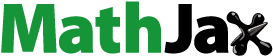
Abstract
Climate change exacerbates the daunting defy on agriculture in general and the livestock sector in particular. The aim of this study was to assess the determinant factors of climate change adaptation choices in the agro-pastoral dry lands of northeastern Ethiopia. Data on the household characteristics and adaptation choices were collected using interview schedule from a sample of 260 household heads. Multivariate probit regression and descriptive statistics were used for data analysis. The results indicated that 63.5% of farmers were applying portfolio diversification, enhancing livestock productivity, agro-ecological practices, and diversifying non-agricultural income choices to acclimatize the climate change. The model revealed that many explanatory variables, such as educational level, gender, farming experience, family size, farmland size, goat flock size, farm and non-farm income, frequency of extension contact, access to credit and climate information were significant factors for adaption choice decisions. The marginal success probability of adapting non-agricultural income diversification was the lowest compared to the agro-ecological practices, portfolio diversification and enhancing livestock productivity. The marginal success probability of jointly adapting agro-ecological practices, portfolio diversification, non-agricultural income diversification and enhancing livestock productivity choices was also lower comparing to their failure. Therefore, any effort projected to relax climate change effects should gear towards the provision of services resembling to eminence extension, vocational trainings, affordable credit, empowering vulnerable groups, community radios as well as local meteorology. Moreover, non-farm income generating initiatives on top of agricultural intensification through input supply would expedite context specific adaptation to the changing climatic status quo.
PUBLIC INTEREST STATEMENT
Climate has been changing in the past and continuous to change in the future, entails a need to understand how farmers are mindful to guide actions apt for future climate risk identification, adaptation and intervention. Several studies on climate change adaptation and determinants carried out in Ethiopia, however most of them were at macro level hence make the result vague to generalize about specific location. Therefore, it’s relevant to conduct context specific enquiry since adaptation choices vary contextually and spatially thus provides room for district and household level analysis. Understanding the local dimensions of climate change helps development practitioners to put in place supportive actions and informed decisions, notably to device community wise policy directives. Likewise, knowledge and experience on viable adaptation choices is vital for concerned stakeholders to capitalize on the superb choices during interventions launched to enhance agro-pastoralists resilience to the climate change.
Competing Interests
The authors declare no competing interests.
1. Introduction
Climate change is the variation in the state of climate that can be characterized by increasing temperature and related climate phenomena, including an increase in frequency and intensity of extreme weather events such as hot spells, droughts, floods and an increase in climatic uncertainty (IPCC [Intergovernmental Panel on Climate Change], Citation2011a). It has emerged as one of the defining scientific, political and socioeconomic issues of the 21st century, as it embodies a new threat and challenge to numerous households, social groups and regions worldwide. The prevalence and frequency of drought in Sub Saharan Africa, India, North America, China and Western Europe have witnessed that it is a topic of global concern (Wilhite, Citation1985). The prolonged social and environmental impact has resulted in continuous land degradation and desertification as it happened in the Sahel regions in the late 1960s and 1970s (Zing, Citation2003).
Climate change adversely affects the agricultural sector through increased water stress, changing the patterns of run-off, shifting seasonality and variation in temperature (FAOSTAT [Food and Agriculture Organization Statistics], Citation2015). Severity and duration, and narrowing of the gap between water supply and demand have remarkably increased in both the developing and developed world (Wilhite, Citation1985). The reflection is more prevalent in developing countries and particularly in Sub-Saharan Africa (SSA) due to their vulnerability and low adaptive capacities, though it is felt across all affected regions (IPCC, Citation2007). Due to the enormity of likely aftermaths of a changing climate on human and natural systems, it has become a matter that man need to understand and respond (Miller & Edwards, Citation2012).
Climate is an important resource to both crop and livestock productions in developing regions as farmers depend largely on rain fed agriculture. Livestock production accounts 30% of the agriculture gross value in Africa, where 92% is coming from the production of beef cattle, dairy cattle, goats and sheep (Coulibaly, Citation2015). Furthermore, the global demand for livestock products will continue to increase significantly in the coming decades, driven by urbanization, population growth and income increases. This increased demand is largely based in developing countries (Peter & James, Citation2015). Livestock production in general and goat farming in particular still provides a livelihood for large number of people in marginal SSA; more in arid and semi-arid areas, where milk and meat are important dietary components (Solomon et al., Citation2014). Countries in SSA are mainly vulnerable to climate change effects since their prevalent poverty limits adaptive capacities. Adverse climate change effects are considered to be strong in countries located around tropical Africa that depend on agriculture as their main livelihood (WSPA (World Society for the Protection of Animals), Citation2009). During the last 4 decades, the SSA has witnessed increased frequency of drought with devastating penalties on humans and natural resources base. Such disasters threaten food security through the disruption of normal cropping, pastoral and marketing activities with negative impacts on economic growth (Ndikumana, Citation2002).
Agriculture in general and livestock production in particular is the main stay of Ethiopian economy. Small ruminants, especially indigenous breeds, are pervasive and vital to the subsistence livelihoods for substantial proportion of resource poor farming households in the country (Zelalem, Aynalem, & Emmanuelle, Citation2009). Its estimated goat population was about 25 million in 2013, accounting for 7.2 and 2.6% of the African and global goat population, respectively. Thus, the contribution of the goat farming sub-sector in Ethiopia is sizeable in improving the livelihood of farmers in marginal agro-pastoral regions (FAOSTAT, Citation2015). Owing to its geographical location, Ethiopian climate is typically tropical and naturally prone to greater climatic variations in general and drought in particular. Its current climatic condition is quite variable and is influenced by the Sahel Saharan desert, the socioeconomic edifice and its various physical characteristics (Philip, Mario, Ade, & Okeyo, Citation2013).
Across Ethiopia, millions of people are already experiencing the changing seasonal patterns of temperature and rainfall, which are expected to depress livestock production particularly the goat farming sub-sector by the coming decades in arid agro-pastoral systems (Stark, Terasawa, & Ejigu, Citation2011). Eastern Amhara region has around 10 million small ruminants, of which 74% are goats and the study district contains 26.2%. However, its contribution to the farmers’ livelihood is lower considering the potential due to traditional goat management practice, high disease prevalence and feed shortage rooted from frequent climate variability and drought in the district (Halima et al., Citation2012).
The key point is therefore, how farmers in the area respond to climate change? Identifying potential adaptation measures thus helps in defining factors that influence the choice decisions of farmers in the study area. Adaptations to climate change are characterized by adjustments to ecological and socioeconomic systems of a particular area (Devereux & Guenther, Citation2007). Experience has shown that nationally identified adaptations do not certainly translate into practice since adaptations are local and sector specific. The ability to adapt to climate change is determined by predictor variables, which are demographic, socioeconomic and institutional in nature (Juana, Kahaka, & Okurut, Citation2013).
All in all, studies on farmers’ climate change adaptation choices and their determinants have been carried out in Ethiopia. Nonetheless, most of them were undertaken at macro level, which might make the result vague to generalize about specific location. Because reactions to climate change incidences are highly diverse and complex as they vary by region, community, social group, households, gender, age, season and time in history (Coulibaly, Citation2015). They also commenced entirely on agriculture and attention to specific commodity and farming system missed. Few attempts have been made to study livestock adaptation to climate change in Ethiopia (Devereux & Guenther, Citation2007; Mc Donald & Simon, Citation2011; Stark et al., Citation2011; Zelalem et al., Citation2009). However, only Zelalem et al. (Citation2009) and Stark et al. (Citation2011) attempted to determine the factors at district level. The fact that adaptation choices vary contextually and spatially (within communities and individuals) hence provides room for location and household level inquiry. A number of studies also identified specific variables, which may positively and/or negatively influence the particular adaptation choice to climate change. These studies were scanty and even focusing on entirely to the wider east African region (Berman, Citation2014; Burney, Citation2014; Jones & Thornton, Citation2008). However, this paper focuses on specific adaptation choices in eastern Amhara since climate change effects are location and context specific in nature.
This study therefore, coined to examine the agro-pastoral farmers’ adaptation choices to climate change and their determinants using multivariate probit model, at specific location. Results from this study will deliver empirical evidence on factors to climate change adaptation choices there by helps to discern wherewithal to exploit the choices sustainably. It also helps for development practitioners to make informed decision on top of capitalizing on superb adaptation choices in their context specific intervention. The section immediately below deals with the research methodology. Section 3 provides the results and discussion part. The last section, presents the conclusion derived from the empirical findings.
2. Research methodology
2.1. Description of the study area
This study was conducted in Abergelie, one of the agro-pastoral districts located in the northeastern part of Amhara region, at 13°20ʹ N’ latitude and 38°58ʹ E’ longitude. The altitude ranges from 1150 to 2500 MASL while the mean annual temperature and rainfall ranges between 23–43°c and 250–750 mm, respectively (Abeje, Ademe Mihiretu, & Tsega, Citation2016). The district is basically classified as plain (10%), undulated (20%), mountainous (55%) and gorge/valley (15%). Because of erratic rainfall and poor soil fertility, crop production is restricted to some pocket areas owing relatively fertile soil and moderate moisture (AWOA [Abergelie Woreda Office of Agricultural Development], Citation2016). Despite all livestock types are kept in the district, 88.2% of farmers are dominantly engaged in goat production. Goats also account 73.4% of the district’s livestock population, having the leading contribution to farmers’ livelihood (Lijalem et al., Citation2013). Farmers’ average flock holding size was estimated to be between 15–30, 60–90 and ≥200 goats for poor, medium and rich households respectively (AWOA, Citation2016). Goats are main assets of farmers, which can be sold at any time when cash is needed. The goat breed is commonly known for its milk and quality meat so that farmers keep for their products and byproducts to use as food and income sources (Halima, Lababidi, Rischkowsky, & Tibbo, Citation2012).
2.2. Sampling and data collection
Two-stage sampling technique was applied to select sample farmers. In the first stage, 4 kebeles from 15 kebelesFootnote1 were selected randomly. In the second stage, considering a fairly acceptable degree of the error term and 95% confidence interval, the sample size was determined using the provided formula: n = N/1 + N(e)2 (Yamane, Citation1967). Using systematic random sampling, a total sample size of 260 household heads was drawn then respondents from each kebele were assigned via probability proportional to size. A cross sectional study design incorporating quantitative aspects was employed to collect data. Employing enumerators, the quantitative data mirroring the demographic, socioeconomic and institutional features of the study population was collected using structured interview schedule. Different climate change adaptation options of farmers were collected to have in-depth understanding of the choices. Farmers were also asked to recall their climate change perception in the 10 years study period (2006–2016). Secondary data including the socioeconomic background and meteorological records of the district across the study years were collected from district office of Agriculture and National Meteorological Service Agency (NMSA), respectively.
2.3. Empirical model specification, variable definition and data analysis
2.3.1. Empirical model
When there are more than two alternatives among which the decision maker has to choose, the appropriate regression model would be either multinomial logit (MNL), multinomial probit (MNP) or multivariate probit (MVP, hereafter). The first assumes independence across outcomes and requires the choice variables to be mutually exclusive (Cappellari & Jenkins, Citation2003). MNP model does not require assumption of the independence of irrelevant alternatives (IIA); hence, the drawback is requisite of multivariate normal integral evaluation to estimate unknown parameters (Temesgen, Hassan, Ringler, Alemu, & Yesuf, Citation2009). However, MVP provides a convenient closed form for underlying choice probabilities without multivariate integration. In reality, farmers consider a bundle of possible options to maximize their utility in averting climate change effects (Tazeze, Haji, & Ketema, Citation2012). Adaptation decision is unsurprisingly multivariate and attempting a univariate modelling masks the convenient economic information found in the interdependent adaptation choices. As a result, MVP model is proper to model simultaneously the effects of explanatory variables on each choice outcome variables, while permitting unmeasured factors to correlate spontaneously. It is also appropriate to take into account the correlation structure among choice variables. An advantage of the MVP over the MNL model is relaxation of the assumption of IIA, which is in many cases idealistic (Piya, Maharjan, & Joshi, Citation2012a).
The MVP model assumes that given a set of explanatory variables, the multivariate response is an indicator of the event that some unobserved latent variable (Z), assumed to arise from a multivariate normal distribution, falls within a certain interval. Following Piya et al. (Citation2012a), the MVP model assumes that each subject has J distinct binary responses. Let be the independent observations,
be the available options of binary responses, and
be a matrix of covariates composed of any discrete or continuous variables.
Let denote the J-dimensional vector of observed binary responses taking values
on the
household and
denote a J-variate normal vector of latent variables such that:
Where a matrix of unknown regression coefficient,
is a vector of residual error distributed as multivariate normal distribution with zero means and unitary variance;
where Ʃ is the variance-covariance matrix. The off-diagonal elements in the correlation matrix
represent the unobserved correlation between the stochastic components of
and
options (Cappellari & Jenkins, Citation2003). The relationship between
and
is:
The likelihood of the observed discrete data is then obtained by integrating over the latent variables
Where, is the interval
if
and the interval
otherwise and
is the probability density function of the standard normal distribution. The MVP coefficients are difficult to interpret, and associating with the jth outcome is tempting and misleading. To interpret the effects of explanatory variables on the probabilities, marginal effects are usually derived as:
Where, δij-denotes the marginal effect, of the explanatory variable on the probability that alternative j is chosen. The marginal effects measure the expected change in probability of a particular choice with respect to a unit change in an explanatory variable (Amdu, Ayehu, & Deressa, Citation2012).
2.3.2. Variable definition
In this study, the dependent variable is “farmers’ adaptation choices to climate change”. Farmers in the study area employed a range of adaptation options thus the most used adaptation measures were 19. To make the dependent variables fit for the model, the identified measures pooled in to four mutually interdependent sets through combining interrelated choices together. This is in line with the finding of Belayneh, Yared, and Bewket (Citation2012) stating a single strategy is scanty to adapt climate change, rather a combination of options are likely to be more effective. The dependent variables in the empirical model were the combined sets of actions specified (Table ). Explanatory variables expected to reflect the demographic, socioeconomic and institutional characteristics of the study population identified based on related literatures. There is mixed information in literature as to the influences of the different household characteristics on their climate change adaptation choices.
Table 1. Summary of explanatory variables for MVP model regression
Table 2. Farmers’ perception on main climate change elements, n = 260
Table 3. Pooled adaptation choices of farmers to climate change, n = 260
The study by Juana et al. (Citation2013) indicated that the farmers’ age had a positive effect on the diverse adaptation choices. The assumption is that as age increases, the farmers are likely to acquire experience in weather forecasting there by increases the likelihood of practicing different adaptation options. However, according to Belayneh et al. (Citation2012), age negatively influenced the decision to adapt options related to technology because older farmers are more risk-averse and less likely to be flexible. Therefore, age of the farmer here hypothesized to have both positive and negative influence on adaptation choice decisions to climate change.
Female headed farmers had less exposure to adaptation measures since they are culturally assigned for activities which are domestic in nature and even had limited access to critical resources (land, cash and labour), which often undercuts the ability to carry out labour-intensive agricultural activities (Temesgen et al., Citation2009). Male-headed farmers on the other hand, had better access to information on climate as they exposed for varied socio-political contacts in the community. Nonetheless, a study in South Africa, found that female-headed farmers were more likely to take up adaptation means (Nhemachena & Hassan, Citation2007). Therefore, male-headed farmers hypothesized to have more likely positive kin with adaptation choice decisions to climate change.
Studies showed that as the higher the education level, the better would be the knowledge on climate change and its adaptation choices. Literate farmers were likely to respond to climate change through making best adaptation choices of his/her preference (Evengelista, Young, & Burnett, Citation2013). In this study, hence farmers with better education expected to understand climate change; thus assumed to influence the adaptation choice decisions positively.
As family size increased, adaptation to various options will also increase since farming activities are obviously labour intensive and household’s labor is the main source in Ethiopian context (Tazeze et al., Citation2012). However, according Tizale (Citation2007), there was a possibility that households with large families enforced to divert part of their labour to non-farm activities to earn income to ease the consumption pressure imposed by large family. Therefore, family size here hypothesized to affect diverse adaptation choices both positively and negatively.
Farming experience increased the probability of taking adaptation options since experienced farmers had better knowledge and information on changes in climatic trends and conditions (Kebede, Citation2013). Farm experience seems to proxy with age, but the circumstance in the local setting shows that there are farmers lived for longer years but have minimum experience in farming. On contrary, there were farmers who lived relatively shorter years but have better experience in farming in the study area. This is due to the presence of several military returnees in the district. Thus, farming experience hypothesized to have a positive influence on adaptation choice decisions to climate change.
Farmland size assumed to encourage adoption of technologies linked to land such as irrigation structures. An increase in farm size would result in higher probability of using crop diversification, soil conservation and planting trees to adapt the climate change (Gebrehiwot & van der Veen, Citation2013. Conversely, studies in agro-pastoral areas indicated that livestock farmers with bigger farmland had less adaptation probability as they can shift from livestock to crop production (Zelalem et al., Citation2009). Hence, this variable was hypothesized to have a negative influence on farmers’ adaptation choice decisions to climate change.
Total livestock is all animals apart from goats kept by the household, which expressed in conversion equivalent to tropical livestock unit (TLU), calculated using the formula: (ILRI [International Livestock Research Institute], Citation2013). Livestock ownership indicated the level of farmers’ dependence on natural resources such as grassland and water points for extensive livestock production (Kemausuor, Dwamena, Bart-Plange, & Kyei-Baffour, Citation2011). Different livestock types in this regard had varying degree of vulnerability to stresses stemmed from recurrent droughts in the changing climate. Therefore, farmers with higher number of livestock were hypothesized to have positive relation with adaptation choice decisions.
Livestock ownership and goat flock size seems to be proxy but in the study area there were farmers engaged in goat production solely as well as in the mixture of livestock types. Accordingly, the investigators pursue to see the relation between goat flock size and the dependent variables since being a small animal, compared to large animals keeping goats is less risky and even restoration of the flock size is easy (Philip et al., Citation2013). On contrary, larger goat flock, size is associated with greater demand for feed and water. Hence, goat flock size of the farmers hypothesized to have positive influence on adaptation choice decisions to climate change.
Increased annual income of the household in USD from different farming activities mainly of selling livestock and crop products expected to increase the opportunity to look alternative climate change adaptation options (Kassaye, Citation2010). Therefore, farm income was hypothesized to have a positive influence on farmers’ adaptation choice decisions to climate change. The non-farm income obtained from sources like transfers, labour wages, and production assets, thus non-farm employment provides constraint to climate change adaptation due to the pressure on the farm managerial time (Zelalem et al., Citation2009). As a result, non-farm income was hypothesized to have a negative influence on adaptation choice decisions to climate change.
Accessing climatic information increased the probability of adapting diversified choices since better information helps farmers to make comparative decision among choices to cope effectively with the changes (Tazeze et al., Citation2012). Therefore, accessing climate information was hypothesized to have a positive influence on farmers’ adaptation choice decisions to climate change.
Increased contacts with support services such as extension agents and climate information providers is supposed to increase farmers’ perception of climate change and its associated risks, there by rises their adaptation decisions (Amdu et al., Citation2012). Ethiopian Agricultural Extension manual dictates every farmer has the right to get advice and technical support at least once a month via the assigned agents (MoA [Ministry of Agriculture], Citation2010). According to the guide, farmers who get technical advice and support at least six times a year named as “extension accessed”. Thus, increased extension contact was hypothesized to have positive influence on farmers’ adaptation choice decisions to climate change.
Perception strongly affects how farmers deal with climate induced risks, thus the nature of their interactive responses to perception shapes adaptation choices. Misconception about climate change and its associated risk may result in no adaptation or maladaptation in turn increased the adverse effects of climate change (Pauw, Citation2013). This variable was therefore, assumed to have a positive influence on climate change adaptation choices.
The study by Juana et al. (Citation2013) revealed that training helps farmers to aware diverse attributes of climate change and then enriched their knowledge of applying diverse adaptation options. Access to training hence hypothesized to influence farmers’ adaptation choice decisions to climate change. Access to affordable credit also increased the financial sources of farmers then their capacity to meet transaction costs associated with adaptation options that they might want to take (Nhemachena & Hassan, Citation2007). Farmers who had access to credit do take more adaptation chances as any investment requires the use of owned/borrowed finance. Accordingly, access to credit was hypothesized to have positive influence on adaptation choice decisions to climate change.
Participation in community based institutions expected to increase awareness about climate change due to the farmer-to-farmer information sharing at their periodic gatherings. Because social networks increase the awareness level of farmers to practice effective adaptation options (Tazeze et al., Citation2012). Being fellow in any community built institutions was hence hypothesized to have a positive influence on farmers’ adaptation choices to climate change. Table depicts the explanatory variables identified for model regression as well as the hypotheses on how each variable influence the different adaptation choices to climate change.
2.4. Data analysis
In this study, the dependent variables are farmers’ adaptation choices to climate change (viz, portfolio diversification, enhancing livestock productivity, agro-ecological practices and non-agricultural income diversification); whereas the explanatory variables were the demographic, socioeconomic and institutional characteristics of farmers under study. Descriptive statistics (frequencies, percentages, measures of central tendencies) and inferential statistics were used to describe the farmers’ household characteristics, climate change perception and adaptation choices on top of MVP model analysis. The model was employed to analyses factors influencing the adaptation choices of climate change. Data on temperature and rainfall patterns were described and triangulated with trend analysis of the 10 years climate records. Multivariate analysis of variance (MANOVA) was also used to describe the household characteristics of farmers through comparing means of the diverse household features against the outcome variables using linear composite factor analysis.
3. Results and discussion
3.1. Farmers’ perception to different climate change elements
Farmers’ perception towards the patterns of main climate elements was assessed, hence 76.8% of farmers were perceived an increasing temperature while the 83.5% were perceived a decreasing rainfall in main rainy season. Climate change perception further examined disaggregating farmers in to different age, sex and education level groups since farmers in each group anticipated to have different awareness intensities (Pauw, Citation2013). Disaggregation by age groups (young, adult and elder), hence indicated that younger farmers were less likely to perceive climate change than their elder counterparts (p ≤ 5%). Disaggregation by education level while revealed variations in temperature, so that literate farmers perceived an increasing temperature than their illiterate counterparts (p = 10%). On the other hand, gender disaggregation showed that insignificant difference among groups (Table ). To confirm farmers’ perception of temperature and rainfall changes, meteorological records of the district regressed for the entire study periods (2006–2016). The result indicated that the mean annual temperature and rainfall of the district was increasing and decreasing by 0.29°C and 23.6 mm, respectively (Figures and , in supplementary section). Thus, farmers’ perception of main climate elements was covenant with meteorological records of temperature and rainfall in the district.
Farmers’ age disaggregation was provided by FAO (Citation2013): young (≤ 35), adults (36–64) and elderly (≥65) age categories
3.2. Farmers’ climate change adaptation choices and barriers
Among farmers who perceived climate change, only 63.5% were taking practical adaptation measures to endure livelihoods in the face of dynamic climate status quo. Most used adaptation measures were identified and pooled in to four mutually interrelated choice sets since farmers were using a bundle of options at a time. Planting dry-resistant and short season crops varieties, practicing intercropping, irrigation and livestock diversification were combined as ‘Portfolio diversification’ since they were considered for risk spreading. Livestock management through supplementary feeding, feed preservation, changing the reproduction season, keeping locally adapted breeds and destocking of goat flock size were pooled as ‘Enhancing livestock productivity’, these options were considered to intensify livestock productivity. Practices like transhumance, agro-forestry, area closure and soil and water conservation were combined in to ‘agro-ecological practices’ because employed to improve land efficiency. Involving in off-farm activities, seasonal migration, considering credit, pursuing relief aid and child schooling were pooled as ‘Non-agricultural income diversification’ since they are the means to increase non-farm income now and then (Table ). On the other hand, 36.5% of farmers who did not respond to climate change despite perceived changes were due to lack of training and extension services, unknown reason, lack of climate information, lack of money and credit access and shortage of labour in descending order (Figure ).
3.3. Characteristics of farmers pursuing different adaptation choices
The MANOVA was employed to understand farmers’ household characteristics that pursue different adaptation choices to climate change. Demographic features (excluding gender, educational level and household family size) were determined the farmers’ adaptation choice preference. Likewise, farmers who were using varied adaptation choices were significantly different in their access to credit despite ample institutional features (Appendix Table ). Therefore, farmers’ wealth status and its correlates, on top of their age, experience and perception were determined the choices of non-agricultural income diversification and agro-ecological practices to relax climate change effects. Farmers with different wealth status on the other hand were choosing portfolio diversification and livestock productivity enhancement as a strategy to deal with climate change effects.
3.4. Empirical model results
This section comprises MVP model regression results. As a result, except for membership in local institutions, the effects of factor variables on adaptation choices were tested. Membership in local institutions was excluded since 99.6% of farmers were involved in thus analysis of determinants on choices is futile since entities are similar for most samples. The rest 15 explanatory variables were thus tracked against the outcome variables. Ordinary least square (OLS) model tested for multicollinearity using variance inflation factor (VIF), so the mean value of 1.49 proved absence of multicollinearity between covariates. The null hypothesis of homoscedasticity was significant for Cook–Weisberg’s test between adaptation choices hence the model estimation directed in robust standard errors. This method does not change the coefficients and significance of the model rather offers relatively accurate probability without heteroscedasticity (Wooldridge, Citation2006). To end with, the probability of adapting each choice and the joint probability of success and failure to adapt the four choice sets was assessed. Estimated coefficients of the MVP model along with level of significance presented below (Table ).
Table 4. Parameter estimation of the multivariate probit model
Results from the empirical model indicated that most explanatory variables influenced the probability of climate change adaptation choices as expected. The direction of covariates’ influence was also as expected except to farmland size of the household. The likelihood ratio statistics of Wald test was significant [χ2 = 265.07, P = 0.000], which reveals that the covariates were amply explained the outcome variables similarly MVP model was the fitting analytical tool for the study. The likelihood ratio test of the null hypothesis, testified that absence of correlation between discrete equations is strongly rejected [χ2 = 152.9, P = 0.000]. Thus, authenticating the estimation of all equations instantly by MVP instead of individual equations was conventional.
Table 5. The marginal effect of determinants on household’s adaptation choices
The sign of correlation but indicates only two positive combinations: portfolio and non-agricultural income diversification as well as enhancing livestock productivity and agro-ecological practices. Coefficients from MVP regression designate the direction of the influence rather than the magnitude so that the interpretation commenced using marginal effects (Table ). Some of factor variables of the households (age, training access, livestock in TLU and perception level) had no influence (P > 0.01) on the household’s choices to climate change adaptation strategies. Explanatory variables that influenced farmers’ adaptation choices positively and significantly were gender (being male), educational level, farming experience, farm income, goat flock size, frequency of extension contact, access to climate information and access to credit services. Farmland size and non-farm income, on the other hand had both negative and positive effects on farmers’ adaptation choices but family size surprisingly had a negative effect on farmers’ adaptation choices. As a result, only statistically significant explanatory variables are discussed below.
Gender of the household head
Being male farmer positively influenced the adaptation of agro-ecological practices, so the marginal effect indicated those male farmers are 76.7% likely to apply adaptation choices to recruit the climate change effects. This can be due to the fact that women are culturally assigned for domestic activities and even have limited access to critical resources (land, cash, and labor), which often undercuts their ability to carry out labour-intensive activities like transhumance and soil and water conservation. This finding is thus in line with Kebede (Citation2013), affirming that male farmers are more likely to access information on climate change and pleased to take risks than their counterparts.
Education level of the household head
Education level of farmers influenced adaptation using enhancing livestock productivity and agro-ecological practices. A one-year increase in formal education hence increases the probability of enhancing livestock productivity and agro-ecological practices by 5.8 and 7.6%, respectively. This could literate farmers who are likely to react changes through evaluating choices that fits best to their knowledge, inclination and capabilities. This finding is covenant with the result of Gebrehiwot and van der Veen (Citation2013) stating that education improved the probability of climate change adaptation through performing crop diversification, soil conservation, changing planting dates and irrigation.
Farming experience of the household head
Farming experience had positive influence on climate change adaptation through agro-ecological practices. The marginal effect result thus indicated that a one-year increase in experience upsurges the probability of using agro-ecological practices by 4.1%. Therefore, practical farming year rather than living longer is influencing the adaptation choices of farmers. Mulatu (Citation2014) similarly found that the experience rather than age matters the adaptation to climate change.
Family size of the household
Family size had surprisingly a significant negative effect on farmers’ adaptation choices to climate change. A unit increase in family size thus would result a decline in the probability of using portfolio diversification by 16.9%. Livestock keeping needs recurrent movement with animals for pasture hence diversifying in sedentary agriculture would be less accustomed. This is in line with the finding of Tazeze et al. (Citation2012) stating households with large families may be forced to divert part of their labour to non-farm activities with an attempt to earn income and ease the consumption pressure imposed by a large family.
Farmland size of the household
The effect of farm size on climate change adaptation is of two types. In one hand, farm size positively influenced adaptation using portfolio and non-agricultural income diversification; hence a one hectare increase in farm size would upsurges the likelihood of using portfolio and non-agriculture income diversification by 42.9 and 27.8%, respectively. This result is in skirmish with the findings of Berman (Citation2014), anticipated that households with larger farms are expected to work on their plot intensively rather than going for alternatives. On the other hand, having large farm linked with the negative likelihood of adaptation using agro-ecological practices. The probability of using agro-ecological practices would decrease by 48.8% in every one-hectare plot size increase. The possible reason is that farmers may think of the benefit from economic scale of production rather than land intensification.
Farm and non-farm income of the household
Farm income of the household had positive effect on portfolio and non-agricultural income diversification hence a 1 USD increase in farm income increased the possibility of portfolio and non-agriculture income diversification at 0.2 and 0.3%, respectively. Similarly, 1 USD increase in non-farm income increased the probability of adaptation using portfolio diversification by 0.1%. These may be due to increasing the financial power of farmers to deal with the costs of investment in different farming inputs as well as source of capital in non-agriculture income diversification (Coulibaly, Citation2015). However, non-farm income negatively affects climate change adaptation using agro-ecological practices though the marginal value is negligible. This is may be due to; non-farm employment might coerce adaptation as it competes time for agro-ecological management and conservation work.
Goat flock size of the household
Goat flock size positively correlated with climate change adaptation through enhancing livestock productivity at 1% significance level. Thus, an increase in flock size would increase the probability of using livestock productivity enhancing choices by 0.6%. This maybe because the increased flock size forces farmers to exercise measures like dropping flock size during the peak period, preserving supplementary feed and altering the reproduction season to protect losses.
Access to information on climate change
This variable determined the four choices of climate change adaptation positively. Access to climate information, increases the probability of adaptation through portfolio diversification, enhancing livestock productivity, agro-ecological practices and non-agricultural income diversification by 73.6, 82.8, 5.2 and 60.6, respectively, in percentage points. This suggests the dissemination of more climate information via extension services, weather reports and other channels will increase the likelihood of climate change adaptation.
Frequency of contact with the extension agents
Frequency of contact with the extension agents had a positive effect on applying agro-ecological practices at 5% significance level. Result from marginal effect, implies that an increase in contact frequency similarly would increase the probability of using climate change adaptation by 47.2%. This result confirmed with the findings of Mulatu (Citation2014), who states farmers having increased contact frequency with the extension agent would have better prospects to climate change information and various farming practices that they can use to adapt the adverse effects.
Households’ access to credit
Accesses to credit have a positive effect on the likelihood of using portfolio diversification to adapt the climate change. If a farmer has an access to credit then his/her probability of adapting portfolio diversification would increase by 40.3%. Access to affordable credit increases the financial resources of farmers and then their ability to come across transaction costs associated with various adaptation options that they might want to take (Berman, Citation2014).
The marginal success probability of using each distinct adaptation choice is reported below (Table ). Therefore, the likelihood of adapting non-agricultural income diversification was lowermost compared to the adaptation of agro-ecological practices, portfolio diversification and enhancing livestock productivity in ascending order. This is a good indication that availability of non-agricultural income generating activities is dared in the study area. This maybe as an agro-pastoral, farmers may emphasis on migrant livestock production than sedentary non-agricultural activities. Nevertheless, non-farm income activities were anticipated to improve the farmers’ financial position and allowed to purchase diverse farm inputs (Temesgen et al., Citation2009). Households were more likely to fail to jointly adapt the four adaptation choice sets (Table ). As a result, the likelihood of farmers to adapt the four choice sets jointly was only 0.38% compared to the failure to adapt the four choices (33.8%).
Table 6. The marginal success probabilities of each adaptation choices
Table 7. The joint probabilities of success or failure of adaptation of the four choices
4. Conclusions and policy implications
The study examined the agro-pastoral farmers’ adaptation choices and determinants to climate change using cross-sectional data employing econometric model. Farmers were asked about the observed changes in main climate elements; hence they were mindful as temperature is increasing while rainfall is decreasing in the study periods. Respondents who perceived changes were using adaptation responses such as portfolio diversification, enhancing livestock productivity, agro-ecological practices and non-agricultural income diversifications. Lack of training and extension services, undefined reason, lack of climate information, lack of finance and credit and labour shortage were the main barriers for climate change adaptation in descending order. Multivariate probit model result also shown that covariates that positively influenced farmers’ adaptation were sex (being male), educational level, farming experience, goat flock size, farm income, frequency of extension contact, access to climate information and credit. Farm size and non-farm income of the farmer on the other hand had both positive and negative effect on diverse adaptation choices, whereas family size surprisingly had negative contribution to portfolio diversification.
The marginal success probability of adapting non-agricultural income diversification was lowest compared to adaptation using agro-ecological practices, portfolio diversification and enhancing livestock productivity. In general, farmers were more likely to fail than success in adopting all choice sets jointly. Responses to climate change needs a collective action and supportive policies at community, regional, national and global stages. Major changes within the farming system may be required to protect the livelihood since wealth and its correlates on top of farmers’ gender had an influence on adaptation choices. Therefore, putting pressure on communal land is not prudent rather promoting agricultural intensification through financing on inputs and technologies is efficient. Concerned organizations should focus on empowering female-headed farmers through creating conducive environment to access: inputs, credit, training and extension service related to climate change and agriculture.
Moreover, access to information, education level, frequency of extension contact and credit access were factors influencing adaptation choices to climate change so that concerned decision-making body “should think” of instituting local meteorology stations and community radio services to deliver too date information on climate. Refining awareness through promoting village-based vocational training would also hasten farmers’ climate change perception and adaptation choices. Extension agents in the community should be proficient on climate variation issues to provide sufficient advice and information on climate. The concerned bodies also should provide due attention in allocating experts at kebele/local level to offer regular visit to farmers as required.
Farmers were accessing credit from informal sources due to high interest rate in formal institutions so that the local government should focus on the provision of affordable credit service on top of diversifying livelihoods to opportunistic non-farm income generation. In this regard, small and micro enterprises can contribute most using households’ surplus labour as a resource. Furthermore, the current study used cross-sectional data, which limit observation across long-term changes in farmers’ climate change perception and adaptation. As a result, the limitation would be kept in mind in the course of conclusion evaluation.
Acknowledgements
The financial support of Amhara Region Agricultural Research Institute (ARARI) is greatly accredited. We also owe gratitude to all Agro-pastoral farmers for their unreserved cooperation. Finally, the authors would like to thank the anonymous reviewers for their constructive comments to improve the paper.
Additional information
Funding
Notes on contributors
Ademe Mihiretu
Ademe Mihiretu (MSc) is full time researcher of Socioeconomics and Agricultural Extension in Sekota Dryland Agricultural Research Center. He holds MSc degree in Rural Development with excellent accreditation to his thesis work from Haramaya University. Ademe has sound practical experience and solid meticulous talent in socioeconomics and extension research. He conducted research related to his area of specialization and produced five articles and eight proceedings published in ARARI annual proceedings.
Eric Ndemo Okoyo
Eric Ndemo Okoyo (PhD) is an Associate Professor in School of Agriculture and Environmental Science, Haramaya University. He has published 18 articles and 1 book in rural/regional development, Environmental Studies and Gender. Eric reviewed 10 articles from reputable journals and advised 80 MSc students.
Tesfaye Lemma
Tesfaye Lemma (PhD) is an Associate Professor in School of Agriculture and Environmental Science, Haramaya University; currently he is a senior lecturer and research fellow.
Notes
1. The smallest administrative unit in Ethiopia, parallel to Peasant Association (PA).
References
- Abeje, A., Ademe Mihiretu, L. A., & Tsega, D. (2016). Participatory evaluation of hybrid sorghum technologies: In mid and low land areas of Wag-Himra zone, Eastern Amhara, Ethiopia. Journal of Agricultural Extension and Rural Development, 8(5), 80–23.
- Amdu, B., Ayehu, A., & Deressa, A. 2012. Farmers’ perception and adaptive capacity to climate change and variability in the upper catchment of Blue Nile, Ethiopia (African Technology Policy Studies Network, ATPS working paper No77). doi:10.1094/PDIS-11-11-0999-PDN
- AWOA (Abergelie Woreda Office of Agricultural Development). (2016). The general agricultural and livestock basic information guide: Agricultural working report on: livestock and livestock characteristics. Niwrawaq, Abergelie. (Unpublished)
- Belayneh, L., Yared, A., & Bewket, W. (2012). Smallholder Farmers’ perception and adaptation to climate variability and climate change in Doba district, Western Hararghe, Ethiopia. Asian Journal of Empirical Research, 3(3), 251–265.
- Berman, R. (2014). Developing climate change coping capacity into adaptive capacity in Uganda. p. 253.
- Burney, J. (2014). Climate change adaptation strategies for smallholder farmers in the Brazilian Sertão’. Climatic Change, 126(1–2), 45–59. doi:10.1007/s10584-014-1186-0
- Cappellari, L., & Jenkins, S. P. (2003). Multivariate probit regression using simulated maximum likelihood. The Stata Journal: Promoting Communications on Statistics and Stata, 3(3), 278–294. doi:10.1177/1536867X0300300305
- Coulibaly, J. Y. (2015). Responding to crop failure: Understanding farmers’ coping strategies in Southern Malawi’. Sustainability (Switzerland), 7(2), 1620–1636.
- Devereux, S., & Guenther, B. 2007. Social protection and agriculture in Ethiopia. Country case study paper prepared for a review commissioned by the FAO on ‘Social protection and support to small farmer development’, Addis Ababa, Ethiopia. doi:10.1094/PDIS-91-4-0467B
- Evengelista, P., Young, N., & Burnett, J. (2013). How will climate change spatially affect agriculture production in Ethiopia? International Journal Devoted to the Description, Causes and Implications of Climatic Change, 119(3), 855–873.
- FAO (Food and Agricultural Organization for the United Nation). 2013. Guidelines for measuring household and individual dietary diversity (p. 53). Rome: Nutrition and consumer protection division, FAO.
- FAOSTAT (Food and Agriculture Organization Statistics). (2015). Food and Agricultural Organization of the United Nations, statistical division. Addis Ababa, Ethiopia. Retrieved from http://faostat3.fao.org/browse/Q/QA/
- Gebrehiwot, T., & van der Veen, A. (2013). Farm level adaptation to climate change: The case of farmer’s in the Ethiopian Highlands. Environmental Management, 52(1), 29–44. doi:10.1007/s00267-013-0039-3
- Halima, H., Lababidi, S., Rischkowsky, B., & Tibbo, M. (2012). Molecular characterization of Ethiopian indigenous goat populations. Tropical Animal Health and Production, 44(6), 1239–1246. doi:10.1007/s11250-011-0064-2
- ILRI (International Livestock Research Institute). (2013). Harnessing the genetic diversity of goats to improve productivity in Africa. University of Dschang, Retrieved from http://www.slideshare.net/ILRI/apm2013
- IPCC (Intergovernmental Panel on Climate Change). (2007). Climate Change 2007: impacts, adaptation and vulnerability: the fourth assessment report of the Intergovernmental Panel. Genebra, Suíça. doi: 10.1256/004316502320517344.
- IPCC (Intergovernmental Panel on Climate Change). (2011a). Climate change: The physical science basis. Contribution of working group I to the fourth assessment report of the IPCC. Cambridge. Cambridge University Press.
- Jones, P. G., & Thornton, P. K. (2008). Croppers to livestock keepers: Livelihood transitions to 2050 in Africa due to climate change. Environmental Science & Policy. doi:10.1016/j.envsci.2008.08.006
- Juana, J., Kahaka, Z., & Okurut, F. (2013). Farmers’ perceptions and adaptations to climate change in sub-Sahara Africa: A synthesis of empirical studies and implications for public policy in African agriculture. The Journal of Agricultural Science, 5(7), 121–135.
- Kassaye, H. (2010). Climate change and the vulnerability context of livestock and effects on livestock based livelihoods in Ethiopia. Proceedings of the 24th Annual conference of Ethiopian Veterinary Association. 2014, July 21–22, Addis Ababa, Ethiopia.
- Kebede, G. (2013). Spatial and temporal uncertainty of rainfall in arid and semi-arid areas of Ethiopia. Science, Technology and Arts Research Journal, 2(4), 106–113.
- Kemausuor, F., Dwamena, E., Bart-Plange, A., & Kyei-Baffour, N. (2011). Farmers’ perception of climate change in the Ejura-Sekyedumase district of Ghana. ARPN Journal of Agricultural and Biological Science, 6(4), 25–37.
- Lijalem, A., Tewodros, A., Luelseged, K., Haile, A., Rischkowsky, B., & Legese, G. (2013). Assessment of Abergelie goat value chain in northern Ethiopia: the case of Wag– Abergelie.
- Mc Donald, M., & Simon, J. (2011).Climate, food security and growth Ethiopia’s complex relationship with livestock. Policy Brief, 3, 123-124. Brighter Green (Unpublished).
- Miller, C. & Edwards, P. N. (2012). Changing the atmospheric expert knowledge and environmental governance. Massachusett: MIT press.
- MoA (Ministry of Agriculture), (2010, 15 September). Ethiopia’s agriculture sector policy and investment framework: Ten Years Road Map (2010-2020) (Draft Final Report).
- Mulatu, N. (2014). Determinants of farmers’ preference for adaptation strategies to climate change: Evidence from North Shoa Zone of Amhara Region, Ethiopia. American Journal of Social Sciences, 2(4), 56–66.
- Ndikumana, J. (2002). Copying mechanisms and their efficacy in disaster-prone pastoral systems of greater horn of Africa. Sustainable livelihoods of farmers and pastoralists in Eritrea.
- Nhemachena, C., & Hassan, R. (2007). Micro-level analysis of farmers’ adaptation to climate change in Southern Africa (IFPRI Discussion Paper No. 00714). Washington, D.C. doi:10.1094/PDIS-91-4-0467B
- Pauw, P. (2013). The role of perception in subsistence farmer adaptation in Africa-enriching the climate finance debate. International Journal of Climate Change Strategies and Management, 5(3), 3. doi:10.1108/IJCCSM-03-2012-0014
- Peter, J., & James. 2015. Perception of farmers on cassava as a potential crop for climate change adaptation in Kigoma region, Tanzania, p. 75.
- Philip, T., Mario, H., Ade, F., & Okeyo, M. (2013). Vulnerability, climate change and livestock research opportunities and challenges for poverty alleviation. International Livestock Research Institute (ILRI). SAT Ejournal, 4(1), 1–23.
- Piya, L., Maharjan, K. L., & Joshi, N. P. (2012a). Perceptions and realities of climate change among the Chepang communities in rural Mid-Hills of Nepal. Journal Contemporary India Studies, 2(1), 35–50.
- Solomon, A. K., Mwai, O., Grum, G., Haile, A., Rischkowsky, B., Solomon, G., & Dessie, T. (2014). Review of goat research and development projects in Ethiopia (ILRI project report). Nairobi, Kenya: International Livestock Research Institute.
- Stark, J., Terasawa, K., & Ejigu, M. (2011, October). Climate change and conflict in pastoralist regions of Ethiopia: Mounting challenges, emerging responses (CMM Discussion Paper No. 4). 1–54
- Tazeze, A., Haji, J., & Ketema, M. (2012). Climate change adaptation strategies of smallholder farmers: The case of Babilie district, East Harerghe Zone of Oromia Regional State of Ethiopia. Journal of Economics and Sustainable Development, 3(14), 2222–2855.
- Temesgen, D., Hassan, R. M., Ringler, C., Alemu, T., & Yesuf, M. (2009). Determinants of farmers’ choice of adaptation methods to climate change in the Nile Basin of Ethiopia. Global Environmental Change, 19(3), 248–255. doi:10.1016/j.gloenvcha.2009.01.002
- Tizale, C. Y. (2007). The dynamics of soil degradation and incentives for optimal management in the Central Highlands of Ethiopia (PhD thesis). Faculty of Natural and Agricultural Sciences, University of Pretoria, Pretoria, South Africa. doi:10.1094/PDIS-91-4-0467B
- Wilhite, D. 1985. Understanding the Drought Phenomenon: The Role of Definitions’. (August 2013), pp. 37–41.
- Wooldridge, J. M. (2006). Introductory econometrics, A modern approach (3rd ed.). Mason, OH: Thomson South Western.
- WSPA (World Society for the Protection of Animals). (2009). Livestock production and climate change. Submission to UNFCCC consultation on the role of livestock in climate change: February 2009.
- Yamane, T. (1967). Statistics: An introductory analysis. (2nd ed.). New York, NY: Harper and Row.
- Zelalem, Y., Aynalem, H., & Emmanuelle, G. (2009, Sep.24–26). Effect of climate change on livestock production and livelihood of pastoralists in selected pastoral areas of Borana, Ethiopia. Proceeding of 17th annual conference of Ethiopian Society of Animal Production (ESAP) (pp. 3–21). Addis Ababa, Ethiopia.
- Zing, N. (2003). Livestock production and climate change: An overview from some African countries. Drought in the Sahel. 302(5647), 999–1000. doi:10.1016/s0006-291x(03)00110-4.
Appendix
Table A1. Multicollinearity test of continuous explanatory variables
Table A2. Multicollinearity test of dummy explanatory variables
Table A3. Heteroskedasticity test of the dependent variables Ho: Constant variance
Table A4. Conversion factor of adult equivalent (labor force)
Table A5. Conversion equivalents of the Sub-Saharan Africa livestock into TLU
Table A6. The mean comparison of households’ multiple adaptation choices (MANOVA)