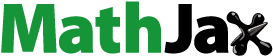
Abstract
Peer learning is a distinct category of student support gaining popularity globally and in South Africa, although these are typically informal approaches which have not been sufficiently researched to examine their influence and impact. Some scholars have criticised the available research as patchy, inconsistent, and methodologically weak. Underpinned by the author’s interest in peer learning in South Africa, this meta-analysis examines the outcomes of formal peer learning approaches on-course performance. The authors identified 1645 articles from four search engines which were published between 2010 and 2021. Of these, only 37 articles written in English, with a control and comparison group, reported effect sizes, were included in the analysis as they met the specified inclusion criteria. The analysis was conducted using R (version 4.0.0) and the metafor package (version 2.4.0). Effect sizes and their variances were computed within each study to allow comparability across studies using the common effect size metric, Hedges’s g statistic. Our findings suggest a moderately strong relationship between peer learning approaches and course performance; however, its significance cannot be ignored. As such, universities should consider whether such moderate effects justify the money, time and effort invested in peer learning approaches. While this meta-analysis provides external validity, more experimental studies are required in South Africa to provide a more contextually robust evidence base to inform policy.
1. Introduction
Student success is arguably one of the most widely deliberated topics in higher education, with several studies showing that it remains a significant problem globally. Why is student progression proving to be such an intractable challenge? The problem is multifarious, but increasingly, studies have identified articulation gaps and student under-preparedness as contributing factors (see, Dhunpath & Subbaye, Citation2018; Dhunpath & Vithal, Citation2012; Lavhelani et al., Citation2020). In response, several higher education institutions have invested significant resources in student support programmes, which include tutoring, mentoring, supplemental instruction, peer-wellness and peer-learning (Dhunpath & Subbaye, Citation2018). In this article, given South Africa’s unsustainably low progression rates, we use a meta-analytic method to appraise the value and efficacy of peer-learning approaches and consider whether significant resource investments are warranted in relation to the available empirical evidence.
The problem of student success is widespread and prevalent even in developed countries. For instance, it is estimated that the average dropout in the Organisation for Economic Co-operation and Development (OECD) countries is approximately 30%, with the status quo being worse in Italy, the United States of America and New Zealand, where the rate is almost twice the regional average (European, Citation2015). Similar trends have also been observed in the BRICS countries (Brazil, Russia, India, China and South Africa), which constitute a grouping of emerging world economies (daCosta, Bispo, & Pereira, Citation2018; Elena, Citation2018). For instance, daCosta et al. (2018) report that Brazil’s average dropout rate is between 40–50%. Russia, which has one of the lowest dropout rates in both OECD and BRICS, on average, 22% of the students leave without completing a degree (Elena, Citation2018).
In South Africa, university progression is perhaps the most analysed topic in higher education, against a backdrop of low participation and degree completion (Council on Higher Education, & CHE, Citation2013), as depicted in Figure below:
Figure 1. Throughput from Grade 1 to university, 2008 matric cohort. ref. CHE, Briefly Speaking, October 2017 (Figure redrawn from: Spaull (2016), ‘Important research inputs on #FeesMustFall’).
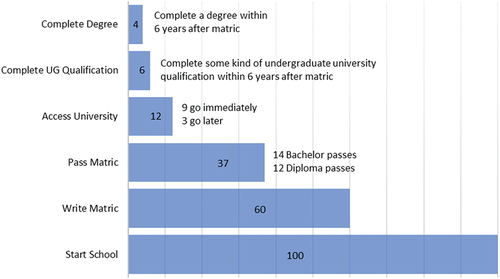
STUDENT SUCCESS IN THE SOUTH AFRICAN EDUCATION SYSTEM
Of those students who manage to enter higher education in South Africa, an estimated 50% leave before graduating (CHE, Citation2013). A broad range of factors that impact student success have been identified. These include demographic characteristics, previous academic achievement, financial aid, self-efficacy, and learning styles. Some scholars have suggested that most students leave university due to adjustment or environmental factors rather than intellectual difficulties (Tinto, Citation1993). The clash between home languages and mediums of instruction is equally influential in determining progression rates (van Rooy & Coetzee Van Rooy, Citation2015). While some of these factors are beyond the control of HEIs, Tinto (Citation1993) has argued that academic support, especially for students from disadvantaged backgrounds, might be the key to closing the achievement gap. Tinto (Citation2017) conceptualised the link between the academic environment and student persistence using three concepts: institutional experiences, academic integration, and social integration. Ever since the notion of social integration has gained traction, universities increasingly recognise the significance of “a process in which students develop a social sense of the university environment that results from positive peer interaction, friendship formation and student participation (Tinto, Citation1993) Consequently, more student-centric learning methods are being promoted to improve student retention. One such method is peer learning, an educational approach rooted in active learning theories (Ten Cate & Durning, Citation2007)
Researchers also trace peer learning to Vygotsky’s social development theory which emphasises the importance of social interaction for learning. In Vygotsky’s view, learning is a sociogenetic process where individuals gain mastery through interacting with adults or more capable peers (Nardo, Citation2021; Vygotsky, Citation1980). He argued that learning is leveraged through the Zone of proximal development (ZPD), i.e., the distance between what an individual can do on their own and what they can do with the help of others. At the same time, the presence of a more capable peer in a learning group can catalyse long-term cognitive growth. While working on their ZPD enables individuals to internalise new knowledge (so that they do not remain reliant on the support), they can also externalise on their own in future instances (Roth & Radford, Citation2010). It has been suggested that students can identify with peer leaders given the latter’s social proximity or cognitive congruence to their experiences (Schmidt & Moust, Citation1995). This is because peer leaders “share the same knowledge framework and familiar language”, enabling them to explain concepts at a level that students can easily understand (Loda et al., Citation2020). This thinking has influenced formal peer learning approaches such as supplemental instruction, peer-assisted learning, peer-assisted study sessions and peer-led team learning which have been used globally to improve course grades (D. R. Arendale, Citation2014; Dawson et al., Citation2014). In these programmes, the role of the peer leader is pivotal in modelling both learning behaviours and course content mastery, allowing students to experiment with these until they reach mastery (D. R. Arendale, Citation2014).
While peer learning interventions have a distinct role in higher education, their effects on student performance remain unclear (Dawson et al., Citation2014), especially in South Africa. This is largely because research on peer learning interventions is scarce and mostly restricted to descriptive and advocacy accounts (Paideya & Sookrajh, Citation2014) Consequently, the evidence that supports these interventions tends to be inadequately defined. This meta-analysis investigates the effect of peer learning interventions on course performance -during the last few years (2010–2021). The findings from the meta-analysis are then contextualised, given the authors’ interest in peer learning interventions in South Africa. While peer learning interventions are not necessarily new, they are now a distinct category of student support in South Africa. To this end, a review of research studies that have adopted evidence-based designs is needed to establish whether the value ascribed to these interventions is justified and warrants the time, effort, and money invested in this form of student support.
1.1. Characteristics of peer learning
Peer learning encompasses various approaches that range from the “traditional proctor model, where senior students provide tutoring for junior students, to models in which students at the same level of study assist each other with both course content and personal concerns” (Boud, Citation2001). Ten Cate and Durning (Citation2007) identify three broad manifestations of peer learning characterised by the distance between the tutor and tutee, the group’s size and the formality of the process. With regard to distance, tutors and tutees may be at the same level of training, also called reciprocal teaching, or at different levels. One example of the latter is when postgraduate students tutor undergraduate students, which is called cross-level tutoring. The process is referred to as near-peer teaching when both the tutor and tutee are at the same level but differ by one or two years (e.g. when a final-year undergraduate student tutors students at the first-year level). The second distinction relates to the size of the group. Peer learning can happen in one-to-one groups or be applied to small groups of more than three participants. Lastly, peer learning can occur as part of a formal learning programme or informally, as peers work together to prepare for class assessments.
Different terminologies have been used in the literature, but perhaps the most well-known model is Supplemental Instruction (SI), which was developed in the United States of America in the 1970s through the work of Deanne Martin (D. R. Arendale, Citation2014; Dawson et al., Citation2014). After observing a sudden change in the student body in the USA and a concurrent sudden rise in attrition rates (D. Arendale, Citation2002), a need was identified for a programme that would enhance learning. SI focuses on high-risk courses and not ‘high-risk students; hence, no remedial stigma is attached to it. Variants of the SI model have been developed in other countries, and the most popular is Peer Assisted Study Sessions (PASS), a name that was adopted in many Australian universities as a form of SI (Dawson et al., Citation2014). Other types of peer learning include peer-led team learning (PLTL), which originated in a Chemistry Course at the City College campus in New York in the 1990s. PLTL began as the “Workshop Chemistry Project”, which drew on peer support in problem-solving for introductory chemistry courses (D. A. I. K. A. I. Gosser et al., Citation2001; D. K. Gosser, Citation2009). This programme has been supported through a grant from the American National Science Foundation, leading to its adoption in more than 100 American institutions (D. R. Arendale, Citation2014). Like SI, PLTL involves peer leaders who have successfully completed the course and are model students. Although peer assisted learning is a generic term that has been used to refer to different forms of peer learning approaches (D. R. Arendale, Citation2014), the term has been mainly used within medical education, and unlike SI, PASS and PLTL use both same level and near peers as facilitators. Nevertheless, despite differences in the origins of the different peer-learning approaches, they all share a similar description of collaborative learning (Vygotsky, Citation1980) and are believed to be somewhat homogeneous internationally regarding the distance, size of the group, and formality of the process (Dawson et al., Citation2014).
1.2. History of peer learning programmes in South Africa
Although peer learning models are not new, they started gaining attention in South Africa in the early 1990s. The impetus for this attention was the high attrition rates that scourged the country, mainly affecting black South African students (Zerger et al., Citation2006). To ameliorate the problem, the SI model was first piloted at the University of Port Elizabeth in 1993, followed by Rhodes University in 1995. This shift coincided with changes in the political front. There was a need to redress social inequalities, which had led to the systemic exclusion of the black populace under colonialism and apartheid (Badat, Citation2019) The increasing number of non-traditional students admitted to higher education forced many institutions to embark on educational reforms to improve retention and throughput. These students, most of whom were first-generation, needed support with the transition and enculturation process (Scott, Citation2017). Consequently, universities were looking for innovative ways of supporting these students.
In most cases, academic support initiatives developed organically in response to contextual needs in the different HEIs (Dhunpath & Subbaye, Citation2018). However, formal peer learning models were slow to catch up (Zerger et al., Citation2006). A review of the university websites in South Africa indicates that not many universities were offering these before 2000- although a supplemental instruction national office had been established at the National Mandela University.
In the early 2000s, scholars in academic development began to question the type and quality of support given to students. Several qualitative and conceptual studies published during this period indicate a growing concern with peer tutoring (B. J. Page et al., Citation2005; Fouche, Citation2007). However, these studies mainly provided historical accounts of peer tutoring interventions (B. J. Page et al., Citation2005), motivational studies or studies of students’ experiences (Thomen & Barnes, Citation2005) or descriptive accounts of the efficacy of these interventions. During the same period, student engagement became a buzzword in South Africa, while Vincent Tinto’s model of student persistence gained paradigmatic status in the academic development literature (Grayson, Citation2014). This led to the Council for Higher Education (CHE) inviting Tinto to a symposium in South Africa, where he offered several lectures on his theory. Ever since, peer learning and student engagement models have enjoyed a resurgence, with many universities adopting peer-mediated support policies (Cupido et al., Citation2022. This resurgence is also evidenced by the biennial conferences first hosted by Nelson Mandela University in 2015. Currently, South Africa is the only country in the world with two national centres for supplemental instruction, indicative of the increasing focus on peer learning interventions in South Africa. However, while there is a strong theoretical and epistemological base, this meta-analysis investigates whether the empirical evidence has matched this shift.
1.3. Empirical studies
As shown in Figure , more than 1645 studies have been conducted on formal peer learning programmes between 2010 and 2021, of which 63% (n = 1034) were done in the second half of the decade. Most of the studies on peer-assisted learning were conducted in medical disciplines, while supplemental instruction was more common in Engineering and peer-led team learning in Chemistry.
Figure 2. Number of research articles returned by year using the following search terms “supplemental instruction” “peer-assisted learning”, “peer-assisted study sessions” or “peer led team learning”.
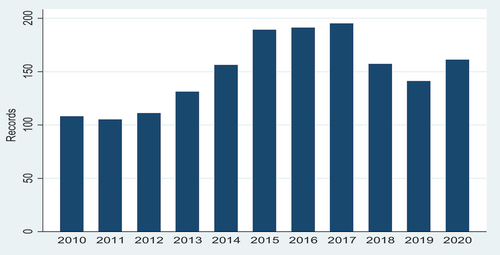
Despite the optimism surrounding peer learning models, little is known regarding their impact on academic performance, at least in South Africa. This is because studies that measure the impact of these programmes continue to be limited, and where these are done, they often lack rigour. In addition, most of this research has been criticised for being patchy and inconclusive (Dawson et al., Citation2014; Zha et al., Citation2019), with relatively few control studies that evaluate the effect of peer learning models on academic performance. To illustrate this, a recent meta-analysis of research conducted between 1993 and 2017 on peer-led learning found only 28 studies that had used quasi-experimental designs to estimate the impact of different student support interventions (Zha et al., Citation2019). Another systematic review of peer-assisted study sessions and supplemental instruction found that only 29 articles had evaluated the impact of these peer learning approaches on academic performance (Dawson et al., Citation2014). As such, there is concern about whether short-term “experiments” can adequately inform policy and practice. This is particularly important when considering the time, effort and funding invested in these programmes.
2. Methods
This study uses a meta-analytic method to examine the impact of peer learning on student performance. A meta-analysis is a statistical technique used to combine and analyse the results of multiple studies on a particular topic (Borenstein et al., Citation2009). It involves quantitatively synthesising data from different studies to estimate the overall effect size of a particular intervention or treatment using a common metric (Rosenthal, Citation1991). In addition, the results of individual studies are weighted based on sample size, and statistical techniques are used to estimate the overall effect size and the degree of variation among the studies (Borenstein et al., Citation2009; Field & Gillett, Citation2010). In this way, studies included in a meta-analysis can be compared using a similar construct (Borenstein et al., Citation2009). This helps to enhance accuracy and affords authors the opportunity to settle controversies arising from conflicting studies.
Support programmes such as peer learning are now a staple in higher education. However, a key question that many university administrators have is whether these will result in tangible benefits to both students and the institutions at large. While several metrics can be used to measure effectiveness, we focus on-course performance in this study. Our review of studies published between 2010 and 2020 is guided by the following question.
What is the effect of formal peer learning programmes on course performance?
We defined course performance as the i) final grade pass grade or mark the students received b in a particular course or ii) the difference in mean marks between the control and experimental groups. Given our interest in peer learning in South Africa, we also contextualise the emerging evidence for potential use in decision-making academic development programmes in South Africa
2.1. Study selection
We conducted a comprehensive search on different search engines such as ERIC, EBSCO, Google Scholar and Digital Dissertations. Our search terms included words such as “peer learning” OR “supplemental instruction” OR “peer-assisted learning” OR “peer learning team learning” OR “effect of peer instruction” OR “effect of supplemental instruction.” We also searched for existing systematic reviews on the topic and scrutinised the references in the resulting studies. This helped us locate some studies that we might have missed in our search using keywords.
2.2. Inclusion criteria
To be eligible for inclusion, studies had to be evaluative and focused on assessing the effect/impact of peer learning on-course performance. These studies also had to have a control or comparison group that did not receive the treatment and reported effect sizes. We also included studies written in the English language and focused on peer learning at the undergraduate level. Finally, we considered studies that were conducted between 2010 and 2021. The research studies were selected following the PRISMA statement (Page et al. Citation2021).
Using a four-stage screening process(Figure ), we initially identified 1645 studies published in English between 2010 and 2021. Two of the investigators then examined whether these articles met the agreed-upon inclusion criteria. After de-duplication, 295 records were excluded, and the remaining 1350 were then screened using the inclusion criteria. This led to the exclusion of 1275 studies i) did not focus on higher education, ii) were qualitative studies, iii) did not have an English translation, or iv) focused on irrelevant topics. We then proceeded to read the full texts and concluded that 75 of these were potentially usable. However, thirty-eight (38) of the studies did not provide adequate information to compute the effect sizes. After the screening process, 37 studies from twelve countries were retained in the analysis (Table ).
Table 1. Frequency distribution of study characteristics
2.3. Coding of studies
We designed a coding form to extract effect sizes and code features from the 37 studies. We selected five variables; publication type, sample size, length of intervention, country of origin, and discipline). To ensure accuracy, the selected studies were double-coded by two researchers. Inconsistencies in coding were reviewed until an agreement was reached. Inter-rater reliability was high in that the average percentage of agreement was 95.0%, and kappa coefficient was 0.82.
The majority (24, 64.9%) of the studies included in this meta-analysis were published between 2010 and 2015, with 81% being published in peer-reviewed journals, while 5 were dissertations. Only two studies were published in conference proceedings. Most of the studies were also in STEM disciplines (28, 75.7%). As expected, supplemental instruction (21, 56.8%) was the most common approach, followed by peer-led team learning (11, 29.7%)) and peer-assisted learning sessions (5, 13.5%). The sample sizes for these studies ranged from n = 56 to n = 11809.
Most peer-led sessions were≤60 minutes long, while SI and PLTL mainly used undergraduate near-peer tutors. PAL studies, on the other hand, used mainly matched peers. Training was compulsory for both PLTL and SI/PASS, with SI training sessions being mainly two days long, while for PLTL, it ranged from a few hours to extended training (weekly sessions) or credit-bearing courses.
Regarding the geographical distribution of the studies included in the meta-analysis, most were from North America, particularly the United States of America 25(67.6%). Australia and South Africa, which had only two studies each (5.4%), Asia (Iran, Israel and Hong Kong), and Europe (United Kingdom, Germany and Sweden) had three studies each (Figure ).
3. Analysis
3.1. Effect size calculation and heterogeneity
Effect sizes and their variances were computed within each study to allow comparability across studies. We utilised a common effect size metric, Hedges’s g statistic. A positive effect size indicates comparatively better academic performance in the intervention group than in the control group. Put differently, an effect size of 0.1 reflects 1 out of 10 improvements in the standard deviation for students in the experimental group relative to the control group. Only one effect size was reported for each study. However, when there were multiple outcome measures or subgroups within a study, composite effect size and variance were calculated for each study (Borenstein et al., Citation2009) by aggregating the effect sizes and corresponding variances.
An initial statistical assessment of the effect sizes flagged two studies (g = 2.45 and 2.02) as outliers and were removed. We fitted a random-effects model and estimated the amount of heterogeneity using the restricted maximum-likelihood estimator (Viechtbauer, Citation2005). We also report the
-test for heterogeneity (Cochran, Citation1954) and the
statistic (Higgins and Thompson Citation2002). To assess the funnel plot symmetry, we used the a regression, ‘which uses the standard error of the observed outcomes as a predictor” test (Sterne & Egger, Citation2005). The analysis was carried out using R (version 4.0.0) and the metafor package (version 2.4.0).
4. Results
We included studies in the final analysis, of which 21 focused on supplemental instruction, 11 peer-led team learning, and four (4), peer-assisted learning. Given the differences in the nature of these programmes, we present the findings for each programme individually.
4.1. Supplemental instruction
We included 21 studies on SI/PASS (Figure ), which all used quasi-experimental designs. Given their similarities, we chose to combine the analysis for these two interventions (Dawson et al., Citation2014). Out of these, 14 were from the USA, two (2) South Africa, two (2) were from Australia, while Canada, Hong Kong and Sweden had one (1) study each. The sample sizes ranged from 70 to 11,738, which was also the largest sample among all studies included in the meta-analysis. A key characteristic of the studies on SI was that all the peer leaders underwent a training programme over two days covering pedagogical, communication and facilitation skills. The peer leaders were also carefully chosen based on their excellent knowledge of the subject matter, successful completion of the SI course with an above-average grade (Malm et al., Citation2016; Terrion & Daoust, Citation2011). Successful implementation of the SI programme required a team consisting of a trained SI supervisor, the academic/instructor teaching on the course, the peer leader and the students. While the common format is to use undergraduate cross-year peer leaders, some studies showed that the peer leaders were postgraduate students (Harding et al., Citation2011) or faculty (Bryan, Citation2013).
Figure 5. Forest plot showing the observed effect sizes and the random-effects model estimates (SI/PASS).
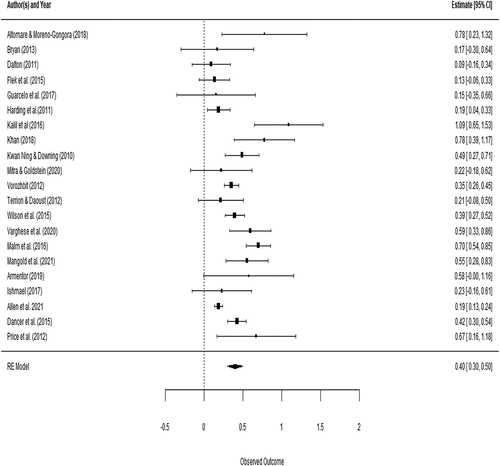
Although most of the studies were in STEM disciplines, there were also a few that focused on psychology (Guarcello et al., Citation2017), business and accounting (Kwan-Ning & Downing, Citation2010 Mangold et al., Citation2021; Mitra et al., Citation2018; Price et al., Citation2012), College reading (Dalton, Citation2011) or multiple subjects (Bryan, Citation2013). Seven studies were published between 2010 and 2015, while the remaining nine (9) were published between 2016 and 2021.
The observed mean effect sizes all positive and ranged from to
The estimated overall effect based on the random-effects model was moderate positive (
; 95% CI:
-
). The overall effect differed significantly from zero (
,
). We also estimated effect sizes for studies that reported regular attendance at SI sessions. The overall effect for studies that reported regular attendance was (
; 95% CI:
-
), while for regular attendance it was (
; 95% CI:
-
). There was also a high level of heterogeneity (Q = 94.47, p < 0.0001,
I2 = 81.97.
4.2. Peer led team learning (PLTL)
For PLTL, we included 11 studies (Figure ) which were all conducted between 2010 and 2018. All these studies were from the USA and focused on STEM subjects, particularly Chemistry and Engineering. PLTL sessions were generally run in workshop form either as a lecture replacement (Lewis, Citation2011) or as an added part of the course (Snyder et al., Citation2015; Liou-Mark, Ghosh-Dastidar, Samaroo & Villatoro, Citation2018). Thus, in all the studies, PLTL was incorporated into the course, with some studies making it compulsory or giving students a penalty for non-attendance. Like the SI model, the PLTL programmes were highly prescriptive, requiring training of peer leaders as either credit-bearing courses (Lewis, Citation2010; Mitchell et al., Citation2012) or weekly meetings (Bénéteau et al., Citation2016; Loui et al., Citation2013). The peer leaders were chosen based on having successfully completed the course and their problem-solving, communication, interpersonal and facilitation skills. The average treatment effect for PLTL was (; 95% CI:
-
) and ranged from 0.18 to 0.90. Heterogeneity statistics also revealed large variability across ES values (Q = 34.37, p < 0.0001,
I2 = 80.37.
4.3. Peer-assisted learning (PAL)
Five studies which estimated the effect of PAL on-course performance were included. All these studies were in the medical field and were conducted between 2010 and 2015 in countries such as the United Kingdom (Batchelder et al., Citation2010), Iran (Turk et al., Citation2015), USA (Hughes, Citation2011), South Korea (Han et al., Citation2015) and Germany (Cremerius et al., Citation2021). PAL sessions were all conducted by peers at the same level and taking the same course. Unlike SI and PLTL, the PAL programmes were less prescriptive in nature and did not emphasise the training of PAL leaders but rather relied on the cognitive congruency (Han et al., Citation2015) between matched or same-level peers rather than near-year peers. Given that PAL sessions were conducted during lecture time, attendance was compulsory. PAL sessions had an average positive effect on course performance (; 95% CI:
-
). There was also a high level of variability in the studies (
,
,
).
An initial assessment of the funnel plot and Egger’s regression test indicated asymmetry (results not shown). We thus used the “trim and fill method”, which imputes the missing values on one side of the funnel plot until there is symmetry around the new effect size. Both the funnel plot (in Figure ) and regression test did not indicate funnel plot asymmetry (). Therefore, there was no evidence of publication bias.
4.4. Moderator analysis
We further explored heterogeneity using study-level independent variables or moderators via meta-regression.32 Homogeneity tests were used to examine whether the variance in the true effect size was accounted for by sampling error alone or by one or more cross-study characteristics. Both Q statistics and an index were adopted to assess the extent of heterogeneity, as used for the overall effect in the earlier Section.
To explain the extra-heterogeneity in a meta-regression, we use the moderators from the data, including the year of publication, publication type, length of intervention, tutor grade, duration of the training, sample size and country (Table ). For SI/PASS. the moderator analyses yielded statistically significant effect sizes for country, length of intervention and subject (STEM). For PLTL the results were statistically significant for length of intervention and tutor grade only. The fitted model significantly reduced the residual heterogeneity ( = 0.016, p < 0.0001) and accounts for 40.7% gains in the amount of heterogeneity explained. The type of intervention (supplemental instruction vs peer-assisted;
) and (peer-led vs peer-assisted;
) is significantly related to the effectiveness of peer tutoring in improving academic performance. Since the residual heterogeneity test is still statistically significant (Q(30) = 74.56,
), it suggests that there are still unknown, uncontrolled moderators that impact the effectiveness of the intervention.
Table 2. Meta-regression of the moderating effect of type of intervention
4.5. Publication bias
Publication bias can occur when studies which have non-significant are not reported or due to location bias where studies from certain countries only are reported (Brender, Citation2006). Most of the studies reported in our meta-analysis were journal articles, followed by dissertations and then conference proceedings. Most of these studies were also from the United States of America, with all studies on PLTL coming from this country- which suggests that we have to deal with publication bias. In terms of statistical significance, 4 out 21 SI studies of the studies reported non-significant results. Only one out of eleven PLTL studies reported non-significant results, whilst 2 out of the five PAL studies had non-significant results.
To statistically assess the possibility of publication bias, we use the funnel plot (of our total sample of 37 studies, (See Figure ). An initial assessment of the funnel plot and the Egger’s regression test indicated asymmetry (results not shown). We thus used the “trim and fill method”, which imputes the missing values on one side of the funnel plot until there is symmetry around the new effect size. Both the funnel plot (in Figure ) and regression test indicated funnel plot symmetry (p = 0.7497). Therefore, there was no evidence of publication bias.
5. Discussion
This study set out to examine the effect of peer learning on-course performance. Despite the optimism surrounding peer learning in the past few years and the increase in the number of studies, very few control studies have evaluated its effect on academic performance during this past decade. For instance, our findings indicate that only 37 studies employed experimental designs or reported sufficient information which could be used to calculate effect sizes. Moreover, most of the studies were conducted in the USA, with supplemental instruction being the most studied programme.
Often, meta-analyses on peer learning have focused on individual forms of peer learning models (Zhang & Maconochie, Citation2022) or combined the different forms to get a single effect size (Zha et al., Citation2019). While both approaches have their merits, they also have limitations. For instance, although the different peer learning models share a similar description of collaborative learning, our findings revealed that they are not homogeneous regarding the distance, size of the group, and formality of the process. For example, while SI (e.g. Altomare and Moreno-Gongora (Citation2018; Guarcello et al., Citation2017) and PASS (Dancer et al., Citation2015; Price et al. (Citation2012) often use near peer leaders, most of the PAL studies (e.g. Han et al., Citation2015; Turk et al. (Citation2015) used matched peers as leaders. The group size for PAL sessions ranged from 6–12 students, while in some cases, for SI, the groups had as many as 60 students. Another difference was that while most SI and PASS leaders were trained for two days, the PLTL leaders were trained for longer periods and in some cases in a credit-bearing course. The training was not a significant component of the PAL sessions. These differences, therefore, justify the separate analyses of the different programmes.
All the 37 studies included in our metanalysis had positive effect sizes, indicating that the students exposed to peer learning had better academic outcomes relative to those exposed to the traditional lecture and/or tutorial methods. Specifically, the weighted effect size of peer-learning against no peer-learning was 0.40 for SI/PASS, 0.42 for PLTL and 0.39 PAL. These results are aligned with findings in other meta-analysis studies. For example, Zha et al. (Citation2019), found a weighted effect size 0.36 of peer-led learning on academic achievement, taking into consideration the duration and task. In Zhang and Maconochie’s study, the effect of PAL on examination performance from 13 studies published up to July 2020 was 0.38. Our analysis, therefore, supports the view that peer learning approaches, in general, positively affect student learning. They are a valuable form of collaborative learning and an alternative teaching and learning mode which can potentially enhance the student learning experiences (Dawson et al., Citation2014; Loda et al., Citation2020). Moreover, augmenting peer learning approaches with traditional teaching methods will help instructors cater for students with different learning preferences (Zhang & Maconochie ., Citation2022). Hence, although the magnitude of the effect size is considered moderate, this effect is significant enough not to be ignored.
In general, most of these studies were in STEM courses, although SI studies had some variability covering subjects such as business management (Kwan-Ning & Downing, Citation2010), psychology (Guarcello et al., Citation2017) and accounting (Mangold et al., Citation2021). The PLTL studies were predominantly Chemistry and or engineering, most likely due to the initiative originating in a Chemistry course (D. K. Gosser, Citation2009). The PAL studies covered medical related subjects such as Anatomy (Han et al., Citation2015; Cremerius et al., Citation2019) or Physiology (Hughes, Citation2011). A review of the literature on peer learning in general also reveals that peer learning models have been used mainly in STEM courses. These courses require analytical thinking (D. K. Gosser, Citation2009) and traditionally have low completion rates in most institutions (Bengesai & Paideya, Citation2018; D. R. Arendale, Citation2014; Dawson et al., Citation2014). Noting the concept of “cognitive and social congruence”, first investigated by (Schmidt & Moust, Citation1995), we, therefore, advance the proposition peer learning might be more efficacious in particular disciplines such as those in the STEM disciplines. The question that warrants further investigation is whether active learning approaches, e.g. peer learning, can benefit students across different subjects. If so, HEIs have a social justice imperative to reform undergraduate education, ensuring that the curriculum accommodates this mode of support more prominently.
Moderator analyses also indicated that the duration of intervention influenced the differences in effect sizes among the studies for both SI/PASS and PLTL. Other significant moderators for SI/PASS include country and subject, while for PLTL, it was tutor grade. Although the duration of an intervention is considered an important characteristic of student engagement (Zha et al., Citation2019), there is little empirical evidence regarding how long peer learning sessions should be. Even in the studies reviewed in this meta-analysis, no justification was given for the duration of the intervention, although some, especially the PLTL (e.g. Reisel et al., Citation2014; Snyder et al., Citation2015) studies indicated that they followed the approved recommendations for the model (D. K. Gosser, Citation2009). Despite this lack of justification for the duration of intervention, our meta-analysis findings suggest that peer learning interventions that are longer than 60 minutes are more likely to be more effective than those shorter. This is most likely because shorter sessions limit the time for protracted problem-solving, especially in STEM subjects (D. K. Gosser, Citation2009; Tan et al., Citation2022). Further, peer leaders might not only fail to attend to all students’ needs in a shorter session but also fail to keep up with the full reinforcement of the lecture material. Of course, additional studies are needed to test specifically the length of intervention based on Peer Instruction and its effect on learning.
Regarding tutor grade, the finding that peer learning sessions facilitated by senior students (postgraduate) or course instructors are more effective than those facilitated by undergraduate cross-year or matched peers is contrary to the principles guiding SI/PASS (Arendale, Citation2014) as well as PLTL (D. K. Gosser, Citation2009) which favour the later. A plausible explanation is that postgraduate students and/or instructors are more experienced in facilitation and might also have deeper content knowledge. Thus, although the common practice remains to use cross-year and matched peers owing to the social and cognitive congruence (Loda et al., Citation2020; Schmidt & Moust, Citation1995), there might be a need for institutions which offer peer learning programmes to seriously consider the level and quality of professional development and training support offered to peer leaders (Clarence, Citation2016). This is especially important considering that the training that peer leaders get is often varied, with some models such as SI and PASS offering training over two days, while for PLTL, training was done weekly. Thus, there is a possibility that, in some cases, peer leaders might be under-supported (Clarence, Citation2016).
5.1. Contextualising the research evidence
Although our primary aim was to examine the impact of peer learning models on academic performance, we also sought to contextualise the findings to South Africa for several reasons. High attrition rates continue to plague the country, and universities consistently try to develop initiatives to improve persistence (Council on Higher Education, & CHE, Citation2013; Pocock, Citation2012). Peer learning models are currently seen as solutions to the problem of unsatisfactory persistence (Bengesai & Paideya, Citation2018; Sneyers & De Witte, Citation2018). However, there has been little empirical evidence to confirm their effectiveness. While the results from independent small-scale studies can provide valuable insights, some scepticism is required when interpreting the results. For instance, the results could be a chance occurrence and not necessarily generalisable to a broader population (Borenstein et al., Citation2009). However, pooled results from multiple studies can provide a generalised view of the state of knowledge, provided we interpret this evidence acknowledging the influence of contextual factors.
There is an apparent lack of impact studies on peer learning models from Africa in general and South Africa specifically. For a country with an established academic development history (McKenna, Citation2012; Scott, Citation2017) and two SI Centres, one would have expected more research that assesses the impact of these programmes. What could explain the status quo? As Scott (Citation2017) notes: academic development initiatives such as peer-assisted learning or supplemental instruction remain peripheral to a university’s core teaching and research activities in South Africa. The marginal nature of academic development is reinforced by the fact that the scholarship of teaching and learning is underrated and not considered a legitimate area of scholarship (Leibowitz & Bozalek, Citation2016). This marginalised nature is well reflected in ad hoc staffing arrangements, with most academic support staff on short-term contracts (Paideya & Dhunpath, Citation2018). Moreover, the political and transformative agenda that influenced South Africa’s academic development resulted in an activist rather than conventional academic ethos (Scott, Citation2017). Consequently, most innovative work has not been researched or published, with much of the evidence informing academic development policy coming from institutional documents and conference proceedings (Paideya & Bengesai, Citation2017) These two factors perhaps explain the limited research on peer learning in South Africa, despite much commentary on its efficacy.
This raises the question of whether universities in South Africa should continue investing time and resources into these peer learning approaches, which continue to exist as interventions rather than core components of a transformed student-centric curriculum (Scott, Citation2017).21 Theoretically, peer learning approaches have potential in South Africa, given that they signal a shift from deficit approaches designed to address perceived “pathologies” in students through remediation to approaches that value students’ schemas (Coleman, Citation2016; Dhunpath & Vithal, Citation2012). Given the discriminatory nature of apartheid education, from which higher education is yet to recover (Scott, Citation2018) typical academic development interventions targeting at-risk students have tended to be racialised (CHE, Citation2013) – targeting the mainly Black South African students (Mayet, Citation2016). However, peer-based learning shifts the emphasis from high-risk students to identifying high-risk courses, thus removing the potential stigma attached to academic development courses.
6. Limitations
This study is not without limitations. First, we acknowledge the possibility of publication bias which has the potential to influence our findings. Although we used the funnel plot to detect publication bias, the possibility that studies with positive effect sizes are more likely to be published cannot be overlooked. Publication bias can result in the overestimation of the effect of the meta-analysis. Another second limitation relates to the search strategy. While we attempted to use various keywords related to peer learning, we might have missed some articles that might not have been indexed using our choice of keywords. Third, given that a meta-analytic method combines different studies, the pooled effect might conceal significant differences between and among studies. We acknowledge that this may potentially obscure true effects. Despite these limitations, the pooled results from our meta-analysis provide external validity of the efficacy of formal peer learning models on-course performance. We also recommend reading our findings as main effects and a quantitative summary of previous research rather than descriptions of individual studies.
7. Concluding comments
Several implications for South African higher education arise from this meta-analysis. First, the evidence from this meta-analysis suggests that peer learning interventions can improve course performance in high-risk courses. Although the effect size is moderate, it is not trivial. Many factors should be considered to determine whether an effect size should be considered large or small (Glass et al., Citation1981). For educational interventions, this would include implementation costs, time invested, feasibility, and the effect sizes from comparable interventions (Sneyers & De Witte, Citation2018) Thus, the question this meta-analysis raises for South African university administrators is whether a 39–42% (pooled results) or a 35 % improvement (based on the South African studies (Harding et al., Citation2011; Varghese et al., Citation2020) in course performance is worth the money, time and effort invested in peer learning interventions. This might require further investigations linking peer learning interventions to student retention and throughput. Such analyses will require longitudinal approaches exploring associations between peer learning and graduation outcomes. In general, longitudinal studies are limited not only in our sample but in the field as a whole, as the focus has largely been on cross-sectional explorations of immediate outcomes such as course grades.
A second implication relates to building research capacity among academic development staff involved in peer learning interventions. One strategy is to develop academic development research units that monitor and evaluate academic development interventions and support emerging practitioners. At the time of writing this article, the University of Free State had launched ELETSA, the Academic Advising Association of South Africa. It is hoped that formations such as these will contribute to the professionalisation of student support.
Thirdly, and from a theoretical perspective, our findings support the usefulness of collaborative learning (Vygotsky, Citation1980) and the notion of social congruence (Schmidt & Moust, Citation1995) in the teaching and learning environment. Thus, even in courses without formal peer learning support, course instructors can adopt some peer learning principles to optimise student learning experiences.
Disclosure statement
No potential conflict of interest was reported by the authors.
Additional information
Funding
Notes on contributors
Annah Vimbai Bengesai
Annah Vimbai Bengesai, is the Head of Teaching and Learning at the University of KwaZulu-Natal. Her research interests are in social statistics, institutional research on student access and success, and the demography of education.
Lateef Babatunde Amusa
Lateef Babatunde Amusa, is a lecturer and researcher at the University of Ilorin. His research interests are in Biostatistics, public health, and machine learning.
Rubby Dhunpath
Rubby Dhunpath is the Director of Teaching and Learning at the University of KwaZulu-Natal
References
- Allen, P. J., de Freitas, S., Marriott, R. J., Pereira, R. M., Williams, C., Cunningham, C. J., & Fletcher, D. (2021). Evaluating the effectiveness of supplemental instruction using a multivariable analytic approach. Learning and Instruction, 75, 101481. https://doi.org/10.1016/j.learninstruc.2021.101481
- Altomare, T. K., & Moreno-Gongora, A. N. (2018). The role and impact of supplemental instruction in accelerated developmental math courses. Journal of College Academic Support Programs, 1(1), 19–19.
- Arendale, D. (2002). History of supplemental instruction (SI): Mainstreaming of developmental education. In D. Lundell & J. Higbee (Eds.), Histories of developmental education Minnesota (pp.15–28). Center for Research on Developmental Education and Urban Literacy, General College, University of Minnesota.
- Arendale, D. R. (2014). Understanding the peer assisted learning model: Student study groups in challenging college courses. International Journal of Higher Education, 3(2), 1–12. https://doi.org/10.5430/ijhe.v3n2p1
- Armentor, M. M. (2019). A Quasi-experimental Study of the Effect of Supplemental Instruction on Course Completion and Persistence at a Two-year College. Unpublished Doctoral study submitted to Northcentral University.
- Badat, S. (2019). The equity-quality/development paradox and higher education transformation post-1994. In J. Reynolds, B. Fine, & R. V. Niekerk (Eds.), Race, class and the post-apartheid democratic state (p. 241). UKZN Press.
- Batchelder, A. J., Rodrigues, C. M. C., Lin, L., Hickey, P. M., Johnson, C., & Elias, J. E. (2010). The role of students as teachers: Four years’ experience of a large-scale, peer-led programme. Medical Teacher, 32(7), 547–551. https://doi.org/10.3109/0142159X.2010.490861
- Bénéteau, C., Fox, G., Xiaoying, X., Lewis, J. E., Ramachandran, K., Campbell, S., & Holcomb, J. (2016). Peer-led guided inquiry in calculus at the university of South Florida. Journal of STEM Education: Innovations & Research, 17(2), 5–13.
- Bengesai, A. V., & Paideya, V. (2018). An analysis of academic and institutional factors affecting graduation among engineering students at a South African University. African Journal of Research in Mathematics, Science and Technology Education, 22(2), 137–148. https://doi.org/10.1080/18117295.2018.1456770
- Borenstein, M., Hedges, L. V., Higgins, J. P. T., & Rothstein, H. R. (2009). Introduction to meta-analysis Chichester, U.K:. John Wiley and Sons.
- Boud, D. (2001). Making the move to peer learning. In D. Boud, R. Cohen, & J. Sampson (Eds.), Peer Learning in Higher Education: Learning from and with each other (pp. 1–20). Kogan Page (now Routledge).
- Brender, J. (2006). Framework for Meta-Assessment of Assessment Studies. In J. Brender (Ed.), Handbook of Evaluation Methods for Health Informatics (pp. 253–320). Burlington: Academic Press.
- Bryan, K. N. Assessing the Impact of Faculty-Led Supplemental Instruction on Attrition, GPA, and Graduation Rates. 2013. Unpublished Doctoral Study submitted to Walden University
- Clarence, S. (2016). Peer tutors as learning and teaching partners: A cumulative approach to building peer tutoring capacity in higher education. CriSTAL, 4(1), 39–54. https://doi.org/10.14426/cristal.v4i1.69
- Cochran, W. G. (1954). Cochran WG. The combination of estimates from different experiments. Biometrics, 10(1), 101–129. https://doi.org/10.2307/3001666
- Coleman, L. (2016). Offsetting deficit conceptualisations: Methodological considerations for higher education research Critical Studies in Teaching and Learning. Critical Studies in Teaching and Learning (CriStal), 4(1), 16–38. https://doi.org/10.14426/cristal.v4i1.59
- Council on Higher Education, & (CHE). (2013). A proposal for undergraduate curriculum reform in South Africa: The case for a flexible curriculum structure. Retrieved from Pretoria:
- Cremerius, C., Gradl Dietsch, G., Beeres, F., Link, B. -., Hitpaß, L., Nebelung, S., Horst, K., Weber, C. D., Neuerburg, C., Eschbach, D., Bliemel, C., & Knobe, M. (2021). Team-based learning for teaching musculoskeletal ultrasound skills: A prospective randomised trial. European Journal of Trauma and Emergency Surgery, 47(4), 1189–1199. https://doi.org/10.1007/s00068-019-01298-9
- Cupido, X., Frade, N., Govender, T., Pather, S., & Samkange, E. (2022). Student Peer Support Initiatives in Higher Education: A collection of South African case studies. Africa Sun Media.
- da Costa, F. J., de Souza Bispo, M., & de Cássia de Faria Pereira, R. (2018). Dropout and retention of undergraduate students in management: A study at a Brazilian Federal University. RAUSP Management Journal, 53(1), 74–85. https://doi.org/10.1016/j.rauspm.2017.12.007
- da Costa, F. J., de Souza Bispo, M., & de Cássia de Faria Pereira, R. (2018). Dropout and retention of undergraduate students in management: A study at a Brazilian Federal University. RAUSP Management Journal, 53(1), 74–85. https://doi.org/10.1016/j.rauspm.2017.12.007
- Dalton, C. (2011). The Effects of Supplemental Instruction on Pass Rates, Academic Performance, Retention and Persistence in Community College Developmental Reading Courses. Unpublished Doctoral study submitted to University of Houston
- Dancer, M. K, Tarr, G., & Tarr, G. (2015). Measuring the effects of peer learning on students’ academic achievement in first-year business statistics. Studies in Higher Education, 40(10), 1808–1828. https://doi.org/10.1080/03075079.2014.916671
- Dawson, P., van der Meer, J., Skalicky, J., & Cowley, K. (2014). On the Effectiveness of Supplemental Instruction: A Systematic Review of Supplemental Instruction and Peer-Assisted Study Sessions Literature Between 2001 and 2010. Review of Educational Research, 84(4), 609–639. https://doi.org/10.3102/0034654314540007
- Dhunpath, R., & Subbaye, R. (2018). Student success and curriculum reform in post-apartheid South Africa. International Journal of Chinese Education, 7(1), 85–106. https://doi.org/10.1163/22125868-12340091
- Dhunpath, R., & Vithal, R. (2012). Alternative access to higher education: Underprepared students or underprepared institutions. Pearson Education South Africa.
- Elena, G. (2018). Elaboration of research on student withdrawal from universities in Russia and the United States. Voprosy Obrazovaniya / Educational Studies Moscow, (1), 110–131. Retrieved from https://vo.hse.ru/en/2018–1/217490404.html. https://doi.org/10.17323/1814-9545-2018-1-110-131
- Field, A. P., & Gillett, R. (2010). How to do a meta-analysis. British Journal of Mathematical and Statistical Psychology, 63(3), 665–694. https://doi.org/10.1348/000711010X502733
- Flek, R., Cunningham, A., Baker, W., Porte, L., & Dias, O. (2015). Supplemental instruction for developmental mathematics: Early results. MathAmatyc Educator, 61, 45–51.
- Fouche, I. (2007). The Influence of Tutorials on the Improvement of Tertiary Students’ Academic Literacy. Per Linguam, 23(1). https://doi.org/10.5785/23-1-50
- Glass, G. V., McGaw, B., & Smith, M. L. (1981). Meta-analysis in social research. Sage Pub.
- Gosser, D. K. (2009). PLTL in general chemistry: Scientific learning and discovery. In N. Pienta, M. Cooper, & T. Greenbowe (Eds.), The Chemists’ Guide to Effective Teaching (Vol. 2, pp. 90–107). Prentice Hall.
- Gosser, D. A. I. K. A. I., Cracolice, M. A. I. S. A. I., Kampmeier, J. A. I. A. A. I., Roth, V. A. I., Strozak, V. A. I., & Varma-Nelson, P. (2001). Peer-Led Team Learning: A guidebook. Prentice Hall.
- Grayson, D. (2014). Vincent Tinto’s lectures: Catalysing a focus on student success in South Africa. Journal of Student Affairs in Africa, 2(2), 1–4. https://doi.org/10.14426/jsaa.v2i2.65
- Guarcello, M. A., Levine, R. A., Beemer, J., Frazee, J., Laumakis, M., & Schellenberg, S. (2017). Balancing student success: Assessing supplemental instruction through coarsened exact matching. Technology, Knowledge and Learning, 22(3), 335–352. https://doi.org/10.1007/s10758-017-9317-0
- Han, E.R, Chung, E.K, Nam, K. I., & Mihlbachler, M. C. (2015). Peer-Assisted Learning in a Gross Anatomy Dissection Course. PLoSone, 10(11), e0142988. https://doi.org/10.1371/journal.pone.0142988
- Harding, A., Engelbrecht, J., & Verwey, A. (2011). Implementing supplemental instruction for a large group in mathematics. International Journal of Mathematical Education in Science and Technology, 42(7), 847–856. https://doi.org/10.1080/0020739X.2011.608862
- Higgins, J. P. T., & Thompson, S. G. (2002). Quantifying heterogeneity in a meta-analysis. Statist. Med, 21, 1539–1558. https://doi.org/10.1002/sim.1186
- Hughes, K. (2011). Peer-Assisted Learning Strategies in Human Anatomy and Physiology. The American Biology Teacher, 73(3), 144–147. https://doi.org/10.1525/abt.2011.73.3.5
- Kalil, A., Jones, C., & Nasr, P. (2015). Application of Supplemental Instruction in an undergraduate anatomy and physiology course for allied health students. Supplemental Instruction Journal, 2(1), 33–52.
- Khan, B. R. (2018). The Effectiveness of Supplemental Instruction and Online Homework in First-Semester Calculus. Unpublished Doctoral study submitted to Columbia University
- Kwan-Ning, H., & Downing, K. (2010). The impact of supplemental instruction on learning competence and academic performance. Studies in Higher Education, 35(8), 921–939. https://doi.org/10.1080/03075070903390786
- Lavhelani, N. P., Ndebele, C., & Ravhuhali, F. (2020). Examining the efficacy of student academic support systems for ‘At Risk’ first entering students at a historically Disadvantaged South African University. Interchange, 51(2), 137–156. https://doi.org/10.1007/s10780-019-09383-z
- Leibowitz, B., & Bozalek, V. (2016). The scholarship of teaching and learning from a social justice perspective. Teaching in Higher Education, 21(2), 109–122. https://doi.org/10.1080/13562517.2015.1115971
- Lewis, E. S. (2011). Retention and reform: An evaluation of peer-led team learning. Journal of Chemical Education, 88(6), 703–707. https://doi.org/10.1021/ed100689m
- Liou-Mark, J., Ghosh Dastidar, U., Samaroo, D., & Villatoro, M. (2018). The peer-led team learning leadership program for first year minority science, technology, engineering, and mathematics students. Journal of Peer Learning, 11, 65–75.
- Loda, T., Erschens, R., Nikendei, C., Zipfel, S., & Herrmann-Werner, A. (2020). Qualitative analysis of cognitive and social congruence in peer-assisted learning – the perspectives of medical students, student tutors and lecturers. Medical Education Online, 25(1), 1801306. https://doi.org/10.1080/10872981.2020.1801306
- Loui, M. C., Robbins, B. A., Johnson, E. C., & Venkatesan, N. (2013). Assessment of peer-led team learning in an engineering course for freshmen. International Journal of Engineering Education, 29(6), 1440–1455.
- Malm, J., Bryngfors, L., & Mörner, L. (2016). The potential of supplemental instruction in engineering education: Creating additional peer-guided learning opportunities in difficult compulsory courses for first-year students. European Journal of Engineering Education, 41(5), 548–561. https://doi.org/10.1080/03043797.2015.1107872
- Mangold, N., Shima, K., & Yang, J. (2021). Long-term impact of supplemental instruction in improving student performance in intermediate accounting courses. Academy of Accounting and Financial Studies Journal, 25(3), 1–15.
- Mayet, R. (2016). Supporting at-risk learners at a comprehensive University in South Africa. Journal of Student Affairs in Africa, 4(2), 1–12. https://doi.org/10.18820/jsaa.v4i2.2
- McKenna, S. (2012). The context of access and foundation provisioning in South Africa. In R. Dhunpath & R. Vithal (Eds.), Alternative access to higher education: Underprepared students or underprepared institutions Cape Town (pp. 50–60). Pearson Education South Africa
- Merkel, J., & Brania, A. (2015). Assessment of peer-led team learning in calculus I: A five-year study. Innovative Higher Education, 40(5), 415–428. https://doi.org/10.1007/s10755-015-9322-y
- Mitchell, Y. D., Ippolito, J., & Lewis, S. E. (2012). Evaluating peer-led team learning across the two semester general chemistry sequence. Chemistry Education Research and Practice, 13(3), 378–383. https://doi.org/10.1039/C2RP20028G
- Mitra, S., Goldstein, & Goldstein, Z. (2018). Impact of supplemental instruction on business courses: A statistical study. Transactions on Education, 18(2), 89–101. https://doi.org/10.1287/ited.2017.0178
- Nardo, A. (2021). Exploring a Vygotskian Theory of Education and Its Evolutionary Foundations. Educational Theory, 71(3), 331–352. https://doi.org/10.1111/edth.12485
- Page, B. J., Loots, A., & du Toit, D. F. (2005). Perspectives on a South African tutor/mentor program: The Stellenbosch University experience. Mentoring & Tutoring: Partnership in Learning, 13(1), 5–21. https://doi.org/10.1080/13611260500039940
- Page, M. J., McKenzie, J. E., Bossuyt, P. M., Boutron, I., Hoffmann, T. C., Mulrow, C. D., & Moher, D. (2021). The PRISMA 2020 statement: An updated guideline for reporting systematic reviews. The BMJ, 372, n71. https://doi.org/10.1136/bmj.n71
- Paideya, V., & Bengesai, A. (2017). Academic support at the University of KwaZulu-Natal: A systematic review of peer-reviewed journal articles, 2010–2015. Journal of Student Affairs in Africa, 5(2), 55–74. https://doi.org/10.24085/jsaa.v5i2.2702
- Paideya, V., & Dhunpath, R. (2018). Student academic monitoring and support in higher education: A systems thinking perspective. Journal of Student Affairs in Africa, 6(1), 33–48. https://doi.org/10.24085/jsaa.v6i1.3064
- Paideya, V., & Sookrajh, S. R. (2014). Student engagement in chemistry supplemental instruction: Representations of learning spaces. South African Journal of Higher Education, 28(4), 1344–1357. https://doi.org/10.20853/28-4-398
- Pocock, J. (2012). Leaving rates and reasons for leaving in an Engineering faculty in South Africa: A case study. South African Journal of Science, 108(3/4), 8. Retrieved from https://sajs.co.za/article/view/9890
- Price, J., Lumpkin, A. G., Seemann, E. A., & Bell, D. C. (2012). Evaluating the impact of supplemental instruction on short- and long-term retention of course content. Journal of College Reading and Learning, 42(2), 8–26. https://doi.org/10.1080/10790195.2012.10850352
- Reisel, J., Jablonski, M. R., Munson, E., & Hosseini, H. (2014). Peer-led team learning in mathematics courses for freshmen engineering and computer science students. Journal of STEM Education, 15(2), 7–15.
- Rosenthal, R. (1991). Meta-analytic procedures for social research. SAGE Publications, Inc. https://doi.org/10.4135/9781412984997
- Roth, W. -M., & Radford, L. (2010). Re/Thinking the Zone of Proximal Development (Symmetrically). Mind, Culture, and Activity, 17(4), 299–307. https://doi.org/10.1080/10749031003775038
- Schmidt, H. G., & Moust, J. H. (1995). What makes a tutor effective? A structural-equations modeling approach to learning in problem-based curricula. Academic Medicine, 70(8), 708–714. https://doi.org/10.1097/00001888-199508000-00015
- Scott, I. (2017). Academic development in south african higher education. In B. E (Ed.), Higher Education in South Africa (pp. 21–49). Sun Press.
- Scott, I. (2018). Designing the South African higher education system for student success. Journal of Student Affairs in Africa, 6(1), 1–17. https://doi.org/10.24085/jsaa.v6i1.3062
- Sneyers, E., & De Witte, K. (2018). Interventions in higher education and their effect on student success: A meta-analysis. Educational Review, 70(2), 208–228. https://doi.org/10.1080/00131911.2017.1300874
- Snyder, J. J., Carter, B. E, Wiles, J. R., & Dirks, C. (2015). Implementation of the peer-led team-learning instructional model as a stopgap measure improves student achievement for students opting out of laboratory. CBE—Life Sciences Education, 14(1), ar2. https://doi.org/10.1187/cbe.13-08-0168
- Sterne, J. A. C., & Egger, M. (2005). Regression methods to detect publication and other bias in meta-analysis. In H. Rothstein, A. Sutton, & M. Borenstein (Eds.), Publication bias in meta-analysis: Prevention, assessment and adjustment (pp. 99–100). Wiley.
- Tan, A. -L., Ong, Y. S., Ng, Y. S., & Tan, J. H. J. (2022). STEM problem solving: inquiry, concepts, and reasoning. Science & Education, 32(2), 381–397. https://doi.org/10.1007/s11191-021-00310-2
- Ten Cate, O., & Durning, S. (2007). Dimensions and psychology of peer teaching in medical education. Medical Teacher, 29(6), 546–552. https://doi.org/10.1080/01421590701583816
- Terrion, J. L., & Daoust, J. -L. (2011). Assessing the impact of supplemental instruction on the retention of undergraduate students after controlling for motivation. Journal of College Student Retention: Research, Theory & Practice, 13(3), 311–327. https://doi.org/10.2190/CS.13.3.c
- Thomen, C., & Barnes, J. (2005). Assessing students’ performance in first-year university management tutorials. South African Journal of Higher Education, 19(5), 956–968. https://doi.org/10.4314/sajhe.v19i5.25538
- Tinto, V. (1993). Leaving College: Rethinking the Causes and Cures of Student Attrition (2nd ed.). The University of Chicago Press.
- Tinto, V. (2017). Through the Eyes of Students. Journal of College Student Retention: Research, Theory & Practice, 19(3), 254–269. https://doi.org/10.1177/1521025115621917
- Turk, A. S., Mousavizadeh, A., & Roozbehi, A. (2015). The effect of peer assisted learning on medical students’ learning in a limbs anatomy course. Research and Development in Medical Education, 4(2), 115–122. https://doi.org/10.15171/rdme.2015.021
- van Rooy, B., & Coetzee Van Rooy, S. (2015). The language issue and academic performance at a South African University. Southern African Linguistics and Applied Language Studies, 33(1), 31–46. https://doi.org/10.2989/16073614.2015.1012691
- Varghese, D., Varghes, B., Maharaj, A., Olaniran, A., & Chetty, N. (2020). Effect of student attendance in supplemental instruction programmes on their academic performance: A case study at the University of KwaZulu-Natal, South Africa. Technology Reports of Kansai University, 62(4), 7003–7019.
- Viechtbauer, W. (2005). Bias and efficiency of meta-analytic variance estimators in the random-effects model. Journal of Educational and Behavioral Statistics, 30(3), 261–293. https://doi.org/10.3102/10769986030003261
- Vorozhbit, M. P. (2012). Effect of Supplemental Instruction on student success. Unpublished Masters submitted toIowa State University.
- Vossensteyn, J. J., Kottmann, A., Jongbloed Benjamin, W. A, Kaiser, F., Cremonini, L., Stensaker , B., Hovdhaugen , E., & Wollscheid Sabine, S. (2015). Dropout and completion in higher education in Europe : Main report. European Union.
- Vygotsky, L. S. (1980). Mind in Society: The Development of Higher Psychological Processes. Harvard University Press.
- Wilson, C. A., Steele, A., Waggenspack, W. N., & Wang, W. (2015). Engineering Supplemental Instruction: Impact on Sophomore Level Engineering Courses. Paper presented at the 122nd ASEE Annual Conference and Exposition Seattle, Washington.
- Zerger, S., Clark-Unite, C., & Smith, L. H. S. I. S. B. F. (2006). Administration, and institutions. In M. Stone & G. Jacobs (Eds.), New visions for Supplemental Instruction (SI): SI for the 21st century (pp. 63–72). San Francisco: Jossey-Bass. (2006). How Supplemental Instruction (SI) benefits faculty, administration, and institutions. In M. Stone & G. Jacobs (Eds.), New visions for Supplemental Instruction (SI): SI for the 21st century (pp. 6372). San Francisco: Jossey6372). San Francisco: JosseyBass .
- Zha, S., Estes, M. D., & Xu, L. (2019). A meta-analysis on the effect of duration, task, and training in peer-led learning. Journal of Peer Learning, 12(2), 5–28.
- Zhang, Y., & Maconochie, M. (2022). A meta-analysis of peer-assisted learning on examination performance in clinical knowledge and skills education. BMC Medical Education, 22(1), 147. https://doi.org/10.1186/s12909-022-03183-3