . The ecosystem thrives, the predators are instrumental in disease eradication, both genotypes of the healthy prey can survive in a predator-free environment, predators elimination is unrelated to the disease presence.
1. Introduction
Mathematical ecogenetic models have recently been introduced (Venturino , Citation2012; Viberti & Venturino , Citation2014). They seem to be a particular example of what are nowadays commonly called “structured populations models,” that have been studied for quite some time, starting from the seminal work of Kostitzin in the 30s of the past century; on this, see the nice review paper (Scudo , Citation1976). In fact, for structured populations, now, one understands mainly populations that are not just dependent on time, but also on age, distinguishing therefore several age cohorts (Cushing , Citation1998). Human survival tables based on age cohorts have been in use among accountants for centuries, in order to calculate life insurance premiums. In ecology, in the simplest case of insects, for instance, these could be the larval, pupa, and adult life stages; for fishes instead, we would have eggs, larvae, immature juveniles, and adults. Structure therefore adds to the population description a new dimension, be it age, as depicted and analyzed in the classical papers (Gurtin & McCamy , Citation1974, Citation1979), and for which analytical approaches are available (Webb , Citation1985), or instead size, for which more sophisticated numerical methods need to be introduced (Angulo & López-Marcos , Citation1999). Indeed, the basic age description corresponds to a linear, or more generally nonlinear (Gurtin & Levine , Citation1979), wave equation, with an initial and a boundary condition at age zero, for which standard numerical schemes are available (Smith , Citation1985). These considerations could be extended to interacting populations (Venturino , Citation1984, Citation1987) A structure can also be superimposed on diseases: the classical Kermack–McKendrick model (Kermack & McKendrick , Citation1927) considers the population partitioned among susceptibles, infected, and recovered individuals. In addition, one could also include a latent period of exposed individuals in which the disease is incubating, but they are not yet able to spread it. A more general description in which all these stages are envisioned in a continuum is contained in Venturino (Citation1985).
In the of models (Venturino , Citation2012; Viberti & Venturino , Citation2014), the main idea is to consider a population which has two identifiable genotypes, and in some way intermingles with another population. In Venturino (Citation2012) the case of predator–prey interactions in considered. Here too, we examine a highly nonlinear system, containing a basic demographic situation of the same type as Venturino (Citation2012), which differs however in a major feature.
In the past 20 years or so, a lot of effort in mathematical biology has been devoted to the study of ecosystems in which also epidemics spread. Thus, in addition to their demographic interactions, populations also experience transmissible diseases. The presence of the latter profoundly influences the system’s outcomes, so that a stable population configuration from the purely demographic point of view becomes instead unattainable in presence of the disease (Beltrami & Carroll , Citation1994; Hadeler & Freedman , Citation1989; Venturino , Citation1994). The basic demographic models can be of the predator–prey type with disease affecting the prey (Chattopadhyay & Arino , Citation1999; Venturino , Citation1995), or also the predators (Auger et al. , Citation2009; Venturino , Citation2002), but can include also e.g. competition (Saenz & Hethcote , Citation2006). Some of the earlier developments of this discipline are contained in Chapter 7 of Malchow, Petrovskii, and Venturino (Citation2008). More recent contributions consider issues such as the study of equilibria (Delgado, Molina-Becerra, & Suarez , Citation2005) and their global stability (Zhen & Haque , Citation2006). Viruses in planktonic and other ecosystems have been considered in Beretta and Kuang (Citation1998) and Singh, Chattopadhyay, and Sinha (Citation2004), but the effect of viruses could also be favorably exploited to control the spread of pests (Bhattacharyya & Bhattacharya , Citation2006). More recent investigations include findings of complex dynamics (Bate & Hilker , Citation2013), and of chaotic behavior (Kooi, van Voorn, & Das , Citation2011), predation switching (Hotopp, Malchow, & Venturino , Citation2010), an idea taken from the purely demographic models (Khan, Balakrishnan, & Wake , Citation2004), applications of harvesting and control theory as measures to contain epidemics (Bairagi, Chaudhuri, & Chattopadhyay , Citation2009; Jana & Kar , Citation2013; Kar & Jana , Citation2013), more sophisticated models including delays (Bairagi, Sarkar, & Chattopadhyay , Citation2008), various functional response functions (Bairagi, Roy, & Chattopadhyay , Citation2007). These ecoepidemic models have also been reformulated as intraguild predations (Sieber & Hilker , Citation2011). A recent, rather comprehensive, review of the field is provided in Venturino (Citation2016).
It is well known that genetic variability may influence the response to pathogens and diseases. For instance, an example of this kind of situation is provided in humans by sickle cell anemia, which affects mainly individuals belonging to a recessive blood type.
In the animal realm, the classical example of evolutionary adaptation in population genetics is provided for instance by the peppered moth Biston betularia. It is well-known that this insect has several melanic and non-melanic morphs that are genetically controlled. About 200 years ago the B. betularia was light colored and this helped against predators, mainly birds, since the trunk of the trees where they rested were mainly of light colors (Grant , Citation1999). In the following century, however, smoke and pollution due to the newly implanted industries in the UK rendered the trunks of dark colors, causing higher moth mortality due to predation, as they were more easily spotted. Conversely, the melanic moth, carbonaria, thrived because with its dark color it could easily hide among the trees. Rather recently, there has been a hot debate on whether this phenomenon is attributable or not to evolutionary mechanisms (Clarke , Citation2003; Hooper , Citation2002).
In Lively and Apanius (Citation1995) it is remarked that parasites respond in different ways to various host genotypes, this being supported by some field evidence, for which parasites attack the most abundant genotype. Evidence, Lively, Craddock, and Vrijenhoek (Citation1990) and Vrijenhoek (Citation1993) shows that the parthenogenetic fish Poeciliopsis spp., which has a sexual and a clonal form coexisting in the population, infected by the trematode larvae Uvulifer spp., harbored more parasites than coexisting outcrossed fish. The result was the opposite in case of a highly inbred population, but later, when sexual fish were reintroduced into the initial inbred population, the clonal fish turned out to be more infected than the sexual ones (Vrijenhoek , Citation1993). This demonstrates that parasites can rapidly spot changes in the genotype frequency in a population.
Also, one strain of nematode that originally was able to infect four species of Drosophila, after being exposed to only one of them for a couple of years, was unable to infect one of the original species (Jaenike, Citation1993). Similarly, experiments with Plodia interpuncella and the granulosis virus (Read , Citation1991) report an increased viral resistance that was much higher in the subpopulation maintained in the presence of the virus than in the virus-free control subpopulation. This evidence indicates that host specificity may change in time due also to genetic changes.
Motivated by the fact that different genotypes may experience different responses to external interferences therefore also in animals, as mentioned above, and thus not only to predators, but also to pathogens, we consider here a hypothetical ecogenetic model, similar to some other systems already studied, that in comparison with the current literature (Venturino , Citation2012; Viberti & Venturino , Citation2014), makes a step forward, namely it introduces a disease among the genetically distinguishable population, that affects only one genotype.
Specifically, we consider two genotypes of the Drosophila suzukii, one of which is affected by nematodes, that are however not explicitly built into the model. They only partition the insects among healthy and infected classes. This fruit fly has several predators, the most common of which are the frogs. In addition, there are some that are even commercially available (Cuthbertson, Blackburn, & Audsley , Citation2014), such as Orius majusculus, Orius laevigatus, Atheta coriaria, Hypoaspis miles, and Anthocoris nemoralis.
The ecosystem under investigation then contains the prey, with two distinct genotypes, of which only one is subject to a disease. In addition, the predators hunt these subpopulations. Possible questions to which such a model could provide an answer is related to whether the presence of the predators is able to extinguish the disease, or whether the infection in the prey can wipe out the predators. The answers are tied to the interpretation of the actual situation at hand. For instance, we would like to get rid of the predators if they are seen as a pest; on the other hand, if the prey are a valuable resource, to eliminate the disease among them would certainly enhance their survival.
The presentation is organized as follows. In the next section, we describe mathematically the system in consideration. Section 3 studies the possible long-term behavior of the model, assessing its equilibria, and in Section 4 their stability is investigated. A final discussion of the findings concludes the paper.
2. The Model
Let X and Y be the genotypes of the prey population. We assume that one of them is prone to a disease, for which we partition its individuals in the two subpopulations of susceptibles S and infected I, so that . Let Z denote the predators.
We make the standard assumptions of mathematical ecogenetic models (Venturino , Citation2012), without using the more sophisticated HTII response terms considered in Viberti and Venturino (Citation2014). The two prey genotypes reproduce exponentially, a fact that is modeled via logistic terms, and produce offsprings of both genotypes with probabilities p and q, .
The reproduction at rate is r, all the offsprings are born healthy, i.e. they belong to class S, in other words the disease is not vertically transmitted. The susceptibles feel the population pressure of the similar individuals and those of the other genotype, but not of the infected. Here also the infected recovering from the disease that re-enter into this class are accounted for. Its losses are due to predation and possible contagion of the disease.
The infected are recruited only via these successful contacts, are hunted and can recover. We assume the disease to be mild and also predation to occur at a fast rate, for which the disease-induced mortality can be disregarded. We also disregard the intraspecific population pressure on the infected.
The second prey genotype population Y reproduces also logistically, feeling the intraspecific population pressure, as well as the one of the other genotype. Furthermore, contacts of Y with infected constitute an additional mortality , which we take at the same rate as the intraspecific population pressure, thus setting
. This asymmetry in the infected behavior is ascribed to the fact that they are poisonous to the predators Z, as we will see below, and in that sense they are also harmful to the genotype Y that is not their own.
The predators hunt the first genotype, independently of whether it is infected or not, and the second one Y possibly at a different rate. Their natural mortality is m, to which a mortality j due to interaction with the infected is added. Note that in describing the hunting of the predators on the infected, we separate the influences that the latter have on the predator: there is a positive effect due to the feeding, but also the contact with them might lead to the predators’ death.
The model is thus:(1)
(1)
where r is the prey reproduction rate, a and b are the intraspecific competition rates of genotypes X and Y, h and g the predators’ hunting rates on genotypes X and Y, respectively. Note that we have also implicitly assumed that it is equally likely for the predators to capture a healthy or an infected individual of genotype X. Further, denotes the conversion factor of captured prey into new predators,
is the disease contact rate and
represents its recovery rate.
3. Equilibria
We find the following system’s equilibria . The origin
, the predator- and disease-free equilibria
and the disease-free point
:
where ,
and
solves
(2)
(2)
with ,
,
. There are two cases, whether this quadratic (Equation2
(2)
(2) ) has one or two nonnegative roots. In the former, by Descartes’ rule, to have a nonnegative root it is enough to impose positivity of the first coefficient, from which we have
. In the second case, we have instead two positive roots if
,
and
. Positivity of
is ensured by
, and this entails the negativity of
and in turn the fact that the roots are real, recalling that
:
We then need the nonnegativity of , which leads to
in the former case, and to the opposite inequality in the latter, in view of the sign of . Now looking once again for sufficient conditions,
implies
in the former case and then asking
yields
. For
instead, we find that requesting
gives
and then
is ensured by
.
In summary, one equilibrium is feasible if(3)
(3)
two such points instead arise and are feasible if(4)
(4)
For the coexistence equilibrium , we solve the second and fourth equations of (Equation1
(1)
(1) ) for S and I, to get
Substitution into the remaining equations gives the following two conic sections(5)
(5)
(6)
(6)
Both are conic sections of the type described by the generic equation , with, using obvious notations,
and
for the first one and for
and
the second one.
Now the invariants of (Equation5(5)
(5) ) are
so that it is a hyperbola since , assuming nondegeneracy,
. Also (Equation6
(6)
(6) ) is a hyperbola, since
with once again and assuming nondegeneracy,
.
These curves are better investigated by solving for each variable, to get:
We seek now sufficient conditions ensuring the existence of the equilibrium where all population thrive.
For , note that if
it follows
,
. If we take also
, then
and
is a quadratic with one positive root in view of Descartes’ rule of signs. These roots
always exist since
. This statement follows observing that
so that easily
. Since
whenever
, with
, the function
is positive only in between
and
, independently of their order.
A similar analysis on shows the same result. Only in the absence of more specific information on the respective hunting rates on the two genotypes, g and h, we assume both
and
, given their asymmetry; the latter would however be a direct consequence of the former if
. Note also that taking
we obtain
. The discriminant then is always positive in view of the opposite signs of the coefficients
and
,
. The function
then is positive only in the interval between the positive root
and the vertical asymptote,
, independently of which one is the smallest.
Evidently since these curves are surjective on their respective half ranges, the half lines and
, respectively, they always meet at a feasible point. Therefore, in summary, sufficient conditions for the feasibility of the equilibrium
are provided by the following inequalities:
(7)
(7)
4. Stability
The Jacobian of (Equation1(1)
(1) ) is
(8)
(8)
with ,
and
.
is unstable, since its eigenvalues are 0,
,
,
.
The eigenvalues of the Jacobian evaluated at are
,
,
,
, implying that this point is stable for
(9)
(9)
Therefore, the predators and the disease establish themselves in the ecosystem if the disease transmission rate exceeds the threshold(10)
(10)
On comparing the last conditions in (Equation9(9)
(9) ) and in (Equation7
(7)
(7) ), we infer that a transcritical bifurcation leading from
to
might arise. The conditional is needed as (Equation7
(7)
(7) ) are only sufficient conditions for the feasibility of the coexistence equilibrium.
Equilibrium , shown in Figure , is achieved by the following choice of system’s parameter values
(11)
(11)
Figure 1. Equilibrium . Parameters listed in (Equation11
(11)
(11) ).
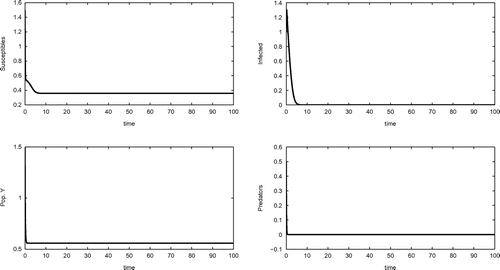
4.1. The Disease-free Equilibrium 

At one explicit eigenvalue is available:
. It gives the necessary stability condition:
(12)
(12)
The Routh–Hurwitz conditions on the remaining minor of order 3 obtained deleting the second row and column of (Equation8
(8)
(8) ) give the following inequalities:
where represents the sum of the principal minors of order 2 of the matrix
. Now, the condition on the trace gives:
from which using the value of we find
Sufficient conditions for the above inequality are(13)
(13)
For the determinant, we have
which, observing that conditions (Equation13(13)
(13) ) already imply negativity of the trace and therefore of the above first and third terms, is ensured by
(14)
(14)
The last Routh–Hurwitz condition is then:
Equilibrium , shown in Figure , is indeed achieved by the system, for the following set of parameter values
(15)
(15)
Figure 2. Equilibrium , for the parameters given in (Equation15
(15)
(15) ).
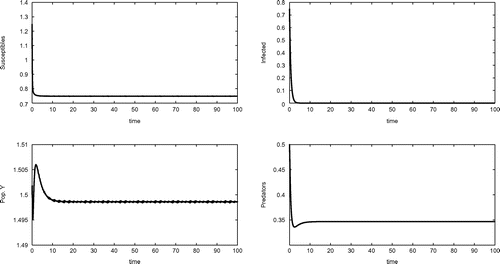
4.2. The Coexistence Equilibrium 

The Jacobian at this point simplifies a bit, in that .
The Routh–Hurwitz conditions in this case are more involved. The condition on the trace gives here(16)
(16)
Denoting by the minor of
obtained by deleting the ith row and jth column, the condition on the determinant instead provides:
which is satisfied if we take both minors negative. This holds if we impose the following two conditions:
(17)
(17)
(18)
(18)
Figure 3. Coexistence equilibrium obtained for the set of parameter values (Equation20(20)
(20) ).
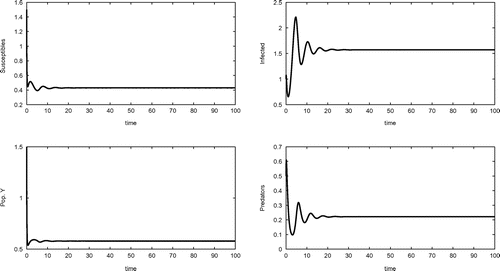
The remaining two conditions are a much more involved. In terms of the coefficients of the monic characteristic equation , they are
(19)
(19)
These coefficients are, in addition to the trace and the determinant
of the Jacobian, the sum of its principal minors of order 2 and 3. Explicitly:
and
Also the coexistence equilibrium is empirically shown to be achievable in a stable way in Figure by the following choice of parameters:(20)
(20)
5. Conclusions
The model considered in this paper extends in a new direction the ones that have been introduced in Venturino (Citation2012) and Viberti and Venturino (Citation2014). Namely, we consider the fact that one of the genotypes can be subject to a disease, that however cannot affect the other one. In addition, we assume that the infected genotype has also some toxic effects for a possible specialist predator, that feeds on both genotypes.
Our findings indicate that the ecosystem in these assumptions cannot completely disappear. The model trajectories can settle toward the disease- and predator-free equilibria, toward the predator-free one, or the system can achieve coexistence of all the populations. Thus, to answer one of the questions raised in the Introduction, the disease can be eradicated, and the predators have a fundamental role in it, as the parameters related to their dynamics appear in the necessary stability condition (Equation12(12)
(12) ). Both genotypes of the healthy prey can survive in an environment where the predators are absent, as it is found also in Venturino (Citation2012). Thus, the elimination of the predators at first sight does not appear to be tied to the presence of the disease in the prey, elucidating then the statement of the Introduction. It is also interesting to note that in the assumptions of this particular ecosystem, the disease cannot endemically survive among the prey in the absence of the predators, which seems to constitute another fact in support of a negative answer to the second claim stated in the Introduction. However, at a deeper analysis, looking at the stability conditions for the equilibrium in which only the two prey genotypes thrive, (Equation9
(9)
(9) ), we observe that the first one is essentially a purely demographic condition, which plays the same role of equation (11) in Venturino (Citation2012). The second one however is new and depends on the epidemic parameters, the disease contact rate
, and its recovery rate
. Evidently, if in the absence of the disease the two genotypes can thrive without the predators, it is possible that in the presence instead of a particularly virulent, i.e. highly transmissible, disease, combined perhaps with a low recovery rate, or in any case if the contact rate exceeds the threshold
, (Equation10
(10)
(10) ), the system can be driven away from this equilibrium. In such case certainly the disease is introduced at an endemic level and, since the endemic predator-free equilibrium here does not exist, in addition the invasion of the predators is made possible. Furthermore, if the disease is unrecoverable, i.e.
, the second condition in (Equation9
(9)
(9) ) cannot be satisfied, implying that either the disease or the predators, or both, are always present in the system.
***As it in general occurs in ecoepidemic models, the major finding of this investigation is thus the fact that the disease deeply influences the underlying demographics of the system.
Additional information
Funding
Notes on contributors
Ezio Venturino
Ezio Venturino , professor of Mathematics, holds a PhD in Applied Mathematics from SUNY at Stony Brook. His research interests concern numerical analysis and mainly mathematical modeling (biological population theory, epidemiology, and socioeconomic applications). He has authored about 40 research papers in numerical analysis and 160 in mathematical modeling. He has coauthored two books for CRC and Birkhaeuser, and serves in the Editorial Board of several international Journals. This paper is part of his scientific interests in mathematical biology, in which he has contributed to found a specific research field, now called ecoepidemiolgy, dealing with interacting populations that are in addition subject to a disease. The latter can deeply influence the ecosystem behavior. Another fairly recent idea accounts for models of herd behavior and pack hunting. Sometimes a richer dynamics is discovered. More recently, he introduced models for genetically distinguishable species interactions, of which this paper is an instance.
References
- Angulo, O., & López-Marcos, J. C. (1999). Numerical schemes for size-structured population equations. Mathematical Biosciences, 157, 169–188.
- Auger, P., Mchich, R., Chowdhury, T., Sallet, G., Tchuente, M., & Chattopadhyay, J. (2009). Effects of a disease affecting a predator on the dynamics of a predator--prey system. Journal of Theoretical Biology, 258, 344–351.
- Bairagi, N., Roy, P. K., & Chattopadhyay, J. (2007). Role of infection on the stability of a predator--prey system with several response functions---A comparative study. Journal of Theoretical Biology, 248, 10–25.
- Bairagi, N., Sarkar, R. R., & Chattopadhyay, J. (2008). Impacts of incubation delay on the dynamics of an eco-epidemiological system---A theoretical study. Bulletin of Mathematical Biology, 70, 2017–2038. doi:10.1007/s11538-008-9337-y
- Bairagi, N., Chaudhuri, S., & Chattopadhyay, J. (2009). Harvesting as a disease control measure in an eco-epidemiological system -- A theoretical study. Mathematical Biosciences, 217, 134–144.
- Bate, A. M., & Hilker, F. M. (2013). Complex dynamics in an eco-epidemiological model. Bulletin of Mathematical Biology, 75, 2059–2078. doi:10.1007/s11538-013-9880-z
- Beltrami, E., & Carroll, T. O. (1994). Modelling the role of viral disease in recurrent phytoplankton blooms. Journal of Mathematical Biology, 32, 857–863.
- Beretta, E., & Kuang, Y. (1998). Modeling and analysis of a marine bacteriophage infection. Mathematical Biosciences, 149, 57–76.
- Bhattacharyya, S., & Bhattacharya, D. K. (2006). Pest control through viral disease: Mathematical modeling and analysis. Journal of Theoretical Biology, 238, 177–196.
- Chattopadhyay, J., & Arino, O. (1999). A predator--prey model with disease in the prey. Nonlinear Analysis, 36, 747–766.
- Clarke, Bryan (2003). Heredity -- The art of innuendo. Heredity, 90(4), 279–280. doi:10.1038/sj.hdy.6800229
- Cushing, J. M. (1998). An introduction to structured population dynamics. Philadelphia: SIAM. doi:10.1038/sj.hdy.6800229
- Cuthbertson, A. G. S., Blackburn, L. F., & Audsley, N. (2014). Efficacy of commercially available invertebrate predators against Drosophila suzukii. Insects, 5, 952–960. doi:10.3390/insects5040952
- Delgado, M., Molina-Becerra, M., & Suarez, A. (2005). Relating disease and predation: equilibria of an epidemic model. Mathematical Methods in the Applied Sciences, 28, 349–362.
- Grant, B. S. (1999). Fine tuning the peppered moth paradigm. Evolution, 53(3), 980–984.
- Gurtin, M. E., & Levine, D. S. (1979). On predator-prey interactions with predation dependent on age of prey. Mathematical Biosciences, 47, 207–219.
- Gurtin, M. E., & McCamy, R. C. (1974). Nonlinearly age-dependent population dynamics. Archive for Rational Mechanics and Analysis, 54, 281–300.
- Gurtin, M. E., & McCamy, R. C. (1979). Some simple models for nonlinear age-dependent population dynamics. Mathematical Biosciences, 43, 199–211.
- Hadeler, K. P., & Freedman, H. I. (1989). Predator--prey population with parasitic infection. Journal of Mathematical Biology, 27, 609–631.
- Hooper, J. (2002). Of moths and men: An evolutionary tale. New York, NY: W.W. Norton & Company.
- Hotopp, I. S., Malchow, H., & Venturino, E. (2010). Switching feeding among sound and infected prey in ecoepidemic systems. Journal of Biological Systems, 18, 727–747. doi:10.1142/S0218339010003718
- Jaenike, J. (1993). Rapid evolution of host specificity in a parasitic nematode. Evolutionary Ecology, 7, 103–108.
- Jana, S., & Kar, T. K. (2013). Modeling and analysis of a prey--predator system with disease in the prey. Chaos, Solitons & Fractals, 47, 42–53.
- Kar, T. K., & Jana, S. (2013). A theoretical study on mathematical modelling of an infectious disease with application of optimal control. Biosystems, 111, 37–50.
- Kermack, W. O., & McKendrick, A. G. (1927). A contribution to the mathematical theory of epidemics. Proceedings of the Royal Society of London, 115, 700–721.
- Khan, Q. J. A., Balakrishnan, E., & Wake, G. C. (2004). Analysis of a predator--prey system with predator switching. Bulletin of Mathematical Biology, 66, 109–123.
- Kooi, B.W., van Voorn, G.A.K. & Das, Kp (2011). Stabilization and complex dynamics in a predator–prey model with predator suffering from an infectious disease. Ecological Complexity, 8, 113–122.
- Lively, C.M., & Apanius, V. (1995). Genetic diversity in host-parasite interactions. In: Grenfell, B.T. & Dobson, A.P. (Eds), Ecology of infectious diseases (pp. 419–449). Cambridge: Cambridge University Press.
- Lively, C. M., Craddock, C., & Vrijenhoek, R. C. (1990). Red Queen hypothesis supported by parasitism in sexual and clonal fish. Nature, 344, 864–866.
- Malchow, H., Petrovskii, S., & Venturino, E. (2008). Spatiotemporal patterns in ecology and epidemiology. Boca Raton, FL: CRC.
- Read, A. F. (1991). Passerine polygyny: A role for parasites? American Naturalist, 138, 434–459.
- Saenz, R. A., & Hethcote, H. W. (2006). Competing species models with an infectious disease. Mathematical Biosciences and Engineering, 3, 219–235.
- Scudo, F. M., & Ziegler, J. R. (1976). Vladimir Aleksandrovich Kostitzin and theoretical ecology. Theoretical Population Biology, 10, 395–412.
- Sieber, M., & Hilker, F. M. (2011). Prey, predators, parasites: Intraguild predation or simpler community modules in disguise? Journal of Animal Ecology, 80, 414–421. doi:10.1111/j.1365-2656.2010.01788.x
- Singh, B. K., Chattopadhyay, J., & Sinha, S. (2004). The role of virus infection in a simple phytoplankton zooplankton system. Journal of Theoretical Biology, 231, 153–166.
- Smith, G.D. (1985). Numerical solution of partial differential equations: Finite difference methods. Oxford: Oxford University Press.
- Venturino, E. (1984). Age-structured predator--prey models. Mathematical Modelling, 5, 117–128.
- Venturino, E. (1985). A generalization of the classical epidemiology model. IMACS Transactions on Scientific Computation ’85. Modelling of Biomedical Systems (Vol. 5, pp. 243–248). North-Holland: Amsterdam.
- Venturino E. (1987). Non-linearly interacting age-dependent populations. Computers and Mathematics with Applications, 13, 901–911.
- Venturino, E. (1994). The influence of diseases on Lotka--Volterra systems. Rocky Mountain Journal of Mathematics, 24, 381–402.
- Venturino, E. (1995). Epidemics in predator-prey models: disease in the prey. In: Arino, O., Axelrod, D., Kimmel, M., & Langlais, M. (Eds), Mathematical population dynamics, analysis of heterogeneity (Vol. 1, pp. 381–393). Winnipeg: Wuerz Publishing.
- Venturino, E. (2002). Epidemics in predator--prey models: Disease in the predators. IMA Journal of Mathematics Applied Medicine and Biology, 19, 185–205.
- Venturino, E. (2012). An ecogenetic model. Applied Mathematics Letters, 25, 1230–1233.
- Venturino, E. (2016). Ecoepidemiology: A more comprehensive view of population interactions. Mathematical Modelling of Natural Phenomena, 11, 49–90.
- Viberti, C. & Venturino, E. (2014). An ecosystem with Holling type II response and predators’ genetic variability. Mathematical Modelling and Analysis, 19, 371–394.
- Vrijenhoek, R. C. (1993). The origin and evolution of clones versus the maintenance of sex in Poeciliopsis. Journal of Heredity, 84, 388–395.
- Webb, G. (1985). Theory of nonlinear age-dependent population dynamics, Monographs and textbooks in pure & applied mathematics series Vol. 89. New York, NY: Dekker.
- Zhen, J. & Haque, M. (2006). Global stability analysis of an eco-epidemiological model of the Salton sea. Journal of Biological Systems, 14, 373–385.