Abstract
This study investigates the use of an assistive humanoid robot for teaching to and learning among young children in an informal learning environment. The robot is called NAO and built by SoftBank Robotics. NAO was used to teach a mathematic concept of measurement to young children. They engaged with the robot through the teaching and learning of presentation slides, worksheets, and playing cards. Their interactions with the robot formed the evidence of their learning. The children also played with the robot in an open and friendly environment, which was video recorded. The efficacy and impacts were measured and analyzed through social signals and verbal responses. Results show that the robot can build a positive and friendly relationship with children while achieving learning outcomes. This study demonstrates the possibilities of introducing NAO into the curriculum and calls for further research toward implementing humanoid robots in classroom settings.
1. Introduction
The rapid development of robotics and artificial intelligence has recently permeated the education sector and related research. Many papers review and explore the implementation of humanoid robots in various subjects, including second language learning, science, technology, engineering, mathematics, and more (Chalmers et al., Citation2022; Hashimoto et al., Citation2013; Papadopoulos et al., Citation2020). A requirement analysis of educational robots reveals that preschool and primary schools are most likely to implement educational robotics in the foreseeable future (Cheng et al., Citation2018).
The versatile and high-performance humanoid robots such as Asimo, Atlas, NAO, Papero, Pepper, Saya, Sophia, and Tiro (Bautista & Wane, Citation2018; Hashimoto et al., Citation2011; Mahdi et al.,Citation2021; Oh & Kim, Citation2010; Okita et al., Citation2009; Osada et al., Citation2006; Robaczewski et al., Citation2021; Tanaka et al., Citation2015) enable teachers to practice new pedagogical methods in classrooms. Among the humanoid robots available on the market, NAO’s friendly appearance and human-like behaviors enable an empathetic link with students, teachers and researchers. Several studies demonstrate the positive effects of using NAO in facilitating learning and pedagogical design (Yousif & Yousif, Citation2020; Crompton et al., Citation2018; Fridin, Citation2014; Mubin et al., Citation2019). NAO can introduce attractive teaching topics and apply project-based learning approaches, thus allowing students to acquire multi-disciplinary skills and simultaneously develop social and emotional skills. The educational development of advanced humanoid robots is relatively new, assistive humanoid robots are not among the popular choices in educational technology for Hong Kong mainstream schools.
In this research, we investigate children’s behavior toward the humanoid robot NAO and its potential as a learning companion to facilitate teaching and learning. In particular, we observe children’s interactions with NAO and provide analysis of the social signals and verbal responses captured from video recordings. We describe the way NAO plays the role of a learning companion, the children’s attitude toward the robot teacher, and the atmosphere of the class.
The paper is organized as follows. We review the literature on humanoid robots for education in Section 2. We identify the pedagogical and technological consideration of our humanoid robotic system in Section 3. We propose the design and method of the experiment in Sections 4 and 5, respectively. We analyze the video recordings and discuss the results in Sections 6 and 7. We conclude the study in Section 8.
2. Literature review
2.1. Application of humanoid robots in education
From robotic toys to humanoid robots, educational robotics has been a subject of research since the 1970s (Belpaeme & Tanaka, Citation2021). The use of humanoid robots for education can be applied onto different age groups, including young children, juveniles, and university students. Researchers have critically reviewed the potential use of educational robotics recently. Papadopoulos et al. (Citation2020) examined twenty-one pre-tertiary studies in the first quarter of 2018 and found that socially assistive robots is generally promising but in the field of science and mathematics is significantly under-represented. This research is filling the gap. Specifically, Robaczewski et al. (Citation2021) reviewed fifty-one papers focusing on NAO used in various contexts. The selected studies were identified according to the six defined categories: social interactions, affectivity, intervention, assisted teaching, mild cognitive impairment/dementia, and autism/intellectual disability. NAO showed both strengths and weaknesses in these categories and the majority of the findings were positive. For example, the researchers found that NAO was an efficient teacher. Its greatest advantage is its use of visual, auditive and kinesthetic modalities in learning. This research could illustrate this advantage.
Several studies focus on the use of educational robotics for young children. Ioannou et al. (Citation2015) did an exploratory study for determining preschoolers’ interest and behavior around a humanoid robot. They proved that young children can interact socially with humanoid robots, particularly when they dance, need help, or show caring behaviors such as kisses and hugs. Vogt et al. (Citation2019) conducted a large-scale study that involved 194 children learning English vocabulary as a foreign language using a NAO robot. Although the robot show no added value compared with using only a tablet, they claimed that the tutoring interaction with the robot is more effective and engaging. A social robot, Tega, was used as a learning companion to engage children in a collaborative game. Researchers reported that children positively respond to the robot’s verbal and non-verbal cues and that they return the same to the robot, denoting their emotional and relational engagement to Tega. The results also show a significant effect on vocabulary acquisition (Chen et al., Citation2020).
Some researchers proposed using educational robotics for secondary school pupils. A robot education agent, DARwIn-OP (Darwin), was integrated into a math-learning scenario to investigate children’s engagement in an algebra test (Brown & Howard, Citation2013). The results show that students enjoy Darwin’s presence and that Darwin effectively decreases idle time and draws the students’ attention. Alemi et al. (Citation2014) examined the effect of robot-assisted language learning with a NAO humanoid robot. They reported that combining human and robot instruction enhances the capabilities of students over conventional English teaching methods. The group that learned with a humanoid robot surpasses the control group significantly in vocabulary achievement. Al Hakim et al. (Citation2022) showcased the use of robots in a learning environment by integrating it with pedagogical approaches. The results show that students who learnt with the robot in an interactive environment outperformed others in learning engagement. Chalmers et al. (Citation2022) demonstrated the use of humanoid Robot NAO in nine secondary schools for year 7–10 students in different subject disciplines such as language, mathematics, and coding. Students in different years demonstrate engagement and develop perseverance throughout the lessons with the robot.
Some studies reported the use of the NAO robot in teaching at the tertiary level. Xu et al. (Citation2014) utilized a NAO robot to act as a lecturer to deliver an introductory course on robotics at a university. It was only a field trial, but most students showed a positive attitude and were interested in the robot’s performance. Lei and Rau (Citation2021) used a NAO robot as a teleoperated dance tutor to teach learners five dance movements and provide performance feedback. The results align with those mentioned above; the robot tutor improves the learners’ motivation, enthusiasm, encouragement, confidence, and happiness. Velentza et al. (Citation2021) adopted NAO as a robot professor to teach first-year students engineering concepts. The results demonstrate that all groups of students who encountered the robot have statistically notably higher enjoyment scores than the group taught by a human tutor. In general, the application of supportive humanoid robots increases the engagement of students and efficacy of the learning process. However, the effect and familiarity with the robot should also be considered when applying educational robots in classroom settings.
2.2. Roles of humanoid robots in teaching and learning
Owing to the advancement in robotics over the past few years, humanoid robots are being used as an engaging tool in education. Mubin et al. (Citation2013) described educational robots as a subset of educational technology used to assist students’ learning and improve their academic performance. A review of previous literature outlines several roles of humanoid robots in facilitating teaching and learning.
According to Belpaeme and Tanaka (Citation2021), social robots are designed and programmed to play the role of tutor, teacher (or assistant), and learning companion. Similarly, this has also been addressed in Mubin et al. (Citation2013) that a robot can take on the role of an instructor and involve at various levels in the learning task. It can also take a passive role as a teaching aid or participate actively as a co-learner, peer or companion.
Several studies explore how humanoid robots play a role as a tutor. It is said to be the most promising and pragmatic role for educational robots (Belpaeme & Tanaka, Citation2021). As a tutor, the robot supports the learning of a single learner or a small group of students. In the literature, Kanda et al. (Citation2004) used Robovie robots as English peer tutors for Japanese students. The robots successfully encourage some students to improve their English. Similarly, Lei and Rau (Citation2021) and Vogt et al. (Citation2019) adopted NAO robots as private tutors.
Whether humanoid robots can take the role of a teaching assistant has been closely investigated. Some examples of these robots are IROBI (Han et al., Citation2008), Mero and Engkey (Lee et al., Citation2010), and a telepresence robot (Kwon et al., Citation2010). Crompton et al. (Citation2018) implemented NAO robots in three preschool classrooms and examined the human–robot interaction among NAO, teachers, and children. Mubin et al. (Citation2019) employed an autonomous NAO robot to help revise mathematics in a local primary school in Abu Dhabi. The studies above provide positive feedback and demonstrate the potential of using humanoid robots as teaching assistants. Moreover, social robots are welcomed by teachers (Crompton et al., Citation2018; Kory Westlund et al., Citation2016).
Humanoid robots can also accompany humans as friends or learning companions. In past research, NAO robots were used as companions to interact with hospitalized children, acting as friends and mentors to keep them comfortable and help them know more about their health condition (Belpaeme et al., Citation2013). The results demonstrate that humanoid robots receive more attention than virtual characters on screen, which opens an opportunity for further research on using robots in education and social interaction. In addition, the literature suggests that young children are willing to engage with social robots as peer-like companions (Chen et al., Citation2020; Shiomi et al., Citation2006). Although these studies highlight the positive feelings toward the use of humanoid robots for educational purposes, longer term and repeated times of experiments are required to explore children’s developmental benefits and the robots’ ability to co-teach with school teachers.
3. Pedagogical and technological consideration of the humanoid robotic system
The effective inclusion of educational technology in classroom can serve as a catalyst to promote teaching and learning. Employing robotics in education is an evolution from traditional teaching methods that can enhance the learning experience and interactivity. Teachers need to integrate learning with digital technologies, present educational materials effectively, and express creativity in teaching. Educational technology can also provide a new form of computer-based assessment. In this section, we briefly discuss the pedagogical and technological considerations for our robotic system. The specific setup and implementation of the learning package using the humanoid robot are detailed in the next section.
3.1. E-learning resources for primary mathematics education curriculum in Hong Kong
Government policies and support are contributors to accelerating the use of educational technology in schools. We researched the primary mathematics education curriculum in Hong Kong to explore its strengths and weaknesses as well as the use of technology in the classroom. According to the Learning and Teaching Resources for STEM Education (KS1—Primary 1 to 3; Citation2023), there are only five learning and teaching resource packages for Primary 1–3 mathematics education. Only one of these packages incorporates educational technology tools into the lesson (i.e., 3D design software and 3D printing technology). The curriculum requires an update to foster the use of new technology elements in education.
According to the Report on Promotion of STEM Education published by the Education Bureau (Citation2016), the 2015 and 2016 Policy Addresses have shown support for the curriculum renewal of mathematics KLAs, enriching learning activities and enhancing the training of teachers for strengthening STEM education (p. 7). The government is also aware that schools appreciate students participating in STEM-related activities within and outside the school. At the same time, tertiary institutions in the city are enthusiastic about providing support for the promotion of STEM-related learning activities. To accomplish such goals in supporting STEM learning activities, we developed and tested an innovative learning package for the topic of measurements in the primary curriculum.
3.2. Pedagogical consideration
Lave and Wenger (Citation1991) first presented the concept of situated learning. It explains the learning process by actively participating in a community of practice. Hanks (Citation1991) also asserted that “learning is a process that takes place in a participation framework, not in an individual mind” (p. 53). Knowledge transfer occurs when one participates in authentic activities and interacts with others. The role of the humanoid robot in our study was to demonstrate measurement skills and provide students with enough scaffolding to solve the problems in the activities.
Our design was to develop a lively robotic learning companion with a new tool powered by artificial intelligence behind. The role of a teacher would be less direct and was changed from mentor to facilitator. This aligns with the constructivist approach. Oliver and Herrington (Citation2000) acknowledged that the principles of situated learning in a classroom-based setting can also be applied to a cloud-based learning system, so they were used as guiding principles to develop the learning package. Table presents the nine learning elements proposed by Oliver and Herrington (Citation2000) and the corresponding features of our learning package using the humanoid robot.
Table 1. Nine learning elements of situated learning and the corresponding features
3.3. Consideration on child–robot interaction
Baxter et al. (Citation2011) pointed out that a system’s dialogue and output strategies directly affect interaction success and user satisfaction. Coping with a wide range of unconstrained verbal input from children and giving nearly real-time responses were challenging for our system.
We chose the Google Cloud Speech-to-Text API and Dialogflow (formerly known as API.AI) as our AI support solutions among the popular cloud platforms available in the market. Google Cloud Speech-to-Text API is an advanced Speech-to-Text API that supports Cantonese for free (with conditions). Dialogflow is an advanced development suite for creating conversational AI applications; it supports Cantonese and features natural language understanding and machine learning. By adding training phrases to each intent, the agent recognized similar expressions and picked them up very well.
Besides Google Cloud, other powerful platforms provided by IBM and Microsoft are available. However, the Speech-to-Text API provided by IBM only supports Mandarin Chinese, and Microsoft Azure has minimal resources on its compatibility with NAO robots. Therefore, we selected Google Speech-to-Text API for the system.
Considering all critical technological decisions on building a smooth child–robot interaction, we implemented our robot learning companion with the support of the Google cloud services. As all the services were from the same provider, we could manage them in the dashboard at a glance.
3.4. Consideration on software implementation
The robot was fully programmed with the Choregraphe Suite. Choregraphe is a multi-platform application that allows users to monitor and control the NAO robot on its graphical user interface with pre-built boxes. Moreover, users may enrich the robot’s behaviors by editing pre-built python codes or importing new libraries. For advanced users, the robot can be programmed in Character User Interfaces such as NAO SDKs or the Robot Operating System. However, these applications require a high level of programming skills. Among the three applications, Choregraphe is the easiest to learn and comprehensive enough for the development of a learning companion software package.
Choregraphe is an official software provided by SoftBank Robotics. It can be used on other SoftBank robots such as Pepper and NAO. Once the software is fully developed, it can be sent to other devices with Choregraphe and run on any supported robots. It supports Windows, Mac, and Linux systems, thus enhancing the ease of setting up the robot–computer connection across different devices. Additionally, this enabled us to collaborate with schools that already own SoftBank Robots, as we only had to provide them with our software and printable learning aids. Schools and individual users who own SoftBank robots can access the learning package online, switching their humanoid learning companion from one topic to another based on their needs.
4. Design and implementation
In this project, a learning package for basic measurements with the aid of a NAO humanoid robot was developed. The expected learning outcomes were: (1) to recognize the concepts of lengths (centimeters/meters), (2) to use different ways to compare lengths, (3) to understand the need to use standard units of measurement, (4) to choose appropriate measuring tools and units, and (5) to estimate the result of measurement in real life. Our system consists of a number of elements incorporating the humanoid robot that are discussed in the following section.
4.1. Establishing the learning package
Figure illustrates the whole system architecture. It starts from pedagogical design, where the teacher designs a supporting teaching and learning package and gives tailored instructions to the Choregraphe development platform that directly communicates with the robot. The learning package was developed for the learning unit 2M1: Length and distance (III). According to Education Bureau (Citation2018), students should be able to (1) recognize meter (m), (2) measure and compare the lengths of and between objects in metres, (3) record the lengths in appropriate measuring units, and (4) estimate the measurement results with an ever-ready ruler.
Figure 1. System architecture.
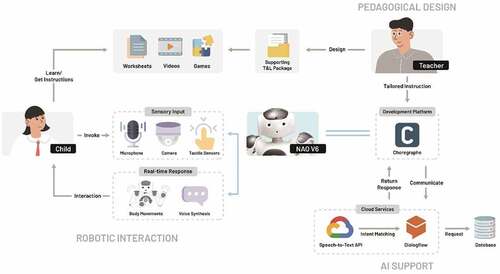
The learning package covers all of these learning objectives and consists of a set of four videos, five worksheets (contents slightly differ between face-to-face version and online version via Zoom) and six playing cards (see, Figure ).
Figure 2. Sets of worksheets and playing cards.
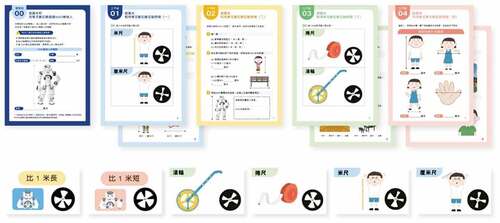
At the beginning of the face-to-face activity, the robot was placed on a table in front of the children at the same eye level. For the Zoom activity, the robot was placed on a table in front of a tablet computer with a camera, microphone, and speaker. For normal body movements and postures, sufficient space in NAO’s arm span was allowed. The NAO robot presented the PowerPoint lessons with a laptop computer next to it. The computer was used to show the slides so that NAO would refer to them. Then, NAO would turn into a learning companion and finish the corresponding worksheets with the students. When the program was executed, NAO took the lead to talk first and then waited for responses.
This human–robot interactive communication consisted of both non-verbal and verbal cues. Children invoked a conversation, and the robot perceived the environment with its sensory units and returned real-time responses. Cameras were activated when the activity required users to use the playing cards. The robot looked for special landmarks with white triangle fans centered at the circle’s center, as shown in Figure . It recognized the NAOMark on the playing cards and returned corresponding responses. The tactile sensors on its hands were set up to provide timely touch to arouse children’s attention throughout the lesson. Its head capacitive sensors were accessed when the robot waited for a non-verbal cue after a measurement exercise.
When NAO was waiting for a verbal cue, the front microphones were activated for 6–8 seconds, with the eyes’ LED turning blue. Successful voice recordings were passed to the Google Speech-to-Text API. The transcribed text would then be passed to Dialogflow. When a matching intent was found, Dialogflow returned a response to the robot. The robot executed the moves as if it was talking expressively.
4.2. Interactive dialogue
Besides conducting the lessons, the robot could engage in a lively conversation. Children could use another set of question cards to enjoy playing with the robot. The robot could answer many questions about hobbies, families, friends, school, and more. The teacher could modify the question bank on Dialogflow to suit the student’s ability and needs.
As NAO is an anthropomorphic robot, several responses were modified to look more human-like. If the robot asked for verbal responses but detected a null message, it would tell the user that it could not hear well and ask them to say again. The robot would also help when it received no response in several minutes or when the user made mistakes more than twice. The loop of this process simulated human-like interactive behaviors and thus achieved social bonding with children.
The robot would check whether the user would like to continue with the conversation. The dialog would end when the user said words like “nothing else” or “bye-bye.” Additionally, there were possibilities that our conversational agent did not recognize an end-user expression. A fallback intent would be automatically matched, and the robot would guide the user to ask another question.
5. Method
5.1. Experimental environment and participants
The children were recruited from a local church center. They were native Cantonese speakers aged between primary 3 and 4. One teaching assistant was present in the room, seated at a table from the connection between the robot and the children. Two video cameras were placed in front and behind the children to record the process. Some of their parents also attended the session to observe the process. Among all the children in the activity, three voluntary participants (1 girl in primary 3 and 2 girls in primary 4) who had participated in all the procedures were selected for a detailed analysis.
5.2. Procedures
The study took place inside a room where the staff usually tells children bible stories. It was a place familiar and relaxing to the children. After their Saturday services, children participants were recruited to meet a new robot friend. They were eager to meet and play with the robot for around an hour.
The staff first welcomed the children and introduced the purpose of the robot. Then, the researcher connected the robot to the cloud services wirelessly based on pre-written programs. The robot introduced itself and asked the children if they had any questions. The children picked question cards and asked the robot. After that, NAO taught the children a 5-minute-long lesson about measurements and gave the children a short quiz. Throughout the lesson, all children had a chance to grasp the robot’s hand, answer questions, and show the playing cards to the robot (see, Figure ). In the remaining time, the children watched the robot dance, act as a musician, and answer more questions. At last, the children were asked to attend a focus group interview to assess their attitude toward NAO as a learning companion.
Figure 3. (a) Children were holding the robot’s hand and (b) showing cards to the robot.
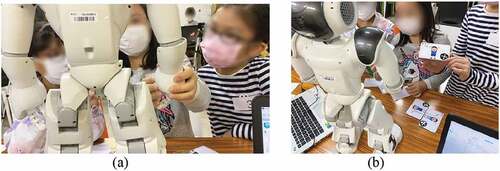
The robot substituted the teacher by delivering a lesson with PowerPoint slides (see, Figure ). It took over the ordinary tasks of a teacher, such as giving lectures, asking questions, and checking the children’s progress. The class helped children understand the measurement topic by explaining the main concepts and demonstrating how to record the lengths. Throughout the lesson, the robot accessed the children’s progress by receiving verbal interactions and visual inputs with activity cards.
5.3. Annotation
The ELAN 6.0 annotation tool (ELAN, Citation2021; see, Figure ) was used to measure the children’s social signals. It is widely adopted in academia, including disciplines in psychology, education, and behavioral studies, and on topics in human–robot interaction, gesture analysis, group analysis, language acquisitions, and more. ELAN can perform systematic analysis in human–robot interaction (Ros et al., Citation2014; Giuliani et al., Citation2015). Qualitative data, such as behavioral and verbal cues, can be visualized in these cases.
Figure 5. Screenshot of an annotated video in ELAN.
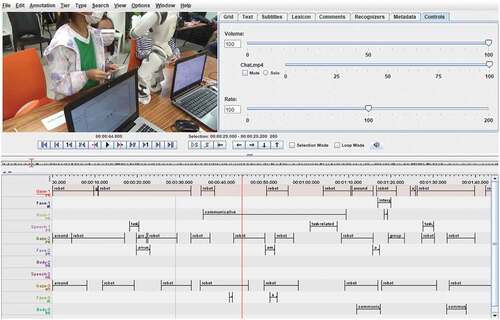
The video was processed separately in three sessions: the Q&A time, the 5-minute-long lesson, and the activity session. All actions and social signals the participants showed were annotated in the coding process. We used the following classes to measure the participation of the children: (Items adapted from Giuliani et al. (Citation2015))
Gaze: instances where the child makes eye contact with someone or something. It includes looking at the sides, the robot, the group members, and the experimenter.
Face: facial expressions that are observable on the child’s face. It includes but not limited to smiles, laughter, yawns, and confused or surprised faces.
Body: any body movements and gestures made with the child’s body and hands, including moving around, showing body gestures, or touching the robot by its initiative or any expressions that indicate agreement and acceptance.
Speech: verbal utterances by the children, including task-related sentences, unexpected replies such as greetings and courtesies, and other replies worth noting.
6. Results
6.1. Video analysis
The video recordings displayed that children were comfortable being with the robot. Although the children were quite hesitant to ask questions at first, they were astonished by the ability of the robot. They showed interest in the robot and paid close attention to its body movements and speech. The communication was smooth. Children touched its head and hands throughout the lesson and asked the robot to demonstrate different tasks as if it was a friend to play with (e.g., “Can you dance please?” and “Can you play the guitar?”).
During the lesson, the robot faced a technical problem; it could not perceive the children’s voices very well. Despite the robot asking them to repeat a few times, the children showed no signs of dissatisfaction or impatience. They got closer to the robot, spoke louder, repeated the answer slowly, and ensured that the robot could hear them. They treated the robot as a peer rather than just a toy because they listened to the robot’s needs and accommodated it in the process.
The children claimed they would love to play with the robot and participate in every part of the lesson. Clearly, they all asked questions, touched the robot, and used the playing cards at least once. Furthermore, they were very excited when the researcher offered them another chance to ask more questions in the remaining time.
Table overviews all annotated social signals in four categories (head movements, facial expressions, body movements and speech) as shown below.
Table 2. Counts for all annotated social signals in head movements, facial expressions, body movements, and speech
Regarding head movements, the participant looked at the robot more than the experimenter and their groupmates. The trends were similar to a prior study by Giuliani et al. (Citation2015). When there were PowerPoint slides, they looked back and forth between the robot and the PowerPoint as if the robot was a human teacher giving them instructions. There was some idle time throughout the experiment, so the children also looked around a few times.
In terms of facial expressions, children were mostly amused. They smiled or raised eyebrows when the robot moved or asked for interaction. This phenomenon aligns with a previous study that students also portrayed more positive facial expressions than that of the human condition (Mubin et al., Citation2019). Facial expressions and body gestures were sometimes noticed when the robot demonstrated its ability to speak Cantonese fluently. However, the children occasionally displayed a bored face when they were waiting for their turn or encountered an error situation such as not hearing well.
Participants also showed body movements while interacting with the robot. Children showed communicative body gestures in front of the robot. They leaned toward the robot to answer questions, and all children grabbed its hand when the robot required a physical touch to continue. During error situations and idle time, children were often distracted, but they never walked away from the robot and returned immediately when the interaction resumed again. Remarkably, they seldom nodded or showed any head movements of agreement and acceptance.
For speech, the children usually spoke when the robot required an answer to move the task forward. They also greeted the robot with “hello” and “goodbye.” Children showed courtesy with “please,” “thank you,” and “can you … ” as if it was a human being. Some audible laughter and verbal communication among group members were observed as well.
6.2. Focus group analysis
The interview emphasized the findings in video recordings. There was a consensus that they liked to learn with the robot and that learning was happier with its presence. Some comments provided by the children were extracted and presented in Table .
Table 3. Some replies from the focus group interview
7. Discussion
The findings from the video recordings and the short quiz in the child–robot interaction demonstrated the positive effects of introducing a NAO robot in informal learning to the young children and, importantly, the possibility of transferring the activity into the classroom of primary mathematics lessons.
7.1. Children’s impression of the NAO robot
A natural interaction between the robot and the children was observed. We assumed that the friendly appearance of the robot aroused their interest and positively impacted the learning atmosphere. They were curious and behaved pleasantly during the robot-theme activities. Yousif and Yousif (Citation2020) mentioned that the appearance of humanoid robots arouses students’ interest to engage with the learning materials and improve communication skills. Socially acceptable humanoid robots provide an interactive learning environment, especially for young children. Besides its anthropomorphic characteristics, its ability to show empathy and provide smooth interaction improves its acceptance as a learning companion (Pelau et al., Citation2021). Although the robot cannot demonstrate facial expressions, it expresses feelings by mimicking human body language (Bertacchini et al., Citation2017). Chalmers et al. (Citation2022) also reported that students are highly engaged, and the robot helps maintain enthusiasm throughout the interaction. The results of this study corroborate and add evidence to the findings of previous works. We noted that the children were eager to talk to the robot and get to know its hobbies and life. The presence of the robot brought excitement and enthusiasm to the session.
We also noted that the children demonstrated caring behavior when they were told that the robot could not hear them. Owing to some technical reasons and the limitation of the environment, the NAO robot could only understand verbal cues when the user stood in front of it and spoke loudly. All children stood up and got close to the robot on their initiative whenever they had to talk with the robot. They waited patiently until the robot’s eyes turned blue before they spoke. The children also grab the robot’s hand and showed correct activity card when it required a physical touch to continue the task. They gathered around the robot, held its hand right before it gave clear instructions, and put an activity card in front of its eyes before it finished asking a question. Whereas past researchers have found 3–5 year old children paid attention to the NAO robot, particularly when it was in need (Ioannou et al., Citation2015), the present study has shown that primary school kids also act the same way. One interpretation of these findings is that children tend to take care of the robot and eventually interact with it as a peer. In other words, the robot motivates the children to respond in the session, thus encouraging effective participation. However, they usually got distracted while waiting but returned after the error was corrected.
Furthermore, consistent with the previous study, our results suggest that young children develop friendly attitudes toward interactive robots (Kanda et al., Citation2007) but lose their attention occasionally during the activity.
7.2. NAO robot as a learning companion in class
Upon further observation and behavior analysis, children actively participated in classroom activities in the presence of the NAO robot. It fitted the need for a learning companion. We found audible laughter from the video recordings and attempts to touch or talk to the NAO robots occasionally. The statistics in Table contribute to a clearer understanding of how students engaged in a learning activity accompanied by NAO. The selected participants displayed active classroom engagements (frequent eye contact and body movements) through robotic activities aligned with current research (Chen et al., Citation2020; Kim et al., Citation2015; Liu et al., Citation2022).
Table 4. Signals shown and their corresponding duration (in seconds) in the selected participants
The robot passed instructions to the children with verbal commands and body postures during the session. Previous research asserts that interactive robots can make use of children’s interests to bring out better interaction, such as by being friendlier with children or hiding its mechanism behind (Kanda et al., Citation2007). They do not make commands like teachers but invite children to study with friendly phrases such as “Let us look at the slides!” or “Let us finish the exercise together!”. The statistics in Table suggest that the NAO robot could be used as a tool to arouse students’ interest and encourage children to engage in the activities during the lesson.
Table 5. Social signals (head movements) and their duration (in seconds) in the selected participants
Students looked at the PowerPoint learning materials frequently. One interesting fact is that the occurrence of looking at the robot is much more than being distracted (looking around). They tended to make eye contact with the robot even if humans were visible in the room. Our participants exhibited a matching phenomenon of previous research by Highfield (Citation2010), where children demonstrate perseverance, motivation and responsiveness to these activities that are not usually evident in their regular programs.
Table displays that NAO robot highly influences communicative body movements. Throughout the experiment, there were high occurrences of communicative body gestures and distractions. Velentza et al. (Citation2021) explained that students will get bored when they become familiar with the robot. In addition, the robot does not set any classroom rules and asks the students to obey. Since obedience and respect are deeply rooted values, especially in traditionally conservative countries (Sun & Shek, Citation2021; Zedan, Citation2010), the classroom atmosphere is intertwined with the teacher’s authority. The data show that the robot’s appearance and voice did not display any authority in front of the children. As the children did not see any authority figures in front of them, they inevitably got distracted from time to time.
Table 6. Social signals (body movements) and their duration (in seconds) in the selected participants
7.3. Successes and challenges
We encountered many successes and challenges throughout the study. Table summarizes these successes and challenges of using a humanoid robot for teaching to and learning with children.
Table 7. Successes and challenges
As the humanoid robot is not a common tool used in Hong Kong schools, teachers and researchers may find it difficult to build upon past experiences and examples. Apparently, one of the biggest challenges of using a humanoid robot to teach is technical issues. In related research studies, we also observed similar problems including voice recognition consistency and Wi-Fi connections (Chalmers et al., Citation2022). An experienced teacher should be present whenever NAO robots are used in class (Majgaard, Citation2015). Students will have to wait for the robot to reset whenever this incident happens and then repeat their answers. In idle time, children may get distracted and lose their patience. Moreover, if only one robot is available for all students, they will get bored and distracted when waiting for their turn. The balance between the number of students and robots should be considered when preparing similar learning activities.
7.4. Limitation and future development
Children had a positive impression and showed no complaints about the robot in the focus group. They tended to compare NAO with teachers and responded that NAO is friendlier and funnier than teachers. In other words, they preferred to learn with NAO rather than human teachers. Our finding suggests that NAO can evoke positive feelings in learning and establish a positive student–teacher relationship with children. NAO may be able to draw students into acquiring new knowledge and encourage them to participate together.
More trials from participants of similar age and in different settings, for example, during class time, after-school, tutorial class, or at home, can provide stronger evidence to support the findings. Other researchers also called for further studies in various settings, such as natural school environments and a more extensive period (Crompton et al., Citation2018; Fridin & Belokopytov, Citation2014).
Academics outline further research directions in robotics, including facial recognition, expressing a broad range of emotions and adapting their immediate feelings to behaviors (Tuna & Tuna, Citation2019). To broaden the scale of the study and promote the use of NAO robots in local school settings, professional training sessions on using educational humanoid robots may be needed to introduce them to the local teachers. Furthermore, working in close collaboration with local primary schools and providing customized solutions to fit students’ needs will lead to the best use of educational humanoid robots.
8. Conclusion
This work corroborates the current findings by Chalmers et al. (Citation2022) and Ioannou et al. (Citation2015). It extends the academic understanding of how humanoid robots can support early mathematics learning in Hong Kong. By developing a robot-driven learning package, this study contributes to the robotic research in education showcasing how the NAO robot as a learning aid can fit into the current mathematics curriculum. Specifically, the results show that NAO robots can teach, maintain a comfortable learning atmosphere, and build healthy two-way relationships with students.
The analysis shows that the NAO robot successfully aroused the children’s interest and delivered a short mathematics lesson to a group of children without a human teacher. The participants demonstrated a variety of social signals, including head movements, facial expressions, body gestures, and verbal cues. They recognized the robot as a friendly learning companion and actively participated in the activities. However, it was challenging to integrate a NAO robot into a formal setting without trained personnel because of unforeseen technical issues and the limitation of the learning environment.
The results demonstrate that the NAO robot can build a positive, friendly relationship with children while achieving learning outcomes in mathematics. Our analysis also shows that video annotations of child–robot interaction are possible and produce practical results when coded in various categories by different social signals. The findings support the deployment of NAO robots in the mathematics curriculum and advocate its use to achieve learning outcomes whilst maintaining a harmonious relationship. Further psychology research regarding children’s behavior is undoubtedly required to validate the positive influences of learning mathematics with humanoid robots.
The social signal and focus group analyses presented in this study offer detailed insights that NAO robots could assist in teaching mathematics in the future. NAO can easily be introduced into the curriculum to provide an interactive learning stimulus with appropriate pedagogical design and technology integration. Altogether, there are new possibilities for humanoid robots in educating young people, but they require further longitudinal and experimental studies to mature the field. The research outcomes of this study could proliferate the pedagogical and technological development of humanoid robotic systems in education for young children.
Acknowledgements
This study was funded by the Central and Faculty Fund of the Faculty of Liberal Arts and Social Sciences, The Education University of Hong Kong
Disclosure statement
No potential conflict of interest was reported by the author(s).
Additional information
Funding
Notes on contributors
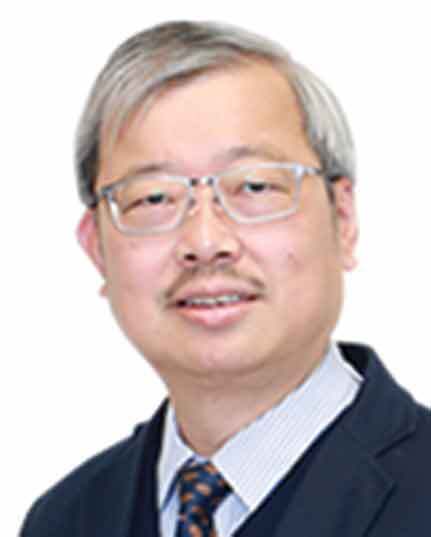
Simon So
Dr. Simon So (BSc, PGDE, MEd, MStatistics, PhD) joined the Hong Kong Institute of Education (now named the Education University of Hong Kong) in 2000 and has been a faculty member in the University with over 20 years of experience in training ICT teachers. He has published research articles widely and his publications include books’ chapters and articles in prestige journals, professional series and international conferences. His services to the international research community include serving as Program Committee member, Organizing Member, Technical Expert and Regional Director of major international conferences and professional organizations in Australia, Europe, Asia and US. He has supervised master and doctorial students in education and has been appointed as the external examiner for a number of doctorial and master research dissertations. He is the reviewer of many prestige journal articles and international conference papers, and was nominated as the top reviewer by a number of prestige journals. Dr. So also served as the subject member and provides consultation works for government bodies in the past. His recent research interests include STEM education, mobile learning, game-based learning, extended reality, robotics and artificial intelligence in education. Before joining the University, he has many years of industrial and academic experience in Canada, Hong Kong and Australia.
References
- Alemi, M., Meghdari, A., & Ghazisaedy, M. (2014). Employing humanoid robots for teaching English language in Iranian junior high-schools. International Journal of Humanoid Robotics, 11(3), 1450022. https://doi.org/10.1142/S0219843614500224
- Al Hakim, V. G., Yang, S.-H., Liyanawatta, M., Wang, J.-H., & Chen, G.-D. (2022). Robots in situated learning classrooms with immediate feedback mechanisms to improve students’ learning performance. Computers and Education, 182, 104483. https://doi.org/10.1016/j.compedu.2022.104483
- Bautista, A. J., & Wane, S. O. (2018). ATLAS robot: a teaching tool for autonomous agricultural mobile robotics. International Conference on Control, Automation and Information Sciences (ICCAIS), 2018, pp. 264–18, https://doi.org/10.1109/ICCAIS.2018.8570494
- Baxter, P., Belpaeme, T., Canamero, L., Cosi, P., Demiris, Y., Enescu, V., & Wood, R. (2011). Long-term human-robot interaction with young users. In IEEE/ACM human-robot interaction 2011 conference (robots with children workshop) (Vol. 80). http://doi.org/10.5898/JHRI.5.1.Coninx
- Belpaeme, T., Baxter, P., Read, R., Wood, R., Cuayáhuitl, H., Kiefer, B., Racioppa, S., Kruijff-Korbayová, I., Athanasopoulos, G., Enescu, V., Looije, R., Neerincx, M., Demiris, Y., Ros-Espinoza, R., Beck, A., Cañamero, L., Hiolle, A., Lewis, M., Baroni, I., Nalin, M., Cosi, P., Paci, G., Tesser, F., Sommavilla, G., & Humbert R. (2013). Multimodal child-robot interaction: building social bonds. Journal of Human-robot Interation, 1(2), 33–53. http://dx.doi.org/10.5898/JHRI.1.2.Belpaeme
- Belpaeme, T., & Tanaka, F. (2021). OECD Digital Education Outlook 2021 Pushing the Frontiers with Artificial Intelligence, Blockchain and Robots: Pushing the Frontiers with Artificial Intelligence, Blockchain and Robots. OECD Publishing, 143. https://www.oecd-ilibrary.org/education/oecd-digital-education-outlook-2021_589b283f-en
- Bertacchini, F., Bilotta, E., & Pantano, P. (2017). Shopping with a robotic companion. Computers in Human Behavior, 77, 382–395. https://doi.org/10.1016/j.chb.2017.02.064
- Brown, L., & Howard, A. M. (2013). Engaging children in math education using a socially interactive humanoid robot. 2013 13th IEEE-RAS International Conference on Humanoid Robot(Humanoids), 2015(February), 183–188. https://doi.org/10.1109/HUMANOIDS.2013.7029974
- Chalmers, C., Keane, T., Boden, M., & Williams, M. (2022). Humanoid robots go to school. Education and Information Technologies, 27(6), 7563–7581. https://doi.org/10.1007/s10639-022-10913-z
- Cheng, Y.-W., Sun, P.-C., & Chen, N.-S. (2018). The essential applications of educational Robot: Requirement analysis from the perspectives of experts, researchers and instructors. Computers and Education, 126, 399–416. https://doi.org/10.1016/j.compedu.2018.07.020
- Chen, H., Park, H. W., & Breazeal, C. (2020). Teaching and learning with children: Impact of reciprocal peer learning with a social robot on children’s learning and emotive engagement. Computers and Education, 150, 103836. https://doi.org/10.1016/j.compedu.2020.103836
- Crompton, H., Gregory, K., & Burke, D. (2018). Humanoid robots supporting children’s learning in an early childhood setting. British Journal of Educational Technology, 49(5), 911–927. https://doi.org/10.1111/bjet.12654
- Education Bureau. (2016). Report on Promotion of STEM Education: Unleashing Potential in Innovation. Education Bureau. HKSAR. https://www.edb.gov.hk/attachment/en/curriculum-development/renewal/STEM%20Education%20Report_Eng.pdf
- Education Bureau. (2018). Explanatory Notes to Primary Mathematics Curriculum (Key Stage 1). Education Bureau HKSAR. https://www.edb.gov.hk/attachment/en/curriculum-development/kla/ma/curr/EN_KS1_e.pdf
- ELAN. (2021). Nijmegen: Max Planck Institute for Psycholinguistics. (Version 6.0) [Computer software]. ((n.d.)): https://archive.mpi.nl/tla/elan
- Fridin, M. (2014). Storytelling by a kindergarten social assistive robot: A tool for constructive learning in preschool education. Computers and Education, 70, 53–64. https://doi.org/10.1016/j.compedu.2013.07.043
- Fridin, M., & Belokopytov, M. (2014). Acceptance of socially assistive humanoid robot by preschool and elementary school teachers. Computers in Human Behavior, 33, 23–31. https://doi.org/10.1016/j.chb.2013.12.016
- Giuliani, M., Mirnig, N., Stollnberger, G., Stadler, S., Buchner, R., & Tscheligi, M. (2015). Systematic analysis of video data from different human–robot interaction studies: A categorization of social signals during error situations. Frontiers in Psychology, 6, 931. https://doi.org/10.3389/fpsyg.2015.00931
- Han, J., Jo, M., Jones, V., & Jo, J. H. (2008). Comparative study on the educational use of home robots for children. Journal of Information Processing Systems, 4(4), 159–168. https://doi.org/10.3745/JIPS.2008.4.4.159
- Hanks, W. F. (1991). Foreword. In J. Lave & E. Wenger (Eds.), Situated learning: Legitimate peripheral participation (pp. 13–23). Cambridge University Press. https://www.cambridge.org/highereducation/books/situated-learning/6915ABD21C8E4619F750A4D4ACA616CD#overview
- Hashimoto, T., Kato, N., & Kobayashi, H. (2011). Development of educational system with the android robot SAYA and evaluation. International Journal of Advanced Robotic Systems, 8(3), 51–61. https://doi.org/10.5772/10667
- Hashimoto, T., Kobayashi, H., Polishuk, A., & Verner, I. (2013). Elementary science lesson delivered by robot. Proceedings of the 8th ACM/IEEE International Conference on Human-Robot Interaction, 133–134. https://doi.org/10.1109/HRI.2013.6483537
- Highfield, K. (2010). Robotic toys as a catalyst for mathematical problem solving. Australian Primary Mathematics Classroom, 15(2), 22–27. https://doi.org/10.3316/informit.150648554236567
- Ioannou, A., Andreou, E., & Christofi, M. (2015). Pre-schoolers’ interest and caring behaviour around a humanoid robot. TechTrends, 59(2), 23–26. https://doi.org/10.1007/s11528-015-0835-0
- Kanda, T., Hirano, T., Eaton, D., & Ishiguro, H. (2004). Interactive Robots as Social Partners and Peer Tutors for Children: A Field Trial. Human-Computer Interaction, 19(1–2), 61–84. https://doi.org/10.1080/07370024.2004.9667340
- Kanda, T., Sato, R., Saiwaki, N., & Ishiguro, H. (2007). A two-month field trial in an elementary school for long-term human-robot interaction. IEEE Transactions on Robotics, 23(5), 962–971. https://doi.org/10.1109/TRO.2007.904904
- Kim, C., Kim, D., Yuan, J., Hill, R. B., Doshi, P., & Thai, C. N. (2015). Robotics to promote elementary education pre-service teachers’ STEM engagement, learning, and teaching. Computers and Education, 91, 14–31. https://doi.org/10.1016/j.compedu.2015.08.005
- Kory Westlund, J., Gordon, G., Spaulding, S., Lee, J. J., Plummer, L., Martinez, M., Das, M., & Breazeal, C. (2016). Lessons from teachers on performing hri studies with young children in schools. 2016 11th ACM/IEEE International Conference on Human-Robot Interaction (HRI), 2016-, 383–390. https://doi.org/10.1109/HRI.2016.7451776
- Kwon, O. H., Koo, S. Y., Kim, Y. G., & Kwon, D. S. (2010). Telepresence robot system for English tutoring. Proceedings of IEEE Workshop on Advanced Robotics and its Social Impacts (ARSO), IEEE, 152–155. https://doi.org/10.1109/ARSO.2010.5679999
- Lave, J., & Wenger, E. (1991). Situated learning legitimate peripheral participation. Cambridge University Press. ((n.d.)) https://bibliotecadigital.mineduc.cl/bitstream/handle/20.500.12365/17387/cb419d882cd5bb5286069675b449da38.pdf?sequence=1
- Learning and Teaching Resources for STEM Education (KS1 – Primary 1 to 3). (2023). The Education Bureau. ((n.d.)): https://stem.edb.hkedcity.net/en/learning-and-teaching-resources-for-steam-education-ks1-primary-1-to-3/
- Lee, S., Noh, H., Lee, J., Lee, K., & Lee, G. G. (2010). Cognitive effects of robot-assisted language learning on oral skills. In Proceedings of the Interspeech 2010 Satellite Workshop on Second Language Studies: Acquisition, Learning, Education and Technology ((n.d.)): http://isoft.postech.ac.kr/publication/iconf/islsw10_lee.pdf.
- Lei, X., & Rau, P.-L. P. (2021). Effect of robot tutor’s feedback valence and attributional style on learners. International Journal of Social Robotics, 13(7), 1579–1597. https://doi.org/10.1007/s12369-020-00741-x
- Liu, -C.-C., Liao, M.-G., Chang, C.-H., & Lin, H.-M. (2022). An analysis of children’ interaction with an AI chatbot and its impact on their interest in reading. Computers and Education, 189, 104576. https://doi.org/10.1016/j.compedu.2022.104576
- Mahdi, Q. S., Saleh, I. H., Hashim, G., & Loganathan, G. B. (2021). Evaluation of robot professor technology in teaching and business. Information Technology in Industry, 9(1), 1182–1194. https://doi.org/10.17762/itii.v9i1.255
- Majgaard, G. (2015). Humanoid robots in the classroom. IADIS International Journal on WWW/Internet, 13(1), ((n.d.)). https://www.iadisportal.org/ijwi/papers/2015131106.pdf
- Mubin, O., Alhashmi, M., Baroud, R., & Alnajjar, F. (2019). Humanoid robots as teaching assistants in an Arab school. ACM International Conference Proceeding Series, 462–466. https://doi.org/10.1145/3369457.3369517
- Mubin, O., Stevens, C. J., Shahid, S., Al Mahmud, A., & Dong, J. J. (2013). A review of the applicability of robots in education. Journal of Technology in Education and Learning, 1(209-0015), 13. http://dx.doi.org/10.2316/Journal.209.2013.1.209-0015
- Oh, K., & Kim, M. (2010). Social attributes of robotic products: Observations of child-robot interactions in a school environment. International Journal of Design, 4(1), 45–55. ((n.d.)). http://www.ijdesign.org/index.php/IJDesign/article/view/578/278
- Okita, S. Y., Ng-Thow-Hing, V., & Sarvadevabhatla, R. (2009). Learning together: ASIMO developing an interactive learning partnership with children. RO-MAN 2009-The 18th IEEE International Symposium on Robot and Human Interactive Communication, 1125–1130. https://doi.org/10.1109/ROMAN.2009.5326135
- Oliver, R., & Herrington, J. (2000). Using situated learning as a design strategy for Web- based learning. In Instructional and cognitive impacts of web-based education, 178–191. IGI Global. https://doi.org/10.4018/978-1-878289-59-9.ch011
- Osada, J., Ohnaka, S., & Sato, M. (2006). The scenario and design process of childcare robot, PaPeRo. ACM international conference proceeding series; Vol. 266: Proceedings of the 2006 ACM SIGCHI International Conference on Advances in Computer Entertainment Technology, 80–es. https://doi.org/10.1145/1178823.1178917
- Papadopoulos, I., Lazzarino, R., Miah, S., Weaver, T., Thomas, B., & Koulouglioti, C. (2020). A systematic review of the literature regarding socially assistive robots in pre- tertiary education. Computers and Education, 155, 103924. https://doi.org/10.1016/j.compedu.2020.103924
- Pelau, C., Dabija, D.-C., & Ene, I. (2021). What makes an AI device human-like? The role of interaction quality, empathy and perceived psychological anthropomorphic characteristics in the acceptance of artificial intelligence in the service industry. Computers in Human Behavior, 122, 106855. https://doi.org/10.1016/j.chb.2021.106855
- Robaczewski, A., Bouchard, J., Bouchard, K., & Gaboury, S. (2021). Socially assistive robots: The specific case of the NAO. International Journal of Social Robotics, 13(4), 795–831. https://doi.org/10.1007/s12369-020-00664-7
- Ros, R., Baroni, I., & Demiris, Y. (2014). Adaptive human-robot interaction in sensorimotor task instruction: From human to robot dance tutors. Robotics and Autonomous Systems, 62(6), 707–720. http://dx.doi.org/10.1016/j.robot.2014.03.005
- Shiomi, M., Kanda, T., Ishiguro, H., & Hagita, N. (2006). Interactive humanoid robots for a science museum. In Proceedings of the 1st ACM SIGCHI/SIGART conference on Human-robot interaction, 305–312. https://doi.org/10.1145/1121241.1121293
- Sun, R. C. F., & Shek, D. T. L. ((2012). Student classroom misbehavior: An exploratory study based on teachers’ perceptions. TheScientificWorld, 2012, 208907–208908. https://doi.org/10.1100/2012/208907
- Tanaka, F., Isshiki, K., Takahashi, F., Uekusa, M., Sei, R., & Hayashi, K. (2015). Pepper learns together with children: Development of an educational application. In Proceedings of the 15th International Conference on Humanoid Robots, IEEE, Seoul, South Korea, 270–275. https://doi.org/10.1109/HUMANOIDS.2015.7363546
- Tuna, A., & Tuna, G. (2019). The use of humanoid robots with multilingual interaction skills in teaching a foreign language: Opportunities, research challenges and future research directions. Center for Educational Policy Studies Journal, 9(3), 95–115. https://doi.org/10.26529/cepsj.679
- Velentza, A.-M., Fachantidis, N., & Lefkos, I. (2021). Learn with surprize from a robot professor. Computers and Education, 173, 104272. https://doi.org/10.1016/j.compedu.2021.104272
- Vogt, P., van den Berghe, R., de Haas, M., Hoffman, L., Kanero, J., Mamus, E., Montanier, J., Oranç, C., Oudgenoeg-Paz, O., Garcia, D., Papadopoulos, F., Schodde, T., Verhagen, J., Wallbridge, C., Willemsen, B., de Wit, J., Belpaeme, T., Göksun, T., Kopp, S., Krahmer, E., Küntay, A., Leseman, P., & Pandey A. (2019), “Second language tutoring using social robots: L2TOR - The Movie”, 2019 14th ACM/IEEE International Conference on Human-Robot Interaction (HRI), https://doi.org/10.1109/HRI.2019.8673016
- Xu, J., Broekens, J., Hindriks, K., & Neerincx, M. A. (2014,). Effects of bodily mood expression of a robotic teacher on students. In 2014 IEEE/RSJ International Conference on Intelligent Robots and Systems, 2614–2620. https://doi.org/10.1109/IROS.2014.6942919
- Yousif, M. J., & Yousif, J. H. (2020). Humanoid Robot as Assistant Tutor for Autistic Children. International Journal of Computation and Applied Sciences, 8(2). ((n.d.)): https://papers.ssrn.com/sol3/papers.cfm?Abstract_id=3616810
- Zedan, R. (2010). New dimensions in the classroom climate. Learning Environments Research, 13(1), 75–88. https://doi.org/10.1007/s10984-009-9068-5