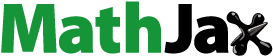
Abstract
PM2.5 and PM2.5–10 aerodynamic diameters (x ≤ 2.5 μm) and (2.5 ≥ x ≤ 10 μm) fractions in six Nigerian urban cities (Aba, Abuja, Lagos, Kano, Maiduguri and Port-Harcourt), were monitored using “Gent” stacked filter unit sampler in order to assess elemental concentrations and to perform source apportionment. Twenty-three elements; (Na, Mg, Al, Si, P, S, Cl, K, Ca, Ti, V, Cr, Mn, Fe, Ni, Cu, Zn, Se, Br, Rb, Sr, Zr and Pb) were analysed with Ion beam analysis (IBA) based proton induced X-ray emission (PIXE) technique. In addition, enrichment factors (EF) and pollution indices (PI) calculations were performed on the elemental concentration data. Results indicated that the elemental concentration varied across the receptor sites with some elements higher than World Health Organization (WHO) guideline values. PM2.5–10 ranged from 9 to 45,243 ng m−3 (Aba), 1 to 281 ng m−3 (Abuja), 5 to 3,936 ng m−3 (Kano), 6 to 3,872 ng m−3 (Lagos), 6 to 19,106 ng m−3 (Maiduguri) and 4 to 7,962 ng m−3 (Port Harcourt) while PM2.5 ranged from 3 to 7,089 ng m−3 (Aba), 1 to 139 ng m−3, 2 to 480 ng m−3, 1 to 153 ng m−3, 1 to 427 ng m−3 and 1 to 1,051 ng m−3 (Port Harcourt). Principal Component Analysis resolved three (soil dust 50%; sea spray 22% and industrial 15%) and four components (soil dust 42%; biomass burning 24%; petroleum products combustion (15%) and industrial emission 5%) as PM2.5–10 and PM2.5 sources, respectively.
Public Interest Statement
It is quite clear from the limited studies available that emerging urban cities in West Africa constitute a huge source of anthropogenic air pollutants and Nigeria have been reported to rank in the top; obviously due to increasing population, economic growth and industrialization. Unfortunately, lack of in situ measurement of ambient air pollutants poses a significant setback for regulators and policy-makers. This challenge prompted us to commence a national screening exercise on one of the criteria pollutants (PM) in six Nigerian potential megacities (Aba, Abuja, Lagos, Kano, Maiduguri, and Port-Harcourt). Chemical signatures of the analysed PM samples indicated that soil dust was prevalent across the country in addition to fingerprints of biomass burning and industrial emission.
1. Introduction
Urban cities in Africa are known to experience elevated levels of particulate matter (PM) pollution (Obioh et al., Citation2013). Nowadays PM have received considerable research interest due to its association with adverse respiratory health impacts, its role in atmospheric photochemistry and chemical composition data could lead to source identification and quantification (Krzyzanowski & Cohen, Citation2008; World Health Organization, Citation2006). Accelerator-based ion beam analysis (IBA) techniques such as proton induced X-ray emission (PIXE) experiment have been applied to the studies of chemical composition of atmospheric PM for many years (Cohen et al., Citation2004). They are well suited to such studies as they have several unique advantages; they have multi-elemental analysis capabilities, low minimum detection limits for a very broad range of elements in the Periodic Table and can quantitatively detect pictograms of material in micrograms of sample (Ezeh, Obioh, Asubiojo, & Abiye, Citation2012).
PM could be formed directly from a primary source such as fossil fuel combustions, industrial activities, soil dust, sea spray, biogenic emissions or indirectly through the conversion of gaseous emissions to the atmosphere from anthropogenic or natural sources. However, sole dependence on biomass energy and other agricultural activities have increased emissions of PM within local regions and due to dynamic nature of air inadvertently have also led to elevated PM concentration in urban areas (Arku et al., Citation2008). As a result, PM are complex mixtures whose chemical compositions presents both challenges and opportunities regarding understanding of its physical and chemical processes and elucidating sources of ambient PM pollution.
Research works into the sources and causes of high PM levels is needed to understand and regulate the factors that contribute to reduced air quality. Source-receptor relationships for particulate matter can be quantified using a number of techniques. Receptor modelling in contrast to emission inventory provides a reliable method to evaluate the relative contributions of different sources based on measurements at receptor sites, associated chemical composition data and source emission chemical profiles. However, most receptor models such as chemical mass balance (CMB) require knowledge of source profile data which are not locally available in most developing nations, thus model output is usually underestimated or overestimated. Again, positive matrix factorization model which does not require knowledge of source profile data requires large data (are often difficult and expensive to obtain) to yield explainable model results. To address these challenges, principal component analysis (PCA) requires relatively small data matrix and no knowledge of local source profiles could be employed (Ezeh, Obioh, & Asubiojo, Citation2015). CMB uses numerical approach to account for statistical variances by deriving the least number of major factors or sources (Chan & Mozurkewich, Citation2007). PCA model classifies elements according their concentration variances implying that the grouped elements are associated with each other and reduces the number of variables that explains the observed variations in the data-set to a minimum. The study utilized the external-beam set-up for PIXE experiment available at Department of Physics and Astronomy, and Instituto Nazionale di Fisica Nucleare laboratory of University of Firenze, Italy for the elemental compositions of PM2.5 and PM2.5–10 collected in Nigerian cities. The data sets obtained from elemental characterization were further analysed with PCA for source identification and apportionment.
2. Methodology
2.1. Study area
Six cities (Figure ) representing six geographical spreads in Nigeria were selected for the study which was conducted between September and October, 2009. The cities were: Aba (5° 07ʹ N, 7° 22ʹ E) a predominantly commercial city located in the south-east region; Abuja (9° 46ʹ N, 7° 22ʹ E), the seat of federal government of Nigeria and represents north-central region; Kano (12° 00ʹ N, 8° 31ʹ E), located in northwest region of Nigeria which is the centre for commerce and industry for Northern Nigeria; Lagos (6° 27ʹ N, 3° 23ʹ E), a coastal city located in south-west region, it has the highest population density (≈ 2,400 person per km2) in Nigeria. Lagos is also the economic and commercial nerve centre of Nigeria. Maiduguri (11° 30ʹ N, 13° 00ʹ E), representing north-east region which is bounded in the North by Chad Republic and in the east by Cameroon, is the most developed city in the north-east region of Nigeria; Port Harcourt (4° 75ʹ N, 7° 00ʹ E) a highly urbanized and industrialized city located in the southern region lies on the coast of Atlantic Ocean. In each of these cities, at least four sampling sites were used as receptor sites due to their reflection of variations in urban infrastructures such as high-density residential (HDR), low-density residential (LDR), industrial (IND), and commercial (COM) features.
2.2. Samplings
Samplings were performed with a “Gent” (M250, Schlumberger, Houston, USA) model stacked filter unit (Maenhaut, Francois, & Cafmeyer, Citation1993) sampler capable of collecting air particulate samples in PM2.5 (x ≤ 2.5 μm) and PM2.5–10 (2.5 ≥ x ≤ 10 μm). Samples were collected on pre-weighed and pre-conditioned Whatman® Nuclepore filters with flow rates between 16 and 18 L min−1. Conditioning consisted of keeping filters at about 25°C and 50% constant humidity for 24 h before exposure. Detailed sampling protocols have been documented (Obioh et al., Citation2013). Mass–balance analyses of exposed filters were achieved in triplicate measurements using a digital micro-weighing balance (Sartorius, Gottingen, Germany) which was calibrated using automated isoCAL function to ensure accuracy. PM10 equals the sum of PM2.5 and PM2.5–10 values. Exposed filters were stored in a desiccator until analysis.
2.3. Elemental analysis
For each PM2.5 and PM2.5–10 sample, elemental concentrations were determined by Particle Induced X–ray Emission (PIXE) technique in an external IBA set-up of 3 MV Tandetron accelerator at the Instituto Nationale di Fisica Nucleare Firenze, Italy (Calzolai, Chiari, Lucarelli, Nava, & Portarena, Citation2010; Calzolai et al., Citation2006). Calibration of PIXE system was performed by irradiating suitable certified thin target standards. Fluorescence X-rays from each sample were energy-analysed using two Silicon Drift Detectors (SDD), one optimized for low Z elements (Na–Ca) and the other for high Z elements (Ca and above). Detailed elemental concentrations were calculated as reported in Ezeh et al. (Citation2014). Twenty-three elements (Na, Mg, Al, Si, P, S, Cl, K, Ca, Ti, V, Cr, Mn, Fe, Ni, Cu, Zn, Se, Br, Rb, Sr, Zr and Pb) were sought for and their concentrations established.
2.4. Principal component analysis
To identify and apportion sources of particulate matter at study area, PCA of the total PM2.5 and PM2.5–10 collected in the cities under study were achieved using Statistical Package for Social Scientist (SPSS) software. Orthogonal transformation method with Varimax rotation was done to get a clearer pattern of components, without changing their relative positions. Thus, retaining principal components with eigenvalues greater one. Due to large numbers of missing concentration values, P and Cs were not used for PCA for PM2.5–10. Similarly, Zr, Rb and Cs were not used for PM2.5. Detailed methodology for performing PCA can be found (Chan & Mozurkewich, Citation2007).
2.5. Enrichment factors
Enrichment factor in metals is an indicator commonly used to assess the presence and intensity of anthropogenic contaminant deposition in the aerosol sample. Enrichment factors (EFs) were calculated by the normalization of one metal concentration in the sample with respect to concentration of a reference element. The EF was calculated as follows;(1)
(1)
where Cn (sample) is the concentration of the examined chemical element in the examined environment, Cref (sample) is the concentration of the examined chemical element in the reference environment, Bn is the concentration of the reference chemical element in the examined environment and Bref is the concentration of the reference element in the reference environment. In this work, crustal composition (Taylor, Citation1964) were used and Ti was used as the normalizing element because it is a crustal element with less anthropogenic influence. Elements with EF next to unity have a strong natural component while elements with high EF could have anthropogenic origin, or are due to other natural sources such as marine aerosols.
2.6. Pollution indices
The pollution level by a given element was evaluated with the single pollution index (PIi) calculated as the ratio between background concentrations (Ci) of elements in the sample and its reference value (Si) metals in continental crust.(2)
(2)
The pollution of the atmospheric particulate matter was classified into three classes; low (PI < 1), moderate (1 < PI ≤ 3) or high (PI > 3) for both size fractions based on dos-Anjos et al. (Citation2000).
3. Results and discussions
3.1. Trace element concentrations
Presented in Tables and are the concentrations of twenty-three (23) elements; Na, Mg, Al, Si, P, S, Cl, K, Ca, Ti, V, Cr, Mn, Fe, Ni, Cu, Zn, Br, Rb, Sr, Zr, Cs, Pb detected for PM2.5–10 and PM2.5 fractions, respectively. Elemental concentrations for PM2.5–10 samples accounted for 10 to 60% of the overall mass loads while about 7% was for PM2.5. This implies that bulk constituents of PM2.5 fraction was not analysed and could probably be elemental carbon or organic compounds as evidenced by black colour of the exposed filters. Measurement errors ranged from ±1 to ±10% for most species in both fractions. As expected, the range (10–60%) of elemental concentration contribution to PM2.5–10 is very large due to its large aerodynamic diameter of which markers of soil dust and sea spray are often far more prominent than markers for other sources.
Table 1. PM2.5–10 average elemental concentrations (ng m−3)
Table 2. PM2.5 average elemental concentrations (ng m−3)
For PM2.5–10 fraction, average concentrations of Al, Si, Ca, Ti and Fe were more pronounced in all the cities; 16,327, 45,243, 5,603, 1,677 and 12,253 ng m−3 (Aba); 133, 281, 85, 10 and 95 ng m−3 (Abuja); 1,588, 3,936, 1,276, 164 and 1,804 ng m−3 (Kano); 1,499, 3,872, 2,309, 181 and 1,579 ng m−3 (Lagos), 5,819, 19,106, 6,000, 602 and 4,110 ng m−3 (Maiduguri); 2,696, 7,962, 1,309, 255 and 1,964 ng m−3 (Port Harcourt), respectively. High concentrations of Al, Si, Ca, Ti and Fe could be due to soil dust which is expected for typical sub-Saharan African cities. Interestingly, Al had strong correlation (r2) of 0.99 with Si while Ca correlated with Fe was r2 = 0.77.
Al–Si ratio ranged from 0.30 (Maiduguri) to 0.47 (Abuja) and it agreed with 0.30 for Accra (Arku et al., Citation2008) and Hong Kong (Cohen et al., Citation2009). Ca–Fe ratios ranged from 0.46 (Aba) to 1.46 (Lagos) and were also close to values for Accra and Hong Kong. Again, soil dust markers were further vindicated by their low EFs (Table ) and were between zero and three the receptor sites studied. Sodium ranged from 21 ng m−3 (Abuja) to 566 ng m−3 (Aba) while Cl registered 12 ng m−3 (Abuja) to 876 ng m−3 (Lagos). Despite variations in Na and Cl concentrations, both elements were strongly correlated (r2 = 0.72) probably due to similar source origin. Evidence from EF values (Table ) revealed that Na could be associated to other sources than sea spray as it showed higher EFs compared to Cl. With exception of Abuja, average concentrations of Ni, V, Zn, Br Cu and Cr were similar, probably due to similar emission source type. Pb average concentration (56 ng m−3) in Maiduguri was slightly above guideline standard2 of 50 ng m−3 and could pose negative health impacts to humans.
Table 3. PM2.5–10 elemental EFs and PIs
PM2.5 average elemental concentrations for the cities under study are presented in Table . Again, soil dust elements (Al, Si, Ca, Fe, and Ti) were more pronounced, though with substantially lower concentrations unlike in PM2.5–10 fraction. For instance, in Aba, Al (3,051 ng m−3) and Si (7,089 ng m−3) mean concentrations in were about 5 times lower than its corresponding values in PM2.5–10 fraction. Other elements with significant mean concentrations are S and K. Sulphur mean concentration ranged from 113 ng m−3 (Maiduguri) to 257 ng m−3 (Aba) while K had 17 ng m−3 (lowest) and 341 ng m−3 (highest) at Abuja and Aba, respectively. The origin of S in PM2.5 fraction could be mainly anthropogenic (fossil fuel combustion, biomass burning, refuse incinerations or industrial emissions) sources. Airborne sulphur rarely occurs as a pure element and it is usually produced as SO2 gas which readily converts to sulphate ions under normal atmospheric conditions. In the atmosphere,
ions can exist as sulphuric acid (producing acid rain) or neutralized to ammonium sulphate (Lee, Kim, Moon, Kim, & Lee, Citation2001). On the other hand, enrichment factor for K were similar across the cities and could suggests similar source origin. Average Ni concentrations in all the cities were lower than 20 ng m−3 World Health Organization guideline for Europe; however, exposure to Ni(CO)4 could cause skin rashes and irritations upon continuous inhalation. Other elements such as V, Cr, Mn, Cu, Br and Pb displayed low concentration (<20 ng m−3) values and were also not enriched (Table ) in the study cities.
Table 4. PM2.5 elemental EFs and PIs
PIs for PM2.5–10 fraction (Table ) varied greatly across the elements and study locations. For instance, Cl displayed high pollution class at Aba and Lagos while Na was in low class at all the cities. Al and Si as well as K, Ca and Fe were in the low pollution range across the cities. Br concentrations displayed moderate and high pollution classes. In Maiduguri and Kano, Pb was in the high and moderate pollution classes respectively. Pb pollution in Maiduguri is worrisome and calls for detailed investigations as few industries are cited in the city. For PM2.5 (Table ), S average concentrations were within low pollution class across the cities while only Br was in the moderate PIs range. Low PIs of most elements in PM2.5 fraction could be a function of the low concentration values.
3.2. Comparison of PM2.5–10 and PM2.5 mean elemental concentrations
For PM2.5–10 (Table ), Na mean concentrations (this study) were lower than 2,707 ng m−3 and 935 ng m−3 for Kenitra city (Tahri et al., Citation2013) and Hong Kong (Cohen et al., Citation2009) respectively. However, Cl concentration (876 ng m−3) in Lagos was higher than values recorded in other cities except for Accra. Interestingly, Al average concentration (16,327 ng m−3) at Aba was ten times more than value for Kenitra city (Morocco) but lower than Accra and Hong Kong values. With the exemption of Abuja, average concentrations of Si (this study) were higher than values for Accra (Arku et al., Citation2008) and Nairobi (Gatari, Boman, & Wagner, Citation2009). On the other-hand, Ca average concentrations; 5,603 ng m−3 (Aba) and 6,000 ng m−3 (Maiduguri) were close but lower than 11,300 ng m−3 for Kenitra. Conversely, high concentrations of soil dust elements were expected in Kenitra owing to its proximity to the Sahara Desert but our assertion was limited as the elemental concentrations of soil dust markers varied across the cities.
For PM2.5 fraction (Table ), average elemental concentrations (this study) were lower than values for reported for most cities. For instance, S average concentrations were lower than 965, 1,300 and 1,700 ng m−3 for Accra (Arku et al., Citation2008), Nairobi (Gatari et al., Citation2009) and Manila respectively. Similarly, K concentrations (this study) were lower than values reported for other cities. However, average concentration of Ca (1,216 ng m−3) and Fe (2,393 ng m−3) in Aba were comparable to values for Kenitra. Vanadium and copper average concentrations (this study) were similar to values reported for other cities. Incidentally, Cr and Ni mean concentrations (this study) were similar with values for other cities except in Kenitra. Zn and Pb concentrations were close to value for Accra but lower than their corresponding concentrations at Nairobi, Kenitra, and Manila. Factors such as sampling height, time and period, type of samplers could contribute to these numerical differences.
3.3. Inter-elemental ratios
To distinguish between source origins elemental compositions, a stringent signature of elemental ratios could be a finger print for specific emissions and could provide information on source identification and apportionment studies (Yli-Tuomi et al., Citation2003). Table displays inter-elemental ratios of some selected emission source markers.
Table 5. Comparison of inter-elemental average ratios
For PM2.5–10, Na–Cl ratios (this study) were similar but lower than 5.14 registered for Hong Kong (Cohen et al., Citation2009). Again, Na–Cl average variance (this study) of 0.65 indicated similar ratios across the cities. Interestingly, Al–Si ratios (this study) were in agreement with values for Kenya (Gatari et al., Citation2009) and Hong Kong (Cohen et al., Citation2009). Again, S–K ratios were also in agreement with other cities except for Hong Kong. Further examination revealed that Ca–Ti ratio (this study) differed across the geopolitical zones. For instance, Ca–Ti ratios in Abuja, Kano and Maiduguri (northern cities) were in the range of 7.77–9.96 while Lagos, Aba and Port Harcourt (southern cities) showed wide disparity as it registered 12.74, 3.34 and 5.13, respectively. Sulphur-Vanadium ratios (this study) were very low compared to 356.67 for Hong Kong probably due to differences in emission source strengths. For PM2.5 (Table ), most inter-elemental ratios were close to values obtained for PM2.5–10 samples. For instance, Na–Cl recorded 1.41 (PM2.5–10) and 1.44 (PM2.5) at Aba while Al–Si ratios at Port Harcourt were the same in both fractions. With an overall variation of 4,827.24), S–V ratios were relatively higher than other cities and could implicate multiple emission sources or varying source strengths. Conversely, Ca–Fe ratios (this study) were lower than data for Accra, Nairobi, Kenitra and Hong Kong; while, V–Ni ratios (this study) were similar to values for other cities. Again, Zn–Pb and Zn–Cu ratios (this study) were also higher than values for other cities.
3.4. Principal component analysis
Table displays factor loadings of each species of PM2.5 and PM2.5–10 fractions, except for % Var. which is the percentage of variance that is explained by different components and factor loading higher than 0.5 were retained and shown. PCA resolved three (PM2.5–10) and four (PM2.5) sources.
Table 6. Loadings obtained by principal component analysis, normalized by Varimax rotation
The numbers in the table are the loadings of each species to the component, except for % Var., which is the percentage of the variance explained by the components. Factor loading higher than 0.5 were retained and shown.
For PM2.5–10, three factors with eigenvalues greater than 1 were resolved and the cumulative explained variance was 88%. Component 1 had loadings of Ti (0.95), Al (0.95), Si (0.94), Zr (0.93), Fe (0.95) and Ca (0.66) could be associated to soil dust (Yli-Tuomi et al., Citation2003). It explained about 50% variances of PM2.5–10 total elemental concentrations. High variance explained by the factor could be due to entrained and re-suspended dust from poorly maintained road networks and unpaved walkways in most Nigerian cities. Again it could be due to Hamarttan season which is characterized by dry soil conditions, low relative humidity, scanty rainfall and north-easterly prevailing winds. Soil dust source could be either natural or anthropogenic and contribute majorly to the crustal loading in the urban environment. Interestingly, second component with high loadings of Na (0.89), Cl (0.86), S (0.93) and Br (0.78) clearly implied a sea spray source (Kothai et al., Citation2008). It explained about 22% of variance of PM2.5–10 elemental concentrations. The third component displayed high loadings of K (0.73), V (0.78), Cr (0.85), Ni (0.82), Cu (0.66), Zn (0.77) and Pb (0.68). This component was identified as industrial emissions (Kelley, Jaffe, Baklanov, & Mahura, Citation1995). However, Zn could also indicate vehicular emissions as it is an indicator for two-stroke engines been used in formulations of lubricating oils (Chueinta, Hopke, & Paatero, Citation2000). The factor accounted for about 15% variations of PM2.5–10 elemental concentrations.
For PM2.5 (Table ), four components with a cumulative explained variance of 86%. were extracted from PCA. The first component had high loadings of Mg (0.83), Al (0.87), Si (0.86), Ca (0.86), Fe (0.88) and Ti (0.89) could be due to influence of soil dust. Other markers with significant loadings apportioned to this factor were Na (0.67), Cl (0.77), Br (0.66) and Pb (0.57). Again, influence of soil dust source was vivid as percentage variance apportioned to the factor is 42%. However, explained variance for PM2.5 soil dust component is slightly lower than 54% reported for Hong Kong (Cohen et al., Citation2004). The second component could be related to biomass burning emissions because it had high loadings of biomass markers; P (0.85), S (0.54), K (0.75) and Cr (0.77). High percentage (24%) of PM2.5 total variance explained by the component could due to use of biomass as alternative source of energy in most cities as well as open burning of waste and farmlands commonly practised in sub-Saharan Africa. Third factor accounted for 15% of the total PM2.5 variances. It displayed high loadings of V (0.96), Ni (0.83) and Zn (0.51) and could be related to emissions from petroleum products combustions. As an estimate, the number of used vehicle imported into Nigeria in the last decade was above 300,000 and it is projected that more than 450,000 by 2020 (Abiye et al., Citation2014) implying more consumption of petroleum product combustions. The fourth resolved component with high loadings of Mn (0.86), Cu (0.73), Zn (0.85) and Pb (0.65) could be attributed to industrial emissions and it accounted for 5% of total variances of PM2.5 elemental concentrations. Automobiles are also known to be major sources of atmospheric Pb due to the use of leaded antiknock additives in gasoline. The effort to phase out Pb in gasoline started worldwide during the last two decades and many countries including Nigeria have seen a dramatic decrease in concentration of airborne Pb. In general, PCA explained 87% (PM2.5–10) and 86% (PM2.5) variances while unexplained variances (~15%) in both fraction could be attributed to unanalyzed data for other sources such as concentrations of carbon and other organic compounds.
4. Conclusions
Twenty-three (23) elements (Na, Mg, Al, Si, P, S, Cl, K, Ca, Ti, V, Cr, Mn, Fe, Ni, Cu, Zn, Se, Br, Rb, Sr, Zr and Pb) were determined for PM2.5 and PM2.5–10 fractions in six potential megacities; Aba, Abuja, Lagos, Kano, Maiduguri and Port-Harcourt representing six geographical zones of Nigeria. The concentration of some elements such as Ni and Pb exceeded World Health Organization guideline standards in some cities. However, most elements were not enriched while PI revealed that some elements were in moderately and high polluted class. Principal components analysis of the elemental data-sets showed that soil dust, biomass burning, sea spray, petroleum products combustions and industrial emissions were major sources of PM2.5 and PM2.5–10 pollutions in the study sites. However, further work is in progress to establish seasonal or yearly time trends of PM2.5–10 and PM2.5 in most Nigerian cities.
Funding
The authors are grateful to International Atomic Energy Agency (IAEA) Vienna Austria, for [grant number NIR 12022] Postgraduate Fellowship granted G.C. Ezeh.
Acknowledgements
We also acknowledge the excellent support of Drs Chiari, Nava, Calzolai and Lucarelli of Department of Physics and Astronomy, University of Firenze and INFN, via Sansone 1, 50019 Sesto Fiorentino (FI), Italy who facilitated the NIR 12022 programme at Firenze. Authors are indeed thankful to Engr E.O. Ojo of Pollution Control Department, Federal Ministry of Environment Abuja Nigeria for mobility support during the field works.
Additional information
Notes on contributors
O.E. Abiye
The author is a research fellow at Centre for Energy Research and Development (CERD) and an associate lecturer at Department of Chemistry both in Obafemi Awolowo University, Ile-Ife Nigeria. Ezeh is also a Fellow of International Atomic Energy Agency (IAEA) Vienna Austria and currently holds a regular associate membership of International Centre for Theoretical Physics (ICTP) Trieste, Italy. His research interest is on air pollution monitoring and modelling, GHG and climate change studies as well as applications of X-ray-based analytical techniques to characterization of cultural heritage materials and other environmental matrices. He is currently a Technical Counterpart (TC) Scientist in the on-going IAEA funded NIR2014004 on Design and Development of National Ambient Air Quality Monitoring Network for Potential Megacities in Nigeria. Dr Ezeh is happily married with children.
References
- Abiye, O. E., Obioh, I. B., Ezeh, G. C., Alfa, A., Ojo, E. O., & Ganiyu, A. K. (2014). Receptor modelling of atmospheric aerosols in Federal Capital Territory (FCT), Nigeria. Ife Journal of Science, 16, 107–119.
- Arku, R. E., Vallarino, J., Dionisio, K. I., Willis, R., Choi, H., Wilson, J. G., … Ezzati, M. (2008). Characterizing air pollution in two low-income neighborhoods in Accra, Ghana. Science of the Total Environment, 402, 217–231. doi:10.1016/j.scitotenv.2008.04.042
- Calzolai, G., Chiari, M., García Orellana, I., Lucarelli, F., Migliori, A., Nava, S., & Taccetti, F. (2006). The new external beam facility for environmental studies at the Tandetron accelerator of LABEC. Nuclear Instruments and Methods in Physics Research Section B: Beam Interactions with Materials and Atoms, 249, 928–931. doi:10.1016/j.nimb.2006.03.193
- Calzolai, G., Chiari, M., Lucarelli, F., Nava, S., & Portarena, S. (2010). Proton induced γ-ray emission yields for the analysis of light elements in aerosol samples in an external beam set-up. Nuclear Instruments and Methods in Physics Research Section B: Beam Interactions with Materials and Atoms, 268, 1540–1545. doi:10.1016/j.nimb.2010.03.002
- Chan, T. W., & Mozurkewich, M. (2007). Simplified representation of atmospheric aerosol size distribution. Atmospheric Chemistry and Physics, 7, 875–886. doi:10.5194/acp-7-875-2007
- Chueinta, W., Hopke, P. K., & Paatero, P. (2000). Investigation of sources of atmospheric aerosol at urban and suburban residential areas in Thailand by positive matrix factorization. Atmospheric Environment, 34, 3319–3329. doi:10.1016/S1352-2310(99)00433-1
- Cohen, D. D., Garton, D., Stelcer, E., Hawas, O., Wang, T., Poon, S., … Cheol-Choi, B. (2004). Multi-elemental analysis and characterization of fine aerosols at several key ACE-Asia sites. Journal of Geophysical Research, 109, D19S12. doi:10.1029/2003JD003569
- Cohen, D. D., Stelcer, E., Santos, F. L., Prior, M., Thompson, C., & Pabroa, P. C. B. (2009). Fingerprinting and source apportionment of fine particle pollution in Manila by IBA and PMF techniques: A 7-year study. X-Ray Spectrometry, 38, 18–25. doi:10.1002/xrs.1112
- dos-Anjos, M. J., Lopes, R. T., de Jesus, E. F. O., Assis, J. T., Cesareo, R., & Barradas, C. A. A. (2000). Quantitative analysis of metals in soil using X-ray fluorescence. Spectrochimica Acta Part B: Atomic Spectroscopy, 55, 1189–1194. doi:10.1016/S0584-8547(00)00165-8
- Ezeh, G. C., Obioh, I. B., Asubiojo, O. I., & Abiye, O. E. (2012). PIXE characterization of PM10 and PM2.5 particulates sizes collected in Ikoyi Lagos, Nigeria. Toxicological and Environmental Chemistry, 94, 884–894. doi:10.1080/02772248.2012.674133
- Ezeh, G. C., Obioh, I. B., & Asubiojo, O. I. (2015). Multi-elemental analysis and source apportionment of urban aerosols in a low density residential area: A case study of Ikoyi, Lagos Nigeria. Ife Journal of Science, 17, 415–427.
- Ezeh, G. C., Obioh, I. B., Asubiojo, O. I., Chiari, M., Nava, S., Calzolai, G., Lucarelli, F., & Nuviadenu, C. K. (2014). Elemental compositions of PM10-2.5 and PM2.5 aerosols of a Nigerian urban city using ion beam analytical techniques. Nuclear Instruments and Methods in Physics Research Section B: Beam Interactions with Materials and Atoms, 334, 28–33. doi:10.1016/j.nimb.2014.04.022
- Gatari, M. J., Boman, J., & Wagner, A. (2009). Characterization of aerosol particles at an industrial background site in Nairobi, Kenya. X-Ray Spectrometry, 38, 37–44. doi:10.1002/xrs.1097
- Kelley, J. A., Jaffe, D. A., Baklanov, A., & Mahura, A. (1995). Heavy metals on the Kola Peninsula: Aerosol size distribution. Science of the Total Environment, 160-161, 135–138. doi:10.1016/0048-9697(95)04351-Z
- Kothai, P., Saradhi, I. V., Prathibha, P., Hopke, P. K., Pandit, G. G., & Puranik, V. D. (2008). Source apportionment of coarse and fine particulate matter at Navi Mumbai, India. Aerosol and Air Quality Research, 8, 423–436. doi:10.4209/aaqr.2008.07.0027
- Krzyzanowski, M., & Cohen, A. (2008). Update of WHO air quality guidelines. Air Quality, Atmosphere & Health, 1, 7–13. doi:10.1007/s11869-008-0008-9
- Lee, J. H., Kim, Y. P., Moon, K. C., Kim, Hui-Kang, & Lee, C. B. (2001). Fine particle measurements at two background sites in Korea between 1996 and 1997. Atmospheric Environment, 35, 635–643. doi:10.1016/S1352-2310(00)00378-2
- Maenhaut, W., Francois, F., & Cafmeyer, J. (1993). The “Gent” stacked filter unit sampler for the collection of atmospheric aerosols in two size fractions: Description and instructions for installation and use (Technical Report No. NAHRES-19). Vienna: International Atomic Energy Agency. Retrieved from http://www-pub.iaea.org/MTCD/publications/PDF/te_825_prn.pdf
- Obioh, I. B., Ezeh, G. C., Abiye, O. E., Alpha, A., Ojo, E. O., & Ganiyu, A. K. (2013). Atmospheric particulate matter in Nigerian megacities. Toxicological and Environmental Chemistry, 95, 379–385. doi:10.1080/02772248.2013.790970
- Tahri, M., Bounakhla, M., Zghaid, M., Noack, Y., Benyaich, F., & Benchrif, A. (2013). An evaluation of airborne particulate matter pollution in Kenitra city, Morocco. Ambi-Agua Taubate, 8, 38–47. doi:10.4136/1980-993X
- Taylor, S. R. (1964). Abundance of chemical elements in the continental crust; a new table. Geochimica et Cosmochimica Acta, 28, 1273–1285. doi:10.1016/0016-7037(64)90129-2
- World Health Organization. (2006). Air quality guidelines for particulate matter, ozone, nitrogen dioxide and sulfur dioxide. Global update. Geneva: Author. Retrieved from http://www.Euro.who.int/Documents/E87950.pdf
- Yli-Tuomi, T., Venditte, L., Hopke, P. K., Basunia, M. S., Landsberger, S., & Viisanen, Y. (2003). Composition of the Finnish Arctic aerosol: Collection and analysis of historic filter samples. Atmospheric Environment, 37, 2355–2364. doi:10.1016/S1352-2310(03)00164-X