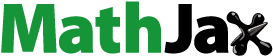
Abstract
Onchocerciasis (river blindness) is a disease spread from black flies to humans. This disease is responsible for chronic morbidity in sub-Saharan Africa. The principal strategy to achieve onchocerciasis elimination is through mass drug administration with ivermectin, a drug that is effective in the short term but wanes quickly. Ivermectin kills the skin-dwelling microfilariae. It may also kill and/or sterilize adult worms. This treatment protocol occurs bi-annually. Consequently, a system of impulsive differential equations is introduced to model both fixed and non-fixed mass drug administration with ivermectin. We determine the threshold for the proportion of treated individuals that reduces the infection in the human population. In the absence of impulsive mass drug administration with ivermectin, we determine the threshold for eradication and carry out stability analysis. The sensitivity analysis results reveal that the disease is unlikely to be eradicated without extremely low transmission levels or strong vector control. If treatment is included, then treatment at fixed intervals can control but not eradicate the disease. Treatment at non-fixed intervals may produce bursts of infection. Thus, bi-annual mass drug administration with ivermectin that is tailored to eradicate onchocerciasis, can only lead to significant reduction of onchocerciasis. However, to achieve 2020/2025 onchocerciasis elimination goals set by World Health Organization, the human-vector contact should be sufficiently reduced and vector control programmes implemented to supplement an intensive and effective mass drug administration with ivermectin.
Public Interest Statement
Impulsive differential equations are very helpful in studying many biological systems and population dynamics that have sudden change in their states. In epidemiology, impulsive differential equations assess the vaccination and or treatment program that consists of periodical repetitions of impulsive vaccinations and or treatment in a population, on all the age cohorts. The results from the pulse control strategy support the fact that eradication of onchocerciasis is possible with tolerable regularity of mass drug administration with ivermectin.
1. Introduction
Onchocerciasis (also known as river blindness) is a neglected tropical disease caused by the filarial nematode Onchocerca volvulus in human hosts (Feasey, Wansbrough-Jones, Mabey, & Solomon, Citation2009). The disease is transmitted by the black fly (Simulium damnosum) (Turner, Basáñez, Walker, & Churcher, Citation2014). Simulium breeds close to oxygen-rich flowing streams and rivers in inter-tropical zones (Crosskey, Citation1990; Kealey, Citation2010). Onchocerciasis endemic areas thus tend to include the most agriculturally fertile land available. The infection of onchocerciasis begins at the third larvae stage in the human host after the vector’s blood meal. The larvae develop into adult filariae in the subcutaneous tissues from which microfilariae migrate throughout the body, predominantly within the skin (Lustigman, MacDonald, & Abraham, Citation2003). This produces an intense itching of the skin and a chronic dermatitis that leads to skin atrophy and depigmentation (Cox, Citation2002). The migration of microfilariae through the anterior and posterior segments of the eye precipitates inflammatory reactions that lead to loss of vision and sometimes blindness (Kealey, Citation2010; Mopecha & Thieme, Citation2003).
Various onchocerciasis control strategies have achieved dramatic success in reducing onchocerciasis transmission (Traoré et al., Citation2009; Winnen et al., Citation2002). The strategies have employed both the use of larvicide spraying at black fly breeding sites and the periodic mass drug administration with ivermectin to the affected human populations. The control programmes have been spearheaded by three international programmes: the Onchocerciasis Control Program, the Onchocerciasis Elimination Programme in the Americas and the African Program for Onchocerciasis Control (APOC). Transmission of onchocerciasis was interrupted in Guatemala and in several hyper-endemic foci of Mali and Senegal following six-monthly mass drug administration with ivermectin (Gonzalez et al., Citation2009). In 2014, Columbia and Ecuador were declared free of onchocerciasis following the implementation of the control strategies for decades by the WHO (Global Health Observatory, Citation2016).
Mass drug administration with ivermectin is the main strategy used to achieve elimination of onchocerciasis in sub-Saharan Africa by 2020/2025 as articulated by the 2012 Roadmap of World Health Organization (WHO) (Hopkins & Boatin, Citation2011; Winnen et al., Citation2002; World Health Organization, Citation2012). Ivermectin kills the skin dwelling microfilariae that are the progeny of adult Onchocerca volvulus and are infectious to biting black fly species vectors. Ivermectin may also kill and/or sterilize adult worms (Gardon, Boussinesq, Kamgno, Gardon-Wendel, & Duke, Citation2002). Multiple rounds of mass treatment are effective in lowering the prevalence and intensity of onchocerciasis. Furthermore, if given for long enough at high enough coverage, it can lead to the interruption of transmission and elimination of the infection (Lovato et al., Citation2014). Mass treatment with ivermectin was initially carried out annually. However, a change in strategy to six monthly mass treatments was adopted to increase the probability of disease eradication (Barrett & Stanberry, Citation2009; Winnen et al., Citation2002).
Although substantial epidemiological work has been done on the study of onchocerciasis transmission dynamics, little has been done to model the disease using impulsive differential equations. Our aim is to examine the effects of mass drug administration with ivermectin using a mathematical model of impulsive differential equations. The model focuses on the effect of fixed and non-fixed mass drug administration with ivermectin in the treatment of onchocerciasis to account for the reduction of infection of onchocerciasis in the human population. Thus, we endeavour to address the following research questions: (1) Can we determine the critical threshold for the proportion of individuals with ochocerciasis that will reduce the infection of the onchocerciasis in the population? (2) Can mass drug administration with ivermectin eradicate onchocerciasis? (3) Does control of onchocerciasis depend on mass drug administration with ivermectin occurring at fixed times? Thus, the potential effect of mass treatment on onchocerciasis transmission dynamics is of great interest.
In this manuscript, our work is organized as follows: related literature is provided in Section 2. In Section 3, we formulate the mathematical model. In Section 4, we analyse the non-impulsive version of the model by calculating the basic reproduction number and carrying out stability analysis. In Section 5, we analyse the model with impulses and determine the effectiveness of mass drug administration with ivermectin for the regular mass treatment. Numerical simulation results are then presented in Section 6. In Section 7, we conclude our project and give relevant recommendation.
2. Related literature
A number of epidemiological models have been formulated and mathematically analysed to describe the behaviour of onchocerciasis in the population. Walker et al. (Citation2017) tested the EPIONCHO and ONCHOSIM models against epidemiological data. The data were collected over two decades from 27 sentinel communities in two onchocerciasis foci in Mali and Senegal where the observed prevalence of infection was brought to zero circa 2007–2009 after 15–17 years of mass treatment with ivermectin. They simulated the models using programmatic information on the frequency and coverage of mass treatments and trained the model projections using longitudinal parasitological data, evaluating the projected outcome of elimination (local parasite extinction) or resurgence. Their findings suggested that meeeting onchocerciasis elimination goals of 2020/2025 depends on when an intervention began; how intensively and effectively it was implemented and on the local transmission conditions. Furthermore, in areas where the prevailing ecological conditions are highly propitious to transmission, elimination by 2020 or 2025 may be unfeasible with ivermectin alone. Thus, they suggested that localized low-cost vector control should be considered as a complement to mass treatment with ivermectin alongside other alternative treatment strategies that include anti-wolbachial therapies to accelerate progress towards the 2020/2025 goals.
Authors in Lont et al. (Citation2017), Golden et al. (Citation2016) extended ONCHOSIM model with new output on the Ov16 antibody serostatus of individuals based on their history of infection. They then used ONCHOSIM simulation model to explore for different transmission settings how the Ov16 antibody prevalence in children 1 year after the last mass drug administration with ivermectin round is related to the duration and coverage of mass drug administration with ivermectin, the microfilariae prevalence in the population and the probability that onchocerciasis is eventually eliminated. The results suggested that the post mass administration of ivermectin microfilariae prevalence in the population aged 5 years and above and the Ov16 antibody prevalence in children aged 0-9 years decline with increasing duration and treatment coverage.
Mopecha and Thieme (Citation2003) developed a model to account for both human and bovine onchocerciasis. They considered cross-reactive immunity in response to inocculation of cattle by larvae. They showed that if parasites are wasted on the “wrong" hosts, then the reproduction number is lowered, while cross-reactive immunity can lead to classical competition phenomena. Basáñez and Boussinesq (Citation1999), Basáñez and Ricárdez-Esquinca (Citation2001) developed a model to describe the population biology and control of human onchocerciasis. Their aim was to evaluate various interventions combining the removal of adult worms (nodulectomy) and the microfilaricidal and sterilizing effects of ivermectin. They derived a threshold condition for disease control involving the vector biting rate and the basic reproduction number. They estimated that the annual biting rate for the Simulium onchraceum s.l is 7665 bites per person per year. This is the minimum below which human onchocerciasis would be unable to become endemic in Central America since the basic reproduction number, , would be less than unity. They recommended that if the worm is sterilised and the vector population is condensed, then the epidemic could be restricted. In Africa, several field studies have achieved significant reduction in the transmission of infection by repeated annual mass treatment with ivermectin (Guillet et al., Citation1995; Plaisier, Alley, Van Oortmarssen, Boatin, & Habbema, Citation1997; Remme, De Sole, & Van Oortmarssen, Citation1990).
Alley et al. (Citation2001) used a microsimulation model to examine the possibility of switching treatment from the microfilaricide ivermectin to a hypothetical macrofilaricide. They showed that elimination was possible using a macrofilaricide, but that high coverage levels were critical. Filipe et al. (Citation2005) developed an age and sex structured model for intensity of infection with parasite regulation within humans and vectors. They showed that heterogeneous age and sex exposure could explain location specific infection patterns of onchocerciasis. Poolman and Galvani (Citation2006) incorporated host heterogeneity into a model in order to evaluate intervention strategies targeting specific populations for treatment with ivermectin. They found that targeted allocation of ivermectin in a highly heterogeneous population could reduce the public health burden of onchocerciasis using 20–25% of the doses of untargeted allocation. Over the years, a very large literature on mathematical modelling of onchocerciasis has been produced and other several reviews pertinent to this study are available (Basáñez, Walker, Turner, Coffeng, de Vlas, & Stolk, Citation2016; Coffeng et al., Citation2017; Lovato et al., Citation2014; Oguoma & Acho, Citation2014; OHanlon et al., Citation2016; Omondi, Orwa, & Nyabadza, Citation2017; Omondi, Nyabadza, Bonyah, & Badu, Citation2017).
This paper is motivated by the work in Omondi (Citation2016) and was done as an Msc. research project by the first author. Stopping the transmission chain of onchocerciasis remains the only viable form of control against the spread of infection. The potential effect of mass administration of ivermectin is thus of great interest. We focus on the application of impulsive differential equations to model the periodic mass administration of ivermectin tailored towards eradicating onchocerciasis. To the author?s best knowledge, impulsive differential equation has not been applied to onchocerciasis disease transmission.
3. The mathematical model
3.1. Model formulation
We develop a model to better understand the transmission dynamics of onchocerciasis and its treatment. We consider a habitat with two interacting populations. The two populations are humans (hosts) and black flies (vectors). The total human population is partitioned into six compartments: the susceptible human compartment, , referring to individuals not infected with onchocerciasis but who are at risk of infection; the exposed compartment,
, referring to the individuals that have been exposed to onchocerciaisis through bites; the infectious compartment,
, referring to individuals with onchocerciaisis infection; susceptible humans undergoing ivermectin treatment,
; exposed humans undergoing ivermectin treatment,
; and the infectious humans undergoing ivermectin treatment,
. The black fly population is partitioned into three compartments: susceptible vectors,
, referring to black flies that have not picked up microfilariae but are at risk of picking up microfilariae during a blood meal; the exposed vector compartment,
, referring to black flies that have picked up microfilariae from an infected human during blood meal; and the infected vector compartment,
.
The total human and vector populations at any given time, t, are respectively given by;(1)
(1)
We assume that the transmission of onchocerciaisis in susceptible hosts is only through contact with an infectious vector. We also assume that susceptible vectors become infectious as a result of contact with infectious hosts during a blood meal. The population under study is assumed to be large enough to be modelled deterministically. The recruitment of new susceptible individuals and susceptible vectors is given by and
, respectively. Assuming
is the blackfly biting rate, that is, the average number of bites per black fly per unit, the rate of infection per susceptible black fly can be represented by
(2)
(2)
where q is the transmission probability from infectious human to black flies and is the modification parameter which measures the relative ability of individuals in class
to cause new infections relative to those in class
. We assume here that the individuals under treatment have a slightly lower probability to initiate new infections, thus,
. Assuming that the total number of bites made by black flies equals the number of bites received by humans, then the average number of bites per human receives per unit time is
. Assuming that the transmission probability per bite from infectious black flies to human is p, the rate of infection per susceptible human is given by
(3)
(3)
We then introduce and
parameters to simplify the infection rates per susceptible human and vector respectively. Individuals in class
progress to class
at rate
. Individuals undergoing ivermectin treatment in class
acquire infection at the rate
, where
defines the reduced effect of infection of the susceptible individuals as a result of treatment. Individuals in class
progress to class
at the rate
, where
is the reduced effect of progression to class
as a result of treatment. Immediately following treatment, infection in the human population is assumed to be reduced by some proportion
. This therefore results in a system of impulsive differential equations (Bainov & Simeonov, Citation1993; Lakshmikantham, Bainov, & Simeonov, Citation1989). It is assumed that individuals undergoing treatment relapse due to waning of the drug at the rate
. We include natural mortality rates given by
and
for the human and vector populations respectively.
We further assume that individuals under treatment have no immunity to the disease upon treatment. In addition, we assume that infected vectors and human do not recover from the infection during the modelling time. The dynamics in the host and vector populations are represented in the schematic diagram in Figure .
Figure 1. A compartmental representation of the epidemics and treatment of onchocerciasis. It is important to note that the model proposed here is an extension of the previous model studied in Omondi et al. (Citation2017).
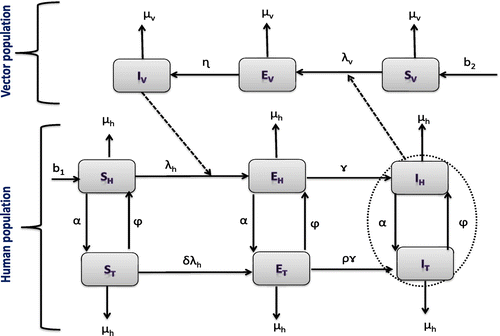
3.2. Model equations
The following system of non-linear ordinary differential equations, with non-negative initial conditions, describes the dynamics of onchocerciasis epidemics:(4)
(4)
subject to the following initial conditions:(5)
(5)
The impulsive conditions are(6)
(6)
Treatment may occur at either fixed or non-fixed intervals.
4. System without impulses
4.1. Well-posedness
System (Equation4(4)
(4) ) describes a human-vector population, so it is therefore necessary to prove that the solutions of system (Equation4
(4)
(4) ) with non-negative initial conditions remain non-negative for all time
and are bounded in the region
(7)
(7)
Theorem 4.1
Let . Then the solutions
of the system (Equation4
(4)
(4) ) are positive for all
. In addition, the region
is positively invariant and all solutions starting in
approach, enter or stay in
.
Proof
Let . Thus
. Then, from the first equation of our system (Equation4
(4)
(4) ), we obtain
Similarly, one can show that . This completes the proof for positivity.
The total population of the humans N and that of the vector population V is governed by(8)
(8)
Hence(9)
(9)
Therefore, N(t) and V(t) are bounded and the domain of biological significance is positively invariant and attracting. Thus, all solutions starting in remain in
.
4.2. Maximal solution
Next, we overestimate the number of infectious individuals in the population. From the system (Equation4(4)
(4) ), we have established that the total human (host) population is bounded above by
. Thus, from the fifth equation of the system (Equation4
(4)
(4) ) we have
If we bound by the total human population and
by the total vector population, we have
(10)
(10)
Solving the inequality in (Equation10(10)
(10) ), we obtain
(11)
(11)
As t approaches infinity, then the solution in (Equation11(11)
(11) ) is bounded by
(12)
(12)
Substituting the expression in (Equation12(12)
(12) ) into the sixth equation of the system (Equation4
(4)
(4) ), we have
whose solution can be expressed as(13)
(13)
Using the solutions obtained in (Equation12(12)
(12) ) and (Equation13
(13)
(13) ) in the second and third equations in the system (Equation4
(4)
(4) ) respectively, we have
(14)
(14)
(15)
(15)
The significance of these inequalities is that they overestimate the number of people with onchocerciasis in the population without necessarily solving the original systems of the differential equations. We will make use of these overestimates shortly.
4.3. Stability of the model equilibria
4.3.1. Disease-free equilibrium
The disease-free equilibrium for the system without impulses is given by(16)
(16)
4.3.2. The basic reproduction number
The basic reproduction number for the system (Equation4(4)
(4) ) is obtained using the next-generation method described in Van den Driessche and Watmough (Citation2002). It is given by
Note that this expression is a threshold, not necessarily the average number of secondary infections (Li, Blakeley, & Smith?, Citation2011).
Theorem 4.2
The disease-free equilibrium, , given in (Equation16
(16)
(16) ) of the system (Equation4
(4)
(4) ) is globally stable if
otherwise unstable.
Proof
Let be a candidate Lyapunov function. The constants
for
are non-negative. We can find the constants
such that the Lyapunov candidate is positive definite. The derivative of the Lyapunov function is given by
where
Setting the coefficients to to zero, we obtain
(17)
(17)
We then use the coefficients obtained in (Equation17(17)
(17) ) into the candidate Lyapunov function. The derivative of the resulting Lyapunov function becomes
(18)
(18)
We note that, whenever , then
. Therefore, by LaSalle’s Invariance principle (LaSalle, Citation1976), the disease-free equilibrium is globally stable whenever
.
4.3.3. Endemic equilibrium
The endemic equilibrium point, , is found by equating the right-hand of the system (Equation4
(4)
(4) ) to zero. Expressing all the variables in terms of
, we get
After some algebraic manipulations, we either have or
(19)
(19)
The case where , gives the disease-free equilibrium, treated earlier. From expression (Equation19
(19)
(19) ), one sees that when
, then
, implying that the system (Equation4
(4)
(4) ) has no positive solution. However, when
, then
and a unique endemic equilibrium exists.
Theorem 4.3
If , then
is unstable and the unique endemic equilibrium
is locally asymptotically stable in the interior of the feasible region
.
Proof
The stability and the direction of bifurcation of the endemic equilibrium is proved by the direct use of the Center Manifold Theory (CMT) as described in Castillo-Chavez and Song (Citation2004). We avoid re-stating the theorem and adopting notations as described in Castillo-Chavez and Song (Citation2004), we compute the values of
and
whose signs determine local dynamics of the model (Equation4
(4)
(4) ). The basic reproduction of the system (Equation4
(4)
(4) ) is established to be
(20)
(20)
Suppose, we choose as the bifurcation parameter so that when
, we have
(21)
(21)
In order to apply the Center Manifold Theory (CMT), it is necessary to make the following changes to the state variables, we let . The system (Equation4
(4)
(4) ) can now be written in the form
, where
. The system (Equation4
(4)
(4) ) therefore becomes
(22)
(22) The system (Equation22
(22)
(22) ) with the bifurcation point
, has a simple zero eigenvalue. Thus, it enables us to use the Center Manifold Theory to analyse the stability of the system (Equation22
(22)
(22) ) near
. Therefore, a right eigenvector w associated with zero eigenvalue has components
(23)
(23)
Similarly, the corresponding left eigenvector v associated with zero eigenvalue has components(24)
(24)
We now compute and
as outlined in Castillo-Chavez and Song (Citation2004). From the system (Equation22
(22)
(22) ), the non-zero partial derivatives of f(x) associated with
are given by
(25)
(25)
Thus, the expression for is given by
(26)
(26)
We finally compute the value of . The non-zero partial derivatives of f(x) associated with b is given by
(27)
(27)
Therefore the expression for is given by
(28)
(28)
Since, and
, we conclude from item (iv) of Center Manifold Theorem (Castillo-Chavez & Song, Citation2004) that the established endemic equilibrium
is locally asymptotically stable for
and there exists a positive unstable equilibrium. This completes the proof.
5. Model with impulses
In this section, we present and analyse the system with pulse treatment of onchocerciasis with ivermectin. Treatment occurs every six months. We, therefore, first examine the case of fixed times , with individuals treated at rate
. This results in system of impulsive differential equations (Bainov & Simeonov, Citation1993; Lakshmikantham, Bainov, & Simeonov, Citation1989; Samoilenko, Perestyuk, & Chapovsky, Citation1995). The administration of ivermectin is thus approximated by an instantaneous change.
5.1. General solution
Here, we overestimate the number of people infected with onchocerciasis in the population. Suppose the maximal number of individuals infected with onchocerciasis is given by inequality (Equation15(15)
(15) ). Then the one-dimensional impulsive differential equation given by
(29)
(29)
overestimates the number of infected individuals.
Letting for notational simplicity, from (Equation29
(29)
(29) ) we have
Let and denote
and
. Here,
is the population proportion of infected individuals immediately before the impulse and
is the value immediately after the impulse. Hence, for a single impulsive cycle
, we have
It therefore follows that(30)
(30)
The solution obtained in (Equation30(30)
(30) ) at the impulse points satisfies
Thus, the general solution becomes(31)
(31)
The general solution obtained in (Equation31(31)
(31) ) defines the maximal number of people with onchocerciasis immediately before the mass treatment with ivermectin. This solution depends on the human and vector recruitment rates, the human-vector transmission contact rate, the incubation period in the human, the waning rate of ivermectin, human and vector death rates, treatment times and the treatment effectiveness. A similar approach can be employed to calculate the maximal number of latently infected individuals using the overestimate obtained in (Equation14
(14)
(14) ). For fixed mass administration of ivermectin, (Equation31
(31)
(31) ) does not depend on time.
5.2. Fixed administration of ivermectin
For a fixed time period , we have
Thus(32)
(32)
The solution obtained in (Equation32(32)
(32) ) is the maximum population of the infected individuals. Note that
implying that the total number of infected humans shrinks to zero as the frequency of mass treatment with ivermectin increases (although note that the impulsive assumptions would break down in this limit). In order to keep the infected individuals under a threshold , we have
which implies that(33)
(33)
The expression in (Equation33(33)
(33) ) gives the maximum period of mass administration of ivermectin in the population to keep the infection of onchocerciasis below
. If we restrict
to
, then the disease can be controlled below the threshold
(but not necessarily eradicated).
5.3. Non-fixed administration of ivermectin
In this section, we consider a situation in which the mass administration of ivermectin is carried out at non-fixed times. However, this would require that the entire history of mass drug administration with ivermectin is known, which is unlikely to be the case (Figure ). Thus, we will assume that mass drug administration with ivermectin occurring more than two events previously has a negligible effect on the number of currently treated individuals. That is,
It then follows from (Equation30(30)
(30) ) that
The above inequality can be approximated as(34)
(34)
The expression in (Equation34(34)
(34) ) gives the number of infected humans that will be below
based on knowing the two previous mass administration times. Then the “next best” mass drug administration with ivermectin satisfies the following:
Next, we compare fixed and non-fixed mass drug administration with ivermectin. Assuming that the mass treatment times in the non-fixed case are constant, , then from (Equation33
(33)
(33) ), we have
(35)
(35)
(36)
(36)
(37)
(37)
If there is no impulse, then from (Equation29(29)
(29) ), we have
. Thus, we can assume that
. Hence,
(38)
(38)
therefore, . Assuming that non-fixed mass treatment with ivermectin occurs indefinitely and further letting
, then the minimum treatment effectiveness satisfies
(39)
(39)
If , then it follows that (39) becomes
The larger root exceeds 1 and hence can be discounted. It follows that the smaller root , satisfies
as stated in (Equation38
(38)
(38) ). This implies that the mass treatment is only effective in the range
.
6. Numerical simulations
In this section, we carry out parameter estimation, sensitivity analysis of the model parameters and numerical simulations for system (Equation4(4)
(4) ) to demonstrate the theoretical results. The simulations are performed using the fourth order Runge-Kutta scheme in Matlab 2014a with the set of parameter values given in Table . We consider the parameters in realistic ranges with guidance from past literature on onchocerciasis epidemics in Ghana.
6.1. Parameter estimation
We consider average parameter values that encompass features of onchocerciasis disease including the rate of infection, the incubation period and the length of infections period in the vector and host populations. Although estimates of some parameters are given in Table , here we give additional explanations and descriptions of some of the parameters.
(1) | The average birthrate in Ghana was estimated to be 31.09 births/1,000 population in 2015 and 30.60 births/1,000 population in 2014 according to the World Fact Book by Central Intelligence Agency (Citation2016) and Ghana Statistical Service report on Demographic and Health Survey (Citation2016), respectively. Owing to unchecked immigration, the recruitment rate is, therefore, estimated to be in the range | ||||
(2) | The natural human death rate is estimated based on average life of 50–70 years in accordance with Central Intelligence Agency data and 2014 demographic data released by Ghana Statistical Service estimates of life expectancy at birth (Central Intelligence Agency: The World Fact Book, Citation2016; Ghana Statistical Service, Citation2016). The average black fly lifespan is 2–3 weeks (Hopkins & Boatin, Citation2011; Simulium spp., Citation2016). | ||||
(3) | It takes | ||||
(4) | The modification parameters ( | ||||
(5) | The mass administration rate |
Table 1. Estimated parameter values in the model for onchocerciasis case. The rates are given per day
6.2. Sensitivity analysis
Sensitivity analysis is the study of how uncertainty in the output of a system can be apportioned to different sources of uncertainty in the model input (Saltelli et al., Citation2008a, Citationb). It is a technique for systematically varying model inputs and determining their effect on the model output. We perform sensitivity analysis in order to investigate the contribution of vital parameters on the model dynamics, specifically to establish the parameters that have significant influence on the onchocerciasis dynamics. We use Latin Hypercube Sampling (LHS), a stratified Monte Carlo sampling scheme applicable to many parameters from a multidimensional distribution (Saltelli et al., Citation2008a, Citationb). It allows for simultaneous determination of an unbiased estimate of the model output for a given set of model input. We perform the sensitivity analysis by computing the Partial Rank Correlation Coefficients (PRCCs) for each parameter value, sampled by the LHS scheme (Blower & Dowlatabadi, Citation1994). Parameters with positive PRCCs will increase when they are increased, whereas parameters with negative PRCCs will decrease
when they are increased. The outcome is the reproduction number
derived from the theoretical model. We determine PRCCs with 1000 simulations per run to determine parameters that have a significant influence on
.
Figure 3. Tornado plots of partial rank correlation coefficients (PRCCs) of the parameters that influence for the input parameters using the values in Table . Parameters with PRCC
increase
when they are increased whereas parameters with PRCC
decrease
when they are increased.
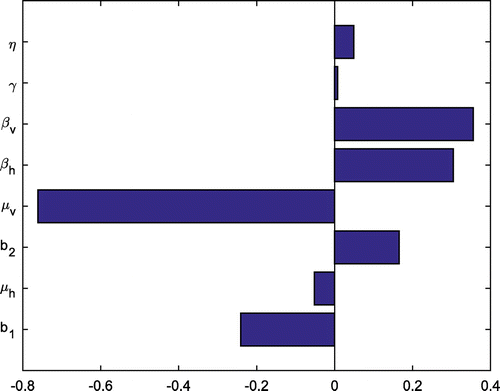
The PRCCs results in Figure illustrate the degree of the effect that each parameter has on the outcome. The three parameters with the most influence on are the transmission contact rate in humans,
, the vector transmission contact rate,
, and the vector death rate
.
Figure displays 1000 Monte Carlo simulations for the input parameters with the most effect on the basic reproduction number. Scatter plots show that is monotone increasing with increasing values of
and
and monotone decreasing with increasing vector mortality rate,
. However,
is only guaranteed to be less than unity when the human–vector and vector-human contact rates are sufficiently small. These results also suggest that, even if vector death rate is extremely high, eradication is not possible.
Figure 4. The Monte Carlo simulations for the three parameters with the greatest influence on the : the transmission contact rate in humans, the transmission contact rate in the vector and the vector death rate for the input parameters using the values in Table and 1,000 simulations per run. Eradication is only possible if the transmissibilities are extremely small or if the vector death rate is extremely high.
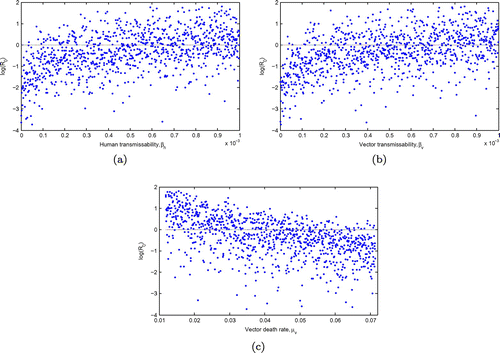
In order to gain more insight into the three parameters with the greatest influence on the basic reproduction number, , all other parameters were fixed at their sample values. A surface plot for
is shown in Figure .
6.3. Simulation results
The results presented in this section demonstrate the dynamics of onchocerciasis for the system (Equation4(4)
(4) ) obtained using Matlab ODE45 solver, which employs simultaneously the fourth and fifth order Runge-Kutta schemes. Unless otherwise stated, parameters are as stated in Table . We give results of system (Equation4
(4)
(4) ) with impulses for both the cases when
and when
as shown in Figures – and Figures –, respectively. In addition, we numerically make a comparison between fixed and non-fixed mass administration of ivermectin in the treatment of onchocerciasis. In order to illustrate the effectiveness of ivermectin, we vary the reduction rate
for both the cases of
.
First, we examine the behaviour of the system (Equation4(4)
(4) ) when the basic reproduction number,
, is greater than unity for the fixed and non-fixed mass administration of ivermectin. It is seen in Figure that when the effectiveness of ivermectin is low, that is,
, the disease spreads in the population. However, the results in Figure show that the endemicity of the disease is reduced when the effectiveness rate of mass administration of ivermectin is increased. We also observe that fixed mass treatment yields a better outcome compared to non-fixed mass administration.
We then examine the case when the basic reproduction number is below unity for both the fixed and non-fixed mass drug administration with ivermectin. We examine two cases of : when
is close to unity and where
is significantly less than unity. We observe in Figure that when
is less than but close to one, the disease may persist in the population when the value of
is small. However an increment in the value of
moves the system towards a disease-free state, but there is no eradication. This is shown in Figure with
. The results in Figure show the dynamics of the population under both fixed and non-fixed mass administration of ivermectin at
keeping the interval
days (6 monthly interval) for fixed mass administration of ivermectin. We observe that the system with impulses attains its disease-free state, suggesting that onchocerciasis dies out. These results are consistent with theorem (). However, when the ivermectin effectiveness is increased, the system attains the disease free equilibrium much faster as shown in Figure .
Figure 7. System behaviour for fixed and non-fixed mass administration of ivermectin with . Note that increasing
improves the outcome but does not lead to eradication.
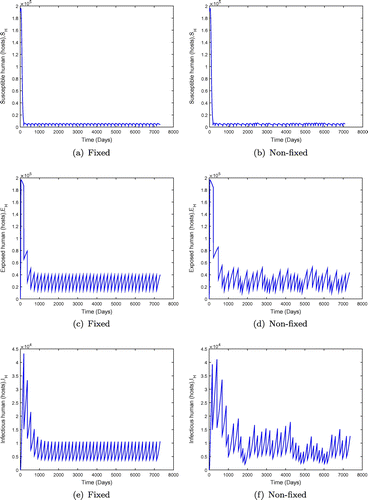
Figure 8. System behaviour for fixed and non-fixed mass administration of ivermectin with . Non-fixed adminsitration may produce lower overall numbers of infected individuals, but the outcome is not predictable.
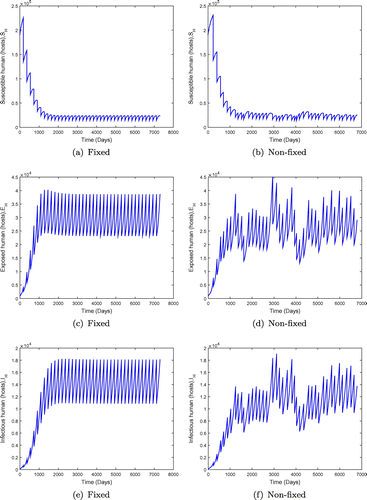
Figure 9. System behaviour for fixed and non-fixed mass administration of ivermectin with . Non-fixed administration may produces bursts of infection, even if the disease would be otherwise kept at low levels.
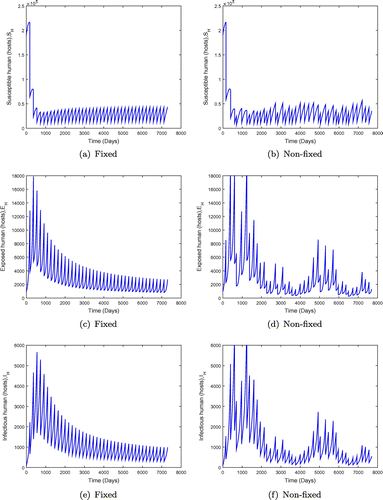
7. Conclusion
Onchocerciasis is one of the neglected tropical diseases that is still to be eradicated. In connection with the WHO’s plan of onchocerciasis elimination, mass drug administration with ivermectin is the most efficient approach towards this effort. This is spearheaded by APOC in Sub-Saharan Africa where the disease is endemic. In order to investigate the effectiveness of ivermectin in the control of onchocerciasis, we formulated a deterministic model that captures the dynamics of this disease in humans and blackflies. The analysis of the model without pulse mass administration of ivermectin shows that, the disease-free state exists and is stable if . On the other hand, if
, the disease-free state loses its stability and the system tends towards the endemic state. Then, sensitivity analysis, we have computed the Partial Rank Correlation Coefficients between
and each parameter of the model. The results show that the parameters
, the transmission contact of infection from an infected vector to a susceptible human and
, the transmission of infection from an infected human to a susceptible vector, have the highest influence on
. The other parameter with an important effect
, the vector removal rate.
We have provided the estimates for the necessary frequency and strength of mass drug administration with ivermectin. We may conclude that the disease can be controlled through mass drug administration with ivermectin with needed frequency and high strength of ivermectin. Furthermore, our results suggest that reducing human-vector contact can be one way of reducing but not eradicating onchocerciasis from the population. This can be done by implementing personal protection practices such as wearing insect repellent, wearing long sleeves and long pants during the day when blackflies bite. In addition, application of insecticides can be effective in reducing the presence of vector in the environment.
To assess the impact of combination of different controls, we have conducted several simulations, using the called “pulse control" technique. We have observed from our numerical results that the eradication of onchocerciasis is possible with tolerable regularity of mass drug administration with ivermectin provided the basic reproduction number is kept (far) below unity. We can conclude that, in the presence of mass administration of ivermectin with six month intervals, the disease can be controlled. Furthermore, if the frequency of mass administration is increased, the system moves towards a better outcome. Our modelling results show that mass administration of ivermectin at regular interval is an effective method of onchocerciaisis eradication if other parameters can be sufficiently controlled. However, emerging new treatment options as suggested in Kuesel (Citation2016), particularly moxidectin which is similar but more efficacious than ivermectin, present promising alternatives (Awadzi, Opoku, Attah, Lazdins-Helds, & Kuesel, Citation2014; Turner et al., Citation2015).
The model presented in this paper has a number of limitations. Mass administration of ivermectin is assumed to occur instantaneously. However, in reality, there is usually a small delay as mass treatment reaches it maximum coverage. It is important to note that the delays do not affect the impulsive assumptions, provided the time interval of mass administration of ivermectin is significantly larger than the instantaneous approximation. Unavailability of experiential data poses a challenge for model verification. Despite the shortcomings, the model still provides some useful insights into the control of onchocerciasis through the implementation of regular mass administration of ivermectin in conjunction with transmission reduction and the implementation of vector control measures.
Acknowledgements
The authors are grateful to the University of Stellenbosch, DST/NRF South African Centre for Epidemiological Modelling and Analysis (SACEMA) and the African Institute for Mathematical Sciences (AIMS) for their support during the preparation of the manuscript. RJS? is supported by an NSERC Discovery Grant. For citation purposes, please note that the question mark in “Smith” is part of his name.
Additional information
Funding
Notes on contributors
E.O. Omondi
E.O. Omondi is currently pursuing his PhD in mathematics at Strathmore University in Kenya. He holds Bsc degree in Applied Statistics with Computing from the University of Eldoret in Kenya, Msc in Mathematical Sciences from African Institute for Mathematical Sciences (AIMS), South Africa and Masters of science (Msc) in Mathematics from University of Stellenbosch in South Africa. His area of interest includes biomathematics and applied statistics.
F. Nyabadza
F. Nyabadza is is a professor in the Department of Mathematical Sciences of Stellenbosch University, South Africa. He has written many research articles and attended several international conferences and seminars. He has supervised several master’s and PhD students as well as post-docs. His area of interest includes Mathematical Epidemiology and specifically the application of mathematics to disease dynamics. He focuses on control dynamics and the investigation of the impact of interventions in human diseases for the purpose of informing public health policy.
R.J. Smith?
R.J. Smith note that the question mark is part of his name) is a professor in Department of Mathematics and Statistics of University of Ottawa in Canada. He has many research articles and attended several international conferences and seminars. He has supervised several masters and PhD students as well as post-docs. His fields of interest include Mathematical modelling of infectious diseases and Impulsive differential equations.
References
- Omondi, E. O. . (2016). A mathematical model for onchocerciasis and its treatment with ivermectin (Master’s thesis), Stellenbosch: Stellenbosch University.
- Alley, W. S. , van Oortmarssen, G. J. , Boatin, B. A. , Nagelkerke, N. J. , Plaisier, A. P. , Remme, J. H. (2001). Macrofilaricides and onchocerciasis control, mathematical modelling of the prospects for elimination. BMC Public Health , 1 (1), 12.
- Animal Diversity Web . (2016). Onchocerca volvulus. . Retrieved May 2016, from http://animaldiversity.org/accounts/Onchocercavolvulus/
- Awadzi, K. , Opoku, N. O. , Attah, S. K. , Lazdins-Helds, J. , & Kuesel, A. C. (2014). A randomized, single-ascending-dose, ivermectin-controlled, double-blind study of moxidectin in Onchocerca volvulus infection. PLoS Neglected Tropical Diseases , 8 , e2953.
- Bainov, D. , & Simeonov, P. (1993). Impulsive differential equations: Periodic solutions and applications (Vol. 66). Boca Raton, FL: CRC Press.
- Barrett, A. D. , & Stanberry, L. R. (2009). Vaccines for biodefense and emerging and neglected diseases . Cambridge, MA: Academic Press.
- Basáñez, M. G. , & Boussinesq, M. (1999). Population biology of human onchocerciasis. Philosophical Transactions of the Royal Society of London B: Biological Sciences , 354 , 809–826.
- Basáñez, M. G. , & Ricárdez-Esquinca, J. (2001). Models for the population biology and control of human onchocerciasis. Trends in Parasitology , 17 , 430–438.
- Basáñez, M. , Walker, M. , Turner, H. , Coffeng, L. , de Vlas, S. , & Stolk, W. (2016). Chapter five-river blindness: Mathematical models for control and elimination. Advances in Parasitology , 94 , 247–341.
- Blower, S. M. , & Dowlatabadi, H. (1994). Sensitivity and uncertainty analysis of complex models of disease transmission: An HIV model, as an example. International Statistical Review/Revue Internationale de Statistique , 229–243.
- Castillo-Chavez, C. , & Song, B. (2004). Dynamical models of tuberculosis and their applications. Mathematical Biosciences and Engineering , 1 , 361–404.
- Central Intelligence Agency: The World Fact Book . (2016). The world of a nation: The center of intelligence. Retrived March 2016, from https://www.cia.gov/library/publications/the-world-factbook/fields/2054.html
- Coffeng, L. E. , Truscott, J. E. , Farrell, S. H. , Turner, H. C. , Sarkar, R. , Kang, G. , ... Anderson, R. M. (2017). Comparison and validation of two mathematical models for the impact of mass drug administration on Ascaris lumbricoides and hookworm infection. Epidemics , 18 , 38–47.
- Cox, F. E. (2002). History of human parasitology. Clinical Microbiology Reviews , 15 , 595–612.
- Crosskey, R. W. (1990). The natural history of blackflies . Hoboken, NJ: John Wiley and Sons.
- Simulium spp. (Insecta: Diptera: Simuliidae) . (2016). Featured creatures . Retrieved May 2016, from http://entnemdept.ufl.edu/creatures/livestock/bfly.htm
- Feasey, N. , Wansbrough-Jones, M. , Mabey, D. C. , & Solomon, A. W. (2009). Neglected tropical diseases. British Medical Bulletin , 93 , 179–200.
- Filipe, J. A. , Boussinesq, M. , Renz, A. , Collins, R. C. , Vivas-Martinez, S. , Grillet, M. E. , ... Basáñez, M. G. (2005). Human infection patterns and heterogeneous exposure in river blindness. Proceedings of the National Academy of Sciences of the United States of America , 102 , 15265–15270.
- Gardon, J. , Boussinesq, M. , Kamgno, J. , Gardon-Wendel, N. , & Duke, B. O. (2002). Effects of standard and high doses of ivermectin on adult worms of Onchocerca volvulus: A randomised controlled trial. The Lancet , 360 , 203–210.
- Golden, A. , Faulx, D. , Kalnoky, M. , Stevens, E. , Yokobe, L. , Peck, R. , & Gantin R. G , (2016). Analysis of age-dependent trends in Ov16 igG4 seroprevalence to onchocerciasis. Parasites & Vectors , 9 , 338.
- Gonzalez, R. J. , Cruz-Ortiz, N. , Rizzo, N. , Richards, J. , Zea-Flores, G. , Domínguez, A. , ... Richards, F. O., Jr (2009). Successful interruption of transmission of Onchocerca volvulus in the Escuintla-Guatemala focus. Guatemala, PLoS Negl Trop Dis , 3 , e404.
- Guillet, P. , Seketeli, A. , Alley, E. , Agoua, H. , Boatin, B. , Bissan, Y. , ... Samba, E. M. (1995). Impact of combined large-scale ivermectin distribution and vector control on transmission of Onchocerca volvulus in the Niger basin, Guinea. Bulletin of the World Health Organization , 73 , 199.
- Ghana Statistical Service . (2016). Demographic and health survey. Retrieved March 2016, from http://www.statsghana.gov.gh/publications.html
- Global Health Observatory (GHO) Data . (2016). Onchocerciasis situation and trend. Retrieved April 2016, fromhttp://www.who.int/gho/neglected_diseases/onchocerciasis/en/
- Hopkins, A. , & Boatin, B. A. (2011). Onchocerciasis. Water and Sanitation-Related Diseases and the Environment: Challenges, Interventions, and Preventive Measures , 133–149.
- How is Onchocerciasis Spread? . (2016). Onchocerciasis . Retrieved April 2016, from http://www.medic8.com/infectious-diseases/onchocerciasis.htm
- Kealey, A. (2010). Neglected tropical diseases: infection, modeling, and control. Journal of Health Care for the Poor and Underserved , 21 , 53–69.
- Kuesel, A. C. (2016). Research for new drugs for elimination of onchocerciasis in Africa. International Journal for Parasitology: Drugs and Drug Resistance , 6 , 272–286.
- Lakshmikantham, V. , Bainov, D. D. , & Simeonov, P. S. (1989). Theory of impulsive differential equations. World Scientific , 6 .
- LaSalle, J.P. (1976). The stability of dynamical systems. SIAM , 25
- Li, J. , Blakeley, D. , & Smith?, R. (2011). The failure of.. Computational and Mathematical Methods in Medicine , 2011 , Article ID 527610
- Lont, Y. L. , Coffeng, L. E. , de Vlas, S. J. , Golden, A. , de los Santos, T. , Lont, Y. L. , ... Stolk, W. A. (2017). Modelling anti-Ov16 igg4 antibody prevalence as an indicator for evaluation and decision making in onchocerciasis elimination programmes. PLoS Neglected Tropical Diseases , 11 , e0005314.
- Lovato, R. , Guevara, A. , Guderian, R. , Proaño, R. , Unnasch, T. , Criollo, H. , ... Mackenzie, C. D. (2014). Interruption of infection transmission in the onchocerciasis focus of Ecuador leading to the cessation of ivermectin distribution. PLoS Neglected Tropical Diseases , 8 , e2821.
- Lustigman, S. , MacDonald, A. J. , & Abraham, D. (2003). CD4+-dependent immunity to Onchocerca volvulus third-stage larvae in humans and the mouse vaccination model: common ground and distinctions. International Journal for Parasitology , 33 , 1161–1171.
- Mopecha, J. P. , & Thieme, H. R. (2003). Competitive dynamics in a model for Onchocerciasis with cross-immunity. Canadian Applied Mathematics Quarterly , 11 , 339–376.
- Nguyen, J. C. , Murphy, M. , Nutman, T. B. , Neafie, R. C. , Burke, D. S. , & Turiansky, C. (2005). Cutaneous onchocerciasis in an American traveler. International Journal of Dermatology , 44 , 125–128.
- Oguoma, I. C. , & Acho, T. M. (2014). Mathematical modelling of the spread and control of onchocerciasis in tropical countries: Case study in Nigeria. In Abstract and Applied Analysis (Vol. 2014). Cairo: Hindawi Publishing Corporation.
- OHanlon, S. J. , Slater, H. C. , Cheke, R. A. , Boatin, B. A. , Coffeng, L. E. , Pion, S. D. , ... Basáñez, M. G. (2016). Model-based geostatistical mapping of the prevalence of Onchocerca volvulus in West Africa. PLoS Neglected Tropical Diseases , 10 , e0004328.
- Omondi, E. O. , Orwa, T. O. , & Nyabadza, F. (2017). Application of optimal control to the onchocerciasis transmission model with treatment. Mathematical Biosciences .
- Omondi, E. O. , Nyabadza, F. , Bonyah, E. , & Badu, K. (2017). Modeling the infection dynamics of onchocerciasis and its treatment. Journal of Biological Systems , 25 , 247–277.
- Onchocerciasis (On-kough-sir-ky-a-sis) . (2016). Parasitic diseases . Retrieved May 2016, from http://www.wellnessproposals.com/health-care/handouts/parasitic-zoonotic-diseases/onchocerciasis-factsheet.pdf
- Plaisier, A. , Alley, E. , Van Oortmarssen, G. , Boatin, B. , & Habbema, J. (1997). Required duration of combined annual ivermectin treatment and vector control in the onchocerciasis control programme in West Africa. Bulletin of the World Health Organization , 75 , 237.
- Poolman, E. M. , & Galvani, A. P. (2006). Modeling targeted ivermectin treatment for controlling river blindness. The American Journal of Tropical Medicine and Hygiene , 75 , 921–927.
- Remme, J. , De Sole, G. , & Van Oortmarssen, G. (1990). The predicted and observed decline in onchocerciasis infection during 14 years of successful control of Simulium spp. in West Africa. Bulletin of the World Health Organization , 68 , 331.
- Saltelli, A. , Ratto, M. , Andres, T. , Campolongo, F. , Cariboni, J. , Gatelli, D. , ... Tarantola, S. (2008a). Global sensitivity analysis: The primer . Hoboken, NJ: John Wiley and Sons.
- Saltelli, A. , Ratto, M. , Andres, T. , Campolongo, F. , Cariboni, J. , Gatelli, D. , ... Tarantola, S. (2008b). Introduction to sensitivity analysis, Global sensitivity analysis. The Primer , 1–51.
- Samoilenko, A. M. , Perestyuk, N. , & Chapovsky, Y. (1995). Impulsive differential equations (Vol. 14). Singapore: World Scientific.
- Traoré, S. , Wilson, M. , Sima, A. , Barro, T. , Diallo, A. , Aké, A. , ... Mas, J. (2009). The elimination of the onchocerciasis vector from the island of bioko as a result of larviciding by the WHO African Programme for Onchocerciasis control. Acta Tropica , 111 , 211–218.
- Turner, H. C. , Basáñez, M. G. , Walker, M. , & Churcher, T. S. (2014). Modelling the impact of ivermectin on river blindness and its burden of morbidity and mortality in African Savannah: Epioncho projections. Parasit Vectors , 7 , 241.
- Turner, H. C. , Walker, M. , Attah, S. K. , Opoku, N. O. , Awadzi, K. , Kuesel, A. C. , & Basáñez, M. G. (2015). The potential impact of moxidectin on onchocerciasis elimination in Africa: an economic evaluation based on the Phase II clinical trial data. Parasites & Vectors , 8 , 167.
- Turner, H. C. , Walker, M. , Lustigman, S. , Taylor, D. W. , & Basáñez, M. G. (2015). Human onchocerciasis: Modelling the potential long-term consequences of a vaccination programme. PLoS Negl Trop Dis , 9 , e0003938.
- Van den Driessche, P. , & Watmough, J. (2002). Reproduction numbers and sub-threshold endemic equilibria for compartmental models of disease transmission. Mathematical Biosciences , 180 , 29–48.
- Walker, M. , Stolk, W. A. , Dixon, M. A. , Bottomley, C. , Diawara, L. , Traoré, M. O. , ... Basáñez, M. G. (2017). Modelling the elimination of river blindness using long-term epidemiological and programmatic data from Mali and Senegal. Epidemics , 18 , 4–15.
- Winnen, M. , Plaisier, A. , Alley, E. , Nagelkerke, N. , Van Oortmarssen, G. , Boatin, B. , & Habbema, J. (2002). Can ivermectin mass treatments eliminate onchocerciasis in Africa? Bulletin of the World Health Organization , 80 , 384–391.
- World Health Organization . (2012). Accelerating work to overcome the global impact of neglected tropical diseases: A roadmap for implementation: Executive summary .