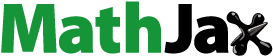
Abstract
This research investigates the abilities of undergraduate students to apply scientific reasoning in Indonesia, with a particular focus on the concept of force and motion. Forty-three first-year undergraduate students from an Indonesian private institution, comprising 20 males and 23 females, performed the Scientific Reasoning Test of Motion (SRTM) in five different patterns of scientific reasoning. The current research combines quantitative and qualitative analysis. The findings reveal that the SRTM is a valid and reliable instrument. While no significant gender-based differences were observed in Correlational Thinking (CT), differences emerged in Control of Variables (CV), Proportional Thinking (PPT), Probabilistic Thinking (PBT), and Hypothetical-Deductive Reasoning (HDR). These results indicate that PBT, HDR, and CT are applicable across gender groups, although students struggle with scientific reasoning. Specifically, the students had misconceptions regarding projectile motion and applying Newton’s second law in practical situations. These findings indicate the necessity for further developing students’ abilities to apply scientific reasoning.
REVIEWING EDITOR:
1. Introduction
Students need to learn science as it is a means of interpreting daily routines. Scientific reasoning, which encompasses the cognitive abilities needed for investigation, inference, and argumentation, has become a primary goal and crucial learning outcome in science education standards across many countries (Bolger et al., Citation2021; Omarchevska et al., Citation2022). Some scholars have proposed that developing students’ abilities for scientific reasoning is essential to academic achievement and daily life (Sutiani et al., Citation2021).
Examining the various studies conducted in this field is necessary to gain a deeper understanding of scientific reasoning. The term ‘scientific reasoning’ describes a set of abilities used in scientific practices, including ‘science inquiry,’ ‘experimentation,’ ‘evidence evaluation,’ and ‘inference.’ Zimmerman et al. (Citation2019) mentioned that scientific reasoning encompasses the cognitive abilities needed for investigation, inference, and argumentation. The reasoning is reaching conclusions based on premises and supporting evidence (Schlatter et al., Citation2021; Van Vo & Csapó, Citation2021). The conceptual framework of scientific reasoning by Engelmann et al. (Citation2016) comprises three interrelated dimensions: (a) scientific reasoning as a scientific discovery process, (b) scientific reasoning as a focus of scientific argumentation, and (c) scientific reasoning in terms of understanding the nature of science.
Many studies have explored the nature of scientific reasoning to understand and develop students’ scientific reasoning (Lawson et al., Citation2000), to comprehend the development of scientific reasoning tests (Lawson et al., Citation2000; Van Vo & Csapó, Citation2021), and to develop scientific reasoning (Choowong & Worapun, Citation2021; Erlina et al., Citation2018). Additionally, studies have been conducted on scientific reasoning development, with a focus on cognitive development and academic level (Boğar, Citation2019), gender (Piraksa et al., Citation2014; Yang, Citation2004), and student’s perceptions of the learning environment (Bezci & Sungur, Citation2021).
Further, the results of gender-based studies showed contradictory findings. Piraksa et al. (Citation2014) concluded that there was no discernible gender difference in scientific reasoning. In contrast, Sagala et al. (Citation2019) found that males and females demonstrated relatively distinct patterns of cognitive characteristics. Kersey et al. (Citation2018) examined the influence of aptitudes and personal circumstances on gender differences in scientific reasoning, and they found that men were likelier than women to complete tasks requiring scientific thinking. It aligns with the evidence by Nieminen et al. (Citation2013), which indicated that men demonstrated superior performance in creating and applying theories. Valanides (Citation1996) argued that men performed significantly better than women when employing probabilistic reasoning. The inconsistency of findings across these studies is the primary reason for the knowledge gap regarding gender differences in scientific reasoning abilities. Therefore, by addressing these gaps, we can better understand the nature and scope of gender inequalities in scientific reasoning, specifically in the context of force and motion.
For centuries, the concepts of force and motion have been fundamental to studying physics. These concepts are crucial to understanding mechanics and other challenging ideas in physics, such as electricity, heat, and waves (Tomara et al., Citation2017). Without an adequate grasp of mechanics, students cannot develop a correct understanding of force and motion law (Wancham et al., Citation2023). A lack of engagement in physics lessons, which focused on complex mathematical equations over the development of conceptual understanding, could potentially lead to misconceptions (Erlangga et al., Citation2021; Levin et al., Citation1990). A common misconception of projectile motion is that the horizontal and vertical motions are independent. Students might think that simultaneously launching an object horizontally and vertically will result in the horizontal motion remaining unaffected by the vertical movement. Indeed, the vertical and horizontal motions are interrelated (Hidayatulloh et al., Citation2021).
Learning about physics should be done through observation and experiments related to scientific reasoning. Force and motion, as a crucial concept of mechanics, can help undergraduate students in their first year effectively learn physics. Therefore, it is necessary to carry out an initial investigation of their scientific reasoning about the concept of force and motion. This research emphasised several relevant issues, including (1) assessing the instrument’s quality using Rasch analysis, (2) a description of the Rasch analysis-based gender inequalities in scientific reasoning, and (3) evaluation of students’ scientific reasoning on the concepts of force and motion. It is expected that the findings can be a resource in developing appropriate media, techniques, strategies, models, and methods so that they can develop their scientific reasoning.
2. Literature review
The Scientific Discovery as Dual Search (SDDS) paradigm represents scholars’ prevailing framework for scientific reasoning (Dunbar & Klahr, Citation2013; Zimmerman, Citation2000). The SDDS conceptualises scientific reasoning as a problem-solving process involving developing and revising hypotheses within two distinct spaces: the hypothesis space and the experiment space. Subsequent studies concerned more specific aspects of scientific reasoning, including integrating diverse causal variables (Kuhn & Pearsall, Citation2000). Moreover, recent developments in scientific reasoning have highlighted the importance of epistemic cognition, or beliefs about knowledge and its development, and the cognition that emerges from these beliefs (Reith & Nehring, Citation2020). Argumentation is critical in this field (Fischer et al., Citation2018).
This research concerns scientific reasoning, the most widely employed topic in science education research. Lawson (Citation2004), who defined scientific reasoning as creating causal inferences for a phenomenon that cannot be directly observable by using mental rules, plans, or tactics, was the initiator. This concept focuses on the mental processes that enable one to extrapolate unobservable mechanisms from observable facts (Lawson, Citation2004, Citation2010). Five subsets of skills, including hypothetical-deductive reasoning, control of variables, proportional thinking, correlational thinking, and probabilistic thinking, are identified as essential elements of Lawson’s conceptualisation of scientific reasoning (Lawson, Citation2004; Zimmerman, Citation2000). These competencies represent various processes frequently essential for successful scientific inquiry (Lazonder & Egberink, Citation2014). Established tests in psychometric and educational research typically measure and assess scientific reasoning. These include Lawson’s Classroom Test of Scientific Reasoning [LCTSR], The Test of Logical Thinking [TOLT], and Group Assessment of Logical Thinking [GALT]). However, few studies have used self-developed instruments, despite the potential benefits of such approaches (Dolan & Grady, Citation2010; Luo et al., Citation2020).
In order to gain a deeper comprehension of scientific reasoning in undergraduate students, it is imperative that we take into account two important theoretical stances: information processing theory and cognitive constructivism theory. As per Jean Piaget’s theory of cognitive development, children go through discrete stages of mental development that are characterized by changes in the thinking processes. This theory offers a framework for comprehending the ways in which people learn and create their worldview. According to Piaget’s thesis, an undergraduate student’s capacity for scientific reasoning is influenced by their cognitive development stage, which in turn affects their capacity for problem-solving and scientific inquiry. Lawson (Citation1985) found that undergraduates in the formal operational stage are able to understand complex scientific concepts and exhibit more abstract thinking than those in the concrete operational stage, which is consistent with Piaget’s theory. In their scientific reasoning, concrete operational stage students typically rely on empirical evidence and observable phenomena. Furthermore, research conducted by Lourenço (Citation2016) revealed that undergraduate students are generally at the formal operational stage, which is defined by hypothetical reasoning and abstract thought. This stance explains how students use reasoning, utilize abstract concepts, and model argumentation to approach scientific thinking at this level.
Furthermore, highlighting the cognitive processes involved in learning, Robert Gagné’s Information Processing Theory concentrates on how people encode, store, and retrieve information. Undergraduate students’ capacity for scientific thinking is impacted by their information processing and manipulation skills, according to Gagné’s notion. According to research by Winne (Citation2013), comprehension and application of scientific concepts are more proficient among undergraduate students who possess strong cognitive processing abilities, such as rehearsal, elaboration, and attentiveness. Gagné’s theory also highlights the importance of practice and feedback in the learning process, implying that students’ capacity for scientific reasoning can be improved via repeated exposure to scientific challenges and constructive criticism (Hasson et al., Citation2015). Avargil et al. (Citation2018) have conducted studies which emphasize the significance of metacognitive techniques, including planning, monitoring, and assessing, in scientific thinking for undergraduate students. Gagné’s Information Processing Theory thus have the potential to inform science teachers’ creation of instructional tactics that maximize students’ cognitive processes and foster more potent scientific reasoning abilities.
Several factors must be considered when evaluating scientific reasoning. Firstly, it is essential to acknowledge the gender difference as a variable. Secondly, more studies are required to clarify the contradictory findings of previous studies on gender differences in scientific reasoning, thinking, or intelligence quotient (Luo et al., Citation2021; Pezzuti et al., Citation2020). For instance, studies by Luo et al. (Citation2021), Nieminen et al. (Citation2013) and Yang (Citation2004) found distinguished scientific reasoning skills between men and women. In contrast, studies by Piraksa et al. (Citation2014) and Waschl and Burns (Citation2020) found no significant difference. A study by Piraksa et al. (Citation2014) stated that the gender variable had no discernible impact on students’ scientific reasoning across all LCTSR sub-dimensions. On the contrary, men performed better than women in formulating and making judgments (Yang, Citation2004). Consequently, further investigation from multiple perspectives is essential to ascertain the underlying causes of these disparate outcomes.
3. Method
3.1 Research design and procedure
The mixed-methods approach integrates quantitative and qualitative approaches, prioritising quantitative over qualitative analysis. The combined results assist students in comprehending scientific reasoning in greater depth. The first stage of this research was to administer the Scientific Reasoning Test of Motion (SRTM) to a group of students. After collecting the test data, Rasch analysis was carried out to assess several factors: dimensionality and fit, item and individual reliability, independence, and interpretation of the Wright map of scientific reasoning. The researchers then examined the written test, which expressed the students’ ideas and thoughts, to comprehend the students’ reasoning in detail. After analysing the responses, a discrepancy was found between the student’s answers and their scientific reasoning. In the end, the researchers combined and discussed the quantitative and qualitative findings to formulate conclusions and offered suggestions based on the information gathered from administering the SRTM and using Rasch analysis to analyse the results. All phases in this research are shown in .
3.2 Participants
The participants were 43 first-year students (aged 18–19) enrolled in introductory physics courses at a private institution in Sidoarjo, Indonesia. gives a detailed overview of the demographic characteristics of the sample.
Table 1. The participants’ demographic characteristics.
3.3 Instrument
The Scientific Reasoning Test of Motion (SRTM) is the instrument in this research. The SRTM consists of 20 items. There are ten questions on two levels. It has a follow-up question to measure the student’s scientific understanding of the process. The concepts measured by the instrument were Proportional Thinking (PPT) (items 1 and 2), Control of Variables (CV) (items 3 and 4), Probabilistic Thinking (PBT) (items 5 and 6), Correlational Thinking (CT) (items 7 and 8), and Hypothetical-Deductive Reasoning (HDR) (items 9 and 10). Three experts examined this SRTM instrument. All the experts have taught physics courses in higher education for the last five years. The evaluation criteria include the relevance and appropriateness of the items to prompt the student’s abilities of scientific reasoning on the topic of force and motion and clarity of items to the population of test-takers, such as these first-year students of STEM majors who were taking introductory physics courses. The results show that SRTM is feasible and has a percentage of agreement (R) of 83%.
Each item in the SRTM has four major components. The first element is a stimulus, which can be data, images, phenomena, or an explanation of a concept. The second is a question to prompt scientific reasoning. The third is a choice of four or five statements from which to choose as replies to prompt scientific reasoning. The last is a blank space where students can express their reasons for choosing those answers. See for an example of a scientific reasoning test used in this research.
3.4 The scientific reasoning abilities’ domains
Patterns of scientific reasoning are PPT, CV, PBT, CT and HDR. The following examples show how to conceptualise the PPT. The process entails choosing two significant extensive variables related to a problem, identifying a constant rate for intensive variables, and using provided information and relationships to either discover an additional value for one extensive variable or to compare two values of the intensive variable computed from the data as a comparison problem. CV is a method of regulating the dependent and independent variables that affect the continuity of the circumstances while testing the hypothesis. PBT refers to a situation in which one is interested in the number of times a specific procedure is carried out, leading to a particular outcome when repeated several times under the same conditions. Predictions in scientific research are made possible by CT, which refers to people’s cognitive patterns for assessing the degree of mutual or reciprocal links between variables. HDR is the quality of reasoning that organises and generates answers to problems at all stages of life.
3.5 Data analysis
The SRTM was administered to the students in the physics course to assess their proficiency in employing scientific reasoning. Assess the SRTM’s psychometric performance using Rasch analysis. Rasch analysis is used to guide the creation of rating scale instruments due to multiple causes. The justification for using Rasch analysis is that the data is ordinal and cannot be assumed to be distributed linearly. It is also employed for various additional purposes, including its ability to assess the instrument’s effectiveness in various ways (e.g., evaluating instrument performance using a Wright Map). The Winsteps computer application is used further to analyse the data (Linacre, Citation2012).
This research assessed the SRTM instrument’s quality by employing Rasch analysis to examine (a) dimensionality and fit and (b) item and person reliability. displays the criteria for unidimensionality values. provides the categories for the person value, item reliability, and Cronbach’s alpha value.
Table 2. Category of unidimensionality value.
Table 3. Values category of item and person reliability, and Cronbach’s alpha.
Following that, a Wright map analysis is to ascertain whether there are any gender-based distinctions in scientific reasoning. The Wright map is a logit scale representing the items and the person’s reliability. The map’s right side shows the distribution of the SRTM item difficulty levels. Conversely, the left side shows the distribution of student proficiency levels, providing each indicator’s scientific reasoning for student performanc.
The final analysis entails a qualitative description of the student’s reasoning in the concepts of force and motion. It also facilitates an investigation of the disparity between students’ reasoning and scientific reasoning regarding open-ended results. For example, students will receive an explanation of scientific concepts when they provide answers and reasons for incorrect concepts. Each indicator will be described using scientific reasoning.
4. Results
4.1 The quality of SRTM
4.1.1. Dimensionality and fit
Each question in the instrument assesses a specific concept to analyse the fit and dimensionality of the SRTM, thereby enhancing researchers’ confidence. Also, it analyses the MNSQ Outfit and Infit and the ZSTD (Outfit Z-Standar) statistics. O’Connor et al. (Citation2016) employed a range of MNSQ from 0.5 to 1.5 and ZSTD from −2.0 to +2.0, and the results showed that all items fit (see ). Moreover, the unidimensionality value is used to calculate the empirical raw variance, elucidated by procedures for calculating the validity value. The results show that the 33.6% of the raw variance explained by the measures may be attributed to unidimensionality. Further, the SRTM demonstrates a high level of validity when classified according to .
Table 4. Rasch item statistics for each SRTM construct.
4.1.2. Item and person reliability
Additionally, Rasch’s approaches evaluate the item, subject, and Cronbach’s alpha reliability values. The person and item reliability value indicates the degree of consistency in students’ answers and the quality of the SRTM items (Sumintono & Widhiarso, Citation2014). On the other hand, Cronbach’s alpha evaluates the reliability and depicts the connection between a person and an item. The SRTM instrument had an item reliability of 0.91, a person reliability of 0.57, and a Cronbach’s alpha of 0.57. Regarding the criteria in , it can be inferred that the item reliability of the SRTM was very good, whereas the personal reliability was weak. It means that the consistency of the students’ answers was poor, although the SRTM items were of high quality.
of the Cronbach’s alpha value indicates that the interaction between person and items in the SRTM is within the acceptable range. The main factor contributing to the SRTM’s weak reliability is the different backgrounds of the first-year physics students from various senior high schools. Several students attended public senior high schools, while others were from private schools that offered customised instruction. Awofala and Lawani (Citation2020) stated that different instruction may strongly affect a student’s foundation of scientific knowledge and reasoning skills.
4.1.3. Wright Map interpretation
The Wright Map (or the item-person map) visually depicts Rasch’s analysis results. It is to depict the connection between the items on a test and the ability level of the test-takers. displays a map where the left represents the students’ abilities, while the right represents the item difficulty. Iramaneerat et al. (Citation2008) determined that higher logits are indicative of students with more excellent abilities (on the left side) and items that are more challenging (on the right side). We can enable the variable map to determine whether the items align with the student’s ability.
Figure 3. A Wright map for the Scientific Reasoning Test of Motion (SRTM) data collected from first-year Student University students attending physics courses.
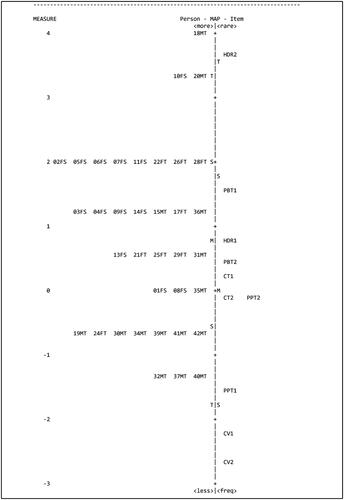
The test items are assigned an average of 0 logits (Iramaneerat et al., Citation2008). Based on the variable map, most students score below the mean of the test items. Only a few students with more remarkable aptitude are assigned a value of +3.69 logits, while a few less capable students are assigned a value of −1.33 logits. These logits’ values are determined based on the highest and lowest metrics. Thus, 23 out of 34 students, equivalent to 67.65% of the student population, possess adequate scientific reasoning abilities, as they can answer the questions and find the required items.
shows that the HDR 2 indicator, with a logit scale of +3.69, is the most challenging item for students. It is followed by the PBT 1, HDR 1, PBT 2, and CT 1 indicators, which have logit scales of +1.60, +0.81, +0.50, and +0.18, respectively. In comparison, students find CV 2 the simplest or the most well-understood, with a logit scale of –2.69. Following CV 2, the indicators of CV 1, PPT 1, CT 2, and PPT 2 are relatively easier. It means that PPT and CV are the most effective indicators of a student’s proficiency in scientific reasoning. The scientific reasoning concepts students struggle with are HDR, PBT, and CT.
4.2 Rasch analysis-based gender inequalities in the scientific reasoning
The results of analysing the scientific reasoning of participant’s genders for each indicator are displayed in . shows no difference between genders in the CT1, CT2, PPT2, and PBT2 indicators. In other words, male and female students demonstrate equal levels of scientific reasoning. Male and female students have different abilities in scientific reasoning as measured by the indices CV1, CV2, PPT1, HDR1, PBT1, and HDR2, respectively. Male students perform at a higher level of proficiency in PPT1, PBT1, and HDR2 indicators than female students, as seen by a higher DIF measure. This indicates that males possess greater aptitude for completing these tasks than females. However, female students outperform male students on the CV1, CV2, and HDR1 indicators because they have higher DIF measurements, which gives them an advantage when answering questions requiring scientific reasoning.
4.3 Evaluation of each scientific reasoning indicators
A qualitative analysis of the scientific reasoning used in each indicator is to ascertain how students’ answers align with the existing scientific concepts. The SRTM items are organised based on scientific reasoning indicators. Specifically, items 1 and 2 are used to measure PPT, items 3 and 4 are for CV, items 5 and 6 are for PBT, items 7 and 8 are for CT, and items 9 and 10 are for HDR.
4.3.1. Proportional thinking (PPT)
displays the findings of the student’s scientific reasoning patterns on the proportional reasoning indicator, with the items PPT1 and PPT2 receiving logit scales of −1.57 and −0.15, respectively. The results show that PPT 1 and PPT2 are more understandable than other indicators of scientific reasoning. PPT is a reasoning pattern that assesses students’ aptitude for establishing connections between variables using data, equations, or figures. The first and second items question about uniformly accelerated motion. Students must select the appropriate graph for the second item based on the provided ticker timer data. The results show that both male and female students can interpret the ticker timer data, demonstrating that the distance between points is progressively increasing and that objects are undergoing uniformly accelerated motion. However, a few students have struggled to determine the exact graph representing uniformly accelerated motion. displays an example of a student’s answer.
4.3.2. Control of variables (CV)
The third and fourth items (see and ) are questions about analysing the correct experimental design for investigating the impact of the track board’s slope on the travel time objects and its effect on the applied force on the object’s average speed. These items assessed students’ aptitude in identifying experimental variables. The results showed that students accurately discerned the relationship between manipulation and response variables. It can be seen that students are aware that the track board’s slope affects the duration of the car’s trip, although their reasoning may not align with scientific concepts, and they may have misconceptions. In , the students demonstrated a lack of understanding that the experimental design was intended to validate Newton’s Second Law, which postulates that a physical object’s acceleration rate is proportional to the net force acting upon it and inversely proportional to its mass. As more force is applied to an item, its acceleration rises. This phenomenon can be expressed by the equation which describes the object’s motion, not
In other words, students have not been able to apply Newton’s second law in a real-world context.
4.3.3. Probabilistic thinking (PBT)
Probabilistic thinking (PBT) is a student’s skill in predicting the opportunities generated when repetition occurs (Erlina et al., Citation2018; Ludwin-Peery et al., Citation2020). The fifth and sixth items measure students’ ability to predict the position of objects that experience free fall motion and the direction of a ball’s motion when its string breaks after being rotated vertically. shows that PBT 1 and PBT 2 have logit scales of +1.60 and +0.50, indicating that these two items were challenging for students to complete. A sample of a student’s response to item number 5, which assesses the PBT 1 indicator, is shown in . The findings reveal that students could correctly estimate the items’ positions in free fall motion. Students were able to use the free-fall equations of motion correctly but were unable to implement these equations into non-routine problems. Nasir et al. (Citation2021) contend that undergraduate students poorly master non-routine problem-solving skills in their first and last years.
4.3.4. Correlational thinking (CT)
Correlational thinking is the student’s aptitude for creating mutual relationships between variables (Bao et al., Citation2022). The seventh and eighth items measure students’ ability to determine the interrelationships of variables in projectile and free fall motion. shows that the logit scale values acquired to see both male and female students typically find working on the CT 2 (Free Fall) questions simpler than the CT 1 (Projectile) topics. The possible reason is that students master the concept of free fall more than the concept of projectiles. In item CT 2, students determine the relationship between the time variable and the object’s weight in free fall. The finding demonstrated that the students already knew that all moving objects in free fall would experience the same gravitational acceleration, namely g = 9.8 m/s2. In the CT1 item, students are asked to choose variables related to the initial velocity in the horizontal direction (vox), and students tend to answer that there are no influencing variables (see ). Few students can determine the variables that affect the initial velocity of objects in projectile motion. Female students can memorise projectile equations of motion but have not been able to implement these equations into non-routine problems. According to Lazonder and Harmsen (Citation2016), guidance is necessary for inquiry learning to assist students in solving non-routine questions.
4.3.5. Hypothetical-deductive reasoning (HDR)
Hypothetical-deductive reasoning (HDR) is students’ ability to formulate hypotheses from general concepts to specific ones. The ninth and tenth questions test students’ ability to plan experiments to ascertain the variables influencing an object’s travel time in a free-falling motion (HDR1) and the factors influencing an object’s horizontal fall in a parabolic motion (HDR2). Based on the logit scale that was acquired, HDR2 has the most extraordinary logit scale (+3.69), indicating that the item that students find to be the most difficult, followed by HDR 1 with a logit scale (+0.81), shows that the item that students find to be the easiest.
displays a sample of a student’s response to question number 10 (HDR2). Their responses showed that students still have misconceptions regarding the concept of projectile motion, where they have not been able to compile a hypothesis about the relationship between the height of an object (h2) and the horizontal distance travelled by an object. Students had a hard time grasping the idea of parabolic motion. It is consistent with other research that explains how students frequently have misconceptions regarding projectile motion (Erlangga et al., Citation2021; Hidayatulloh et al., Citation2021; Levin et al., Citation1990).
5. Discussion
This research provides a framework for analysing students’ scientific reasoning, particularly at the undergraduate level, and how gender affects reasoning patterns. shows that SRTM exhibits promising psychometric features regarding the Rasch analysis. The SRTM items, which assess students’ reasoning aptitudes in force and motion, generally perform as expected. The SRTM instrument also has the requisite dimensionality, as evidenced by the fit analysis, which indicates that each item assesses a unifying construct. This finding supports the SRTM’s reliability as a measurement tool for scientific reasoning. Researchers can confidently use this instrument in similar investigations or interventions to assess the mentioned abilities. It also has implications for future studies.
Two outcomes of the Rasch analysis presented in depict how gender influences scientific reasoning patterns. First, gender does not influence correlational reasoning (CT) patterns. However, there are differences between male and female proficiencies in scientific reasoning, especially in specific markers such as control variables (CV), probabilistic reasoning (PBT), proportional reasoning (PPT), and hypothetical-deductive reasoning (HDR). These results align with those studies which confirmed that there are gender differences in scientific reasoning ability, such as Sagala et al. (Citation2019), Kersey et al. (Citation2018), and Nieminen et al. (Citation2013).
Analysing each scientific reasoning indicator also provides essential insights into students’ knowledge and misconceptions regarding various scientific reasoning concepts. The results of Wright’s map analysis () indicate that the logit scales for PPT 1 and PPT 2 are −1.57 and −0.15, respectively. These results demonstrate that students readily comprehend the proportional thinking indicators. Students have grasped the concept of accelerated motion with constant acceleration when associated with motion material. Students can interpret the data on the ticker timer in an appropriate graphical form. It is because of the often use of multiplicative relationships in their everyday lives. The ability to comprehend relationships between quantities and their proportions is a hallmark of the concrete operational stage, which is when Piaget claims that proportional thinking usually manifests. This is reinforced by Gagné’s hypothesis, which highlights the importance of cognitive functions including rehearsal, elaboration, and attention in achieving proficiency on tasks involving proportional reasoning. According to Misnasanti et al. (Citation2017), proportional reasoning ability involves the relationship between multiplication and the ability to apply concrete operations in a formal context, which can be developed using students’ existing experiences. portrays a discrepancy in performance between male and female students on the PPT 1 indicator, with male students exhibiting a more excellent proficiency than their female counterparts. It was discovered that males were more adept than females at developing and applying theories (Nieminen et al., Citation2013).
In , the logit scales for CV 1 and CV 2 are −2.22 and −2.69, respectively. These figures indicate that students have mastered the control variables indicators. The CV is the ability to construct an adequate experimental design, identify appropriate variables, interpret valid data, and understand the many components’ role in the experimental system (Lazonder & Egberink, Citation2014; Van Vo & Csapó, Citation2021). According to Piaget’s theory, people may conduct systematic experiments and comprehend cause-and-effect linkages when they have the ability to manipulate variables, which appears during the formal operational stage. According to Gagné’s theory, mastery of control over variables requires both practice and feedback. Moreover, indicates that female students are better at identifying experimental variables than male students, representing a significant difference in scientific reasoning ability in the lens of CV2 and CV 1 indicators. These results are similar to Tairab’s (Citation2015) findings, which demonstrated that more female than male students could provide correct answers and reasoning to the sub-idea “selecting appropriate experimental settings.”
The Wright map analysis result in shows that the logit scale for PBT 1 and PBT 2 are 1.60 and 0.50, respectively. This result demonstrates that the problems encountered by the students in solving PBT1 indicators are complex. PBT 1 problems pertain to students’ understanding of free fall motion. Students are assigned to predict the position of an object in free fall at the second. Nevertheless, students tend to rely solely on mathematical calculations to determine distance, with less emphasis on the position. As indicated by Piaget’s theory, students might still be improving their probabilistic reasoning skills, particularly in difficult situations like figuring out where an object will fall in free fall. This validates Piaget’s theory that adolescents continue to develop their capacity for abstract reasoning throughout adolescence.
It is evident that male students exhibited a higher DIF in the performance of the PBT1 indicators than their female counterparts (read ). It indicates that males are more proficient in these tasks than females. This research’s outcomes are pertinent to the study by Sari et al. (Citation2017), who posited that male students exhibited multi-structural probabilistic thinking in completing probability tasks, whereas female students demonstrated unstructured probabilistic thinking. Similarly, Valanides (Citation1996) emphasised that males outperformed females in probabilistic reasoning. However, both male and female students have equal abilities to solve question 6 or the PBT 2 indicator.
The logit scale for CT 1 and CT 2 in indicates that students still had difficulties solving problems related to CT1, which requires them to determine the quantities regarding the initial velocity in the horizontal direction in projectile motion. According to Hidayatulloh et al. (Citation2021), students hold numerous misconceptions about projectile motion in the context of graphic and visual representations. In order to effectively address correlation-related challenges, students may need to acquire cognitive processes including problem-solving and decision-making, as defined by Gagné’s Information Processing Theory. On the one hand, there is no discernible difference between male and female students in CT 1 and CT 2, as illustrated in . On the other hand, Yenilmez et al. (Citation2005) found that females scored higher on controlling variables and correlational reasoning. Hence, further qualitative research is required to ascertain the potential causes of these findings.
In addition, the student population encountered significant HDR difficulties. This finding aligns with research by Erlina et al. (Citation2018) and Novia and Riandi (Citation2017), which found that students experienced considerable difficulty with HDR. In considering the need to construct and test hypotheses, Piaget’s theory indicates students may still be developing their capacity for abstract and organized thought. The HDR1 and HDR2 indicators demonstrated significant gender-based differences. Male students performed a higher DIF measure for the HDR2 indicator than their female counterparts, suggesting that males may possess a more excellent aptitude for completing this task than females. Conversely, female students performed better than male students on the HDR1 indicator, conferring them a competitive advantage in addressing problems requiring scientific reasoning. The findings of Radulović and Stojanović (Citation2019) also showed no statistically significant correlation between gender and HDR. Future research is recommended to elucidate the underlying causes of these discrepancies and investigate strategies to foster scientific reasoning skills equally among male and female students.
6. Conclusion
A Rasch analysis indicates that the SRTM questions, which assess students’ reasoning abilities in force and motion, exhibit favourable psychometric features. Each item effectively assesses a single underlying construct and demonstrates high levels of reliability with minimal dependency. Findings of a Wright map uncover that HDR, PBT, and CT are indicators of scientific reasoning that present significant challenges for students, whereas PPT and CV are more readily accessible. The results of the Rasch analysis showed differences in scientific reasoning between male and female students on the CV, PPT, HDR, and PBT indicators. However, no significant differences were observed for the CT indicators. Regarding the qualitative analysis, students’ answers showed misconceptions about projectile motion and difficulties in applying Newton’s second law to practical issues. It highlights the necessity for a learning strategy to improve students’ understanding of motion and force concepts.
It must be acknowledged that this research has significant limitations. Due to the limited sample size and the inadequate analytical strategy, the results may not represent the larger population. Rasch analysis can provide helpful insights in some situations, but it may restrict the depth of understanding of the data. It is known for its ease of use and capacity to transform ordinal data into interval-level measurements. However, it has the potential to oversimplify the intricacies of the underlying components under investigation, potentially missing subtle patterns or interactions. Therefore, it is recommended that future research use a bigger and more varied sample size and investigate complementary analytical techniques to provide a more comprehensive and reliable analysis.
Disclosure statement
No potential conflict of interest was reported by the author(s).
Additional information
Notes on contributors
Noly Shofiyah
Noly Shofiyah is a PhD student in the natural science education study program at Universitas Negeri Surabaya (Unesa). Her main research interest is 21st century skills such as scientific literacy, collaboration skills, and scientific reasoning, as well as innovative learning such as inquiry, PjBL, and STEM.
Nadi Suprapto
Nadi Suprapto is a Professor at the Departement of Physics, Faculty of Mathematics and Natural Science, Universitas Negeri Surabaya (Unesa). His research interests in the physics literacy, physics education, 21st Century teaching and learning, assessment, cultural studies, curriculum, and philosophy of science.
Binar Kurnia Prahani
Binar Kurnia Prahani is a Associate Profesor at the Departement of Physics, Faculty of Mathematics and Natural Science, Universitas Negeri Surabaya (Unesa). His research interests in the physics literacy, physics education, 21st Century teaching and learning, computer science, and Critical thinking.
Budi Jatmiko
Budi Jatmiko is a Professor at the Departement of Physics, Faculty of Mathematics and Natural Science, Universitas Negeri Surabaya (Unesa). His research interests in the physics literacy, physics education, 21st Century teaching and learning, computer science, and thinking skills.
Desak Made Anggraeni
Desak Made Anggraeni is a PhD student in the natural science education study program at Universitas Negeri Surabaya (Unesa). Her main research interest is Education, Thinking skill, e-learning, Technology in education
Khoirun Nisa’
Khoirun Nisa’ is is a student at the Departement of Physics, Faculty of Mathematics and Natural Science, Universitas Negeri Surabaya (Unesa). Her research interests in assessment, physics education, and cultural studies.
References
- Avargil, S., Lavi, R., & Dori, Y. J. (2018). Students’ metacognition and metacognitive strategies in science education. In Y. J. Dori, Z. R. Mevarech, & D. R. Baker (Eds.), Cognition, metacognition, and culture in STEM education: Learning, teaching and assessment (pp. 33–64). Springer. https://doi.org/10.1007/978-3-319-66659-4_3
- Awofala, A. O. A., & Lawani, A. O. (2020). Increasing mathematics achievement of senior secondary school students through differentiated instruction. Journal of Educational Sciences, 4(1), 1–19. https://doi.org/10.31258/jes.4.1.p.1-19
- Bao, L., Koenig, K., Xiao, Y., Fritchman, J., Zhou, S., & Chen, C. (2022). Theoretical model and quantitative assessment of scientific thinking and reasoning. Physical Review Physics Education Research, 18(1), 010115. https://doi.org/10.1103/PhysRevPhysEducRes.18.010115
- Bezci, F., & Sungur, S. (2021). How is middle school students’ scientific reasoning ability associated with gender and learning environment? Science Education International, 32(2), 96–106. https://doi.org/10.33828/sei.v32.i2.2
- Boğar, Y. (2019). Evaluation of the scientific reasoning skills of 7th grade students in science course. Universal Journal of Educational Research, 7(6), 1430–1441. https://doi.org/10.13189/ujer.2019.070610
- Bolger, M. S., Osness, J. B., Gouvea, J. S., & Cooper, A. C. (2021). Supporting scientific practice through model-based inquiry: A students’-eye view of grappling with data, uncertainty, and community in a laboratory experience. CBE—Life Sciences Education, 20(4), ar57. https://doi.org/10.1187/cbe.21-05-0128
- Choowong, K., & Worapun, W. (2021). The development of scientific reasoning ability on the concept of light and image of grade 9 students by using inquiry-based learning 5E with prediction observation and explanation strategy. Journal of Education and Learning, 10(5), 152–162. https://doi.org/10.5539/jel.v10n5p152
- Dolan, E., & Grady, J. (2010). Recognizing students’ scientific reasoning: A tool for categorizing complexity of reasoning during teaching by inquiry. Journal of Science Teacher Education, 21(1), 31–55. https://doi.org/10.1007/s10972-009-9154-7
- Dunbar, K., & Klahr, D. (2013). Developmental differences in scientific discovery processes. In J. St. B. T. Evans, & K. Frankish (Eds.), In two minds: Dual processes and beyond (pp. 337–356). Routledge. https://doi.org/10.4324/9780203761618-9
- Engelmann, K., Neuhaus, B. J., & Fischer, F. (2016). Fostering scientific reasoning in education – Meta-analytic evidence from intervention studies. Educational Research and Evaluation, 22(5–6), 333–349. https://doi.org/10.1080/13803611.2016.1240089
- Erlangga, S. Y., Winingsih, P. H., & Saputro, H. (2021). Identification of student misconceptions using four-tier diagnostic instruments on straight motion materials. Compton: Jurnal Ilmiah Pendidikan Fisika, 8(2), 65–67. https://jurnal.ustjogja.ac.id/index.php/COMPTON/article/view/12923 https://doi.org/10.30738/cjipf.v8i2.12923
- Erlina, N., Susantini, E., Wasis, W., Wicaksono, I., & Pandiangan, P. (2018). Evidence-based reasoning in inquiry-based physics teaching to increase students’ scientific. Journal of Baltic Science Education, 17(6), 972–985. https://doi.org/10.33225/jbse/18.17.972
- Fischer, F., Chinn, C. A., Engelmann, K., & Osborne, J. (2018). Scientific reasoning and argumentation: The roles of domain-specific and domain-general knowledge. In K. S. Taber, & B. Akpan (Eds.), Science education (pp. 89–104). Springer. https://doi.org/10.1007/978-3-319-58685-4_6
- Hasson, U., Chen, J., & Honey, C. J. (2015). Hierarchical process memory: Memory as an integral component of information processing. Trends in Cognitive Sciences, 19(6), 304–313. https://doi.org/10.1016/j.tics.2015.04.006
- Hidayatulloh, W., Kuswanto, H., Santoso, P. H., Susilowati, E., & Hidayatullah, Z. (2021). Exploring students’ misconception in the frame of graphic and figural representation on projectile motion regarding the COVID-19 constraints. JIPF (Jurnal Ilmu Pendidikan Fisika), 6(3), 243–254. https://doi.org/10.26737/jipf.v6i3.2157
- Iramaneerat, C., Smith, E. V., Jr, & Smith, R. M. (2008). An introduction to Rasch measurement. In J. W. Osborne (Ed.), Best practices in quantitative methods (pp. 50–71). Sage.
- Kersey, A. J., Braham, E. J., Csumitta, K. D., Libertus, M. E., & Cantlon, J. F. (2018). No intrinsic gender differences in children’s earliest numerical abilities. Npj Science of Learning, 3(1), 12. https://doi.org/10.1038/s41539-018-0028-7
- Kuhn, D., & Pearsall, S. (2000). Developmental origins of scientific thinking. Journal of Cognition and Development, 1(1), 113–129. https://doi.org/10.1207/S15327647JCD0101N_11
- Lawson, A. E. (1985). A review of research on formal reasoning and science teaching. Journal of Research in Science Teaching, 22(7), 569–617. https://doi.org/10.1002/tea.3660220702
- Lawson, A. E. (2004). The nature and development of scientific reasoning: A synthetic view. International Journal of Science and Mathematics Education, 2(3), 307–338. https://doi.org/10.1007/s10763-004-3224-2
- Lawson, A. E. (2010). Basic inferences of scientific reasoning, argumentation, and discovery. Science Education, 94(2), 336–364. https://doi.org/10.1002/sce.20357
- Lawson, A. E., Clark, B., Cramer-Meldrum, E., Falconer, K. A., Sequist, J. M., & Kwon, Y. J. (2000). Development of scientific reasoning in college biology: Do two levels of general hypothesis-testing skills exist? Journal of Research in Science Teaching, 37(1), 81–101. https://doi.org/10.1002/(SICI)1098-2736(200001)37:1<81::AID-TEA6>3.0.CO;2-I
- Lazonder, A. W., & Egberink, A. (2014). Children’s acquisition and use of the control-of-variables strategy: Effects of explicit and implicit instructional guidance. Instructional Science, 42(2), 291–304. https://doi.org/10.1007/s11251-013-9284-3
- Lazonder, A. W., & Harmsen, R. (2016). Meta-analysis of inquiry-based learning: Effects of guidance. Review of Educational Research, 86(3), 681–718. https://doi.org/10.3102/0034654315627366
- Levin, I., Siegler, R. S., & Druyan, S. (1990). Misconceptions about motion: Development and training effects. Child Development, 61(5), 1544–1557. https://doi.org/10.1111/j.1467-8624.1990.tb02882.x
- Linacre, J. M. (2012). Winsteps® Rasch measurement computer program user’s guide. Winsteps.com.
- Lourenço, O. M. (2016). Developmental stages, Piagetian stages in particular: A critical review. New Ideas in Psychology, 40, 123–137. https://doi.org/10.1016/j.newideapsych.2015.08.002
- Ludwin-Peery, E., Bramley, N. R., Davis, E., & Gureckis, T. M. (2020). Broken physics: A conjunction-fallacy effect in intuitive physical reasoning. Psychological Science, 31(12), 1602–1611. https://doi.org/10.1177/0956797620957610
- Luo, M., Sun, D., Zhu, L., & Yang, Y. (2021). Evaluating scientific reasoning ability: Student performance and the interaction effects between grade level, gender, and academic achievement level. Thinking Skills and Creativity, 41, 100899. https://www.sciencedirect.com/science/article/pii/S1871187121001140 https://doi.org/10.1016/j.tsc.2021.100899
- Luo, M., Wang, Z., Sun, D., Wan, Z. H., & Zhu, L. (2020). Evaluating scientific reasoning ability: The design and validation of an assessment with a focus on reasoning and the use of evidence. Journal of Baltic Science Education, 19(2), 261–275. https://doi.org/10.33225/jbse/20.19.261
- Misnasanti, M., Utami, R. W., & Suwanto, F. R. (2017 Problem based learning to improve proportional reasoning of students in mathematics learning [Paper presentation]. In AIP Conference Proceedings, August (Vol. 1868). AIP Publishing.
- Nasir, N. A. M., Singh, P., Narayanan, G., Han, C. T., Rasid, N. S., & Hoon, T. S. (2021). An analysis of undergraduate students’ ability in solving non-routine problems. Review of International Geographical Education Online, 11(4), 861–872. https://doi.org/10.33403/rigeo.8006800
- Nieminen, P., Savinainen, A., & Viiri, J. (2013). Gender differences in learning of the concept of force, representational consistency, and scientific reasoning. International Journal of Science and Mathematics Education, 11(5), 1137–1156. https://doi.org/10.1007/s10763-012-9363-y
- Novia, N., & Riandi, R. (2017). The analysis of students’ scientific reasoning ability in solving the modified Lawson classroom test of scientific reasoning (MLCTSR) problems by applying the levels of inquiry. Jurnal Pendidikan IPA Indonesia, 6(1), 116–122. https://doi.org/10.15294/jpii.v6i1.9600
- O’Connor, J., Penney, D., Alfrey, L., Phillipson, S., Phillipson, S., & Jeanes, R. (2016). The development of the stereotypical attitudes in HPE scale. Australian Journal of Teacher Education, 41(7), 70–87. https://doi.org/10.14221/ajte.2016v41n7.5
- Omarchevska, Y., Lachner, A., Richter, J., & Scheiter, K. (2022). Do video modeling and metacognitive prompts improve self-regulated scientific inquiry? Educational Psychology Review, 34(2), 1025–1061. https://doi.org/10.1007/s10648-021-09652-3
- Pezzuti, L., Tommasi, M., Saggino, A., Dawe, J., & Lauriola, M. (2020). Gender differences and measurement bias in the assessment of adult intelligence: Evidence from the Italian WAIS-IV and WAIS-R standardizations. Intelligence, 79, 101436. https://doi.org/10.1016/j.intell.2020.101436
- Piraksa, C., Srisawasdi, N., & Koul, R. (2014). Effect of gender on student’s scientific reasoning ability: A case study in Thailand. Procedia - Social and Behavioral Sciences, 116, 486–491. https://doi.org/10.1016/j.sbspro.2014.01.245
- Radulović, B., & Stojanović, M. (2019). Research and evaluating of hypothetically-deductive student reasoning in Republic Serbia. Facta Universitatis, Series: Physics, Chemistry and Technology, 16(3), 249–256.
- Reith, M., & Nehring, A. (2020). Scientific reasoning and views on the nature of scientific inquiry: Testing a new framework to understand and model epistemic cognition in science. International Journal of Science Education, 42(16), 2716–2741. https://doi.org/10.1080/09500693.2020.1834168
- Sagala, R., Umam, R., Thahir, A., Saregar, A., & Wardani, I. (2019). The effectiveness of STEM-based on gender differences: The impact of physics concept understanding. European Journal of Educational Research, 8 (3), 753–761. https://doi.org/10.12973/eu-jer.8.3.753
- Sari, D. I., Budayasa, I. K., & Juniati, D. (2017). The analysis of probability task completion; taxonomy of probabilistic thinking-based across gender in elementary school students [Paper presentation]. In AIP Conference Proceedings, August (Vol. 1868). AIP Publishing.
- Schlatter, E., Molenaar, I., & Lazonder, A. W. (2021). Learning scientific reasoning: A latent transition analysis. Learning and Individual Differences, 92, 102043. https://doi.org/10.1016/j.lindif.2021.102043
- Sumintono, B., & Widhiarso, W. (2014). Aplikasi model Rasch untuk penelitian ilmu-ilmu sosial (edisi revisi). Trim Komunikata Publishing House.
- Sutiani, A., Situmorang, M., & Silalahi, A. (2021). Implementation of an inquiry learning model with science literacy to improve student critical thinking skills. International Journal of Instruction, 14(2), 117–138. https://www.scopus.com/inward/record.uri?eid=2-s2.0-85101769206&partnerID=40&md5=a023ff175c9e8e4e83e9400b010b7cbc https://doi.org/10.29333/iji.2021.1428a
- Tairab, H. H. (2015). Assessing students’ understanding of control of variables across three grade levels and gender. International Education Studies, 9(1), 44–54. https://doi.org/10.5539/ies.v9n1p44
- Tomara, M., Tselfes, V., & Gouscos, D. (2017). Instructional strategies to promote conceptual change about force and motion: A review of the literature. Themes in Science & Technology Education, 10(1), 1–16.
- Valanides, N. C. (1996). Formal reasoning and science. In P. Davies (Ed.), Ethnographies of reason (pp. 99–107). Routledge. https://doi.org/10.4324/9781315580555-2
- Van Vo, D., & Csapó, B. (2021). Development of scientific reasoning test measuring control of variables strategy in physics for high school students: Evidence of validity and latent predictors of item difficulty. International Journal of Science Education, 43(13), 2185–2205. https://doi.org/10.1080/09500693.2021.1957515
- Wancham, K., Tangdhanakanond, K., & Kanjanawasee, S. (2023). Sex and grade issues in influencing misconceptions about force and laws of motion: An application of cognitively diagnostic assessment. International Journal of Instruction, 16(2), 437–456. https://doi.org/10.29333/iji.2023.16224a
- Waschl, N., & Burns, N. R. (2020). Sex differences in inductive reasoning: A research synthesis using meta-analytic techniques. Personality and Individual Differences, 164, 109959. https://doi.org/10.1016/j.paid.2020.109959
- Winne, P. H. (2013). Self-regulated learning viewed from models of information processing. In B. J. Zimmerman & D. H. Schunk (Eds.), Self-regulated learning and academic achievement (pp. 145–178). Routledge.
- Yang, F. Y. (2004). Exploring high school students’ use of theory and evidence in an everyday context: The role of scientific thinking in environmental science decision-making. International Journal of Science Education, 26(11), 1345–1364. https://doi.org/10.1080/0950069042000205404
- Yenilmez, A., Sungur, S., & Tekkaya, C. (2005). Investigating students’ logical thinking abilities: The effects of gender and grade level. Hacettepe Üniversitesi Eğitim Fakültesi Dergisi, 28, 219–225.
- Zimmerman, C. (2000). The development of scientific reasoning skills. Developmental Review, 20(1), 99–149. https://doi.org/10.1006/drev.1999.0497
- Zimmerman, C., Olsho, A., Brahmia, S. W., Loverude, M., Boudreaux, A., & Smith, T. (2019 Towards understanding and characterizing expert covariational reasoning in physics [Paper presentation]. In Physics Education Research Conference Proceedings (pp. 693–698). https://doi.org/10.1119/perc.2019.pr.Zimmerman