Abstract
The primary aim of the study was to examine the validity of the Indonesian version of the 23-item WhatsApp Social Support Scale (WSSC) adapted from an earlier study by Chung, Yang and Chen . A total of 214 in-service English as a foreign language (EFL) teachers across school levels in Indonesia participated in the study. Rasch statistical analyses were performed to validate the adapted questionnaire, including unidimensionality assessment, reliability analysis, Rasch model fit analysis, and differential functioning analysis. The findings of the study showed that WSSC primarily measured a single latent variable, that was, the online social support received by the English as a foreign language (EFL) teachers in the WhatsApp group. The Rasch analysis of the WSSC resulted that the scale fitted the Rasch model with high reliability levels for both person and item levels. Although redefining category 2–3 is required, an evaluation of WSSC items with DIF notes is suggested to improve the scale’s reliability.
PUBLIC INTEREST STATEMENT
Surveys with a rating scale have been regarded as a common method for collecting data about specific types of educational, social and behavioural constructs. A good survey must possess a sufficient level of reliability before its use as a data-collecting instrument. Unfortunately, many validation analyses of the survey have revealed two critical issues, including the inappropriate use of ordinal scale to represent the construct and the inability of the validation method to address the sample’ ability level and to examine the single latent construct. The current work attempts to address the two issues by examining the psychometric assessment of a teachers’ online social support survey. Particularly, it illustrates the application of modern psychometric theory, i.e. Rasch Model, to examine the psychometric characteristics of an Indonesian-translated instrument that explores the online social supports received by English as a foreign language (EFL) teacher in WhatsApp groups.
1. Introduction
Surveys with a rating scale have been regarded as a common method for collecting data about specific types of educational, social and behavioural constructs (DiStefano & Jiang, Citation2020; Van Zile-Tamsen, Citation2017). For example, in the context of higher education, Van Zile-Tamsen (Citation2017) has argued that many universities have developed local scales to explore their staffs’ and students’ satisfaction, teaching and learning effectiveness, educational climates and some others. A similar use of surveys is also reported in several studies in school contexts, such as in Bear, Gaskins, Blank and Chen (Bear et al., Citation2011), McEwan and Carnoy (Citation2000) and many others.
Particularly, surveys are frequently used to assess online social support that teachers and students have shared and received from social networking sites. To name a few, Chung, Yang and Chen (Chung & Chen, Citation2016, Citation2018; Chung et al., Citation2014; Tang et al., Citation2016) are among those who are consistent with using surveys in assessing the exchanges of teachers’ and students’ online social support in higher education settings. It is essential to highlight here that the term ’social support’ is operated as an interaction between people that reflects emotional concern, instrumental assistance, information and appraisal exchanges (House, Citation1981), and the “online social support” is exercised as the transmission of social support in online environments (Chung & Chen, Citation2018; Tang et al., Citation2016).
As regards the teachers’ online social support, Chung and Chen (Citation2018), for example, developed an online questionnaire to collect data for the assessment of the relationship between the social support exchanges among teachers in online teachers groups and teachers’ self-efficacy. The five-point Likert scale online questionnaire was written in Chinese and comprised of three scales, such as 15 items of self-efficacy scale, 13 items of providing online social support scale and 13 items of receiving online social support scales.
In a study evaluating the online social support received by Facebook users and the extent it affects stress coping, Chung et al. (Citation2014) developed 23 Facebook Social Support Scale (FSSC) items to assess particular support perceived by Facebook users. The scale included 10 items of information support, seven items of appraisal support and six items of emotional support. The alternative responses of FSSC also adopted the five-point Likert scale.
A construct of a particular scale used to explore a particular context of variables must possess sufficient psychometric characteristics such as reliability and validity assessments, facility index, discrimination index for diagnostic testing (Chan & Subramaniam, Citation2020). As in Chung and Chen (Citation2018), the construct validity of the three scales they had developed was assessed using confirmatory factor analysis with 554 teacher participants. The assessment result revealed the model fitness to the data, and the Cronbach's alpha for the constructs was reported higher than .80. A similar method was also done to evaluate Chung et al.’s (Citation2014) FSSC, showing the internal consistency for each scale in an acceptable range of .70—.95 (information scale α = 0.90, appraisal scale α = 0.86, and emotional scale α = 0.74).
Despite the satisfying result from the validation analysis using the conventional method, two critical issues emerge from the calculation. The first issue concerns the application of an ordinal scale to represent the construct (DiStefano & Jiang, Citation2020). DiStefano and Jiang (Citation2020) argue that, when validating a questionnaire, many researchers tended to sum item responses to obtain a total score and reflect the construct of interest. Such action is difficult to justify to the extent that the summed scores fail to provide sufficient consideration to items that “may vary due to the item’s placement relative to the construct” (DiStefano & Jiang, Citation2020, p. 32). More importantly, Wright (1992, cited in Kreijns et al., Citation2020) argues that the summed scores are considered not linear, and the interval of two consecutive total scores cannot be assumed equal. This inequality has issued insufficient intervals of the data.
The other issue deals with the inability of the validation method to address the sample’s ability level and to examine the single latent construct. Van Zile-Tamsen (Citation2017) suggests that the conventional method has limited the opportunity to assess the role of individual items to determine the effectiveness of the items regarding the target population and their contribution to the evaluation of the overall latent construct.
The current work attempts to address the critical issues of validation analysis of teachers’ online social support scale. Particularly, it illustrates the application of modern psychometric theory, i.e. Rasch Model, to examine the psychometric characteristics of an Indonesian-translated instrument that explores the online social supports received by the Indonesian EFL teachers in WhatsApp groups.
2. Literature review
The current study examines the psychometric characteristics of an Indonesian-translated instrument that explores the online social supports received by the Indonesian EFL teachers in WhatsApp groups. To this end, it is critical to comprehend the concept of social support and its application in teacher’s online communities.
Social support has been regarded as one coping structure of individual stressful experiences and symptoms (Zimet et al., Citation1988). House (Citation1981) perceived “social support” as an interaction between people that reflects emotional concern, instrumental assistance, information and appraisal exchanges. House’s perceived definition of social support has indicated the types of supports that people may provide or receive from their community, such as emotional support, informational, instrumental support and finally appraisal support. The primary aims of the support are mainly to promote better mental health by buffering the negative influence of stressful life events and reduce the particular experience of depression (Li et al., Citation2015; Zimet et al., Citation1988). What should be emphasized is that social support is unlikely unidirectional; instead, it concerns with the exchange of social support resources requiring at least two individuals that receive and give supportive acts or behaviour to each other (Li et al., Citation2015).
The term “online social support” in this paper is perceived as a type of social support transmitted in online environments (Chung & Chen, Citation2018; Tang et al., Citation2016). The wide use of the internet for online communication and collaboration has created new opportunities for teachers to help one another by sharing effective classroom instruction and classroom management strategies, giving other perspectives on particular topics and policies in an educational setting (Chung & Chen, Citation2018). In such interactions, teachers are likely enabled to express their ideas, thoughts, and arguments about particular issues, giving teachers the sense of emotional supports and reward from the members of certain professional communities (DeWert et al., Citation2003). According to DeWert et al. (Citation2003), the emotional supports and rewards may decrease teachers’ feeling of being isolated, boost their self-confidence and improve their ability to make better decisions about their professional practices.
Online social support is apparently different from the support interaction in a face-to-face (F2F) environment. The absence of direct physical contact available in the online classroom environment is believed to limit teachers’ opportunity to benefit from their peer supports as in F2F classroom interaction. However, findings from some earlier studies have recorded the benefits from online social support in promoting teachers’ self-efficacy for creative teaching (Chung & Chen, Citation2018), besides its association to psychological well-being and positive personal adjustment (Li et al., Citation2015). Chung and Chen (Citation2018) have argued that social persuasion in teachers’ online community has strengthened teachers’ belief and self-confidence in carrying out their instructional tasks and activities.
According to Chung and Chen (Citation2018), teachers’ professional groups that have proliferated on social media, such as Facebook, Twitter, WhatsApp, may be transformed into an online community of practice and be used to facilitate the exchange of social support. In his argument, teachers’ online group can be regarded as an online community of practice if several teachers build and share their expertise within a lively social interaction persistently (Wenger, Citation1998). Furthermore, an online community of practice should include teachers’ evaluation of their own or peers’ professional practices by considering various ideas, thoughts and perceptions (Ekici, Citation2018). Finally, Zhang and Watts (Citation2008) have argued that the online community of practice enables the participants to recall their discussion as the participation history is recorded and restored in certain particular elements of the technology application.
3. Method
3.1. Instrument
The study evaluated the psychometric characteristics of the Indonesian-translated version of the 23-item Facebook Social Support Scale (FSSC) adapted from Chung et al. (Citation2014) instrument. In classroom teaching, the FSSC has been used to examine the exchange of teachers’ online social support (Chung & Chen, Citation2016, Citation2018). Originally, the instruments were used to examine the exchange of online social support on the selected Facebook group (Chung & Chen, Citation2016, Citation2018; Chung et al., Citation2014). In the current study, the wordings were adapted to the online social support that teachers received from the selected WhatsApp group. For such a purpose and further discussion, we operated the term “WhatsApp social support scale” or WSSC to refer to the FSSC questionnaire to acknowledge WSSC adaptation from the original questionnaire and the emphasis of WhatsApp application use instead of Facebook.
WSSC scale included 23 items classified into three subscales (Chung & Chen, Citation2016): information, appraisal, and emotional (see ). All FSSC scale items were developed using a five point-Likert rating scale, with “strongly agree” (scored 5), “agree” (scored 4), “fair” (scored 3), “disagree” (scored 2), and “strongly disagree” (scored 1). In its early development, the internal consistency for each subscale remains high for information (α = 0.90) and appraisal (α = 0.86), and good for emotional (α = 0.74).
Table 1. WSSC subscales
3.2. Translation process
In the published paper, WSSC was originally written in English and, for the current study, was translated into the native Bahasa Indonesia. The translation was conducted by the first author, who was fluent in both English and Bahasa Indonesia. Then, the second author, who was fluent in English and Bahasa Indonesia, validated the translated questionnaire and proofread it. As suggested in the literature, the wordings of the Indonesian translated questionnaire were evaluated and refined to improve the questionnaire readability (Mulyono et al., Citation2020).
3.3. Sample
The study adopted a non-probability sampling method to target the participants. In this method, a survey of WSSC was distributed to groups of Indonesian English as a foreign language (EFL) teachers using Google form. The EFL teachers were informed that the survey was on a voluntary basis in which they were freed to opt out from participating in the study. A total of 214 Indonesian EFL primary and secondary school teachers agreed to participate and then completed the survey. Thus, consent was obtained prior to the data collection. Details of the participant demography are presented in .
Table 2. Demography of the sample population
4. Rasch model analysis
A Rasch-based analysis was adopted to provide valid evidence for the WSSC. Rasch analysis has been widely used for instrument validations and maintains the instrument quality (Mulyono et al., Citation2020; Yu, Citation2020). Rasch model analysis is viewed as an analysis of a latent trait model developed to reflect “the probability that a person provides a certain response to an item as a function of the person and item characteristics” (Colledani et al., Citation2020, p. 2). Such an analysis is developed under the item-response theory (IRT), aiming to explain the interaction between the survey respondents and the items using probabilistic models (Ackerman, Citation1994). According to Wright, as cited in Yu (Citation2020), Rasch analysis enables the instrument validator to perform objective and precise measurement for the analysis by figuring out invariant measurement characteristics across a diverse situation. Moreover, Rasch analysis has been the best alternative in addressing the weighing and interval level issues in assessing an ordinal scale from a construct of interest (DiStefano & Jiang, Citation2020).
4.1. Data tabulation and screening process
The collected data from Google form was downloaded from the site server and was converted to an Excel file. The data were coded, and any information related to the participant identities was removed to keep the participant anonymous. The raw data then was converted into logits (or log-odds unit) score. The term odds refer to “the probability of non-desired outcomes, relative to the probability of the desired outcome”. The logit is conceptualized as “the natural logarithmic scale of the odds” (Yu, Citation2020, p. 56). The following equations represent the relationship between probabilities (P) and odds:
Odds = P/(1—P) (1)
P = Odds/(1 + Odds) (2)
The raw-score to logits conversion was done because the data from ordinal rating are considered linear and thus cannot be used for any parametrical statistical analyses (Boone et al., Citation2014). According to Colledani et al. (Citation2020), the conversion has enabled the Rasch model to obtain measurement units at the same interval size so that the length between any two measures is meaningful (Colledani et al., Citation2020, p. 2). In the current study, such a conversion of raw data into logit value was done using a WINSTEP application.
After the conversion, the logit data values underwent two rounds of analysis. The first analysis is concerned with the data screening process. In this process, the data from misbehaving participants (i.e. participants who did not seriously complete the questionnaire) was considered misfit responses and removed (Goh et al., Citation2010; Linacre, Citation2010). In our analysis, the respondent logit data with z-standardised (Zstd) infit and outfit mean square less than −2 or above +2 were observed to misfit. Of 214 samples, 114 were shown to misfit with the Rasch model and thus were excluded for the second round analysis (Huang et al., Citation2020; Linacre, Citation2002b, Citation2010; Mulyono et al., Citation2020). It is worth noting that the amount of 100 samples as used in the current study still met the minimum requirements for sample size in Rasch analysis, which is 50 samples (Linacre, Citation1994).
In the second round, Rasch statistical analysis using WINSTEP version 4.5.1 was performed mainly to address the Rasch modelling assumptions such as unidimensionality, a monotonic scale and the Rasch fit model (DiStefano & Jiang, Citation2020). The following subsection will further describe the analysis process and the findings.
4.2. Assessment of unidimensionality
The first assumption of Rasch analysis concerns with unidimensionality aspect of a measure. A measure is unidimensional for its ability to measure a single construct or concept (Yu, Citation2020). The assessment of the WSSC unidimensionality aspect was done by evaluating the Principle Component Analysis (PCA) of residuals for the general scale of WSSC and each sub-scale (i.e. information, appraisal and emotional subscale). The evaluation of PCA aimed to identify a particular association pattern among the WSSC constructs and determine the number of components that explained the maximum variance in the data (Colledani et al., Citation2020). details the result of the unidimensionality assessment of WSSC.
Table 3. Unidimensionality assessment of WSSC
As shown in the above , Rasch Principal Component Analysis (PCA) outcome for global scale and all subscale has exceeded the threshold value of 20% of the variance data (Chan & Subramaniam, Citation2020; Sumintono & Widhiarso, Citation2014). As a result, the global scale raw variance was reported at 48.7%, and the information, appraisal and emotional subscales were observed at 62.1%, 59.2%, and 50.5% consecutively. In addition, the PCA first eigenvalue of the global scale and all subscales was reported lower than the unidimensionality threshold of 3 (i.e. EMS = 2.0; INS = 1.9; and APS = 1.8) (Galli et al., Citation2008). These findings indicate that WSSC fits the Rasch model, providing statistical evidence of a unidimensionality measurement of the scales for both global scale and the subscales. In other words, the WSSC primarily measures the social support received by Indonesian EFL teachers in WhatsApp groups, and the WSSC subscales mainly measured the information, appraisal and emotional supports.
4.3. Assessment of the item and person separation reliability
According to Chan and Subramaniam (Citation2020), the assessment of an item and person separation reliability should be performed in conjunction with the assessment of the unidimensionality aspect of the Rasch model. Such assessment helps to indicate the potential reproducibility of item and person locations on the latent traits continuum (Chan & Subramaniam, Citation2020; Colledani et al., Citation2020). Adam and Khoo, as cited in Ben (Citation2020, p. 85), defines separation reliability index as “an indication of the proportion of the observed variance that is considered true”. In the classical test theory, the person separation reliability is viewed as Cronbach’s alpha or KR-20, while the item separation reliability cannot be found in the classical test theory (Colledani et al., Citation2020). details the item and person separation reliability.
Table 4. The item and person separation reliability
As in , the analysis of Rasch reliability resulted that the WSSC scale and subscales possessed a very high level of internal consistency (α ≥ .90) (Cohen et al., Citation2018). In other words, the person-level reliability of the WSSC scale and subscale maintains a sufficient level of generalisability of the measurement to new samples (Van Zile-Tamsen, Citation2017).
Besides, item separation was observed to have a high level of reliability for the global scale and information and emotional subscales, while the appraisal subscale was reported as “acceptable”. The WSSC global scale and subscales were reported to have a high level of person separation reliability.
Regarding the separation indexes, the item and person separation index values were shown to be sufficient (see Kreijns et al. Kreijns et al., Citation2020). The item separation index has shown the obtained value ranging from 1.43 to 3.30 and the strata from 2.24 to 4.73, suggesting that the item difficulty can be classified into at least two strata. The person separation values from 2.57 to 3.67 have indicated that at least three groups were identified from the logit data. This finding also suggests that the WSSC scale and subscales could distinguish between the high and lower performance from the responding person sample (Linacre, Citation2018).
4.4. Assessment of the likert rating scale and the item properties
The second assumption of the Rasch model concerns with the extent to which the WSSC scale can suggest the monotonical increase of its interval scale (Bond & Fox, Citation2015). To evaluate such an assumption, we assessed the Rasch ordering functioning of the WSSC scale. As discussed earlier, the WSSC was developed using five rating units of the Likert scale for the questionnaire (5 = “Strongly agree” to 1 = “Strongly disagree”).
The result of ordering functioning analysis in showed that the adjacent threshold distance of the rating scale ranged between 0.94 and 4.69 logits. The outfit MNSQ were satisfactory, showing that each rating unit value was less than the threshold of 2. Such value not only had fitted the Rasch model statistically well but also suggested that the scale category did not introduce noise into the analysis (DiStefano & Jiang, Citation2020).
Table 5. The ordering functioning of the rating scale
As in , each of the rating scale step categories was observed to have more than a minimum of 10 people. Also, the average calibration of the rating scale step category was arranged in order and revealed a monotonical increase from −1.28 logits (category one or “strongly disagree”) to 4.49 logits (category five or ‘strongly disagree). With regard to the distance threshold, the distance between each category was maintained within the range of 1 logit and less than five logits (Bond & Fox, Citation2015). The distance between the step category 3–4 and 4–5 was above the threshold, while the distance of step category 2–3 remained relatively close to 1 logit. A similar increase was also reported at the step threshold, indicating that no disorder had been identified in the Rasch model.
4.5. Wright map assessment
Wright map was developed to figure out the distribution of person and item location on the same scale (Colledani et al., Citation2020). A Wright map can also be used as a graphical representation of the overall item and person levels. The map is divided into a Y-axis: The left side-person measures show teacher participants’ location by their logit values. The codes “#” and “.” in the person measure indicate two persons and one person, respectively. The other right side—item measure presents the relationships between items and their construct. Particularly, the item measure provides information on item difficulty levels.
presents a Wright map that plots 23-items of WSSC corresponding to the person ability. In the current study, the level of item difficulty ranged between −1.16 and .92. For example, as shown in , the item Q15, “On WhatsApp groups, I have someone to consult with things about daily life affairs”, had the value of .92 logits and was considered the most difficult item to agree. Two other items with high levels of difficulty included Q17 “When I have bad moods on Facebook, someone will comfort and encourage me with a message” with .86 logits and Q15 “On WhatsApp, there is someone I can ask for information about school clubs” with .76 logits. In contrast, item Q2, “On WhatsApp group, I have friends to share happiness with me”, was regarded as the most accessible item to agree with −1.16 logits, followed by Q7, “My friends in WhatsApp groups discuss schoolwork with me”, with −0.80 logits.
4.6. Potential item bias
A good questionnaire should address the issue of scale and item scale appropriateness with particular groups of participants. To this end, differential item functioning (DIF) was evaluated using Rasch–Welch tests. DIF occurs when the DIF contrast value is more significant than 0.5 logits and is observed to be significant (Rasch–Welch p < .05) (Chan & Subramaniam, Citation2020; Linacre, Citation2018; Ling Lee et al., Citation2020; Mulyono et al., Citation2020). In the current study, DIF was examined in reference to the participants’ demographic aspects, including gender, age, background of education, teaching experiences, and level of teaching. The evaluation of DIF enables us to identify the item bias in the questionnaire items and provides statistical evidence of consequential validity (see Chan & Subramaniam, Citation2020). evaluates DIF on each item influenced by teachers’ gender, age, background of education, teaching experience, and teachers’ level of teaching.
Table 6. DIF evaluation on the scale item
As shown in the above Table, item Q2, “On WhatsApp group, I have friends to share happiness with me”, was considered bias in reference to all demographic aspects. For example, female participants were shown to respond to item Q2 easier compared to the males. Item Q6 “On WhatsApp group, someone pokes and invites me to play games, making me feel welcomed” was shown as DIF for the aspect of teachers’ age and teaching experience. The other items, such as Q10, Q17, Q18, and Q22, were biased for the demography of age, and Q3 was biased in reference to teachers’ level of teaching.
5. Discussions and conclusions
The constructs of WSSC measuring the online social supports that EFL teachers received from certain WhatsApp groups were assessed using the Rasch model. To the best of our knowledge, the Indonesian version of FSSC and its adoption to explore the online social supports that EFL teachers received from particular WhatsApp groups have not been reported elsewhere, suggesting a valuable contribution of the current study to the literature.
The Rasch evaluation of the unidimensionality aspect of the scale has shown that the PCA of the residuals was higher than 20% of the variance and with the PCA first eigenvalue of the global scale and all subscales was lower than the unidimensionality threshold of 3 (Chan & Subramaniam, Citation2020; Galli et al., Citation2008; Ling Lee et al., Citation2020). These findings provided statistical evidence that the WSSC essentially measures a single latent variable: the online social support received by EFL teachers in the WhatsApp group. Moreover, the internal consistency of the WSSC scale and subscales was reported very high. A similar report was also found in the item and person separation reliability. These findings had provided statistical evidence that the WSSC scale and subscale had the reproducibility of the obtained measures of the scale and the subscale. Specifically, the WSSC items were shown to have the capability to be reproduced by another group of samples (Van Zile-Tamsen, Citation2017).
Furthermore, the Rasch model analysis has identified two strata of item difficulty and three groups of participants. Concerning the findings from the person separation index, the finding suggests that the WSSC scale and subscales had a capability to distinguish between the high and lower performance from the responding person sample (Linacre, Citation2018). Regarding the rating scale properties, the step category of the scale was maintained in order and increased monotonically. No disorder had been identified in the rating scale. However, the step category 2–3 with .94 logit distance should be interpreted with a notice, and redefining the step categories is urged to suggest broader substantive meanings of the category (Linacre, Citation2002a). In other words, changing the range from a 5-point Likert scale into 4 points can be an alternative to address the issue regarding step category 2–3. When the logit data were classified in reference to the demography aspects (i.e. gender, age, the background of education, teaching experiences, and level of teaching), DIF was noted in many of the items; particularly Q2 items identified in all aspects and Q6 for the age and teaching experience aspect.
To sum up, the WSSC provides sufficient psychometric characteristics. The Rasch analysis of the WSSC scale has shown that the scale fit the Rasch model with high reliability levels for both person and item levels. The result of response category analysis has indicated redefining the category 2–3, and an evaluation of WSSC items with DIF notes is suggested in the future update of the scale. Specifically, modifying the scale type from a 5-point Likert scale into 4 points scale can eliminate the potential misfit data and enable the scale to function at its maximum capability (DiStefano & Jiang, Citation2020). As so, the reliability of data can be increased and accordingly, precise measurement can be obtained (Linacre, Citation2002a).
Acknowledgements
We thank Dr Bambang Sumintono and Regitha Saskia for their feedback and discussion on the statistical analysis results. We are also thankful to the anonymous reviewers for their valuable feedback on our paper.
Disclosure statement
The authors reported no potential competing interest.
Additional information
Funding
Notes on contributors
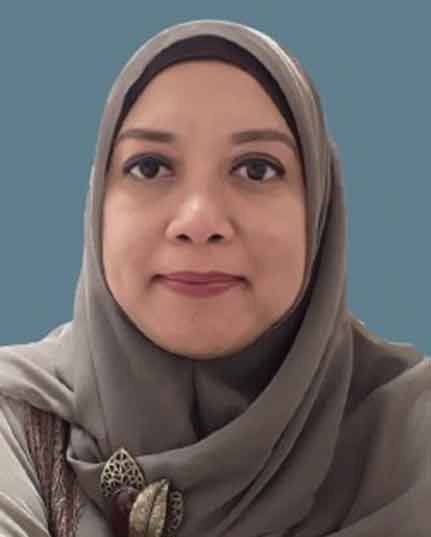
Sri Kusuma Ningsih
Sri Kusuma Ningsih received his B.A. degree in French language education from Universitas Negeri Jakarta in 2001 and an M.A. degree from UHAMKA in 2011. At present, she is a doctoral student at Graduate School, Universitas Pendidikan Indonesia, Bandung, Indonesia.
Herri Mulyono
Herri Mulyono received M.A. in English Education from UHAMKA in 2010. He received the PhD degree in TESOL from University of York, United Kingdom, in 2018.
Rafikah Ar Rahmah
Rafikah Ar Rahmah is currently a final year student at the department of English Education, Faculty of Teacher Training and Pedagogy, UHAMKA. She is also a research assistant at L8 Education Research Group at the University.
Nurul Aprilia Fitriani
Nurul Aprilia Fitriani is a final year student at the department of English Education, Faculty of Teacher Training and Pedagogy, UHAMKA. She has been joining L8 Education Research Group as a research assistant since August 2020.
References
- Ackerman, T. A. (1994). Using multidimensional item response theory to understand what items and tests are measuring. Applied Measurement in Education, 7(4), 255–13. https://doi.org/https://doi.org/10.1207/s15324818ame0704_1
- Bear, G. G., Gaskins, C., Blank, J., & Chen, F. F. (2011). Delaware school climate survey—student: Its factor structure, concurrent validity, and reliability. Journal of School Psychology, 49(2), 157–174. https://doi.org/https://doi.org/10.1016/j.jsp.2011.01.001
- Ben, F. (2020). Re-examining the utility of the Individualised Classroom Environment Questionnaire (ICEQ) using the Rasch model. In M. S. Khine (Ed.), Rasch measurement: Applications in quantitative educational research (pp. 77–97). Springer Singapore. https://doi.org/https://doi.org/10.1007/978-981-15-1800-3_5
- Bond, T., & Fox, C. M. (2015). Applying the Rasch model: Fundamental measurement in the human sciences. Routledge.
- Boone, W. J., Staver, J. R., & Yale, M. S. (2014). Rasch analysis in the human sciences. Springer.
- Chan, M., & Subramaniam, R. (2020). Validation of a science concept inventory by Rasch analysis. In M. S. Khine (Ed.), Rasch measurement: Applications in quantitative educational research (pp. 159–178). Springer Singapore. https://doi.org/https://doi.org/10.1007/978-981-15-1800-3_9
- Chung, T.-Y., & Chen, Y.-L. (2016). Characteristics of membership of online teacher community and correlates of providing and receiving online social support: The case of a Facebook teacher group. Research of Educational Communications and Technology, 113, 63–88. https://doi.org/https://doi.org/10.6137/RECT.2016.113.04
- Chung, T.-Y., & Chen, Y.-L. (2018). Exchanging social support on online teacher groups: Relation to teacher self-efficacy. Telematics and Informatics, 35(5), 1542–1552. https://doi.org/https://doi.org/10.1016/j.tele.2018.03.022
- Chung, T.-Y., Yang, C.-Y., & Chen, M.-C. (2014). Online social support perceived by Facebook users and its effects on stress coping. European Journal of Economics Management, 1, 196–216. https://www.researchgate.net/publication/278671988_Online_social_support_perceived_by_Facebook_users_and_its_effects_on_stress_coping
- Cohen, L., Manion, L., Morrison, K., & Bell, R. (2018). Research methods in education (8th ed.). Routledge.
- Colledani, D., Anselmi, P., & Robusto, E. (2020). Rasch models in the analysis of repgrid data. Journal of Constructivist Psychology, 33, 1–21. https://doi.org/https://doi.org/10.1080/10720537.2020.1852461
- DeWert, M. H., Babinski, L. M., & Jones, B. D. (2003). Safe passages: Providing online support to beginning teachers. Journal of Teacher Education, 54(4), 311–320. https://doi.org/https://doi.org/10.1177/0022487103255008
- DiStefano, C., & Jiang, N. (2020). Applying the Rasch rating scale method to questionnaire data. In M. S. Khine (Ed.), Rasch measurement: Applications in quantitative educational research (pp. 31–46). Springer Singapore. https://doi.org/https://doi.org/10.1007/978-981-15-1800-3_3
- Ekici, D. I. (2018). Development of pre-service teachers’ teaching self-efficacy beliefs through an online community of practice. Asia Pacific Education Review, 19(1), 27–40. https://doi.org/https://doi.org/10.1007/s12564-017-9511-8
- Galli, S., Chiesi, F., & Primi, C. (2008). The construction of a scale to measure mathematical ability in psychology students: An application of the Rasch Model. TPM (Testing Psicometria Metodologia), 15(1), 1–16. http://www.tpmap.org/wp-content/uploads/2014/11/15.1.1.pdf
- Goh, J. W. P., Quek, C. J., & Lee, O. K. (2010). An Investigation of students’ perceptions of learning benefits of weblogs in an east Asian context: A Rasch analysis. Journal of Educational Technology & Society, 13(2), 90–101. http://www.jstor.org/stable/jeductechsoci.13.2.90
- House, J. S. (1981). Work stress and social support. Addison-Wesley Publishing Company.
- Huang, F., Huang, L., & Oon, P.-T. (2020). Constructs evaluation of student attitudes toward science—A Rasch analysis. In M. S. Khine (Ed.), Rasch measurement: Applications in quantitative educational research (pp. 139–157). Springer Singapore. https://doi.org/https://doi.org/10.1007/978-981-15-1800-3_8
- Kreijns, K., Bijker, M., & Weidlich, J. (2020). A Rasch analysis approach to the development and validation of a social presence measure. In M. S. Khine (Ed.), Rasch Measurement: Applications in quantitative educational research (pp. 197–221). Springer Singapore. https://doi.org/https://doi.org/10.1007/978-981-15-1800-3_11
- Li, X., Chen, W., & Popiel, P. (2015). What happens on Facebook stays on Facebook? The implications of Facebook interaction for perceived, receiving, and giving social support. Computers in Human Behavior, 51(Part A), 106–113. https://doi.org/https://doi.org/10.1016/j.chb.2015.04.066
- Linacre, J. M. (1994). Sample size and item calibration stability. Rasch Measurement Transactions, 7(4), 328. https://www.rasch.org/rmt/rmt74m.htm
- Linacre, J. M. (2002a). Optimizing rating scale category effectiveness. Journal of Applied Measurement, 3(1), 85–106. https://citeseerx.ist.psu.edu/viewdoc/download?doi=10.1.1.424.2811&rep=rep1&type=pdf
- Linacre, J. M. (2002b). What do infit and outfit, mean square and standardized mean? Rasch Measurement Transactions, 16(2), 878. https://www.rasch.org/rmt/rmt162f.htm
- Linacre, J. M. (2010). When to stop removing items and persons in Rasch misfit analysis? Rasch Measurement Transactions, 23(4), 1241. https://www.rasch.org/rmt/rmt234g.htm
- Linacre, J. M. (2018). A user’s guide to Winsteps Ministep Rasch-model computer programs (version 4.3.1). Institute for Objective Measurement, Inc Available at https://www.winsteps.com/a/Winsteps-Manual.pdf (accessed March 19, 2019).
- Ling Lee, W., Chinna, K., & Sumintono, B. (2020). Psychometrics assessment of HeartQoL questionnaire: A Rasch analysis. European Journal of Preventive Cardiology, 204748732090232. https://doi.org/https://doi.org/10.1177/2047487320902322
- McEwan, P. J., & Carnoy, M. (2000). The effectiveness and efficiency of private schools in Chile’s voucher system. Educational Evaluation and Policy Analysis, 22(3), 213–239. https://doi.org/https://doi.org/10.3102/01623737022003213
- Mulyono, H., Saskia, R., Arrummaiza, V. S., Suryoputro, G., & Monacis, L. (2020). Psychometric assessment of an instrument evaluating the effects of affective variables on students’ WTC in face-to-face and digital environment. Cogent Psychology, 7(1), 1823617. https://doi.org/https://doi.org/10.1080/23311908.2020.1823617
- Sumintono, B., & Widhiarso, W. (2014). Aplikasi model rasch untuk penelitian ilmu-ilmu sosial B. Trim (ed.). Trim Komunikata. Second
- Tang, J.-H., Chen, M.-C., Yang, C.-Y., Chung, T.-Y., & Lee, Y.-A. (2016). Personality traits, interpersonal relationships, online social support, and Facebook addiction. Telematics and Informatics, 33(1), 102–108. https://doi.org/https://doi.org/10.1016/j.tele.2015.06.003
- Van Zile-Tamsen, C. (2017). Using Rasch analysis to inform rating scale development. Research in Higher Education, 58(8), 922–933. https://doi.org/https://doi.org/10.1007/s11162-017-9448-0
- Wenger, E. (1998). Communities of practice: Learning, meaning, and identity. Cambridge university press.
- Yu, C. H. (2020). Objective measurement: How Rasch modeling can simplify and enhance your assessment. In M. Khine (Ed.), Rasch measurement (pp. 47–73). Springer. https://doi.org/https://doi.org/10.1007/978-981-15-1800-3_4
- Zhang, W., & Watts, S. (2008). Online communities as communities of practice: A case study. Journal of Knowledge Management, 12(4), 55–71. https://doi.org/https://doi.org/10.1108/13673270810884255
- Zimet, G. D., Dahlem, N. W., Zimet, S. G., & Farley, G. K. (1988). The multidimensional scale of perceived social support. Journal of Personality Assessment, 52(1), 30–41. https://doi.org/https://doi.org/10.1207/s15327752jpa5201_2