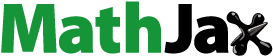
Abstract
Natural science in education can be summarized as a complex system. The professional and methodological task in the teaching of natural sciences is to convey this complexity. The terms and models of graph theory can be used to model the system approach, such as networks. Networks consist of elements and connections between them, which can be well represented by graphs. Growing networks have a scale-independent property, i.e. the rich get richer principle applies. Internal connections may also appear within the networks, which strengthen the network. New theories related to education appeared at the turn of the 19th and 20th centuries and are called reform or progressive pedagogical methods. Their common basic ideas include children’s freedom, getting to know and helping the child as an individual, the coordinating and helping role of the teacher, and the existence of the appropriate quality of the pedagogical environment.
Keywords:
1. Introduction
Teaching natural science (NS) is a serious challenge nowadays, as it is no longer possible without an understanding of system complexity, it is no longer possible, since the challenges of the 21st century require us to break away from disciplinary frameworks (Coil et al., Citation2010). Consequently, through the ability to systematize, with the help of the system approach, we must form a unified NS picture during any adequate problem management (Mizikaci, Citation2006). It is known, that in order to define a system, we must necessarily define the concept of the elements that make up the system. In this context, the element in the NS system is nothing more than the smallest independent functional unit of the system. When we examine this independent unit at the level of complexity of the entire system, we cannot break it down any further because then it loses its previously interpreted role. The constituent elements of the conceptual system of NS form mutually connected total networks. What is the fundamental property of NS as a disciplinary complexity with respect to system elements? The elements of the NS system responsible for disciplinary complexity form a set of infinite degrees of freedom at the mentioned system level, where they can be identified as an indivisible unit (Coyne et al., Citation2016). There must be some (e.g., logical) connection between the elements of the set defining the system, through which the elements in relation to the other elements show the characteristics of the system as a whole (Johnson, Citation2019). Since each element has direct or indirect connections with all other elements at the same time, neither the properties of the system nor the elements can be understood without considering the connections. According to system theory, the system depends on and is related to the condition that determines it (Johnson, Citation2019). In general, the next step in the content description of the properties of systems is to record the hierarchical structure, in which the potential divisibility of a system means that the elements themselves can be considered systems but at other system levels, e.g., as a subsystem (Johnson, Citation2019). At the same time, the given system itself (in our case, the system of complex NS) also appears as an element of another broader system (the system of sciences interpreted in earthly terms). The hierarchical structure therefore characterizes the relations and connections of the system interpreted at the examination level (Johnson, Citation2019). NS, as a large, summarizing conceptual system, is a multidisciplinary complex with a complex knowledge base covering many scientific fields, which can be well studied with a system theory approach. By identifying the relationships of the elements that make up the complex NS system, their correct interpretation, and the exploration of their cause-and-effect relationships, the characteristics of the system can be determined (Noursi et al., Citation2021). If, when using the NS system, we do not treat problems with an emphasis on complexity, it is likely that the processes and dynamisms that dominate the system will become unintelligible, without the exploration of which we will be less likely to be able to provide relevant answers to the problems that arise (complex NS education, environmental problem management, etc.). Different fields of NS are an integral part of everyday life, even if we are often not aware of this (Charlton, Citation2006). In light of all this, the question arises: how can NS subjects be taught within the framework of education divided into scientific fields in such a way that—taking into account the possibilities—students develop the systems approach to NS disciplines at the highest level that can be expected? This problem generates further questions. How is NS structured as a system? What pedagogical methods can make the development of the desired system approach effective? Can these methods also be applied during integrated teaching of NSs? With system-based education of complex natural sciences (NSs), will the challenges of the 21st century be of greater interest in the search for future solutions? Can the uniform professional needs of NS and technology be visibly combined in this way?
The purpose of this work is to explore the system-level network connections of the complex NSs in order to find answers to the above questions by following the needs of the education criteria system. Furthermore, we would like to make an attempt to recommend such organizing and principled modules that can facilitate the development of the system approach to be applied. To achieve our goals, we intend to use the network analysis toolbox with which we wish to contribute to the implementation of interdisciplinary and complex education.
NS and the methodology of reform pedagogy can be a key in the teaching of NSs, the presentation of which will be explained in the following units of this study. In order to explore the relationships, it is important to know the theory of teaching science concepts, as well as the model of experimental learning (EL).
2. Bibliography analysis
For a systematic review, we identified peer-reviewed journal articles using consistent search strategy. A bibliometric search was carried out in the databases of online literature sources were: PubMed, ResearchGate, SCOPUS, and Web of Science. The search was carried out using the following keywords: conceptualization, complex NS, EL, networks, thinking operations, and reform pedagogy. The studied literatures were fully benchmarked along the specified keywords. The method of the literature review was based on presented works (Dutta et al., Citation2023; Mondal et al., Citation2023; Palas et al., Citation2022). In this study, to focus the complex NS subject, the curriculum in the form of developing network by Barabási was mapped, in which the clusters and islands, nodes and elements of the small world that make up the system became clearly visible. The constituent parts of the network and its structure are cardinal issues from the point of view of curriculum theory and curriculum development, which can help in making the pedagogical work more transparent and also in the more effective teaching-learning process.
3. Results of literature review
The literature review is interpreted on the basis of subject classification, in such a way that the theoretical base necessary for complex NS education is established, and that the NS relationships in the curriculum can be discovered with the help of network analysis.
3.1. Experimental learning
When NSs are examined according to their complexity, a system-organizing “force” is needed that creates the opportunity to organize knowledge into a structure in such a way that it can be visualized as easily as possible on a conscious level; EL can be the starting point for this. The EL model described by Kolb may be an accepted and effective pedagogical method for making NS learning mechanisms more effective. Kolb’s EL theory is related to the constructivist point of view, according to which the construction of motives, knowledge, skills, and abilities is realized from an individual standpoint. According to Kolb, four stages are necessary for an effective learning process: (1) concrete experience, or the ability that is necessary to acquire concrete experience; (2) reflective observation skills; (3) abstract conceptualization ability; (4) the need for active experimentation and experience. The creation of an optimal learning environment and the balanced enforcement of the four conditions mentioned above are the criteria for an effective learning-teaching process. According to Kolb, the individual must first detect, map, and then interpret the knowledge to be learned, and finally fit it into the structure of the entire mental knowledge. The key role in the teaching-learning process is the ability of the individual to identify the key elements of the knowledge, to find connections and relationships. This construction is nothing more than a knowledge transfer, i.e., an implementation from one cognitive structure to another cognitive structure (Bergsteiner et al., Citation2010). Knowledge transfer takes place in vertical and horizontal directions. The vertical axis is the dimension of knowledge understanding, or the dimension of prehension (process apprehension), which are connected to concrete experiential elements or abstract conceptualization elements (or even to both). The horizontal axis is the dimension of knowledge transformation or knowledge construction. This construction element begins with observation (reflective observation) or active experimentation, which plays a key role in education with a complex approach. In Kolb’s model, apprehension (conceptual grasp) and comprehension (understanding) are independent elements of the construction of knowledge, while the extension of the element connected to attention and knowledge will become independent elements of the transformative experience. Kolb’s model will be characterized by a duality; a synthesis of an active and concrete learning situation and a passive, abstract learning situation. This duality of concrete/active/primary and abstract/passive/secondary changes dynamically in the process of learning the conceptual and knowledge categories are interchangeable, but at the same time they are also very closely connected. However, appropriate thinking skills will be essential for all these projections (Abdulwahed & Nagy, Citation2009).
3.2. Concept teaching in the natural sciences
A cardinal element of the teaching of scientific concepts is that the knowledge to be developed is made both vertically and horizontally perceivable by the students in their minds, so that a complex system of knowledge is formed that is well adapted to the laws and rules formulated by science in the given grade and in the following grades as well (Freeman et al., Citation2014).
It is important that the basic concepts, i.e. the factual material, be developed into a system of basic connections, and this must be displayed in the students’ minds. However, this requires thorough subject preparation and knowledge of the specifics of scientific concepts. The teaching of concepts has three very important factors, these are: 1. interoperability, 2. processing with a dominant aspect, 3. multilevel processing (Wieman, Citation2014). Interoperability means that the concepts to be taught are organized into a hierarchical unit in such a way that it can be interoperable both vertically and horizontally, so the given knowledge must fit into the already existing set and into the superior hierarchical system. In the case of processing with dominant aspect, an important point is that the concepts are processed on several levels, based on a dominant set of criteria, so that the name of the concept is mapped and characterized by as many signs as possible. In the case of multi-level processing, it is necessary to go from the most concrete to the most abstract concept, during the construction of knowledge. The didactics of the acquisition of concepts requires that the elementary structure of each concept be found, based on which it is necessary to construct the concept and connect it to the existing knowledge. A concept is nothing more than a mental representation of something which carries the essential properties of the given thing, so this means that a system of knowledge is formed in the minds of the students, which accurately depicts reality. Every NS concept is built from the name, i.e. the symbol denoting the item, and the sign, which is the symbol denoting the particularity of the item, i.e. none other than the interpreter.
We also need to discuss why concepts are important, that is, what functions they have. On the one hand, they have cognitive, mapping, and learning characteristics, and on the other hand, they have interactivity, i.e. communication functions (Levitt et al., Citation2022).
When we teach NS concepts, we also pay special attention to the scope of the concept, which displays the totality of objects and phenomena that apply to the content of the concept. Here, the relationships of the scope in the teaching-learning processes are formed as desired, but in accordance with the requirements. One of the essential elements of making knowledge permanent is the understanding of the content, i.e. the presentation of elementary connections regarding the content and scope of the concept. In this process, knowledge transfer has a prominent place, which means the need for the student to apply the acquired conceptual structure in general. The first element is generalization and later analogical creation, when the student searches for systematic analogies, revealing common elements and differences between concepts (Kuhn, Citation2007). The resulting concepts will be comprehensive patterns of experience that mutually influence each other. We can state that scientific concepts are general and abstract at the same time and can be connected with other general concepts, they are learned deductively, and their understanding depends on everyday concepts (which the receiver has heard before) (Kuhn, Citation2007).
3.3. Thinking in the natural sciences
Thinking can be interpreted in terms of cognitive, metacognitive, chemical, and biological processes, so it can be established that complex thinking skills are needed to process the information that reaches the individual, which are problem solving, synthesizing, analyzing, evaluating, forming relationships, etc.; these are indispensable for the acquisition of scientific knowledge. The conceptualization process initiates and directs the development of thought operations. Thinking can be a visual, concrete or abstract event, so examining scientific processes through experiments often requires the functioning of the three reference systems of the individual (Heong et al., Citation2012). Thinking is a complex process that has many operational phases and one of the basic directions of which is formal operational thinking. This means that the individual becomes capable of examining several logical operators at the same time on the level of consciousness, i.e. he becomes capable of interpreting several outputs in an optimized way along the logic of reasoning. All of this can be manifested in NS thinking in such a way that individual elements of the input variables are interpreted in the mind by the individual and then unified through synthesis. For example, the process of photosynthesis can be interpreted with these conditions, where the individual interprets the input variable environmental conditions separately in his consciousness, but a central oscillator (central logic circuit) organizes it into a unit, so he will be able to identify the output elements of photosynthesis. If we interpret this level along the cognitive development curve, then, according to Piaget, it develops in children by approximately the age of 15, and they can perform formal logical operations. The cognitive conflict appears in dimensions in which the students will necessarily be able to perform the given logical operation because very complicated abstractions appear in the NSs, which can only be understood with the help of carefully structured elements (Adey & Shayer, Citation1993).
Metacognitive abilities are also significant; this means that the individual becomes aware of the essential element by which he is able to rearrange and restructure his own thinking algorithm. Metacognition can be divided into three basic theses: first, one must have the ability to know one’s own mental processes, how one becomes aware of one’s beliefs and motivation; second, by seeing the connections between declarative and procedural epistemology; and third, it must have the ability to self-regulate in the perspective of the entire spectrum of the thinking process (Brown, Citation1987).
In this relationship, it is important to prioritize inductive and deductive thinking. The development of these types of thinking is essential in science classes, because this way students can create appropriate analogies and draw conclusions. Deductive and inductive thinking are among the general abilities of scientific competence. This means that the student encounters this skill not only in mathematics classes, during mathematics learning, but also in other subjects, and even integrated in everyday life. Basically, in inductive thinking, the basis of individual micro-operations, such as classification, analysis, synthesis, analogy, rule formation, is the extent and efficiency with which the individual recognizes the similarities and differences between the separate objects (Sanz de Acedo Lizarraga et al., Citation2009). In this context, it is also very interesting to what extent the individual will be able to restructure individual knowledge in a unique way and transfer it to another context. According to the results of research on the development of thinking, the development of both deductive and inductive thinking takes place over many years, with large individual differences. Some components of the two basic abilities reach a good level of development in some people already in preschool, whereas in the case of others, the development is much slower and they may not even develop to an adequate level by the end of secondary school (Adey & Shayer, Citation1993).
3.4. Networks as tools for teaching science concepts
Partial systems of knowledge organized through thinking become complex systems in the process of learning. If we examine the insight created by NS knowledge, we can recognize the fact that the knowledge will form conceptual networks in the mind, during which the disciplinary frameworks form an interdisciplinary framework. In this approach, network theory and graph theory can be used as methodological aids. However, first it is necessary to identify the common concepts that help the systematic mapping of NS. In Table , it can be seen that, along with the logical organizing principles, there are common elements that show the common reference relationships of NS, graph theory, and network theory.g
Table 1. Conceptual relationships in the relationship system between graph theory—network science—natural science
In any case, the system interpreted in any field is made up of system elements (e.g. constituent elements). The set of elements is made into a system by the relationships between them, systems of relationships, correlations, algorithms (e.g. functions), i.e. the various relationships.
Knowledge must be organized into a logical unit, in which the organizing principle will be provided by the individual basic operations of thinking because the individual realizes the perception of regularities through inductive or deductive thinking, through analogy formation, analysis, relationship formation, and synthesis.
Complex subnetworks are embedded in the higher-level main network, that is, how they are implemented (Avena-Koenigsberger et al., Citation2015). On the other hand, in order for the sufficiently strong main network to be built, stable subnetworks (small worlds) are needed, which can maintain their position for a long time. They form such a main network, for example, when we teach the key concepts of NSs in the knowledge system, those that can also function independently in the disciplinary system. If we examine what has been outlined in connection with the teaching of evolution, the dominant step is the prokaryotic organization, which, explained with the future endosymbiont theory, makes the eukaryotic-mitochondrium system understandable. From this, on the other hand, we can identify the adaptation to the oxidative atmosphere as an increase in the complexity of the knowledge network, where the subnetworks continue to be organized through weak connections. All of this can also be interpreted as a case of symbiosis-driven embedding of subnetworks (Bonner, Citation2019), which gains meaning in a complex way in knowledge transfer, forming a global knowledge system. Knowledge systems can turn into a dynamic and self-sustaining network, in which regulation is an important controlling factor (Kitano, Citation2002). In terms of the acquisition of complex knowledge, the central framework provided by societies’ educational management curricula will play an important role. A network can be said to be stable if, after a small disturbance of the original state, its parameters approach their original state again, i.e. the system strives to maintain the state of equilibrium. Balance is also the basic condition of social systems (Westerhoff & Palsson, Citation2004).
Concepts and their associated images (mental representations of things) are known to be connected to each other in one’s memory through associations, and it is particularly important that the connection between the condition and the resulting system operation in connection recognition is sufficiently logical. This will be especially important when teaching complex concepts. As a concrete example, we examine the concept of solar energy. To assign the appropriate knowledge to the concept, the first step is to be aware of the concept of the Sun. The social application of solar energy can be built on a basic program of consciousness that understands the operation of the Sun, the differences inherent in its structure, their description, and differentiation.
The exploratory method of learning can be the most effective, i.e. the student must associate practical elements of examples with the system of knowledge, for example, plant, function, the process of photosynthesis, the role of solar energy in water balance, etc. Comparison plays a very important role, and details also contribute to the formation of the appropriate conceptual network.
The child must be aware that there are many other stars in the Universe, but they do not play the same role in the earthly evolutionary cascade as the star of the solar system. So, we must mention two essential factors in the mechanism of concept creation: the student must be able to describe in a relevant dimension the object surface within which he must learn the new relations, and he must look for apt examples to find associations. Another important area is how the new knowledge can be connected to the old knowledge circle, since the elements of the new knowledge material can only be recalled if they activate the corresponding places in the memory. This can be interpreted in terms of a production system, so the student must form “if-then” groups. We need to summarize all the conditions that must be met if we want to perform a certain operation, this could be e.g., the classification of a concept. In the following, in accordance with our basic example, placing the emphasis on solar energy, we can make conditional formations with which the stability of knowledge becomes feasible.
Condition 1: If a celestial body has independent light, then it is a star.
Condition 2: If the star at the center of the solar system is a sun, then it radiates energy into the universe.
Condition 3: If the Sun radiates energy to the universe and to Earth as well, then its energy may be sufficient for the development of terrestrial evolution.
Condition 4: If the Sun’s energy is sufficient, photosynthesis can start in autotrophic organisms.
Condition 5: If the Sun’s energy is sufficient, then it can be used for energy production in society as well.
Condition 6: If the Sun’s energy is sufficient, it can be used not only to operate a solar thermal panel, but also to operate a solar panel.
The outlined production rules make it possible to store knowledge, namely in such a way that it can be used to draw conclusions and solve problems.
It is important to emphasize that the meaning of a concept consists of a relationship with other concepts. This is easy to see, since, e.g., the concept of solar energy shows a close connection with the use of wind energy, but also with other renewable resources. This type of energy is the driving spring. Therefore, networks of relationships are formed in the memory, and conceptual networks are realized in nodes. There can be several types of relationships, depending on the level of abstraction we want to teach the concept: class, property, and example. A common characteristic of graphs and networks is that they both contain elements (vertices), some of which are connected to each other by connections (edges) (Concas et al., Citation2022). The described initial network theory models led to the fact that, based on them, Barabási created his scale-independent network model, in which instead of the previously assumed normal distribution, the so-called power function distribution describes the structure of networks (Albert & Barabasi, Citation2000). The knowledge network can also be mapped on this principle, which is constantly expanding, e.g. through knowledge mediated by educational systems. However, the key element of this is conceptuality, on the basis of which the knowledge must be integrated into the knowledge network according to a good logical structure. An important task of network research is to model real networks (Albert & Barabasi, Citation2000; Fortunato et al., Citation2018). The production of Erdős-Rényi random networks is based on very easy-to-understand mathematical regularity. Consider the number N of elements and the probability p. A pair of elements can be selected from N elements. If this number is greater than p, then there is a connection between the two elements; if it is smaller than that, then the two elements are not connected to each other (Barabási & Czégel, Citation2021). The resulting network can explain the existence of small worlds (Albert & Barabasi, Citation2000; Fortunato et al., Citation2018). Small worlds are networks in which the average path length between two arbitrarily selected elements is short (4–6 connections), and strong group formation within the network is characteristic (Albert & Barabasi, Citation2000; Fortunato et al., Citation2018). A small world network consists of many islands of elements connected to each other by strong connections (Figure ), and the islands are connected to each other by a few weak connections (Albert & Barabasi, Citation2000; Fortunato et al., Citation2018). Such a knowledge network appears when, for example, the student learns the knowledge of zoning, because the climate zones can be described with unique characteristics, but at the same time, along a unified organizing principle, the weak connections shape them into a unit, giving the Earth’s global climate system. The teaching of the concepts can therefore also be realized through the interpretation of network theory, which can be a stronger tool for the interpretation of complexity since the key concepts become identifiable.
Figure 1. A network of small worlds - the network shown in the figure consists of three small worlds, the points of which are connected by strong connections (thicker, darker gray line). The islands formed in this way are connected by a weak (thinner, lighter gray line), forming the entire network.
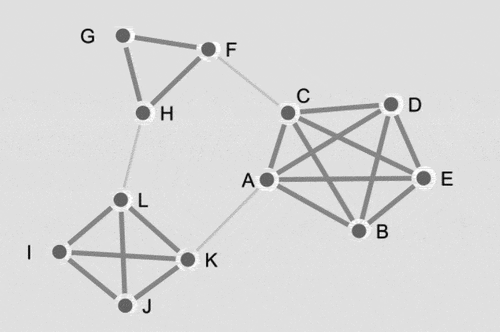
Analyzing real networks, it can be observed that only a few nodes with many connections can be found within the network. On the contrary, the number of nodes with few connections is already large (Albert & Barabasi, Citation2000). Although the Erdős-Rényi and Watts-Strogatz models described the networks as static, the model presented by Barabási speaks of their dynamic nature (Albert & Barabasi, Citation2000). This means that additional points and connections can be added to the existing network, but it can also happen that points and connections disappear along with them or independently of them. The network grows by adding up to N new connections to it at each step so that they connect a single given new element with a given number of already existing but arbitrarily chosen elements of the network. This procedure is continued until there are unconnected elements in the network (Albert & Barabasi, Citation2000).
Several regularities affect the points between which connections are made. The suitability of the point plays a decisive role in the number of connections acquired by a given point. Aptitude is the ability of the point that shows the extent to which the given point can acquire and maintain relationships. One principle, the winner-takes-all concept (Figure ), does not fit the scale-independent approach. According to this, the point with the greatest suitability obtains almost all connections (links), leaving only very few for the other points. According to the other principle, the rich get richer (Figure ), the point with the greatest aptitude has the most connections, but this point is closely followed by the other points in the number of connections. In this case, despite competition, the network maintains a scale-independent topology (Manning et al., Citation2018). Through preferential connection, larger elements grab connections from smaller elements, thus ultimately becoming nodes (Han et al., Citation2019).
Figure 2. Winner takes all principle - It can be seen that point “A” has almost all the connections.
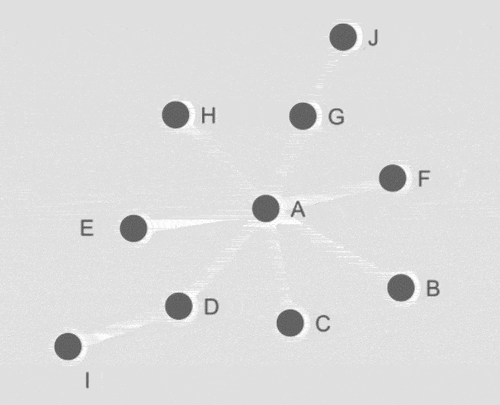
Figure 3. The rich get richer principle - It can be seen that although point “A” has the most connections (7), the other points closely followed it in the number of connections: point “B” has 5, points “D” and “K” both have 4 connection.
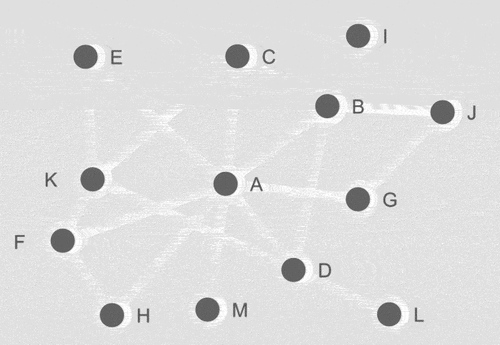
Network science as a method is an important research tool for understanding complexity. Network science focuses primarily on the structure and function of a system consisting of many elements and the connections between them (Albert & Barabasi, Citation2000). On the basis of the latter, graph theory can be a good aid for network science, since it is also built from elements (peaks) and the connections between them (edges). In fact, the components of the two methods can be matched in this way (Table ). Taking this as a basis, we can examine the problems of network science with the appropriate tools of graph theory, making use of its visualization advantages and mathematically proven procedures. We created a knowledge network based on Hungarian curriculum peculiarities, representing a complex interpretation of science subjects in elementary schools (Figure ).
It can be clearly seen that the given network can be described dominantly well by a simple connected graph. So, in such networks, if we removed any edge, i.e. connection, they would fall apart into separate parts. From the point of view of the curriculum, this fact allows us to conclude that the knowledge can be taught effectively in a linear fashion, moving from one knowledge to the next related to it, so the simple network model does not support education with a complex approach. However, if the knowledge material divided into disciplines is included in the network, it becomes self-developing, causing the appearance of complexity and even the growth of the network, in the number of both elements and connections (Figure , in which the network was designed according to the curriculum requirements of the interdependent grades). The entry of a new element is no longer necessarily accompanied by the appearance of a new relationship. The growing network now fits into the Barabási-Albert scale-independent topology. In this way, not only one, but several different paths can lead between any two elements of the network.
3.5. Pedagogical perspectives, reform pedagogy as a tool
According to the above, network analysis helps to identify complex units of NS, which can provide a toolbox for identifying the cardinal elements of the teaching process. By providing a deeper understanding of causality, the complex teaching of NS differs from traditional methods of educational organization for which certain elements of progressive pedagogy can provide a good basis. It can be considered an ancient recognition that the integration of a child into society without learning is unthinkable (Maras et al., Citation2018).
The 18th century was also called the century of pedagogy, during which the issue of education and schooling increasingly became a matter of public concern (Verstraete, Citation2022). Those who believed in the ideals of the Enlightenment believed that the education of the youth was the guarantee of a better future (Maras et al., Citation2018). This idea also fueled the emergence of reform pedagogy in the 19th and 20th centuries. The tools of reform pedagogy can be a useful starting point for complex science education. Among the philosophers of the Enlightenment was Jean-Jacques Rousseau, who, like many philosophers of his time, himself dealt with pedagogical issues (Cook, Citation2007). Rousseau’s ideas were a turning point in the development of pedagogy (Cook, Citation2007). Rousseau believes that the primary task of education is to educate a child to become a person (not a soldier, judge, etc.) (Cook, Citation2007). In Rousseau’s pedagogy, the essential element is that pedagogy is organized around the child; all pedagogical procedures must focus on the child’s characteristics (Cook, Citation2007).
The Swedish Ellen Key was the first representative and effective advocate of reform pedagogy. According to her creed, the school, as an implementer of teaching and learning, needed changes. In England, in 1889, the first new type of school was founded by the name “New School” under the direction of Cecil Reddie (Pironi, Citation2017). The first major phase of the reform pedagogy can be dated from the last decade of the 19th century to the First World War and includes thinkers such as Cecil Reddie, Adolph Ferrière, Maria Montessori or Ovide Decroly (Maras et al., Citation2018). The thinkers of the first phase emphasized the freedom and independence of the individual child and wanted to implement it in their pedagogy (Maras et al., Citation2018). They wanted to achieve all of this with institutions and tools designed for children, as well as with a significant transformation of the internal world of the school, the methods used there, and the curriculum (Maras et al., Citation2018). The second phase lasted from the end of the First World War until about the 1950s. The era was characterized by reform pedagogy becoming a global movement. Among the representatives of the nascent trends we find Wilhelm Heard Kilpatrick, Célestin Freinet, Rudolf Steiner, Helen Parkhurst and Peter Petersen. In the pedagogy of the last three, the social aspects of education also came to the fore (Maras et al., Citation2018). The third phase of reform pedagogy can be dated from the 1950s to the present day, since even today continuous innovation and reforms are needed for children to become competent adults (Kissel & Blum, Citation2021).
3.6. Pedagogical points of view, working methods at the focal point of complex natural science education
In the course of education, we can encounter many different forms of work in addition to the most basic, face-to-face education. The widely used frontal education is based on one-way communication (Alacapinar, Citation2007). The center of education is the teacher (Sanger & Osguthorpe, Citation2011), who, under his direct control, tries to keep in touch with the class as a whole with his explanations, questions, and assigned tasks (Alacapinar, Citation2007). The students seem to progress together, listen to the explanations, answer the questions addressed to them, and in the most favorable case they themselves ask the teacher questions (Alacapinar, Citation2007). The problem with this method is that, although everyone seems to be following the flow of the lesson, due to individual differences, this is probably not the case, and although all students may appear to be active, it can easily happen that the students are not actually thinking. During face-to-face education, students do individual work, but their social skills develop better in the context of other forms of work that are already known today, and other forms of work may be more beneficial during complex science education (Cho et al., Citation2003). The essence of joint forms of work is that learning takes place in the framework of some kind of cooperation between students, just as often happens in the case of problem solving in real life (Allen et al., Citation2011).
Pair work signifies the cooperation of two students. Depending on the principle and purpose of forming the pairs, we can talk about pair work (pair work) or activities performed in student pairs (Otienoh, Citation2015). In the case of the former, it is about pairs formed on occasion, in which case the most effective work can be hoped for in pairs of students of similar abilities, while in the latter, students of different abilities help each other (Otienoh, Citation2015).
Group work bases effective problem solving on the social (in addition to individual) skills that appear in this form of the learning process (Gillies, Citation2003). We can talk about group education when we temporarily form smaller groups to solve assigned tasks (Gillies, Citation2003). During group work, small groups of 3–6 people work together; the essence of the work is for students to cooperate for a common goal (Gillies, Citation2003). It can be designed based on students’ abilities by dividing them into heterogeneous or homogeneous groups (Gillies, Citation2003). Although it may seem equal in design to group work, cooperative learning is not the same (Thurston et al., Citation2010). We can talk about cooperative learning if the work process applies all of the cooperative principles at the same time, which are: 1) the presence of simultaneous social interactions, 2) interdependence, 3) the existence of individual responsibility, and 4) the equal participation of members (Thurston et al., Citation2010).
There are several types of cooperative methods, such as group mosaics, expert mosaics, group rotation, etc (Lewis, Citation2011), but it is true for all of them that all students study the same curriculum and none of the students is in a permanent tutor role (Thurston et al., Citation2010). The essence of cooperative work is that the presence of others is advantageous for each of the group members (Thurston et al., Citation2010). Another form of cooperative learning is called collaborative learning. In the course of which, the students take joint responsibility for solving the task, but everyone works on a different part of the problem, thus displaying individual responsibility as well (Lewis, Citation2011).
The project method, or project education as it is also called, could rightly have been included in the chapter presenting progressive pedagogical trends in the present work. Although the method can be traced all the way back to the work of architectural academies of the 17th century, it gained wide recognition only in the second phase of reform pedagogy, thanks to an American educator named William Heard Kilpatrick (Zhylkybay et al., Citation2014). The main principle of the concept is to use purposeful activities in the pedagogical process (Zhylkybay et al., Citation2014). Among the forms of work based on cooperation, this can be considered the most complex and time-consuming type of problem-solving, during which students work independently and acquire knowledge themselves (Zhylkybay et al., Citation2014). The aim of the method, which can be used in different types of school, is to develop children’s independence, judgment, sense of responsibility, and democratic community behavior. The focus of project education is planned, problem-oriented action. Students collaborate to solve problems that cross disciplines, by using interdisciplinary methods, the result of which is a product to be presented, while they have the opportunity to gain a wide range of experience (Zhylkybay et al., Citation2014).
4. Discussion
NS literacy in the 21st century became an essential factor for societies because the complexity of natural and social processes determines the prioritization of complex, systemic thinking as a requirement. The question arises as to how knowledge can be organized in such a way that it can form a conceptual network that is properly fixed in the individual since this is a prerequisite for the optimal consciousness structures to become anchored. NS is organized along complex organizing principles, the understanding of which can be optimized with the help of logical and planned pedagogical work (Gobert & Buckley, Citation2000). In the algorithm of knowledge acquisition, knowledge at the most abstract level must create a structure of consciousness in the individual’s mental world that perceives and interprets scientific knowledge in a unified manner. This means that the physical, chemical, biological, and geological units of the objective laws must be recognized by the individual and he must be able to identify them at a skill level. In this work, we tried to develop a thematic and necessary methodological basis, with which we can make the curriculum, knowledge and the consolidation of conceptual systems transparent. We have shown that for this, the connecting elements of each discipline must be developed into a uniform network of guidelines. In order for the acquisition of knowledge and the development of thinking skills to be realized through the interpretation of complexity, an organizing principle must be applied that allows for a space for thinking in unity, for which we used the method of network analysis. The basic units of a system are the elements, the logical and causal connection of which makes the “structural and functional blueprint” of the system easily visible. Connections require the recognition of relatedness, insight into relationships from the network creator. When we talk about systems, networks, and graphs, even though they may seem like separate areas, they still have common organizing laws (Albert & Barabasi, Citation2000). There are peaks in every network, which will be organized into a functional whole by the edges that map their connections. From the point of view of the complexity of the network, the role of weak and strong bonds will therefore be decisive. This is especially important when creating a knowledge network, i.e. knowledge is represented in such a way that the network of connections between elements is realized according to deterministic rules (causality). All of this can facilitate the individual’s understanding processes, as well as the optimal operation of their thinking abilities (inductive, deductive) through a network that can be interpreted disciplinary-wise, but still must form a NS-based knowledge content unit at the end of the teaching-learning process.
The Erdős-Rényi and Watts-Strogatz models describe networks as static, but the development of the knowledge network is dynamic and self-developing. This means that we can assign additional peaks and edges to the existing knowledge network or even remove them, since many edges hold the network together, so it can preserve its complexity. Networks of this type are scale-independent networks. When knowledge is connected in the form of networks with the help of the curricula, it becomes clear that most of the networks can be described well with a single connected graph, so the knowledge material can be best taught linearly, moving from one idea to the next. The simple network model does not allow for education with a complex approach. Networks show that the principle of the rich getting richer seems to prevail, all of which fits into the Barabási-Albert scale-independent approach.
5. Conclusions
According to the literature, there has not been much experience with the complex teaching of NSs. On the other hand, we can reasonably hope that approaching NS through its own characteristics, i.e. complexity, can be beneficial, and this study was aimed to review the toolkit for this.
Disclosure statement
No potential conflict of interest was reported by the author(s).
Additional information
Notes on contributors
Zsolt Molnár
Zsolt Molnár is one of the members of the Environmental Toxicology Research Group and leader of the Integrated Natural Science Teaching Methodology Research Group of Juhász Gyula Faculty of Education. Their research topic area is the study of the environmental system, during which the exposures or impact factors are physical, chemical, and biological in nature. The target areas of studies are also the parameters of natural science education, such as attitude, thinking operations, concept teaching in the natural sciences, which are also characterized in terms of learning and teaching conditions.
References
- Abdulwahed, M., & Nagy, Z. K. (2009). Applying Kolb’s experiential learning cycle for laboratory education. Journal of Engineering Education, 98(3), 283–14. https://doi.org/10.1002/j.2168-9830.2009.tb01025.x
- Adey, P. S., & Shayer, M. (1993). An exploration of long-term far-transfer effects fol- lowing an extended intervention programme in the high school science curriculum. Cognition and Instruction (Mahwah, NJ), 11(1), 1–29. https://doi.org/10.1207/s1532690xci1101_1
- Alacapinar, F. G. (2007). Traditional education, computer assisted education, systematic learning and achievement. Eurasian Journal of Educational Research, 7(29), 13–24. https://scholar.google.com/scholar?hl=hu&as_sdt=0%2C5&q=Alacapinar%2C+F+G.+%282007%29.+Traditional+education%2C+computer+assisted+education%2C+systematic+learning+and+achievement.+Eurasian+Journal+of+Educational+Research%2C+7%2829%29%2C+13-24.&btnG=
- Albert, R., & Barabasi, A. L. (2000). Topology of evolving networks: Local events and universality. Physical Review Letters, 85(24), 5234–5237. https://doi.org/10.1103/PhysRevLett.85.5234
- Allen, D., Donham, E., S, R., & Bernhardt, S. A. (2011). Problem-based learning. New Directions for Teaching and Learning, 128, 21–29. https://doi.org/10.1002/tl.465
- Avena-Koenigsberger, A., Goñi, J., Solé, R., & Sporns, O. (2015). Network morphospace. Journal of the Royal Society Interface, 12(103), 20140881. https://doi.org/10.1098/rsif.2014.0881
- Barabási, D. L., & Czégel, D. (2021). Constructing graphs from genetic encodings. Scientific Reports, 11(1), 13270. https://doi.org/10.1038/s41598-021-92577-2
- Bergsteiner, H., Avery, G. C., & Neumann, R. (2010). Neumann R. Kolb’s experiential learning model: Critique from a modelling perspective. Studies in Continuing Education, 32(1), 29–46. https://doi.org/10.1080/01580370903534355
- Bonner, J. T. (2019). The evolution of evolution. Journal of Experimental Zoology Part B: Molecular and Developmental Evolution, 332(8), 301–306. https://doi.org/10.1002/jez.b.22859
- Brown, A. L. (1987). Metacognition, executive control, self-regulation, and other more mysterious mechanisms. In F. E. Weinert & R. Kluwe (Eds.), Metacognition, motivation, and understanding (pp. 65–116). L. Erlbaum Associates.
- Charlton, B. G. (2006). Science as a general education: Conceptual science should constitute the compulsory core of multi-disciplinary undergraduate degrees. Medical Hypotheses, 66(3), 451–453. https://doi.org/10.1016/j.mehy.2005.12.005
- Cho, H. S., Kim, J., & Choi, D. H. (2003). Early childhood teachers’ attitude towards science teaching: A scale validation study. Educational Research Quarterly, 7(2), 33–44. https://scholar.google.com/scholar?hl=hu&as_sdt=0%2C5&q=Cho%2C+H.+S.%2C+Kim%2C+J.%2C+%26+Choi%2C+D.+H.+%282003%29.+Early+childhood+teachers’+attitude+towards+science+teaching%3A+A+scale+validation+study.+Educational+Research+Quarterly%2C+7%282%29%2C+33-44.&btnG=
- Coil, D., Wenderoth, M. P., Cunningham, M., & Dirks, C. (2010). Teaching the process of science: Faculty perceptions and an effective methodology. CBE Life Science Education, 9(4), 524–535. https://doi.org/10.1187/cbe.10-01-0005
- Concas, A., Fenu, C., Reichel, L., Rodriguez, G., & Zhang, Y. (2022). Chained structure of directed graphs with applications to social and transportation networks. Applied Network Science, 7(1), 64. https://doi.org/10.1007/s41109-022-00502-x
- Cook, A. (2007). Botanical exchanges: Jean-Jacques Rousseau and the Duchess of Portland. History of European Ideas, 33(2), 142–156. https://doi.org/10.1016/j.histeuroideas.2006.08.010
- Coyne, M. D., Cook, B. G., & Therrien, W. J. (2016). Recommendations for replication research in special education: A framework of systematic, conceptual replications. Remedial and Special Education, 37(4), 244–253. https://doi.org/10.1177/0741932516648463
- Dutta, D., Rautela, R., Gujjala, L. K. S., Kundu, D., Sharma, P., Tembhare, M., & Kumar, S. (2023). A review on recovery processes of metals from E-waste: A green perspective. Science of the Total Environment, 859(Pt 2), 160391. https://doi.org/10.1016/j.scitotenv.2022.160391
- Fortunato, S., Bergstrom, C. T., Börner, K., Evans, J. A., Helbing, D., Milojević, S., Petersen, A. M., Radicchi, F., Sinatra, R., Uzzi, B., Vespignani, A., Waltman, L., Wang, D., & Barabási, A. L. (2018). Science of science. Science: Advanced Materials and Devices, 359(6379), eaao0185. https://doi.org/10.1126/science.aao0185
- Freeman, S., Eddy, S. L., McDonough, M., Smith, M. K., Okoroafor, N., Jordt, H., & Wenderoth, M. P. (2014). Active learning increases student performance in science, engineering, and mathematics. Proceedings of the National Academy of Sciences of U S A, 111(23), 8410–8415. https://doi.org/10.1073/pnas.1319030111
- Gillies, R. M. (2003). Structuring cooperative group work in classrooms. International Journal of Educational Research, 39(1–2), 35–49. https://doi.org/10.1016/S0883-0355(03)00072-7
- Gobert, J. D., & Buckley, B. C. (2000). Introduction to model-based teaching and learning in science education. International Journal of Science Education, 22(9), 891–894. https://doi.org/10.1080/095006900416839
- Han, C., Witthaut, D., Timme, M., & Schröder, M. (2019). The winner takes it all-Competitiveness of single nodes in globalized supply networks. PLoS One, 14(11), e0225346. https://doi.org/10.1371/journal.pone.0225346
- Heong, Y. M., Yunos, J., Othman, W., Hassan, R., Kiong, T. Z., & Mohamad, M. M. (2012). The needs analysis of learning higher order thinking skills for generating ideas. Procedia - Social & Behavioral Sciences, 59, 197–203. https://doi.org/10.1016/j.sbspro.2012.09.265
- Johnson, O. (2019). General system theory and the use of process mining to improve care pathways. Studies in Health Technology and Informatics, 263, 11–22. https://doi.org/10.3233/SHTI190107
- Kissel, M., & Blum, S. D. (2021). Let’s talk teaching: Progressive pedagogy in anthropology courses. American Journal of Human Biology, 34(1), e23706. https://doi.org/10.1002/ajhb.23706
- Kitano, H. (2002). Systems biology: A brief overview. Science: Advanced Materials and Devices, 295(5560), 1662–1664. https://doi.org/10.1126/science.1069492
- Kuhn, D. (2007). Reasoning about multiple variables: Control of variables is not the only challenge. Science Education, 91(5), 710–716. https://doi.org/10.1002/sce.20214
- Levitt, H. M., Surace, F. I., Wu, M. B., Chapin, B., Hargrove, J. G., Herbitter, C., Lu, E. C., Maroney, M. R., & Hochman, A. L. (2022). The meaning of scientific objectivity and subjectivity: From the perspective of methodologists. Psychological Methods, 27(4), 589–605. https://doi.org/10.1037/met0000363
- Lewis, C. M. (2011). Is pair programming more effective than other forms of collaboration for young students? Computer Science Education, 21(2), 105–134. https://doi.org/10.1080/08993408.2011.579805
- Manning, H. G., Niosi, F., da Rocha, C. G., Bellew, A. T., O’Callaghan, C., Biswas, S., Flowers, P. F., Wiley, B. J., Holmes, J. D., Ferreira, M. S., & Boland, J. J. (2018). Emergence of winner-takes-all connectivity paths in random nanowire networks. Nature Communications, 9(1), 3219. https://doi.org/10.1038/s41467-018-05517-6
- Maras, N., Topolovcan, T., & Matijevic, M. (2018). Constructivist didactics and neurodidactics in discourse of the reform pedagogy. Theoretical premises, dilemmas and comparison. Nova Prisutnost, 16(3), 561–577. https://doi.org/10.31192/np.16.3.9
- Mizikaci, F. (2006). A systems approach to program evaluation model for quality in higher education. Quality Assurance in Education, 14(1), 37–53. https://doi.org/10.1108/09684880610643601
- Mondal, T., Choudhury, M., Kundu, D., Dutta, D., & Samanta, P. (2023). Landfill: An eclectic review on structure, reactions and remediation approach. Waste Management, 164, 127–142. https://doi.org/10.1016/j.wasman.2023.03.034
- Noursi, S., Saluja, B., & Richey, L. (2021). Using the ecological systems theory to understand black/white disparities in maternal morbidity and mortality in the United States. Journal of Racial and Ethnic Health Disparities, 8(3), 661–669. https://doi.org/10.1007/s40615-020-00825-4
- Otienoh, R. O. (2015). Implementation of pair work and group work for creation of interaction opportunities for learners in large classes: The viability of the two strategies. Journal of Education & Practice, 6(10), 171–179. https://files.eric.ed.gov/fulltext/EJ1081701.pdf
- Palas, S., Dey, S., Kundu, D., Dutta, D., Jambulkar, R., Mishra, R., Ghosh, A. R., & Kumar, S. (2022). An insight on sampling, identification, quantification and characteristics of microplastics in solid wastes. Trends in Environmental Analytical Chemistry, 36, e00181. https://doi.org/10.1016/j.teac.2022.e00181
- Pironi, T. (2017). Educating the European conscience in the correspondence between Ellen Key and Romain Rolland (1911-1924). History of Education & Children Literature, 12(1), 615–631. https://hdl.handle.net/11585/594904
- Sanger, M. N., & Osguthorpe, R. (2011). Teacher education, preservice teacher beliefs, and the moral work of teaching. Teaching and Teacher Education, 27(3), 569–578. https://doi.org/10.1016/j.tate.2010.10.011
- Sanz de Acedo Lizarraga, M. L., Sanz de Acedo Baquedano, M. T., Mangado, T. G., & Cardelle-Elawar, M. (2009). Enhancement of thinking skills: Effects of two intervention methods. Thinking Skills and Creativity, 4(1), 30–43. https://doi.org/10.1016/j.tsc.2008.12.001
- Thurston, A., Topping, K. J., Tolmie, A., Christie, D., Karagiannidou, E., & Murray, P. (2010). Cooperative learning in science: Follow up from primary to high school. International Journal of Science Education, 32(4), 501–522. https://doi.org/10.1080/09500690902721673
- Verstraete, P. (2022). The silent child: Reform pedagogy, the self and the problematization of shyness in classroom. Historia y Memoria de la Educación, 15(15), 297–321. https://doi.org/10.5944/hme.15.2022.30653
- Westerhoff, H. V., & Palsson, B. O. (2004). The evolution of molecular biology into systems biology. Nature Biotechnology, 22(10), 1249–1252. https://doi.org/10.1038/nbt1020
- Wieman, C. E. (2014). Large-scale comparison of science teaching methods sends clear message. Proceedings of the National Academy of Sciences of U S A, 111(23), 8319–8320. https://doi.org/10.1073/pnas.1407304111
- Zhylkybay, G., Magzhan, S., Suinzhanova, Z., Balaubekov, M., & Adiyeva, P. (2014). The effectiveness of using the project method in the teaching process. Procedia - Social & Behavioral Sciences, 143, 621–624. https://doi.org/10.1016/j.sbspro.2014.07.448