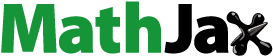
Abstract
In this research article, a new mathematical delayed human immunodeficiency virus (HIV-1) infection model with two constant intracellular delays, is investigated. The analysis of the model is thoroughly discussed by the basic reproduction numbers and
. For
, the infection-free equilibrium
is shown to be locally as well as globally stable. Similarly, the single-infection equilibrium
is proved to be locally as well as globally asymptotically stable if
. Our derived results show that the incorporation of even small intracellular time delay can control the spread of HIV-1 infection and can better the quality of the life of the patient. Finally, numerical simulations are used to illustrate the derived theoretical results.
Public Interest Statement
Time delays are usually incorporated into the mathematical models for the purpose of accurate representations of HIV-1 infection process. There are time delays between initial viral entry into a cell and subsequent viral production. Therefore, discrete delays are used in mathematical models to prove that large time delays can help to eliminate the virus and control the infection of HIV-1.
1. Introduction
Human immunodeficiency virus (HIV-1) is a lentivirus that causes acquired immunodeficiency syndrome (AIDS). HIV attacks CD4 cells and weakens the immune system. This infection passes though three different phases: the primary infection, the chronic infection and and AIDS is the last stage of HIV-1 infection. To control this infection, many scientists and researchers have been focusing on it but in spite of this, there is no effective way to cure AIDS. In the recent research, recombinant virus is used for controlling the infection of HIV-1 (see for example, Nolan, Citation1997; Wagner & Hewlett, Citation1999). Revilla and Garcya-Ramos (Citation2003) established a five-dimensional ordinary differential equation system to investigate the control of the infection by introducing a recombinant virus. Jiang, Yu, Yuan, and Zou (Citation2009), introduced a constant injection rate of the recombinant virus and presented various bifurcation patterns. A control strategy of the HIV-1 epidemic model was given in Yu and Zou (Citation2012). In Revilla and Garcya-Ramos (Citation2003), the authors analyzed the structure of equilibrium solutions and presented some simulations. Jiang et al. (Citation2009), presented the stability of all possible equilibrium solutions and bifurcations between these equilibria, as well as proved the existence of Hopf bifurcation. Yu and Zou (Citation2012), incorporated a control parameter to measure the injection rate of the recombinant for controlling/eliminating the HIV virus.
The following system of differential equations is standard and classic in-host model for HIV-1 infection (Perelson & Nelson, Citation1999)(1)
(1)
where x(t), y(t) and v(t) represent the densities of uninfected cells, infected cells and the free virus, respectively, at time t. The constant parameters in system (1) are explained as below: the positive constant is the rate at which new target cells are generated, d is their specific death rate and
is the constant rate at which a T-cell is contacted by the virus. It is assumed that once cells are infected, they may die at rate a due to the immune system or the virus. In the mean time, each of the infected cells produces new virus particles at a rate k. In Revilla and Garcya-Ramos (Citation2003), a second virus is added into model (1) which may cause the infected cells to have a second infection. Then, these cells are called double-infected cells. The system (1) is modified to the following form:
(2)
(2)
Here w(t) and z(t) represent the density of genetically modified (recombinant) virus describes and double-infected cells, respectively. The rate of infection of double-infected cells is denoted by . The recombinant are removed at a rate qw. The double-infected cells die at a rate of bz and release recombinant virus at a rate cz.
Tian, Bai, and Yu (Citation2014) and Perelson and Nelson (Citation1999) introduced the time delay in the system (2). Because, time is required for the virus to contact a target cell and then the contacted cells to become infected. They introduced the time lag into model (2) and modified the model as follows:(3)
(3)
where stands for the average time needed for a viral particle to go through the eclipse phase. Here a is the constant death rate of those infected cells which are not virus-producing cells yet. Therefore,
is the probability of surviving of cells in the time period from
to t (see also Ali, Zaman, & Algahtani,, Citation2016; Culshaw, Ruan, & Webb, Citation2003; Herz, Bonhoeffer, Anderson, May, & Nowak, Citation1996; Lv & Yuan, Citation2009; Miao & Abdurahman, Citation2013; Mittler, Markowitz, Ho, & Perelson, Citation1999; Nelson, Murray, & Perelson, Citation2000; Nelson & Perelson, Citation2002; Tian et al., Citation2014; Wang, Citation2015). However, the case where the contact process between the uninfected cells and pathogen virus is not instantaneous (see Tian et al., Citation2014) also should be examined (Tian et al., Citation2014). Here, we assume the same values of delays in both terms. This assumption is for simplicity and that it is valuable to analyze the case where the two types of time delays do not have the same values. This process was considered directly in the work of Tian et al. (Citation2014). Also, in Miao and Abdurahman (Citation2013) the authors considered delays in the model dealing with the investigations of global dynamics for a system of delay differential equations which describe a virus–immune interaction but ignored delay in rate of contact between virus and target cells. But our proposed model investigates both the local and global dynamic for a system of delay differential equations and discuss the effect of recombinant virus. In this paper, we introduce time delay, similar to the disease transmission term, in the rate of contact term. By introducing delay in the mentioned term, our proposed model becomes
(4)
(4)
We will study the dynamical behavior of the proposed model, and will show how delays influence stability of the model. We will discuss the well-posdeness of the solutions of model and the stability of all equilibrium points. Moreover, the basic reproduction numbers will be found. It will be shown that infection-free equilibrium is locally as well as globally asymptotically stable. We also show that the
(recombinant absent equilibrium) is locally as well as globally asymptotically stable.
The rest of this paper is organized as follows. The Section 2 is devoted to preliminarily results. In Section 3, local stability is discussed. Section 4 is devoted to global stability. Numerical simulation is discussed in Section 5. Finally, conclusion and discussion are drawn in Section 6.
2. Preliminary results
In this section, we will discuss the well-posedness, basic reproduction numbers, and the existence of equilibria of the proposed model (4).
Theorem 2.1
Under the given initial conditions (5), all the solutions of the system (4) are non-negative and bounded.
Proof
Let be the Banach space of continuous mapping from
to
equipped with the sup-norm. The following initial conditions
(5)
(5)
are satisfied for the system (4), where . The fundamental theory of functional differential equations (see e.g. Hale & Verduyn Lunel, Citation1993), implies that there exists a unique solution
for the given initial conditions in (5).
Using constant of variation formula, we get the following solutions of system (4).
Which clearly indicate that all the solutions are positive.
Let us define the following function to show the boundedness of the solution :
(6)
(6)
Calculating the derivative of Equation (6), we obtain
Here . This means that
is bounded, so all the solutions
are bounded.
The system (4) has the following three possible biologically meaningful equilibria: disease-free equilibrium , single-infection equilibrium
and double-infection equilibrium
, which are given by
The interpretation of each equilibrium point can be described as: is an infection-free equilibrium corresponding to maximal levels of healthy CD
T cells. The second equilibrium
corresponds to positive levels of healthy CD
T cells, infected cells, pathogen virus, but not to recombinant virus.
represent positive levels of healthy CD
T cells, infected cells, and both pathogen and recombinant virus.
The basic reproduction number (see Perelson & Nelson, Citation1999), can be defined as
where is the density of healthy cells available for infection,
is the average number of host cells that each HIV infects and
is the average number of virons that an infected cell produces. If
, then
is the only biologically meaningful equilibrium. If
, there is another equilibrium
but
exists if and only if
, where
Let , then
if and only if
,
3. Local stability
In this section, we will show the local dynamical behavior of the system (4).
Theorem 3.1
When , then the disease-free equilibrium
is locally asymptotically stable while for
,
becomes unstable and the single infection equilibrium
occurs.
Proof
The linearized system of model (4) around can be written as
(7)
(7)
The characteristic equation corresponding to the Jacobian matrix of linearized system (7) is given by(8)
(8)
where stands for eigne value. The three negative roots of the characteristic Equation (8) are
, and
and the remaining roots can be determined from the following equation:
(9)
(9)
If has non-negative real part, then modulus of the left-hand side of Equation (9) satisfies
While modulus of the right hand side of (9) satisfies
This leads to contradiction. Thus, when , then all the eigne values have negative real part. Therefore, the infection free state
is locally asymptotically stable. For
, we have
Now and
. By the continuity of
there exists at least one positive root of
. Thus, the infection-free equilibrium
is unstable if
.
Theorem 3.2
If , then the recombinant present equilibrium
is locally asymptotically stable while
become unstable for
.
Proof
The linearized system of model (4) at is given by
(10)
(10)
The characteristic equation corresponding to the Jacobian matrix of system (10) can be written in simplified form as , where
Now can be simplified as
which indicates that has two roots with negative real part if and only if
(i.e.
), or one positive and one negative root if
(i.e.
). Therefore, if
, then single-infection equilibrium
is unstable. After some simplification
, can be written as
(11)
(11)
where
It is easy to see that is not a root of (11) if
, since
When , then (11) becomes
(12)
(12)
Applying the Routh–Hurwitz criterion (see Gantmacher, Citation1959), we know that all the roots of (12) have negative real part, because
Similarly,
Thus, any root of (11) has negative real part when . Now, we consider the distribution of the roots when
. Let
be the purely imaginary root of (11), then
The modula of the above equation result in(13)
(13)
Since
Thus, all the coefficients of are positive. Therefore, the function
is monotonically increasing for
with
. This implies that Equation (13) has no positive roots if
. Hence, all the roots of (11) have negative real parts for
if
.
4. Global stability
In this section, we will study the global stability of equilibria of system (4) by using suitable Lyapunov functionals and LaSalles invariant principle.
Theorem 4.1
When the disease-free equilibrium
is globally asymptotically stable.
Proof
Let us consider the following Lyapunov functional(14)
(14)
where stands for Lyapunov functional at
, the derivative of (14) and the use of (4), yield the following equation
After further simplification, the above equation becomes(15)
(15)
Thus, when , then Equation (15) implies that
and the equality holds if and only if
,
,
,
,
. Therefore, by LaSalle’s invariance principle (see LaSalle, Citation1976), we conclude that
is globally asymptotically stable when
.
Theorem 4.2
For , the single infection equilibrium
is globally asymptotically stable.
Proof
Let us construct the Lyapunove functional(16)
(16)
where stands for Lyapunov function at single infection equilibrium
. The derivative of Equation (16) yields
(17)
(17)
The model (4) at single-infection equilibrium becomes
If is very large, i.e. when delay in contact of targeted cells with virus and the latent period is very large, then the rate of infection will be very small and contrarily if
is very small, then the infection will spread more rapidly. Therefore, we suppose that delay is very large, then
(18)
(18)
Therefore, Equation (17) becomes(19)
(19)
The following inequalities hold
By using the above inequalities, Equation (19) implies that when
, and the equality holds when
. Then, by LaSalle’s invariance principle (LaSalle, Citation1976), we conclude that
is globally asymptotically stable.
5. Numerical simulation
In this section, we illustrate the theoretical results obtained in previous sections numerically. We discuss some numerical results and simulations by using dde23 from the software MATLAB R2010a. These results show that delays play an important role in determining the dynamic behavior of the HIV-1 modeling. The delay can change the dynamic behavior quantitatively.
For numerical simulation, we have taken some of the values estimated and some of them experimental. (Density of CD
T cells in the healthy human blood is
(Michie, McLean, Alcock, & Beverly, Citation1992). Assumed equilibrium, their production
equal loss
. Assumed that a fraction
of new generated cells are activated
(Herelle, Citation1926)).
(Average life span of CD4+T cell is two years, so
(Michie et al., Citation1992). From modeling,
(Stafford et al., Citation2000)).
(Assumed indirectly as a small value that preserves both infections. For single infection
(Michie et al., Citation1992)),
(Stafford et al., Citation2000)).
(Based on life span of HIV-1 infected cells of three days (Michie et al., Citation1992). Also, Other estimates:
(Perelson, Neumann, Markowitz, Leonard, & Ho, Citation1996),
(Michie et al., Citation1992)).
(Estimated indirectly as a small value that preserves the double infection. Taken identical to
).
(Based on observations of virus release within 8 h of infection before lysis (Schnell, Johnson, Buonocore, & Rose, Citation1997)).
(Based on life span of 1 / 2 day (Michie et al., Citation1992). Another value,
(Perelson et al., Citation1996)).
(
.
is total number of infectious HIV-1 produced by a cell:
(Ali, Zaman, & Chohan, Citation2016; Layne, Spouge, & Dembo, Citation1989).
(
.
is total number of infectious re-combinant produced by a double-infected cell. In vitro total number of recombinants per cell is
(Schnell et al., Citation1997). Assumed
).
(estimated identical to p).
days (Estimated).
Figure shows the simulation of system (4) at and represents convergence to the stable equilibrium
. If we decrease the value further, i.e.
, then
will lose its stability and the double-infection equilibrium
will occur, which is shown in Figure . Simulation of system (4) for
are shown in Figure . Comparing the results in Figure with that in Figure shows that the solution trajectory takes longer to converge to its steady-state value, as we see that all the components have more oscillating behaviors having amplitude very large, and they take longer time to converge to
. Also, it can be noted that the amplitudes of the oscillations increases. Therefore, the incorporation of even small delay in model (4) can produce significant quantitative changes in its solutions. This significance of delay can not be seen from the model without delay. Hence, time delays are very important for the modeling of HIV-1 infection and cannot be ignored.
6. Conclusion
In this paper, a delayed HIV-1 model is presented. It has been shown that our proposed model with delay has three equilibrium solutions: the disease-free equilibrium , single-infection equilibrium
, and double-infection equilibrium
. Also, we have shown that a series of bifurcations occur as the basic reproduction number
is increased. It is noted that to reduce the density of pathogen virus, a strategy should aim to reduce the value of
to below one. From the derived formula for
, we see that
can be decreased by increasing either of the time delays. Also, the incorporation of intracellular delay plays a positive role in preventing the virus. It is observed that keeping all other parameters fixed, larger
can bring
to a level lower than one, making the infection-free equilibrium point globally asymptotically stable. It has been shown that
is locally as well as globally asymptotically stable for
, and becomes unstable at the transcritical bifurcation point
, and bifurcates into
, which is stable for
. Time delay may change dynamic behavior quantitatively and qualitatively even in the normal range of values. Therefore, time delay is a very important fact and cannot be ignored for reducing the infection of HIV-1.
Additional information
Funding
Notes on contributors
Nigar Ali
Nigar Ali has done his MSc mathematics from university of peshawer, Pakistan and MPhil in Mathematics from Malakand University, Pakistan. He is a PhD scholar in Department of Mathematics, UOM, Pakistan. His area of interest includes biomathematics, fractional differential equations, and fluid mechanics.
Gul Zaman
Gul Zaman is working as an associate professor in Department of Mathematics, UOM, Pakistan. He has many research papers and attended several national and international conferences and seminars. His area of interest includes biomathematics, fractional differential equations, and fluid mechanics.
Muhammad Ikhlaq Chohan
Muhammad Ikhlaq Chohan is an assistant professor at Department of Business Administration and Accounting, Buraimi University College, AlBuraimi, Oman. His area of interest includes biomathematics and applied mathematics.
References
- Ali, N., Zaman, G., & Algahtani, O. (2016). Stability analysis of HIV-1 model with multiple delays. Advances in Difference Equations, 1, 1–12.
- Ali, N., Zaman, G., & Chohan, M. I. (2016). Dynamical behavior of HIV-1 epidemic model with time dependent delay. Journal of Mathematical and Computational Science, 6, 377–389.
- Culshaw, R. V., Ruan, S., & Webb, G. (2003). A mathematical model of cell-to-cell HIV-1 that include a time delay. Journal of Mathematical Biology, 46, 425–444.
- Gantmacher, F. (1959). The theory of matrices (Vol. 2). New York, NY: Chelsea.
- Hale, J., & Verduyn Lunel, S. (1993). Introduction to functional differential equations. New York, NY: Springer Verlag.
- Herelle, P. (1926). The bacteriophage and its behavior. Baltimore, MD: Williams and Williams.
- Herz, A. V. M., Bonhoeffer, S., Anderson, R. M., May, R. M., & Nowak, M. A. (1996). Viral dynamics in vivo: Limitations on estimates of intracellular delay and virus decay. Proceedings of the National Academy of Sciences USA, 93, 7247–7251.
- Jiang, X., Yu, P., Yuan, Z., & Zou, X. (2009). Dynamics of an HIV-1 therapy model of fighting a virus with another virus. Journal of Biological Dynamics, 3, 387–409.
- LaSalle, J. (1976). The stability of dynamical systems. Philadelphia: SIAM.
- Layne, S. P., Spouge, J. L., & Dembo, M. (1989). Quantifying the infectivity of human immunodeficiency virus. Proceedings of the National Academy of Sciences USA, 86, 4644–4648.
- Lv, C., & Yuan, Z. (2009). Stability analysis of delay differential equation models of HIV-1 therapy for fighting a virus with another virus. Journal of Mathematical Analysis and Applications, 352, 672–683.
- Miao, H., & Abdurahman, X. (2013). Global stability of HIV-1 infection model with two time delays. Abstract and Applied Analysis, 12 pages. Article ID 163484.
- Michie, C. A., McLean, A., Alcock, C., & Beverly, P. C. L. (1992). Lifespan of human lymphocyte subsets defined by CD45 isoforms. Nature, 360, 264–265.
- Mittler, J. E., Markowitz, B., Ho, D. D., & Perelson, A. S. (1999). Improved estimates for HIV-1 clearance rate and intracellular delay. AIDS, 13, 1415–1417.
- Nelson, P. W., Murray, J. D., & Perelson, A. S. (2000). A model of HIV-1 pathogenesis that includes an intracellular delay. Mathematical Biosciences, 163, 201–215.
- Nelson, P. W., & Perelson, A. S. (2002). Mathematical analysis of delay differential equation models of HIV-1 infection. Mathematical Biosciences, 179, 73–94.
- Nolan, G. (1997). Harnessing viral devices as pharmaceuticals: Fighting HIV-1s fire with fire. Cell, 90, 821–824.
- Perelson, A., & Nelson, P. (1999). Mathematical models of HIV dynamics in vivo. SIAM Review, 41, 3–44.
- Perelson, A. S., Neumann, A. U., Markowitz, M., Leonard, J. M., & Ho, D. D. (1996). HIV-1 dynamics in vivo: Virion clearance rate, infected cells life-span, and viral generation time. Science, 271, 1582–1586.
- Revilla, T., & Garcya-Ramos, G. (2003). Fighting a virus with a virus: A dynamic model for HIV-1 therapy. Mathematical Biosciences, 185, 191–203.
- Schnell, M. J., Johnson, E., Buonocore, L., & Rose, J. K. (1997). Construction of a novel virus that targets HIV-1 infected cells and control HIV-1 infection. Cell, 90, 849–857.
- Stafford, M. A., Corey, L., Cao, Y., Daar, E. S., Ho, D. D., & Perelson, A. S. (2000). Modeling plasma virus concentration during primary HIV infection. Journal of Theoretical Biology, 203, 285–301.
- Tian, Y., Bai, Y., & Yu, P. (2014). Impact of delay on HIV-1 dynamics of fighting a virus with an other virus. Mathematical Biosciences and Engineering, 11, 1181–1198.
- Wagner, E., & Hewlett, M. (1999). Basic virology. New York, NY: Blackwell.
- Wang, J. (2015). K, Wang, Z. Jiang, Dynamical behaviors of an HTLV-I infection model with intracellular delay and immune activation delay, Advances in Difference Equations, 2015, 243.
- Yu, P., & Zou, X. (2012). Bifurcation analysis on an HIV-1 Model with constant injection of recom- binant. International Journal of Bifurcation and Chaos, 22, 21 pages. 1250062