Abstract
In 2018, Nielsen and Kreiner provided evidence that the Attitudes and Relationship to Statistics—Revised (HFS-R) questionnaire provides essentially valid, objective, and reliable measurement of three statistical anxiety constructs and one statistical attitude construct among students of sociology and public health. The purpose of the study described in this paper was to revisit the HFS-R instrument to assess the degree to which the properties of the instrument and its subscales extends to students of psychology. The study confirmed that this was largely the case. HFS-R is again found to provide essentially valid, objective and reliable measures of a three-dimensional construct related to Statistical Anxiety and a unidimensional construct associated with attitudes towards statistics. This paper provides the documentation supporting these claims.
PUBLIC INTEREST STATEMENT
The research reports further evidence on the criterion-related construct validity of the Danish contemporary instrument (HFS-R) for the measurement of attitudes and relationship to statistics for use in higher education research. Analyses were conducted using Rasch measurement models. These models were chosen for their adherence to strict standards for measurement quality, and in order to be able to compare results to the original development study. The results confirm that the four short subscales (Test and Class Anxiety, Interpretation Anxiety, Fear of Asking for Help, and Worth of Statistics) have very good psychometric properties, that the three anxiety scales are separate scales and do not make up a single unidimensional scale, as suggested by other researchers. The research confirms that the instrument can be utilised in contemporary research on statistical anxiety and attitudes towards statistics.
1. Introduction
Statistical anxiety is the anxiety experienced in relation to learning statistics, and has by Zeidner (Citation1991) been described as feelings of extensive worry, intrusive thoughts, mental disorganization, tension, and physiological arousal, when encountering or contemplating statistics content or the context of learning statistics. There is no doubt that statistical anxiety is a pervasive problem within many higher education disciplines, as statistical anxiety has been linked to inadequate learning behaviour in students (e.g., Macher et al., Citation2011; Onwuegbuzie, Citation2004) as well to poorer academic outcome (e.g., Macher et al., Citation2011, Citation2013).
Having taught statistics for many years in several social science and health programs, where courses in statistics are methods courses linked to or integrated into the main academic discipline to varying degrees, we have ourselves experienced and dealt with student’s statistical anxiety and their attitudes towards statistics. These experiences eventually lead us to develop the HFS-RFootnote1 (Nielsen & Kreiner, Citation2018), in order to be able to assess statistical anxiety as well as attitudes towards statistics validly in the Danish higher education context.
While several instruments for the assessment of statistical anxiety exist, we developed the HFS-R on the basis of the Statistical Anxiety Rating Scale (STARS; Cruise et al., Citation1985; Cruise & Wilkins, Citation1980). 1) we reviewed 50 articles and found that the STARS was used in 78% of these, and thus chose the STARS as the basis for developing the HFS-R, with which the present study is concerned. 2) the STARS contains subscales addressing both statistical anxiety and attitudes towards statistics, which according to Chew and Dillon (Citation2014) it is a necessary to distinguish between statistical anxiety and attitudes towards statistics to get detailed insights into the psychological barriers for learning statistics.
The number of different dimensions measured by STARS is an important issue that a study of validity has to address. Nielsen and Kreiner (Citation2018) described how several lines of research have looked into this problem and have reached somewhat different conclusions. One line of research started with Hsiao’s (Citation2010) small sample CFA study, which suggested that the STARS is two-dimensional, and that composite scores should be formed across subscales relating to respectively anxiety and attitudes. This was followed up by a German research group, who, also based on CFA studies, came to the conclusion that the STARS is two-dimensional, and thus also proposed that anxiety and attitude composite scores should be formed and used instead of the six original subscales and scores (Macher et al., Citation2011, Citation2013; Papousek et al., Citation2012). In another line of research, stemming from various and seemingly non-connected researchers, additional CFA results supported the claims that STARS consists of the original six subscales and that these are correlated (Chew, Citation2018; DeVaney, Citation2016; Steinberger, Citation2020). However, other studies disagreed. For example, Chew (Citation2018, page 9) asserted that ” … it does not make substantiative sense to have six correlated factors in research” preferring the two scales measuring anxiety and attitude. In a third line of research, Nielsen and Kreiner (Citation2018) used Rasch models to test whether the three anxiety subscales in the HFS-R, derived from the STARS, were one unidimensional scale, and found that they were not.
Another important issue during assessment of the validity of measures of statistical anxiety is whether measurement is invariant across subgroups of students. Invariance is important because many previous studies have focused on group-wise differences of measurements of statistical anxiety and attitudes toward statistics provided by STARS. For example, Baloğlu et al. (Citation2011) found country-wise differences on four STARS subscales: American students scored higher than Turkish students on Interpretation Anxiety, Fear of Asking for Help, Fear of Statistics Teachers, and Worth of Statistics. In addition to this, they also provided evidence of gender differences on the Test and Class Anxiety and the Interpretation Anxiety subscales, with the female students obtaining the highest scores. More recently, a small-sample study of MacArthur (Citation2020) found differences between male and female undergraduate social science students attending an introductory course on statistics. Here female students scored higher than males on Test and Class Anxiety as well as Interpretation Anxiety one week into course, but they found no differences at the end of the semester.
However, none of these studies addressed the issue of measurement invariance of STARS subscales and thus whether differences between groups were due to measurement bias. These issues were taken up by Teman (Citation2013) disclosing evidence of differential item functioning (DIF) relative to level of study (undergraduate versus graduate students) for six STARS items. Furthermore, Nielsen and Kreiner (Citation2018) found evidence of DIF for four items of the HFS-R: one Interpretation Anxiety item—unchanged from the STARS—in relation to statistics course attended (first, second). Another Interpretation Anxiety item—very slight variation on STARS item 18—in relation to the academic program attended (sociology, public health). One Test and Class Anxiety item—very slight variation on STARS item 22—in relation to age (three age groups). And finally, on a Worth of statistics item—developed for the HFS-R—in relation to Statistics courses attended (first, second).
To our knowledge, only one previous study (Teman, Citation2013) has employed the Rasch model to investigate the validity of the six individual subscales of the STARS. Teman (Citation2013) used the rating-scale model (RSM; Andrich, Citation1978) for analyses of the six STARS subscales on data from a sample of 431 American university students. In addition to DIF (c.f. the above), Teman also found evidence of item misfit for 20 items that had to be eliminated from the scales. Thus, only 31 of 51 items remained, six of which suffered from DIF. Teman’s (Citation2013) findings suggested that only 21 items across the four subscales comparable to the HFS-R scales (Nielsen & Kreiner, Citation2018) employed in the present study were valid, and among these, three items should be adjusted for DIF relative to the study level.
Nielsen and Kreiner (Citation2018) developed the HFS-R to acquire a contemporary and valid Danish measure of attitudes and relationship towards statistics for use with higher education students taking statistics courses within other academic disciplines. During translation, two of the STARS subscales were excluded due to a lack of conceptual unidimensionality and derogatory content. In the remaining four subscales, single items were modified for face and content validity enhancement. Furthermore, construct validity was investigated using Rasch and graphical log-linear Rasch models to conduct item analyses including analysis of conditional independence of items and analysis for DIF. First with a pilot version of the instrument, and secondly with a revised version of the instrument. The final instrument consists of four subscales totalling 26 items; Test and Class Anxiety (TCA), Interpretation Anxiety (IA), Fear of Asking for Help (FAH), and Worth of Statistics (WS). The FAH subscale fitted the Rasch model, whereas the TCA, IA, and WS subscales each fit graphical log-linear Rasch models (GLLRMs) each with evidence of differential item functioning (DIF) (c.f. the above). The IA and TCA subscales were both found to be well targeted to the study population, while targeting of FAH and WS was poorer. Finally, unidimensionality across the three anxiety subscales (FAH, TCA, and IA) was rejected (c.f. the above).
Cui et al. (Citation2019) suggested that future research on statistical anxiety should address issues related to “improvement of measurement tools”. Unfortunately, their systematic overview of research on and definition of statistics anxiety did not include Teman’s (Citation2013) study of construct validity and evidence of problems. Nielsen and Kreiner (Citation2018) study documenting measurement quality in the HFS-R developed from the STARS was also unnoticed.
1.1. Assessment of validity and objectivity
Analyses attempting to provide validity of psychometric tests by assessment of fit of items to a psychometric model are confirmatory. The analysis presupposes a theoretical framework describing (1) the effects of the conjectural constructs on responses to items, (2) the association of the constructs and other variables, and (3) the populations where measurements of the constructs are meaningful. Even though all these prerequisites exist, psychometric analyses can never prove that the construct exists and that measurement by the items of a psychometric model is construct valid. Evidence supporting such claims always consists of conclusions that it has been impossible to falsify the assumptions of validity and the fit of responses to a psychometric model. For this reason, analyses of validity can never stand alone. The results depend on the power of the statistical tests and the risk of overlooking departures from the assumptions of the psychometric models.
The risk of overlooking evidence of misfit between the psychometric model and data depends on three essential issues. First, that the analysis addresses all assumptions of validity. During a test of fit of items to a Rasch model, it is not enough to calculate item fit statistics. Test of local independence, invariance and no DIF, and unidimensionality against meaningful multidimensional alternatives are also required. Second, that the statistical tests are unbiased, efficient and as powerful as possible. Many statistical tests are available and some are more powerful than others. In addition to this, some have questionable properties and some are biased with a high risk of either rejecting fit or accepting misfit. The choice of fit statistics is therefore crucial. Finally, sample size has to be adequate. Small-sample studies and studies with moderate samples with n <500 always entail a risk that something will be missed even though the above issues have been adequately addressed.
The previous study, Nielsen & Kreiner (Citation2018) addressed the first two issues as carefully as possible, but the sample was at best moderate and we cannot claim that nothing was overlooked and that statistical type II errors did not occur. For this reason, analysis of new data supporting the claims that measurement is valid is warranted. This paper tries to meet this requirement. Even though we can only describe the available sample size for the current study as moderate, failure to falsify the claims of the previous study, will strengthen the evidence supporting validity.
1.2. Assessment of reliability and targeting
Reliability and targeting of scale items are inherently population and sample dependent, and therefore need to be assessed in every study employing the scale.
Reliability depends on the variability in a sample. Reliability is only high if there is sufficient variability in the sample of persons. For example, with the present instrument, the reliability of the Fear of Asking for Help subscale was good for the student sample of sociology and public health students in the development study (Nielsen & Kreiner, Citation2018), as the inclusion of students from two different academic disciplines being taught and having different statistics curriculums, ensured enough variability in the sample. However, in a new study with a different student sample from a single academic discipline, such as the present sample, reliability might very well be lower, due to greater similarity within the sample.
Standard error measurement (SEM) and to some degree of measurement bias depend on the level of the trait a scale attempts to measure. Targeting is good if SEM is close to the smallest minimum SEM value that the instrument can provide precise measurement for the majority of person in the study population and less than good if measurement is imprecise for a large part of the population. Targeting depends on the degree to which items are aligned with the persons. In educational testing items that are too easy or too difficult for the students are out of target, thus providing little information on the majority of students and measurement will typically be imprecise. In the study of Fear of Asking for Help among sociology and public health students (Nielsen & Kreiner, Citation2018) targeting was poor because students were not even close to being fearful of asking for the kind of help reflected in the items of the scale. To provide precise measurement of the level fear of asking for help needed other items relating to help that there was more reason to evade asking for. However, in another sample of students in another academic discipline, with another teacher and with another curriculum, students might be more fearful of asking for help and thus be better aligned with the items, producing measurement that is more precise. If this were the case, the items would be better targeted to such a population than the population of sociology and public health students.
1.3. Research questions
The current study is a second validity study attempting to confirm or refute the results of Nielsen and Kreiner (Citation2018) within another student population. To fulfil this aim, we addressed the following research questions.
RQ1: Are the psychometric properties of the four HFS-R subscales comparable to those in a sample of sociology and public health students? Is measurement valid, precise and reliable?
Nielsen and Kreiner (Citation2018) addressed the issues of validity, precision and reliability of the HFS-R for the first time. The purpose of this study is to attempt to replicate the previous analysis on a different population of students and to compare the results of the different analyses. For this reason, the current study must provide answers to the following three questions.
RQ2: Do responses to items fit the same kind of IRT and Rasch models as in the previous study?
RQ3: Is local dependence (LD) and differential item functioning (DIF) among psychology students similar to LD and DIF among sociology and public health students?
RQ4: Do the analyses confirm that three anxiety subscales (TCA, IA and FAH) measure qualitatively different traits that cannot be combined to a single anxiety scale in a meaningful way?
2. Methods
2.1. Instrument
We use the same HFS-R instrument as in the previous study. We refer to Nielsen and Kreiner (Citation2018) for the development and validation of the HFS-R questionnaire (HFS-R; Holdninger og Forhold til Statistik—Revideret) and for details relating HFS-R to STARS.
The HFS-R contains 26 items collected in three subscales measuring anxiety and one subscale measuring attitudes: the Test and Class Anxiety scale (TCA) with 7 items, the Interpretation Anxiety scale (IA) with eight items, the Fear of Asking for Help (FAH) with five items, and the Worth of Statistics scale (WS) with six items (item are shown divided into subscales in the English version in the Appendix). The anxiety items in TCA, IA and FAH ask about the degree of anxiety the students experience in the situations related to their statistics course. Responses are defined by four ordinal categories (1 = no anxiety, 2 = a little anxiety, 3 = some anxiety, and 4 = a lot of anxiety). For the attitude scale, WS, students are asked to state their agreement with the statement in the items using another set of ordinal categories (1 = definitely disagree, 2 = disagree more than agree, 3 = agree more than disagree, and 4 = definitely agree).
The HFS-R questionnaire used in the current study as well as instructions for scoring are available from the first author in the Danish language version.Footnote2 As we are currently conducting a validation study of the English and German language versions of the HFS-R with colleagues in the UK, Germany, and Switzerland, the English language version could not be included with this article, but will be published as part of the documentation of the international validation study.
2.2. Participants and data collection
Data were collected from one Danish university in statistics classes for students enrolled in the Bachelor of Psychology degree program. Data were collected in the fifth statistics lecture with paper-pencil completed questionnaires in the first semester (i.e. 1 month into the BA psychology program) in 2-year cohorts of psychology students. Students had been informed of the data collection by the statistics lecturer beforehand, and the lecturer had allowed time for this within the lecture. Students were informed of the purpose of the data collection, how data would be utilized, that participation was voluntary, and they were provided with an information sheet which included contact information for the responsible researcher. In the questionnaire students actively consented to use of their data for research and were given the opportunity to also allow their data to be utilized by the statistics lecturer for exercises after proper anonymization and publication of research.
A total of 169 and 172 students consented to participate, corresponding to 76,8% and 78,9% of the two cohorts. The distribution of gender and age is provided for each cohort and the total study sample in . As we only had available information on gender and age for students, who chose to participate in the study, it was not possible to compare responders to non-responders. However, as data were collected just 5 weeks into the psychology program, the official information from the Danish ministry of Higher Education on admitted students to the Bachelor of Psychology degree program in the university in question, can add some insight, as the official number were only a few months older than our collected data.Footnote3 The higher frequencies of female students in the two cohorts reflect the gender distribution in the student population admitted to the program in the university in question in the 2-years data were collected; respectively 79.1% and 83.4% female students. It is not possible to compare directly the average age in our sample to those of the admitted students, as the Ministry of higher education only provide age categories. In the student population admitted to the program, the percentages of students in categories matching our categorization of age for DIF-analysis were; 29.6%, 25.2%, 45.2% respectively 34.8%, 24.8%, 40.4% in the 2 years.
Table 1. Gender and age distributions in the two cohorts of the study sample
2.3. Rasch models
The Rasch model (RM; Rasch, Citation1960) is a latent variable model. The RM is a member of the Item Response Theory (IRT) models with particularly desirable properties (Fischer & Molenaar, Citation1995; Christensen, Kreiner & Mesbah, Citation2013). In statistical terms, the RM is a parsimonious model describing the causal effect of a latent trait variable on responses to items. Contrary to other psychometric IRT and CFA models, the RM does not need assumptions on the distribution of the latent variable.
Since its conception, the RM has been associated with theories of measurement in philosophy of science meeting certain requirements that other psychometric models do not meet. However, Boorsboom (Citation2005) distinguishes between realist theories of measurement and representational measurement theories and claims that the RM addressing the sources of variation in test scores is an implementation of realist thinking. For this reason, Borsboom dismisses the claims that the RM is a necessary and sufficient requirement of the kind of fundamental measurement required by representational measurement theories. We agree with Borsboom. To us, the RM model as one among several latent variable models that may provide valid measurement of unobservable traits. We prefer to use the RM as our point of departure because it is a parsimonious model and because it is the only IRT model that may provide specific objective measurement (Rasch, Citation1961 and Boorsboom, Citation2005, page 125).
In Rasch and other IRT models, the value of the latent variable is treated as an unknown person parameter. Among such models, the Rasch model is unique because it is the only model where the raw score over all items is a sufficient statistic for the person parameter. Sufficiency is an attractive property for many reasons, one of which is that it allows assessment of fit and estimation of item parameters that do not depend on a specific distribution of the latent variable. We refer to Andersen (Citation1973) for information on the conditional maximum likelihood (CML) estimates of item parameters and the overall conditional likelihood ratio (CLR) tests used during the analysis of the HFS-R scales. Boorsboom (Citation2005, page 9) insists that the question of test validity is best considered within a framework defined by latent variable models. We agree and subscribe to the definition of criterion-related construct validity defined by Rosenbaum (Citation1989). Since the requirements for fit to the Rasch model meet Rosenbaum’s requirements we claim that RMs provide valid measurement. These requirements are (Kreiner, Citation2013):
Unidimensionality: The items of the scale must only assess one single underlying latent construct. In this case study: the TCA, IA, FAH, and WS subscales assess four different constructs. Taken separately they are unidimensional. The total anxiety score, TCA+IA+FAH is not unidimensional and therefore not construct valid.
Monotonicity: The expected item scores have to increase with increasing values of the latent variable.
Local independence of items (no local dependence; LD): Responses to items should be conditionally independent of the response given to another item of the scale given the latent variable. In other words, the partial correlations among items given the outcome on the latent variable should be equal to zero. Local independence implies that the response to an item only depends on the level of construct measured, and not on responses to other items.
Invariance and no differential item functioning (no DIF): Items and exogenous (i.e. background variables) should be conditionally independent given the latent variable. Responses to a scale items must only depend on the level of the construct measured. Gender or other subgroupings of students enrolled may have a direct effect on the construct being measured, but the effect on responses to items should be indirect mediated by the construct.
Homogeneity: Ranking of items by the expected item scores should be the same at all levels of the latent variable. In this case: the item requiring the least of the construct measured to be endorsed should be the same for students at a high level of the construct as for students at a lower level of the construct.
The first four requirements adhere to all parametric and non-parametric IRT models and provide criterion-related construct validity as defined by Rosenbaum (Citation1989). The requirement of homogeneity is only satisfied by double monotonic non-parametric IRT models (Mokken, Citation1971) and by the Rasch model.
In this study, we used the Partial Credit model (PCM; Masters, Citation1982) for ordinal categorical items generalizing Rasch model for dichotomous items. The PCM provides the same measurement properties as the model for dichotomous items (Mesbah & Kreiner, Citation2013).
2.3.1. Graphical log-linear Rasch models
DIF and/or local dependence (LD) are often challenging for measurement scales in psychology, sociology and health-related science, including the previous study of TCA, IA and WS scales. To avoid eliminating items from relatively small sets of items, Kreiner and Christensen ((Citation2002, Citation2004, Citation2007)) proposed a class of extended and generalized Rasch models referred to as graphical log-linear Rasch models (GLLRMs).
In GLLRMs, items may be locally dependent if the strength of the association between dependent items is constant across all levels of the latent variable and items may function differently in different subpopulations defined by background variables if the direct effect of the background variable on the item is constant across all levels of the latent variable. Kreiner and Christensen referred to DIF and LD satisfying these requirement as uniform DIF and uniform LD and claimed that Rasch models with uniform DIF and uniform LD provide essentially valid and objective measurement (Kreiner, Citation2007; Kreiner & Christensen, Citation2007).
Adding uniform LD and DIF to Rasch models was first proposed by Kelderman (Citation1984). The difference between Kelderman’s log-linear Rasch model and GLLRM is that GLLRMs insert log-linear Rasch model in multivariate chain graph models together with background variables and other variables.
The terminology of chain graph models refers to graphs because they use graphs with nodes representing variables to illustrate associations among variables. In these graphs, missing edges or arrows between nodes denote that the variables are conditionally independent, given the remaining variables in the model. An arrow connecting two variables in a chain graph model may refer to a causal relationship and undirected edges illustrate that the variables are conditionally dependent without causality assumed. We refer to Lauritzen (Citation1996) for a comprehensive introduction to the theory of graphical models.
Items in GLLRMs follow the same rules as other variables. Items that are not connected by an edge in a GLLRM graph are conditionally independent given the latent variable (i.e. items are locally independent). Likewise, if there is no arrow or edge between an item and a background variable it means that they are conditionally independent given the latent variable and the other variables in the model. In other words, that there is no DIF. Items are connected to the latent variable to indicate that the relationship is causal. Furthermore, if background variables are directly associated with the latent variable, it may - depending on the variable - indicate that measurement is criterion valid. shows the graphs defining the GLLRMs that fit the items of the four subscales of the HFS-R subscales. We suggest that the reader takes a look at these graphs before reading the rest of the paper.
Figure 1. The final models for the four HFS-R subscales. Test and Class Anxiety (top left), Fear of Asking for Help (top right), interpretation anxiety (bottom left), worth of statistics (bottom right)
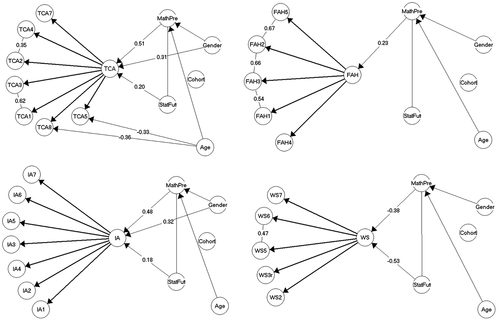
In supplemental file 2, we have provided further explanations of the methods used for the particularly interested reader. First, on how GLLRMs attempt to do the same for item analysis by RMs as structural equation models do for confirmatory factor analysis (CFA). Second, on essential validity and objectivity.
2.3.2. Item analysis by Rasch models and GLLRMs
A rigorous test of the fit of an RM or a GLLRM to a set of items in a single scale includes the following steps:
Overall test of homogeneity of item parameters across low and high scoring groups.
Overall tests of invariance relative to important background variables.
Tests of no DIF for all items relative to the same background variables.
Tests of local independence for all item pairs.
Fit of the individual items to the RM.
Tests of unidimensionality if subject matter considerations suggest that more than one latent variable could lie behind item responses.
The steps may do not need to be taken in the order presented above. If evidence of LD or DIF turns up, log-linear interactions are added to the model and the steps repeated until no further evidence is disclosed.
When the final step has been taken the following steps conclude the analysis.
Person parameters estimates, standard error and bias of measurement are assessed.
Targeting and reliability relative to the current study population are evaluated.
If analyses of validity include fit of models to more than one subscale, the analysis includes tests of unidimensionality across these to confirm that subscales measure different latent traits.
In this study, the fit of individual items to the RM was tested by comparing the observed item-rest-score correlations with the expected item-restscore correlations under the model (Christensen & Kreiner, Citation2013; Kreiner & Christensen, Citation2004). Overall tests of homogeneity and invariance were conducted using Andersen conditional likelihood ratio test (CLR; Andersen, Citation1973). The local independence of items and absence of DIF was tested using Kelderman’s (Citation1984) likelihood-ratio test, and if evidence against these assumptions were discovered the magnitude of the local dependence of items and/or DIF was informed by partial Goodman–Kruskal gamma coefficients conditional on the restscores (Kreiner & Christensen, Citation2004). DIF-analyses were done in relation to five background variables: Cohort (1, 2), perceived adequacy of mathematics ability (more than adequate, adequate, less than adequate), expectation to work with statistics in the future (yes, maybe, no), gender (male, female), and age group (20 years and younger, 21 years, 22 years and older).
Reliability was calculated with Hamon and Mesbah's (Citation2002) Monte Carlo method, which takes into account any local dependence between items in a GLLRM. Targeting was assessed numerically with two indices (Kreiner & Christensen, Citation2013): the test information target index (the mean test information divided by the maximum test information) and the root mean squared error target index (the minimum standard error of measurement divided by the mean standard error of measurement). Both indices should have a value close to one. We also estimated the target of the observed score and the standard error of measurement of the observed score. Lastly, to provide a graphical illustration of targeting and test information, we plotted item maps showing the distribution of the person locations against the item location, with the inclusion of the information curve. Person location was plotted as weighted maximum likelihood estimates of the person parameters (i.e. the latent scores) and person parameter estimates assuming a normal distribution (i.e. the theoretical distribution). Item locations were plotted as item thresholds.
Unidimensionality across the three anxiety scales (TCA, IA & FAH) scales was tested by comparisons of the observed and expected gamma (γ) correlation of the TCA, FAH, and WS subscales (Horton et al., Citation2013). Scales measuring different constructs will be significantly weaker correlated than what is expected under the common unidimensional model.
All the test statistics effectively tests whether item response data comply with the expectations of the model, and all results were evaluated in the same way; significant p-values signify evidence against the model. In line with the recommendations by Cox et al. (Citation1977), we evaluated p-values as a continuous measure of evidence against the null, distinguishing between weak (p < 0.05), moderate (p < 0.01), and strong (p < 0.001) evidence against the model, rather than applying a deterministic critical limit of 5% for p-values. Furthermore, we used the Benjamini and Hochberg (Citation1995) procedure to control the false discovery rate (FDR) due to multiple testing to reduce the amount of false evidence against the model.
2.4. Software
All item analysis was conducted with the Diagram software package (Kreiner, Citation2003; Kreiner & Nielsen, Citation2013), while the item maps were generated in SPSS.
3. Results
This section only reports the final results for the four subscales. Additional results are provided in Supplementary file 1.
After exclusion of item IA8 (Seeing a fellow student concentrating on their output from statistical analyses), the 7-item IA scale fitted a pure Rasch model (RM) with no evidence against homogeneity or invariance relative to cohort, gender, age, perceived adequacy of mathematics ability, or expectation to work with statistics in the future (, ).
Table 2. Global tests of homogeneity and invariance for the final HFS-R subscale models in
The analysis disclosed evidence of LD in TCA, FAH, and WS subscales and evidence of DIF relative to age in TCA. Since all subscales fitted GLLRMs, we claim that measurement by these subscales is essentially valid. shows the GLLRMs. shows the CLR tests of homogeneity and invariance. Table S1 presents the item fit statistics and Table S2 shows the CLR tests supporting the claims of LD and DIF together with partial gamma coefficients measuring the strength of LD and the effect of Age on two FAH items. includes a total CLR test for the four-dimensional GLLRM defined by the four subscales. Like the CLR tests of the separate subscales, the total test makes no assumptions concerning the distributions of the four latent variables. For this reason, the total CLR tests do not assume that the latent variables are independent. Table S1 in the supplemental file 1 shows item fit statistics for each of the subscales.
3.1. Local dependence
The analyses did not reveal any evidence against conditional independence of the IA item, but disclosed evidence against conditional independence among some items in the TCA, FAH, and WS subscales (). Four items in the TCA subscale were pairwise locally dependent, with strong partial γ correlation (items TCA2 and TCA4) and very strong (items TCA1 and TCA3) (, top left). The first locally dependent pair of items was TCA1 (Studying for an examination in a statistics course) and TCA3 (Doing the final examination in a statistics course), and the second pair was TCA2 (Doing the homework for a statistics course) and TCA4 (participating in statistics exercises).
In the FAH subscale, four items were locally dependent on strong partial γ correlations between these items were very strong (, top right). The locally dependent item pairs were: FAH1 (Going to ask my statistics teacher for individual help with material I am having difficulty understanding) and FAH3 (Asking one of your tutors for help in understanding a printout), FAH3 and FAH2 (Asking for help with statistical analyses in class), and FAH2 and FAH5 (Asking a fellow student for help in understanding a printout).
Finally, two items in the WS subscale as locally dependent, and strongly so (, bottom right): WS5 (Statistics is useful) and WS6 (Statistics is interesting).
3.2. Effect of differential item functioning
Evidence of differential item functioning was discovered only in the analysis of the TCA subscale. For this reason, the raw sum scores of the TCA subscale had to be equated for the DIF, to make scores comparable across age groups. The score-equation table is provided as Table S4 in the supplemental file 1, while the DIF results and the effect of adjusting for the DIF are presented below in .
Table 3. Comparison of observed and equated mean TCA scores in age groups
TCA item 5 (Finding that another student in class got a different answer than you did to a statistical problem) and item 8 (Going through an exam assignment in statistics after the grade has been given) both functioned differentially relative to age with decreasing risk of reporting anxiety with increasing age. shows that the difference in the mean scores for age groups became larger as a result of adjustment for DIF. In addition to this, the average observed scores in age groups 18–20 and 21 were not significant whereas the differences of average equated scores were significant (p = 0.007). In this case, adjustment for DIF changed the outcome of comparisons of TCA scores in different age groups.
3.3. Targeting and reliability
summarizes the results of the analysis of targeting and reliability. In this table, the target of a subscale is the value of the person parameter where test information is maximized and standard error of measurement assessed by the RMSE is minimized. To assess the degree of targeting the table reports the average person parameter under assumption that the distribution of persons is normal together with the average test information and the average RMSE in the study population. Target indices are defined by the ratio between the average test info and the maximum test info and by the ratio between the minimal RMSE and the average RMSE in the study population. Targeting was more than adequate for the
Table 4. Targeting and reliability of the test and class anxiety, fear of asking for help, interpretation anxiety and worth of statistics subscales
TCA, IA and WS scales, but less than adequate for the FAH that only collected 52% of the maximum test information.
The item maps in tell the same story relating RMSE and test info to the distribution of students. Item maps for the TCA scale in the three age groups demonstrate this very good targeting in different ways; panel A shows how the distributions of the person parameters and the item thresholds appear well aligned in their location on the latent TCA scale for the three age groups. Panel B of shows that even though the test information can be considered to be high, it is higher in the upper half of the estimated person parameter, when assuming that this is normally distributed, and thus targeting is better for the two oldest groups as the range with the highest information covers more of the students in these groups. Finally, panel C in shows how the standard error of measurement (SEM) is also rather high along the estimated person parameter under the assumption of a normal distribution, but highest at the low end of the latent TCA scale (the opposite of the test information).
Figure 2. Item maps for the test and class anxiety TCA subscale (TCA) in subgroups defined by age groups (DIF variable)
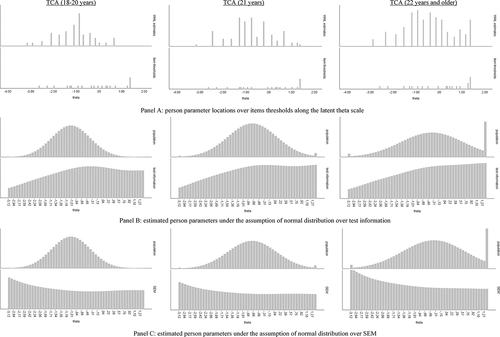
The IA scale was equally well targeted for all students (88% of the maximum test information obtained on average), as was the WS scale (80%) (). The item maps for the IA and WS scales show that while most information was found in the higher half of the IA scale and in the lower half of the WS scale, the level of information was generally high and thus well aligned with the student locations ().
Targeting was poor for the FAH scale, as only between 52% of the maximum test information was obtained on average (). The item map revealed that the alignment between student locations and item threshold was poor as students were located further towards the lower end of the scale (). Furthermore, high information was only present for the higher end of the scale, where hardly any students were located.
The reliability of the TCA scale was found to be at a less than satisfactory level by conventional standards (i.e. r < 0.70) only for the group of students aged 20 years and younger (). The highest reliability was found for the IA scale (r = 0.83).
Table 5. Test of unidimensionality of the anxiety subscales
3.4. Unidimensionality of the anxiety subscales
In order to re-test the assumption of unidimensionality across the three anxiety subscales proposed in previous research (Macher et al., Citation2011; Papousek et al., Citation2012) and rejected in more recent research (Nielsen & Kreiner, Citation2018), pairwise tests of the hypothesis of unidimensionality were conducted for the three anxiety subscales. The test compares the observed and expected correlations between subscale scores and rejects the hypothesis of unidimensionality if the observed correlation is weaker than the expected correlation under a unidimensional model. We refer to Horton et al. (Citation2013, pages 145–146) for information on the test. shows the results. The three subscales are significantly correlated implying that the same is true for the underlying latent traits, but correlations are significantly weaker than expected under unidimensionality. Unidimensionality is rejected.
4. Discussion
4.1. Differential item functioning
In the current study, we included a total of five background variables for DIF analyses. Three of these were also included in Nielsen and Kreiner (Citation2018), namely gender, age, and perceived adequacy of mathematics ability. In line with the previous study, we found no evidence of DIF relative to gender or perceived adequacy of mathematics ability, in this study. However, as in the previous study, we presently found evidence of age-related DIF in the TCA subscale, and even though two TCA items had DIF in the present study versus only one in the previous study, the magnitude was the same and likewise resulting in increasing bias the older the students were.
In the current study, we did not test for DIF relative to first or second statistics course or academic discipline, as we included only psychology students taking their first statistics course. Instead, we included cohort and expectation to work with statistics in the future in the DIF analyses and found evidence for neither.
4.2. Locally dependent items
For the TCA subscale, we found evidence against local independence of item pairs TCA2-TCA4 and TCA1-TCA3, which are the same items found to be locally dependent in Nielsen and Kreiner (Citation2018) and with the same magnitude of the correlations. With regards to the IA subscale, we found no evidence against fit to the Rasch model in the current study, which is slightly different to Nielsen and Kreiner (Citation2018), where two items lacked local independence (IA2 and IA5). For the FAH subscale, we found evidence against local independence of items FAH1, FAH2, FAH3, and FAH5 in the current study, whereas Nielsen and Kreiner in the 2018 study found no evidence against fit to the Rasch model. With regards to the WS subscale, we found evidence of against local independence of items WS5 and WS6, which was not an issue in the 2018 study. The biggest discrepancy between the current and the 2018 study was, however, that we in the current study discovered against the fit of item WS8 in the WS subscale such that we had to eliminate the item to obtain a fitting model, whereas Nielsen and Kreiner (Citation2018) study found no evidence against fit to the Rasch model for all six items of this scale.
4.3. Reliability and targeting
Compared to Nielsen and Kreiner (Citation2018), reliability of the TCA subscale for the current sample of psychology students was comparable to that reported for sociology and public health students, a little lower for the FAH subscale, and a little higher for the IA and WS subscales. Targeting in the current study was a little better for the TCA subscale compared to the 2018 study, the same for the FAH and IA subscales, and substantially better for the WS subscale.
Testing the fit of Rasch models and does not require specific distributions or sampling of persons. And since assessment of fit of these models is what we need to assess whether measurement is valid we do not need to be concerned about whether subpopulations are equivalent or samples representative. What we need are samples including student at high or low levels of the traits to test for homogeneity and background variables to test for invariance and DIF. However, in addition to students at all levels we need items with targets distributed across the entire range of the student distribution on the scale in order to obtain good targeting. While the low reliability for some groups of students on the TCA subscale, might be due to lack of variation, it is evident across the two studies that the poor targeting of the FAH subscale is due to the lack of items that are relatively easy do endorse; i.e. items on fear of asking for help which requires less fear to be endorsed, as the students from the three included academic disciplines are clearly not very fearful of asking for help.
4.4. Implications for future research and application of scales
The psychometric differences between construct validity as defined by Rosenbaum (Citation1989) (c.f. the method section) and the notion of essential validity as defined by Kreiner (Citation2007) and Kreiner and Christensen (Citation2007) (c.f. supplemental file 2), invites different action depending on the definition followed. Thus, for researchers who are uncomfortable with the definition of essential validity and prefer to follow the Rosenbaum definition, or who does not have access to specialized software for analysis by log-linear Rasch models, there are two possible courses of action to ensure validity.
The first is to eliminate locally dependent items and items suffering from DIF. It follows from the results of this paper that measurement by TCA and FAH is not possible, if such items are eliminated, because TCA7 and FAH4 would be the only items surviving the elimination. The WS subscale does better with three remaining items (WS2, WS3r, and WS7), but the effect of elimination on SEM and bias would be considerable.
The second is to replace locally dependent items by super items and to split items suffering from DIF into different items, one for each value of the origin of DIF. Replacement of locally dependent items by super items would mean that measurement by TCA would be based on TCA1+ TCA3, TCA2+ TCA4, TCA5, TCA7, and TCA8, out of which two items suffer from DIF relative to age. Measurement by FAH would be provided by two items: FAH12+ FAH2+ FAH3+ FAH5 and FAH4, but the analysis cannot provide information on the four items included in the super item. Finally, measurement by WS would depend on four items, WS2, WS3r, WS5+ WS6 and WS7. Splitting items for DIF would mean that in TCA, both TCA5 and TCA8 will be redefined as three items, one for each age group with missing responses in the other age groups. These options are also available for Rasch analyses by RUMM2030 (Andrich, Sheridan and Luo, Citation1978), and the RUMM2030 model is formally the same as the log-linear Rasch model, as both splitting items and making super items strictly speaking also only provides essentially valid and objective measurement.
To us, the above alternatives are not preferable to the application of GLLRM and the concept of essential validity, for a number of reasons: we cannot recommend sacrificing measurement of two important dimensions of statistical anxiety (Test and Class Anxiety and Fear of Asking for Help), simply because there are issues with lack of independence between items and DIF, when these issues can be dealt with efficiently, as demonstrated in this paper or in ways commonly used with both Rasch analysis and other IRT analysis. Elimination of the items with these issues would leave us with one item in the TCA subscale and two items in the FAH scale, which de facto would not be scales. Single item “measurement” would be very imprecise, and validity could not be assessed. Thus, we prefer to retain content relevant items, when these can be shown to fit a GLLRM with uniform locally dependent items and/or uniform DIF (i.e. an IRT model) and adjusting the person parameter estimates accordingly, and to openly show that this is what we do rather than attempting to dismiss evidence of local dependence or DIF to live up to certain definitions of validity.
In summation, we found minor differences between the models for psychology students (this study) and the models for sociology and public health students (Nielsen & Kreiner, Citation2018), which has to be looked into in the next studies:
This misfit of item WS8 in the current study. That the item “Statistics provides the most objective and firm knowledge” was excluded for psychology students and not sociology and public health students, might allude to substantial differences in the attitudes towards statistics for students within these three disciplines.
The local dependencies among FAH items in the current study. Local independence was accepted in the 2018 study, however, with p-values close to significance, and for this reason we cannot rule out that the missing interactions among items in 2018 were indeed Type I errors.
The test of item fit of IA5 was weakly significant (p = 0.01) in the current study, but was disregarded by the Benjamini and Hochberg (Citation1995) procedure adjusting for multiple testing. However, the 2018 study found evidence of local dependence between items IA2 and IA5. For this reason, we cannot rule out that our acceptance of the local independence of IA items in the current study could be another Type I error.
To resolve whether the few differences between the 2018 and the current study, might be due to type I or type II errors (too many or too few significant results depending on the study of focus) or whether they allude to more substantial differences, we intend to conduct further studies with the HFS-R, and encourage other researchers to do the same: we intend to do a joint analysis of the part of the two data set that are directly comparable; i.e. students in taking their first university statistics course, while also collecting additional data to improve power in the item analyses. An expanded version of the HFS-R should be developed for the purpose of improving the targeting of the Fear of Asking for Help subscale by adding items that are easier to endorse. Furthermore, we are presently conducting a validation study with the English language version of the HFS-R in the UK as well as a validation study with the German language version in Germany and Switzerland. These studies will also enable cross-cultural item analysis and unbiased comparisons of statistical anxieties and worth of statistics, for example, within specific academic disciplines.
5. Conclusion, implications, and future research
Despite some differences between the 2018 and 2020 models, we conclude that the current study extends the previous findings to psychology students, as the four subscales fitted either Rasch or graphical log-linear Rasch models. Statistical anxiety is a three-dimensional construct and measurement of statistical anxiety and worth of statistics by the HFS-R is essentially valid and objective. While the Fear of Asking for Help subscale should be expanded to improve targeting, the HFS-R might already assist statistics teachers in designing courses and conducting classes in statistics within other academic disciplines such as psychology, sociology, and public health studies, in manners appropriate to the level of anxiety and attitudes by providing insight into student anxiety and attitudes that are associated with outcome of statistics classes.
Supplemental Material
Download PDF (1.8 MB)Acknowledgements
We would like to thank all participating students and teachers for providing the opportunities for data collection.
Supplementary material
Supplemental data for this article can be accessed here
Additional information
Funding
Notes on contributors
Tine Nielsen
Tine Nielsen is an Associate Professor at the Department of Applied Research in Education and Social Sciences, UCL University College, Odense, Denmark. Her research is focused within the fields of applied educational research, higher education psychology, and psychometrics applied to instruments with relevance for education. The present study is one in a series of validity studies aimed at providing the Danish educational research community with valid and reliable measures. She is an active member of several education as well as psychometric societies and network groups, e.g., the European Association for Research in Learning and Instruction (EARLI), The European Rasch Research and Teaching Groups (ERRTG), and the Danish Measurement network (DM), which she founded in 2016. She is a subject editor (higher education and psychometrics) at the Scandinavian Journal of Educational Research. Svend Kreiner is a Professor Emeritus at the Biostatistics Unit at the Department of Public health, University of Copenhagen.
Notes
1. HFS-R is short for “holdninger og forhold til statistik—Revideret” in Danish. In English the equivalent would be “Attitudes and Relationship to Statistics—Revised”
2. The Danish HFS-R is available from the first author here: here
3. Our data were collected at the end of September. Official intake data made public every year on July 28th, Source: The Danish Ministry of Higher Education—webpage only available in Danish: https://ufm.dk/uddannelse/statistik-og-analyser/sogning-og-optag-pa-videregaende-uddannelser/grundtal-om-sogning-og-optag/ansogere-og-optagne-fordelt-pa-kon-alder-og-adgangsgrundlag
References
- Andersen, E. B. (1973). A goodness of fit test for the Rasch model. Psychometrika, 38(1), 123–20. https://doi.org/https://doi.org/10.1007/BF02291180
- Andrich, D. (1978). A rating scale formulation for ordered response categories. Psykometrika, 43, 561–573.
- Baloğlu, M., Deniz, M. E., & Kesici, Ş. (2011). A descriptive study of individual and cross-cultural differences in statistics anxiety. Learning and Individual Differences, 21(4), 387–391. https://doi.org/https://doi.org/10.1016/j.lindif.2011.03.003
- Benjamini, Y., & Hochberg, Y. (1995). Controlling the false discovery rate: A practical and powerful approach to multiple testing. Journal of the Royal Statistical Society. Series B (Methodological), 57 (1), 289–300. http://www.jstor.org/stable/2346101
- Boorsboom, D. (2005). Measuring the mind. Conceptual issues in contemporary psychometrics. Cambridge University Press.
- Chew, D. (2018). An examination of the internal consistency and structure of the Statistical Anxiety Rating Scale (STARS). PloS One, 13(3), e0194195–e0194195. https://doi.org/https://doi.org/10.1371/journal.pone.0194195
- Chew, P. K., & Dillon, D. B. (2014). Statistics anxiety update: Refining the construct and recommendations for a new research agenda. Perspectives on Psychological Science, 9(2), 196–208. https://doi.org/https://doi.org/10.1177/1745691613518077
- Christensen, K. B., & Kreiner, S. (2013). Item fit statistics. In K. B. Christensen, S. Kreiner, & M. Mesbah (Eds.), Rasch models health (pp. 83–104). ISTE and John Wiley & Sons, Inc. https://doi.org/https://doi.org/10.1002/9781118574454.ch1
- Christensen, K. B., Kreiner, S., & Mesbah, M. (2013). Rasch models in Health. ISTE & John Wiley & Sons.
- Cox, D. R., Spjøtvoll, E., Johansen, S., van Zwet, W. R., Bithell, J. F., Barndorff-Nielsen, O., & Keuls, M. (1977). The role of significance tests [with discussion and reply]. Scandinavian Journal of Statistics, 4 (2), 49–70. http://www.jstor.org/stable/4615652
- Cruise, R. J., Cash, R. W., & Bolton, D. L. (1985). Development and validation of an instrument to measure statistical anxiety. Paper presented at the proceedings of the american statistical association.
- Cruise, R. J., & Wilkins, E. M. (1980). STARS: Statistical anxiety rating scale, unpublished manuscript. Andrews University, Berrien Springs.
- Cui, S., Zhang, J., Guan, D., Zhao, X., & Si, J. (2019). Antecedents of statistics anxiety: An integrated account. Personality and Individual Differences, 144, 79–87. https://doi.org/https://doi.org/10.1016/j.paid.2019.02.036
- DeVaney, T. A. (2016). Confirmatory factor analysis of the statistical anxiety rating scale with online graduate students. Psychological Reports, 118(2), 565–586. https://doi.org/https://doi.org/10.1177/0033294116644093
- Fischer, G. H., & Molenaar, I. W. (Eds.). (1995). Rasch models – Foundations, recent developments, and applications. Springer-Verlag.
- Hamon, A., & Mesbah, M. (2002). Questionnaire reliability under the Rasch model. In M. Mesbah, B. F. Cole, & M. L. T. Lee (Eds.), Statistical methods for quality of life studies. design, measurement and analysis. (pp. 155–168) Kluwer Academic Publishers. https://doi.org/https://doi.org/10.1007/978-1-4757-36250_13
- Horton, M., Marais, I., & Christensen, K. B. (2013). Dimensionality. In K. B. Christensen, S. Kreiner, & M. Mesbah (Eds.), Rasch models health (pp. 137–158). ISTE and John Wiley & Sons, Inc. https://doi.org/https://doi.org/10.1002/9781118574454.ch9
- Hsiao, T. Y. (2010). The statistical anxiety rating scale: Further evidence for multidimensionality. Psychological Reports, 107(3), 977–982. https://doi.org/https://doi.org/10.2466/07.11.pr0.107.6.977-982
- Kelderman, H. (1984). Loglinear Rasch model tests. Psychometrika, 49(2), 223–245. https://doi.org/https://doi.org/10.1007/BF02294174
- Kreiner, S., & Christensen, K. B. (2002). Graphical Rasch models. In M. Mesbah, B. F. Cole, & M.-L. T. Lee (Eds.), Statistical Methods for quality of life studies: Design, measurements and analysis (pp. 187–203). Springer US. https://doi.org/https://doi.org/10.1007/978-1-4757-3625-0_15
- Kreiner, S. (2003). Introduction to DIGRAM. Copenhagen. Department of Biostatistics, University of Copenhagen.
- Kreiner, S., & Christensen, K. B. (2007). Validity and objectivity in health-related scales: Analysis by graphical loglinear Rasch models. In von Davier & Carstensen (Eds.), Multivariate and mixture distribution Rasch models (pp. 329–346). Springer. https://doi.org/https://doi.org/10.1007/978-0-387-49839-3_21
- Kreiner, S. (2007). Validity and objectivity: Reflections on the role and nature of Rasch models. Nordic Psychology, 59(3), 268–298. https://doi.org/https://doi.org/10.1027/1901-2276.59.3.268
- Kreiner, S., & Christensen, K. B. (2013). Person parameter estimation and measurement in Rasch models. In K. B. Christensen, S. Kreiner, & M. Mesbah (Eds.), Rasch models health (pp. 63–78). ISTE and John Wiley & Sons, Inc. https://doi.org/https://doi.org/10.1002/9781118574454.ch4
- Kreiner, S. (2013). The Rasch model for dichotomous items. In K. B. Christensen, S. Kreiner, & M. Mesbah (Eds.), Rasch models health (pp. 5–26). ISTE and John Wiley & Sons, Inc. https://doi.org/https://doi.org/10.1002/9781118574454.ch1
- Kreiner, S., & Christensen, K. B. (2004). Analysis of local dependence and multidimensionality in graphical loglinear Rasch models. Communication in Statistics –theory and Methods, 33(6), 1239–1276. https://doi.org/https://doi.org/10.1081/sta-120030148
- Kreiner, S., & Nielsen, T. (2013). Item analysis in DIGRAM 3.04. Part I: Guided tours. Research report 2013/06. University of Copenhagen, Department of Public Health.
- Lauritzen, S. L. (1996). Graphical models. Clarendon Press.
- MacArthur, K. (2020). Avoiding over-diagnosis: Exploring the role of gender in changes over time in statistics anxiety and attitudes. Numeracy: Advancing Education in Quantitative Literacy, 13(1), 1. https://doi.org/https://doi.org/10.5038/1936-4660.13.1.4
- Macher, D., Paechter, M., Papousek, I., & Ruggeri, K. (2011). Statistics anxiety, trait anxiety, learning behavior, and academic performance. European Journal of Psychology of Education, 27(4), 483–498. https://doi.org/https://doi.org/10.1007/s10212-011-0090-5
- Macher, D., Paechter, M., Papousek, I., Ruggeri, K., Freudenthaler, H. H., & Arendasy, M. (2013). Statistics anxiety, state anxiety during an examination, and academic achievement. British Journal of Educational Psychology, 83(4), 535–549. https://doi.org/https://doi.org/10.1111/j.2044-8279.2012.02081.x
- Masters, G. N. (1982). A Rasch model for partial credit scoring. Psychometrika, 47(2), 149–174. https://doi.org/https://doi.org/10.1007/BF02296272
- Mesbah, M., & Kreiner, S. (2013). The Rasch model for ordered polytomous items. In K. B. Christensen, S. Kreiner, & M. Mesbah (Eds.), Rasch Models Health (pp. 27–42). ISTE and John Wiley & Sons, Inc. https://doi.org/https://doi.org/10.1002/9781118574454.ch2
- Mokken, R. J. (1971). A theory and procedure of scalar analysis. Mouton & Co.
- Nielsen, T., & Kreiner, S. (2018). Measuring statistical anxiety and attitudes towards statistics: Development of a comprehensive Danish instrument (HFS-R). Cogent Education, 5(1), 1521574. https://doi.org/https://doi.org/10.1080/2331186X.2018.1521574
- Onwuegbuzie, A. J. (2004). Academic procrastination and statistics anxiety. Assessment & Evaluation in Higher Education, 29(1), 3–19. https://doi.org/https://doi.org/10.1080/0260293042000160384
- Papousek, I., Ruggeri, K., Macher, D., Paechter, M., Heene, M., Weiss, E. M., … Freudenthaler, H. (2012). Psychometric evaluation and experimental validation of the statistics anxiety rating scale. Journal of Personality Assessment, 94(1), 82–91. https://doi.org/https://doi.org/10.1080/00223891.2011.627959
- Rasch, G. (1960). Probabilistic models for some intelligence and attainment tests. Danish Institute for Educational Research.
- Rasch, G. (1961). On General Laws and the Meaning of Measurement in Psychology. The Regents of the University of California. Available from: http://projecteuclid.org/euclid.bsmsp/1200512895
- Rosenbaum, P. R. (1989). Criterion-related construct validity. Psychometrika, 54(4), 625–633. https://doi.org/https://doi.org/10.1007/BF02296400
- Steinberger, P. (2020). Assessing the statistical anxiety rating scale as applied to prospective teachers in an Israeli teacher-training college. Studies in Educational Evaluation, 64, 100829. https://doi.org/https://doi.org/10.1016/j.stueduc.2019.100829
- Teman, E. D. (2013). A Rasch analysis of the statistical anxiety rating scale. Journal of Applied Measurement, 14(4), 414–434.
- Zeidner, M. (1991). Statistics and mathematics anxiety in social science students: Some interesting parallels. British Journal of Educational Psychology, 61(3), 319–328. https://doi.org/https://doi.org/10.1111/j.2044-8279.1991.tb00989.x