Abstract
Insights from psychology and cognitive science have, as yet, barely entered hospital choice research. This conceptual article closes this gap by reviewing and conceptually framing the current literature on hospital choice and patient information behavior and by discussing which tools are needed to advance scientific methodology in the study of patient decision-making strategies in hospital choice. Specifically, we make a call for more experimental research in hospital choice in order to complement existing theories, methods, and tools. This article introduces computerized process-tracing tools in hospital choice research, and also outlines a hands-on example, to provide a basis for future research.
Public Interest Statement
Patients often have to select a hospital for elective surgery or other medical treatment, and this choice is not an easy decision. As laymen, patients often use decision strategies that support simplification of the decision process. By analyzing what is already known about patient decision-making strategies in the scientific literature, this study summarizes the important decision strategies, usually effort reduction processes, which patients apply when choosing a hospital. Importantly, the scientific tools to investigate these behavioral schemes in experimental settings are lacking. Therefore, we propose to use experimental research tools, such as computerized process-tracing, to investigate patients’ decision strategies. To facilitate future experimental research, this study also outlines a hand-on example.
Competing interests
The authors declare no competing interests.
1. Introduction
The topic of hospital choice has received significant academic attention in health care marketing and related research fields for more than 30 years, and its study has long been dominated by traditional economic theories and methodology (e.g. Akinci, Healey, Tengilimoglu, & Parsons, Citation2004). However, during the past decade, it has been increasingly argued that a more cognitive perspective should complement the traditional economic view, thereby facilitating insight into patients’ hospital choice (e.g. Frank, Citation2004). Specifically, knowledge from psychology and cognitive science may serve as a vital basis for a better understanding of hospital choice preferences and underlying cognitive mechanisms. Patients’ decision-making strategies in hospital choice are largely unknown today, and hence, little is known about how people acquire and use information in hospital choice. Moreover, the determinants of the application of specific decision-making strategies in hospital choice settings are not well understood either (Damman, Spreeuwenberg, Rademakers, & Hendriks, Citation2012). Gaining deeper understanding of patients’ decision-making strategies, however, could challenge existing theoretical frameworks, question existing assumptions in health care politics, reform marketing strategies of health care providers, and also improve patients’ autonomy for optimal decision outcomes (Victoor, Delnoij, Friele, & Rademakers, Citation2012).
Against the background of this increasing importance of psychology and cognitive science in the research field of hospital choice, the present article has the goal to review and integrate insights from health care management, marketing science, and cognitive psychology in order to advance hospital choice research on both a theoretical and methodological basis. Specifically, we address three major research questions:
(1) | Which theoretical framework can be used to advance hospital choice research? | ||||
(2) | What do we know about patient decision-making, especially regarding patient information behavior and relevance of decision criteria, in hospital choice? | ||||
(3) | Which methodological tools are needed to advance the scientific understanding of patients’ decision-making strategies in hospital choice? |
Answers to these questions are given by reviewing and conceptually framing the current literature on hospital choice and patient information behavior. Based on review, we make a call for more experimental research in the field of hospital choice. Analyses of the current literature revealed that many studies are purely conceptual in nature, and those studies that are empirical usually used surveys and interviews. It follows that experimental research deliberately manipulating independent variables (e.g. amount of information or decision complexity) to study the resulting effects on dependent variables (e.g. information acquisition patterns or decision-making strategies) is rare. Rather, research based on self-reported data dominates. While this existing stream of research has contributed to scientific progress in the field, a new perspective is advantageous to stimulate future research and to foster knowledge development in the field of hospital choice.
Specifically, we outline the potential of computerized process-tracing (CPT) tools for the study of patient decision-making strategies in hospital choice. Such software tools, to the best of our knowledge, have not been used in hospital choice research so far, despite their well-known importance in the investigation of people’s decision-making strategies in experimental settings, a fact that explains their enormous relevance in cognitive psychology and behavioral decision-making (Schulte-Mecklenbeck, Kühberger, & Ranyard, Citation2011a). Hence, we argue that these tools should become part of the researcher’s toolbox in the field of hospital choice.
This paper is structured as follows. In Section 2, we describe the theoretical background to experimentally study patient decision-making strategies by introducing the adaptive decision-making framework by Payne, Bettman, and Johnson (Citation1993), and illustrate how it applies to the hospital choice context. In Section 3, we provide a literature review on patient information behavior and decision criteria for hospital choice. In Section 4, we describe foundations of CPT tools, and we outline how such tools can be used to study patients’ decision-making behavior in hospital choice scenarios in the context of laboratory experiments. Subsequently, we analyze existing CPT tools. Moreover, as a complement to this section, we provide a concrete research example in the Appendices A and B, based on an extensive review of decision attributes relevant in hospital choice. Finally, in Section 5, we conclude the paper.
2. Theoretical background
2.1. Adaptive decision-making as theoretical foundation for the application of experimental research in hospital choice
Modern cognitive psychology acknowledges that human resources such as time, knowledge, and computational power are limited. Following bounded rationality theory, therefore, patients have only limited information processing capabilities. Thus, they are generally not able to process all information available and cannot act with perfect rationality in decision-making situations, in the sense that their choice is based on an optimal combination of probability and utility evaluations (Simon, Citation1959, Citation1990). Following Simon’s (Citation1959) satisficing approach, individuals rather settle for making a choice that is good enough to meet their predefined aspiration level. Thus, patients typically apply choice heuristics, defined as “strategies that guide information search and modify problem representations to facilitate solutions” (Goldstein & Gigerenzer, Citation2002, p. 75), to cope with difficult decisions, especially under uncertainty (Kahneman, Slovic, & Tversky, Citation1974). Generally, this “heuristics approach” explains that people do not always base their decisions on probability evaluations; rather, people have a variety of intuitive strategies, such as cognitive shortcuts, for solving decision-making problems (Griffin, Gonzalez, & Varey, Citation2001; Kahneman et al., Citation1974). Among these heuristics are, for example, the fast and frugal heuristic (Gigerenzer & Goldstein, Citation1996; Goldstein & Gigerenzer, Citation2002), and the adaptive decision-making model (Payne et al., Citation1993). Those heuristics stress the adaptive and goal-driven nature of judgment and decision processes (Griffin et al., Citation2001).
As the aim of this research is to elucidate which distinctive patient decision-making strategies occur within the hospital choice context, in this article we draw upon the adaptive decision-making model developed by Payne et al. (Citation1993). Within this seminal framework, people are described as actors who flexibly adjust their information processing and decision effort as a function of the complexity of information and the context within which decisions are made (Bettman, Johnson, & Payne, Citation1991). Thus, decision strategy selection is predominantly influenced by characteristics of the decision problem (e.g. complexity), characteristics of the decision-maker (e.g. experience), and social context variables (e.g. need to justify a decision).
Within this framework, decision strategy is defined as a “sequence of mental and effector (actions on the environment) operations used to transform an initial state of knowledge into a final goal state of knowledge where the decision-maker views the particular decision problem as solved” (Payne et al., Citation1993, p. 9). Decision strategies, therefore, include the sub-processes of information acquisition, evaluation, and choice (Payne et al., Citation1993). Patients have a repertoire of heuristics available in their set of decision strategies, which they assess on their advantages (or benefits) and disadvantages (or costs) in light of their individual goals and constraints. However, according to the adaptive decision-making model, individuals will always use the heuristic anticipated as “best” for the choice task with regard to a maximum of accuracy and a minimum of effort as they intelligently trade off accuracy and effort evaluations when making a decision (Payne, Bettman, Coupey, & Johnson, Citation1992; Payne et al., Citation1993). Besides these two meta-goals (accuracy and effort), recent evidence has shown that decision strategy selection is further influenced by people’s tendency towards minimization of negative emotions and maximization of justifiability (Bettman, Luce, & Payne, Citation2012). Importantly, this theoretical approach of heuristic decision-making has been previously transferred to medical decision-making (Marewski & Gigerenzer, Citation2012), substantiating the notion that this framework, generally, is useful in the field of health care. Moreover, the adaptive decision-making model is the conceptual basis of several algorithms underlying CPT tools (see Section 4).
2.2. Applying the adaptive decision-making model to the hospital choice context
According to the adaptive decision-making model, scholars need to consider several determinants of strategy selection before empirically investigating hospital choice scenarios. Important determinants of decision strategy selection in the hospital choice context are: the decision problem, the decision-maker, and the social context in which the patient is embedded (see Figure ). Only by understanding these factors, human decision behavior can be interpreted (Bettman et al., Citation1991; Payne et al., Citation1993).
First, the decision problem must be understood. A decision problem is mainly characterized by task variables (e.g. time pressure, the number of alternatives, or the plethora of information describing those alternatives) and context variables (e.g. similarity of alternatives, reference points, and framing) (Payne et al., Citation1993; Pfeiffer et al., Citation2014). Like many other health-related choice problems, the selection of a hospital represents a highly complex decision task due to the interaction of multiple situational variables (Lubalin & Harris-Kojetin, Citation1999; Pierce, Citation1996; Pierce & Hicks, Citation2001; Victoor et al., Citation2012). Complexity in decision-making is essentially determined by the number of alternatives available (Payne, Citation1976). However, the number of accessible health care providers for patients may vary depending on the health care system and other factors (e.g. geographic aspects). Nevertheless, many consumer-driven health care systems exist in provision-oriented countries where patients enjoy comparatively smooth access to providers, and where universal health service coverage exists (Wendt, Citation2009). Moreover, decision complexity may stem from a high number of relevant attributes (i.e. decision criteria) (Payne et al., Citation1993). Here, extant research on hospital choice has examined a multitude of attributes, all of which may have impact on patients’ hospital selection (Victoor et al., Citation2012). However, a comprehensive overview is missing in the literature, and hence we provide a corresponding discussion in Section 2.4 where we describe a list of decision criteria in hospital choice. These criteria form the basis for experimental research based on CPT tools (see Section 3).
The characteristics of the decision-maker must also be considered when studying decision strategy selection in hospital choice. Here, the patient’s cognitive ability, knowledge, and expertise are key factors (Bettman et al., Citation1991). Moreover, socio-demographic and disease characteristics have also been shown to affect hospital preference formation (Victoor et al., Citation2012).
In general, patients are limited in their role as decision-maker. First of all, health care services are credence goods as patients are usually not able to accurately judge the quality of the treatment they receive (Kahn et al., Citation1997). Blatant information asymmetries between patients and medical professionals exist that lead to a principal-agent constellation between the two parties (Gafni, Charles, & Whelan, Citation1998). In order to reach optimal outcomes, policy makers currently pursue a shift from traditional paternalistic choice models towards enhanced patient autonomy and engagement in shared decision-making (Coulter, Citation2010; Elwyn, Edwards, Kinnersley, & Grol, Citation2000). However, not all patients want to be equally involved in shared medical decision-making together with the health care professionals (Flynn, Smith, & Vanness, Citation2006; Rosén, Anell, & Hjortsberg, Citation2001). Secondly, situational properties emerging from the health care context turn patients into idiosyncratic decision-makers (Berry & Bendapudi, Citation2007; Crié & Chebat, Citation2013). The infrequent necessity for medical service is mostly unplanned and brings patients into a position of high involvement, high stress, and high emotional vulnerability (Kahn et al., Citation1997; Pierce & Hicks, Citation2001). Sometimes, patients even experience fear or anxiety (Frank, Citation2004; Tang, Citation2012). Thirdly, the concepts of health literacy and patient empowerment are both crucial to the individual capability of health-related decision-making as they influence the way patients understand and handle information (Schulz & Nakamoto, Citation2013). Yet, these factors have only recently begun to become the subject of scientific investigation. A recent study indicates that lower levels of individual health literacy and patient activation are associated with less-active provider choice (Rademakers, Nijman, Brabers, de Jong, & Hendriks, Citation2014).
Hospital choice, importantly, is not performed in a vacuum, but is also influenced by numerous social factors (Bettman et al., Citation1991). In their framework, Payne et al. (Citation1993) refer mainly to the concept of accountability (i.e. the need to justify the decision to others) and group membership constraints. However, with regard to hospital choice the patient needs to be regarded within the context of all relevant others, including general practitioners, family, and friends. Commonly, patients generally rely significantly on the advice of general practitioners as the role between the patient and the caregiver is strongly intertwined (Kahn et al., Citation1997; Victoor et al., Citation2012). Likewise, people tend to highly value the experience of other patients (de Groot et al., Citation2012) and the recommendations of relatives and acquaintances (Leister & Stausberg, Citation2007). Thus, the processes of self-evaluation and the evaluation of significant others are of importance in the hospital choice context (Bettman, Luce, & Payne, Citation1998; Payne et al., Citation1993).
3. Literature review on patient decision-making strategies
3.1. Patient information behavior
Studying decision-making strategies of patients implies a solid understanding of their information acquisition and information processing behavior. Even though the literature on the topic of health information behavior and health communication is extensive (Kreps, Citation2012), our study has a focus on patient information behavior in the context of hospital choice. In this specific domain, research is much more limited. Major empirical and conceptual research results in this domain are summarized in the following.
Patients have a multitude of information channels at their disposal. Especially in today’s digital world, patients increasingly search the Internet for relevant information on health care providers (Aase & Timimi, Citation2013; Drevs & Hinz, Citation2013; Huesch, Currid-Halkett, & Doctor, Citation2014). Even though online media are becoming increasingly important, they cannot fully substitute personal information exchange in health communication (Baker, Wagner, Singer, & Bundorf, Citation2003; Cline & Haynes, Citation2001). Thus, patients’ hospital information behavior can be split into formal (mostly impersonal) and informal (mostly personal) sources.
Formal information sources cover all available data in public on providers such as comparative reports or performance data published on the Internet, hospital quality reports, or information in other media outlets (e.g. newspapers). Interestingly, evidence indicates that performance information that is publicly available has only limited influence on provider choice, a fact that has been demonstrated in the UK (Dixon, Citation2010; Laverty, Dixon, & Millett, Citation2013), in Germany (de Cruppé & Geraedts, Citation2011), and in the Netherlands (de Groot, Otten, Smeets, & Marang-van de Mheen, Citation2011). Research also shows that patients rarely use formal information sources on hospitals because they have difficulties in understanding the information provided, are not interested in the nature of the information, lack trust in the underlying data, or have problems to access the sources (Damman, Hendriks, Rademakers, Delnoij, & Groenewegen, Citation2009; Hibbard, Slovic, & Jewett, Citation1997; Marshall, Shekelle, Leatherman, & Brook, Citation2000). Overall, formal information sources do not represent the primary information base for patients.
Patients often rely on information from informal, usually personal contacts, and experiences. For example, de Groot et al. (Citation2011) showed that even patients who were already familiar with comparing hospitals still mostly relied on their own and other people’s knowledge rather than on information which is publicly available. Generally, the main sources of information used in hospital choice are the patient’s own experiences, advice of the general practitioner (GP), and recommendations from the immediate social environment such as family and friends (Dealey, Citation2005; Laverty et al., Citation2013; Moser, Korstjens, van der Weijden, & Tange, Citation2010b; Victoor et al., Citation2012). In this context, it is important to note that several studies report that the GP is seen as the most trustworthy source of information with great influence on decision-making (Dixon, Citation2010; Hesse et al., Citation2005; Wilson, Woloshin, & Schwartz, Citation2007). In particular, elderly persons value the GP’s advice (Schwartz, Woloshin, & Birkmeyer, Citation2005). Other studies indicate that personal experience is a highly valuable information source, predominantly because it is, at least sometimes, the only available reference for patients (Moser et al., Citation2010b; Victoor et al., Citation2012).
Moreover, Jung, Feldman, and Scanlon (Citation2011) stress the influence of satisfaction with previous hospital stays on future hospital choice. However, the experience-based information provided by other patients is also an esteemed benchmark in hospital selection (de Groot et al., Citation2012). Narrative, anecdotal information can even reinforce the effect of performance data (Huppertz & Carlson, Citation2010). Hence, the entire social network of patients is considered to be a reliable information source (Moser, Korstjens, van der Weijden, & Tange, Citation2010a; Victoor et al., Citation2012). This social influence extends its significance towards the phenomenon of hospital reputation. Considered as a general quality indicator (Drevs, Citation2013), hospital reputation can be one of the main reasons for hospital choice (Dijs-Elsinga et al., Citation2010; Jung et al., Citation2011).
Our analyses suggest that the concepts of trust and patient skills play a vital role in patient information behavior. Whereas trust in comparative consumer information (e.g. reports on the Internet) proves to be rather low, patients place more trust in information from peers (Moser et al., Citation2010b). Overall, physicians remain the most trustworthy source of health information even if the source credibility of online media is increasing (Erdem & Harrison-Walker, Citation2006; Hesse et al., Citation2005). Considering the difficulties involved in acquiring and processing hospital information, the multidimensional concept of trust is a decisive mediator in hospital choice. Information sources linked with multiple positive attributes, such as accuracy, knowledge, and concern with public welfare, lead to more patient trust (Frewer, Howard, Hedderley, & Shepherd, Citation1996).
After patients have accessed an information source, they need to be able to evaluate the information provided. This skill is mainly determined by health literacy and patient empowerment (Zwijnenberg et al., Citation2012). For example, patient information and processing behavior varies with the individual capability of handling different types and quantities of health-related information (Hibbard & Peters, Citation2003) and the individual active or passive orientation towards the health topics (Dutta-Bergman, Citation2004).
3.2. Review of decision criteria and patient decision-making strategies for hospital choice
In this section, we discuss crucial decision criteria for hospital choice. Based on these findings, important decision strategies are portrayed. Based on a comprehensive literature review, Victoor et al. (Citation2012) distinguish three categories of provider characteristics: structure variables (e.g. type and size of the provider), process variables (e.g. waiting time), and outcome indicators (e.g. mortality rates). As a major conclusion of their study, Victor and colleagues write that “whether and how patients choose a provider and their eventual choices are determined by the interplay between patient and provider characteristics” (Victoor et al., Citation2012, p. 11).
Research also indicates that patient and provider characteristics relate to each other. For example, research shows that a preference for short waiting times is correlated with lower education (de Groot et al., Citation2012) and masculinity (Birk, Gut, & Henriksen, Citation2011). Other studies show that age, as well as social status, affect whether patients bypass the nearest local hospital (Roh & Moon, Citation2005; Varkevisser & van der Geest, Citation2007). Besides these social-demographic variables, the importance that patients attach to different preference variables also depends on their disease characteristics and their knowledge, attitudes, and beliefs (Victoor et al., Citation2012).
Several provider characteristics (e.g. quality of care) cannot be judged accurately by most patients. This leads to the usage of proxy variables to assess providers fit for individual needs (McCullough & Dodge, Citation2002). As patients find it hard to assess a multitude of potentially relevant factors, they develop a tendency to use more intuitive decision-making patterns and tend to apply decision heuristics, instead of more formal decision algorithms such as the expected utility model (Boyce, Dixon, Fasolo, & Reutskaja, Citation2010; Lubalin & Harris-Kojetin, Citation1999). Hibbard et al. (Citation1997) elucidate that patients give more weight to precise and concrete variables, such as costs or location, and less weight to “fuzzy” variables, such as vague quality indicators. Thus, patients base their choice on a limited amount of information rather than all data available (Hibbard et al., Citation1997; Victoor et al., Citation2012). As a result of this simplification strategy, patients are likely to overvalue single, easy-to-understand information cues when choosing a hospital, and hence are likely to select one dominant choice factor that is easily assessable to them, even though this information may even be inaccurate (Lubalin & Harris-Kojetin, Citation1999). These mental shortcuts, or intuitive heuristics in decision-making, reduce the demand on the patient’s cognitive capacity (Shah & Oppenheimer, Citation2008).
Based on the presented evidence and reasoning processes, we hereafter assume that patients in a hospital choice context predominantly use decision heuristics. However, it is unclear which exact heuristics are effectively applied. As both the theoretical and empirical work on the phenomenon of decision heuristics is extensive and conceptually overlapping, Shah and Oppenheimer (Citation2008) propose an effort reduction framework for studying heuristics, which complements the adaptive decision-making model developed by Payne et al. (Citation1993). Shah and Oppenheimer note that all decision-makers employ heuristics to reduce cognitive effort. Accordingly, they suggest that people make use of five distinct effort reduction principles: (i) examining fewer information cues, (ii) reducing the difficulty associated with retrieving and storing the cue values, (iii) simplifying the weighting principles for information cues, (iv) integrating less information, and/or (v) examining fewer alternatives. Overall, integrating existing knowledge about consumer choice theories, patient information behavior, and decision criteria for hospital selection, we argue that patients are likely to use these effort reduction mechanisms in hospital choice tasks. It follows that patients seldom use highly formalized decision strategies, such as the multi-attribute utility model or similar decision strategies where a decision-maker chooses the alternative with the highest weighted overall utility score, defined as the sum of the weighted attribute values (for a review, see Riedl, Brandstätter, & Roithmayr, Citation2008). Some scholars even argue that patients simply rely on “default” health care provider options (Bryant, Bown, Bekker, & House, Citation2007). Moreover, research shows that patients presented with simple information formats comprehended that information better and are more active in using these information cues (Peters, Dieckmann, Dixon, Hibbard, & Mertz, Citation2007).
Altogether, our discussion of related work suggests that one goal of patients during the decision-making process in hospital choice is to reduce information complexity and cognitive effort. It follows that patients are more likely to apply decision strategies which are characterized by properties that are related to low levels of information complexity and cognitive effort. Based on a review of decision strategies by Riedl et al. (Citation2008), who investigated 13 widely known decision strategies, Table defines and classifies these strategies. We use the following two properties (formulated as questions) for classification.
• | Property 1: Does the application of the strategy imply that information on attribute values of the decision alternatives is ignored? | ||||
• | Property 2: Does the application of the strategy imply quantitative or qualitative reasoning? |
Table 1. Decision strategies and two important properties
Strategies that involve adding, subtracting, and/or multiplying values, as well as counting, are considered to be quantitative, while strategies that simply compare values are regarded as qualitative. Strategies which (i) ignore attribute values (thereby not processing all available information), and (ii) are based on qualitative reasoning, imply lower levels of information complexity and cognitive effort than strategies which do not have these two attributes. The classification in Table shows that five strategies are characterized (i) by not processing all available information (i.e. YES in the second column) and (ii) by using qualitative reasoning (i.e. QL in the third column), namely the disjunctive strategy (DIS), elimination-by-aspects (EBA), lexicographic strategy (LEX), recognition heuristic (REC), and satisficing heuristic (SAT).
Figure presents a summary of our discussion, and serves as a theoretical basis for the sections to follow. In particular, we make a call for experimental research in the field of hospital choice. Almost all papers discussed in the preceding paragraphs are either conceptual in nature or used surveys and interviews as data collection methods. This state is problematic because both theory and practice would benefit greatly from experimental studies in which researchers deliberately manipulate independent variables (e.g. decision complexity, operationalized via the number of alternatives and attributes) in order to study the resulting effects on dependent variables (e.g. decision-making strategies used to select a hospital) and to understand mediating mechanisms (e.g. information acquisition patterns). Findings based on experimental research could be used to validate existing research findings which were derived through application of other methods. In particular, it could be assessed whether results based on self-reports collected in field settings are similar to future findings based on behavior data collected in laboratory environments. Generally, while having contributed to scientific progress, previous research has largely neglected consideration of internal validity of research findings defined as an attribute of research studies which reflects the extent to which a causal conclusion is warranted. Self-reports predominantly reflect people’s beliefs, and hence causal conclusions about reality based on self-reported data should always be validated based on other sources of data, such as observation of behavior.
Figure 2. Expected cognitive heuristics in hospital choice derived from evidence of hospital choice strategies.
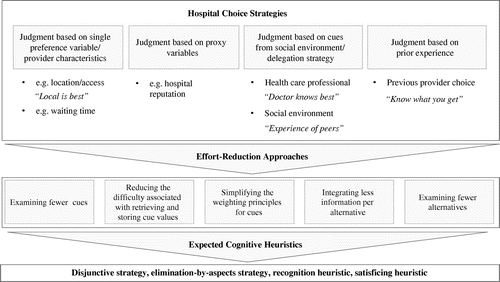
Against this background, in Section 4, we describe CPT tools. These tools are computer programs designed to study people’s decision behavior, including information acquisition and integration. Thus, the tools constitute a way to observe, supported by computer technology, patient’s decision-making strategies in hospital choice in a laboratory setting. To the best of our knowledge, CPT tools have not been used so far in hospital choice research. Because such tools offer enormous potential for the study of patient decision-making, we make a call for the application of CPT tools in future research.
4. Experimental research in hospital choice with computerized process-tracing tools
4.1. Introduction to CPT tools
Recently, Scammon et al. (Citation2011, p. 19) emphasized the necessity for a better understanding of how people such as health care consumers integrate information from various sources and how patients make trade-offs between information. However, there is scant work addressing this issue and proposing research methods to conduct corresponding studies in health service research.
We surmise that one major reason for this research deficit is health service researchers’ missing awareness of the availability of CPT tools. These tools are designed to reveal human information acquisition and decision behavior in experimental decision-making situations. More specifically, the aim of process tracing is to investigate human information acquisition behavior and to reveal the cognitive processes which lead to a final decision or solution when facing a decision problem (Riedl et al., Citation2008; Svenson, Citation1979).
Process tracing, in general, involves techniques used to trace the decision process by collecting data during a decision task. These techniques can provide insight into the cognitive activities that occur between the onset of a stimulus (i.e. a decision problem) and the resulting choice (e.g. Cook & Swain, Citation1993). Among the most important techniques which are in use in contemporary research are (i) verbal protocols (e.g. Ericsson & Simon, Citation1980; Payne & Ragsdale, Citation1978), (ii) eye-tracking (e.g. Pfeiffer et al., Citation2014; Russo & Rosen, Citation1975), and (iii) computer-based analysis of information behavior (e.g. Johnson, Payne, Schkade, & Bettman, Citation1986; Riedl et al., Citation2008). In this article, we focus on the third category only, namely CPT tools. Readers interested in verbal protocols and eye-tracking are referred to the scientific literature.Footnote1
In a typical CPT tool decision experiment, a subject faces an “alternatives × attributes” decision matrix on a computer screen (the matrix is usually referred to as information display matrix [IDM]). In most studies, stimuli are attribute values that are presented in the cells of the matrix (Ford, Schmitt, Schechtman, Hults, & Doherty, Citation1989; Riedl et al., Citation2008). At the beginning of a choice experiment, all cells in the matrix are closed. To arrive at the final decision, a subject has to open cells of the matrix, either by clicking on it or by moving the cursor over the cell. While the participant opens a new cell, the previously opened cell automatically closes. Hence, during a typical CPT tool experiment, there is always only one cell opened at a time. After the final response has been given by the subject (either the selection of one preferred alternative or a rank order of alternatives), a computer algorithm analyzes the subject’s information acquisition behavior, and based on this analysis, the researcher can infer participants’ cognitive processes and thereby the decision strategy used. Thus, CPT tools can be used to experimentally study health care consumers’ decision strategies in hospital choice scenarios. In 1986, Johnson et al. introduced the first CPT tool called “Mouselab” (Johnson et al., Citation1986) which represents the conceptual foundation of all other CPT tools. A screenshot of this IDM-based tool is shown in Figure .
The screen shows a 3 × 3 decision matrix, where the subject has to choose one house out of three available alternatives on the basis of three attributes. In this experiment, the alternatives are presented row-wise (“House A,” “House B,” and “House C”) and the attributes column-wise (“Cost,” “Size,” and “Neighborhood”). A click with the mouse pointer on a particular cell opens it and reveals the hidden information (see “Suburbs” in Figure ). Moreover, there are three cells below the decision matrix, one for each alternative. When the subject has examined as many information items as necessary to make a decision, a click on one of the three cells (“House A,” “House B,” and “House C”) indicates the final decision, that is the preferred house. Figure illustrates that the subject, in this example, has chosen “House B.”
While a subject clicks on the various information items on the screen, the computer program automatically traces every click of the subject, thereby generating an individual clickstream pattern. Based on this clickstream data (i.e. information acquisition behavior and final choice), along with information on the alternatives and attributes which is specified by the researcher before the experiment, computer programs determine a number of metrics, which, in turn, provide evidence for the application of different decision strategies. Example metrics are (sorted by the date of their publication): decision time (Hogarth, Citation1975); proportion of information searched, search index (SI), and variability in the amount of information searched per option (Payne, Citation1976); reacquisition rate (Jacoby, Chestnut, Weigl, & Fisher, Citation1976); variability in the amount of information searched per attribute and a contingency measure (Klayman, Citation1982); total amount of processing, total amount of time spent on the information in the boxes, and average time spent per item of information acquired (Payne et al., Citation1993); a strategy measure (Böckenholt & Hynan, Citation1994); a multiple-step transition index (Ball, Citation1997); and ratio of option-wise transitions to attribute-wise and mixed transitions, ratio of time spent on options, correlation between attribute rank and number of boxes opened for each attribute, and rank order of options (Riedl et al., Citation2008). Further details on metrics, as well as an example algorithm of the CPT tool DecisionTracer, are provided in Riedl et al. (Citation2008).
4.2. Analysis of CPT tools
We reviewed the scientific literature to identify CPT tools. The search was conducted during fall 2015. Data sources included the following Internet databases: ACM, EbscoHost, IEEE, and Social Science Citation Index. To identify relevant articles, we used the words “computerized process tracing,” “process tracing,” and “information display matrix” which we filtered by title and by topic. Altogether, we identified 12 tools, and papers on these tools were published in the period from 1988 to 2012. Importantly, to be included in further analyses, a tool had to meet two criteria: (1) the tool has to be matrix-based (IDM), and (2) the tool has to be available and technologically usable (i.e. not outdated due to old-fashioned operating systems on which the program runs). The following eight tools did not meet one or both criteria: Mouselab (Johnson et al., Citation1986) (1: yes, 2: no), SearchMonitor (Brucks, Citation1988) (1: no, 2: no), ISLab (Cook & Swain, Citation1993) (1: yes, 2: no), ComputerShop (Huneke, Citation1996) (1: no, 2: no), IScube (Tabatabai, Citation1998) (1: yes, 2: no), MouseTrace (Jasper & Shapiro, Citation2002) (1: yes, 2: no), WebDiP (Schulte-Mecklenbeck & Neun, Citation2005) (1: no, 2: yes), and Flashlight (Schulte-Mecklenbeck et al., Citation2011b) (1: no, 2: yes).
However, the following four tools met both criteria: IDM Visual Processor (Schmücker, Citation2002), MouselabWEB (Willemsen & Johnson, Citation2005), DecisionTracer (Riedl et al., Citation2008), and InterActive Process Tracing (Reisen, Hoffrage, & Mast, Citation2008). What all four tools have in common is that they were developed to directly uncover the cognitive processes that take place between the onset of a decision task and the final choice of the decision-maker. Yet, the tools can be distinguished based on their individual features. Next, we describe a framework which serves as a conceptual basis to compare the four CPT tools. Based on this comparison, health service researchers and scholars from related fields (e.g. psychology) interested in studying people’s decision behavior in hospital choice based on CPT tools can make an informed decision concerning whether or not to use a specific tool.
Researchers using CPT tools deliberately manipulate independent variables to study the effects on dependent variables. Moreover, moderator variables (altering the independent variables’ influence on dependent variables) can be included into studies based on CPT tools.
The scientific literature reports on a number of variables which can be considered as independent variable candidates in process-tracing research (e.g. Bettman, Johnson, Luce, & Payne, Citation1993; Johnson & Payne, Citation1985; Payne et al., Citation1993). The candidates can be divided into two categories: task and context variables (see also Figure ). Task variables refer to general structural characteristics of a particular decision problem, such as the number of alternatives or attributes available in a decision set, the response mode (i.e. either the selection of one preferred alternative or a rank order of alternatives), or time pressure. Context variables, in contrast, refer to the particular attribute values of the alternatives. Examples are the similarity of the attribute values across alternatives or the existence of a dominant alternative in the decision set (i.e. one that is at least as good as every other alternative on all attributes and better on at least one attribute).
The major dependent variables in process-tracing research are specific decision strategies (see, for example, the list in Table ). However, it is also possible to use specific properties of decision strategies as dependent variables in process-tracing studies (Riedl et al., Citation2008). Research has identified several such properties (reviewed in Riedl et al., Citation2008). The characterization of a decision strategy and whether its underlying information search is alternative-based or attribute-based is among the most important properties, and research has developed formal metrics to assess, based on a specific information acquisition pattern in a IDM, whether search is more alternative or attribute based. In alternative-wise processing, the attribute values of a single alternative are considered before information about the next alternative is processed (e.g. a subject reveals information about “Cost,” “Size,” and “Neighborhood” for “House A” before continuing with “House B” or “House C,” Figure , or “Access,” “Reputation,” and “Prior Experience” of “Hospital A” before considering “Hospital B”). In attribute-wise processing, the values of several alternatives on a single attribute are processed before information about a further attribute is processed (e.g. a subject reveals information about “Cost” for “House A,” “House B,” and “House C” before continuing with “Size” or “Neighborhood,” Figure ). Payne’s (Citation1976, p. 376) SI, among other metrics (Böckenholt & Hynan, Citation1994), provides a formal way to calculate the “direction of search” that can be either “interdimensional” (alternative-wise) or “intradimensional” (attribute-wise). Specifically, SI puts the number of alternative-wise transitions (ralt) in relation to the number of attribute-wise transitions (rattr). SI varies from −1 to + 1, with −1 indicating a completely attribute-wise search and + 1 indicating a completely alternative-wise search; SI = (ralt−rattr):(ralt+rattr). Researchers studying patients’ decision-making behavior in hospital choice scenarios, along with underlying information acquisition patterns, would greatly benefit from tools which automatically calculate metrics such as the SI, or even provide information on the specific decision strategy used by a subject. Importantly, such tools generally provide not only information on metrics such as the SI, but also on more basic variables, such as amount of information searched (e.g. a subject who clicks on 7 out of the 9 cells in Table 3 before making the final choice would “produce” a value of 78% searched information) or total decision time (i.e. the time from the first click in the IDM until the final response). Thus, while the major dependent variables in process-tracing research are specific decision strategies and specific properties of decision strategies (e.g. direction of search, SI), it is also possible to investigate the effect of independent variables (e.g. number of alternatives) on basic variables such as decision time. The application logic of independent and dependent variables in an experiment based on a CPT tool is summarized through a flow chart, see Figure .
Figure shows that a CPT tool directly allows for the consideration of independent and dependent variables in a decision-making experiment. The independent variables can be split into task and context variables. The ultimate dependent variables in process-tracing research are specific decision strategies (Riedl et al., Citation2008), and precursor elements are characteristics of human information acquisition behavior. These characteristics may also be used as dependent variables either directly (e.g. decision time), or indirectly via calculation of metrics (e.g. direction of search, SI). As shown, task and context variables may have an influence on the characteristics of human information behavior, and thus may also affect the use of specific decision strategies because algorithmic combinations of various metrics (which are, in turn, calculated based on characteristics of information acquisition behavior) have been shown to allow for precise identification of decision strategies (Riedl et al., Citation2008).
The following paragraph leads to a point-by-point feature analysis of the four identified CPT tools. This analysis is based on distinguishing features that can be split into two major categories: task factors and technology factors. Task factors refer to the general structural characteristics of a particular decision problem and correspond to task variables (e.g. number of alternatives, time pressure). Technology factors refer to the technology of a CPT tool and the general technical capabilities (e.g. online or offline, visualization capabilities, algorithmic capabilities). Based on these factors, we compared the four CPT tools that we identified in the pre-selection phase: IDM Visual Processor (Schmücker, Citation2002), MouselabWEB (Willemsen & Johnson, Citation2005), DecisionTracer (Riedl et al., Citation2008), and InterActive Process Tracing (Reisen et al., Citation2008). Our analysis of the four CPT tools shows the strengths and weaknesses of the individual tools (see Table ).
Table 2. Analysis of CPT tools
Although all of these tools are designed to reveal human information acquisition behavior in experimental decision-making situations, a researcher should be aware of the fact that no tool dominates all other tools on the described factors. Thus, depending predominantly on the research scenario at hand (i.e. research questions and hypotheses to be tested), scholars interested in using a specific tool must make a deliberate selection. In order to equip health care marketing scholars with a blue print of a process-tracing experiment in the hospital choice context (independent from the specific CPT tool that is used for the study), in the Appendices, based on an extensive review of the literature, we provide a list with relevant attributes, along with a detailed description of these attributes. Importantly, these attributes are the input for the preparation of an IDM in a decision experiment. Researchers are invited to select attributes from this list for their experiments, and we have made an attempt to provide a list which is as comprehensive as possible. However, this list does not claim to be exhaustive, as it cannot be ruled out that attributes which are not in our list turn out to be important in a specific research context.
5. Conclusion
The topic of hospital choice has received considerable attention in health care marketing. Increasingly, more often scholars apply a cognitive perspective, complementing the traditional view that considered patients as rational decision-makers. In this paper, we argued that knowledge from psychology and cognitive science may serve as a vital basis for a better understanding of hospital choice preferences and underlying cognitive mechanisms. Patients’ decision-making strategies in hospital choice are largely unknown today, and hence little is known about how people acquire and use information in hospital choice. One potential reason for this research deficit is health service researchers’ missing awareness of the availability of CPT tools. These tools make investigation of human information acquisition and decision behavior possible in experimental decision-making situations.
We addressed three main research questions in this article: (1) Which theoretical framework can be used to advance hospital choice research? (2) What do we know about patient decision-making, especially regarding patient information behavior and relevance of decision criteria, in hospital choice? (3) Which methodological tools are needed to advance scientific understanding of patients’ decision–making strategies in hospital choice? To answer the first question, we introduced the adaptive decision-making model by Payne et al. (Citation1993) and outlined its applicability to the hospital choice context. Regarding question 2, we reviewed the existing literature on patient information behavior and decision criteria. As a result, we outlined expected cognitive heuristics in hospital choice. With respect to question 3, we argued that CPT should become part of the health service researcher’s toolbox. Specifically, we reviewed the scientific literature to identify CPT tools. Four tools were analyzed in detail: IDM Visual Processor (Schmücker, Citation2002), MouselabWEB (Willemsen & Johnson, Citation2005), DecisionTracer (Riedl et al., Citation2008), and InterActive Process Tracing (Reisen et al., Citation2008). All four tools in common were developed to directly uncover the cognitive processes that take place between the onset of a decision task (presented in a matrix format, IDM) and the final choice of the decision-maker. Based on our comparison of the four tools, health service researchers and scholars from related fields (e.g. health marketing) can make an informed decision about whether or not to use a specific tool. Moreover, we provide a comprehensive Appendix in which we outline an IDM matrix example, as well as a concrete list of preference variables and attributes which have been identified as critical for hospital choice. Therefore, in this paper, we not only provided conceptual foundations of process-tracing research and related theoretical foundations, but also provided concrete information necessary for researchers to get started with corresponding experiments.
Despite our call for more experimental research in the field of patients’ decision behavior in hospital choice settings, we note that experimental studies have limitations that must be taken into account, particularly if compared to the more traditional survey-based research stream. We point to the concept of external validity, defined as the extent to which the results of an (experimental) study can be generalized to other (real-life) situations. Obviously, human decision-making behavior in laboratory settings never equals behavior in real-life situations. Thus, results of laboratory experiments based on CPT tools must be interpreted carefully. Despite this general limitation of laboratory experiments, it is difficult to imagine that future results of CPT tools experiments will not contribute to a better understanding of decision-making in hospital choice scenarios. It will be rewarding to see what insight future research will reveal in this respect.
Finally, we would like to broaden the research perspective of this paper by emphasizing that the approach of this study can serve as blue print for scholars who aim at analyzing service provider selection processes from a psychological perspective. Decision-making strategies of service recipients in many other industrial sectors could be analyzed with CPT tools, including provider choice of financial service agents, or insurances and real estate brokers. Therefore, this research not only contributes to the advancement of health care research, but to service research in general.
Additional information
Funding
Notes on contributors
Sophia Fischer
Sophia Fischer was a research associate at research group InnoTech4Health, Technische Universität Dresden, Germany. Currently, she is working as product manager in the health care sector. Her doctoral dissertation focuses on hospital marketing and patient choice behavior. When studying decision-making processes, she developed a joint research interest with Stefanie Pelka and René Riedl which led to a cooperative partnership.
Stefanie Pelka
Stefanie Pelka worked in the financial sector as a software and license manager and is presently on maternity leave.
René Riedl
René Riedl is a professor of Digital Business and Innovation at the University of Applied Sciences Upper Austria. Moreover, he is an associate professor at the Department of Business Informatics—Information Engineering, University of Linz, Austria. He has published in the following journals, among others: Behavior Research Methods, Journal of Information Technology, Journal of the Association for Information Systems, Journal of Management Information Systems, MIS Quarterly.
Notes
1. A verbal protocol requires the subject to “think aloud” while facing a decision problem. The subject is asked to verbalize every single thought that comes into the mind during the decision process. With eye-tracking tools, it is possible to record people’s visual fixations, eye-movements (saccades), and pupil dilation (often used as a proxy for emotions) during a decision task (further information on these two methods in the context of process-tracing research is available in Schulte-Mecklenbeck, Murphy, & Hutzler, Citation2011b).
2. Weisse Liste is a German health care information and provider rating website that also covers hospital rankings (Weisse Liste, Citation2013). Weisse Liste has a methodologically sound survey instrument to evaluate patient’s experience of hospitals. This instrument is based on both public reporting and patient assessments. For more details on project Weisse Liste please see Fischer (Citation2014).
References
- Aase, L., & Timimi, F. K. (2013). Health care social media. Clinical Obstetrics and Gynecology, 56, 471–476. doi:10.1097/GRF.0b013e31829d6058
- Adams, E. K., Houchens, R., Wright, G. E., & Robbins, J. (1991). Predicting hospital choice for rural medicare beneficiaries: The role of severity of illness. Health Services Research, 26, 583–612.
- Akin, J. S., & Hutchinson, P. (1999). Health-care facility choice and the phenomenon of bypassing. Health Policy and Planning, 14, 135–151.10.1093/heapol/14.2.135
- Akinci, F., Esatoğlu, A. E., Tengilimoglu, D., & Parsons, A. (2004). Hospital choice factors: A case study in Turkey. Health Marketing Quarterly, 22, 3–19. doi:10.1300/J026v22n01_02
- Baker, L., Wagner, T. H., Singer, S., & Bundorf, M. (2003). Use of the internet and e-mail for health care information. JAMA, 289, 2400–2406. doi:10.1001/jama.289.18.2400
- Ball, C. (1997). A comparison of single-step and multiple-step transition analyses of multiattribute decision strategies. Organizational Behavior and Human Decision Processes, 69, 195–204.10.1006/obhd.1997.2681
- Berry, L. L., & Bendapudi, N. (2007). Health care: A fertile field for service research. Journal of Service Research, 10, 111–122.
- Bettman, J. R., Johnson, E. J., Luce, M. F., & Payne, J. W. (1993). Correlation, conflict, and choice. Journal of Experimental Psychology: Learning, Memory, and Cognition, 19, 931–951. doi:10.1037/0278-7393.19.4.931
- Bettman, J. R., Johnson, E. J., & Payne, J. W. (1991). Consumer decision making. In T. S. Robertson & H. H. Kassarjian (Eds.), Handbooks of consumer theory and research (pp. 50–84). Englewood Cliffs, NJ: Prentice-Hall.
- Bettman, J. R., Luce, M. F., & Payne, J. W. (1998). Constructive consumer choice processes. Journal of Consumer Research, 25, 187–217. doi:10.1086/209535
- Bettman, J. R., Luce, M. F., & Payne, J. W. (2012). Consumer decision making: A choice goal approach. In C. P. Haugtvedt, P. M. Herr, & F. R. Kardes (Eds.), Handbook of consumer psychology (pp. 589–610). New York, NY: Taylor and Francis.
- Beukers, P. D. C., Kemp, R. M., & Varkevisser, M. (2013). Patient hospital choice for hip replacement: Empirical evidence from the Netherlands. The European Journal of Health Economics, 15, 927–936. doi:10.1007/s10198-013-0535-7
- Birk, H., Gut, R., & Henriksen, L. (2011). Patients’ experience of choosing an outpatient clinic in one county in Denmark: Results of a patient survey. BMC Health Services Research, 11, 262. doi:10.1186/1472-6963-11-262
- Birk, H. O., & Henriksen, L. O. (2006). Why do not all hip- and knee patients facing long waiting times accept re-referral to hospitals with short waiting time? Health Policy, 77, 318–325. doi:10.1016/j.healthpol.2005.08.002
- Böckenholt, U., & Hynan, L. S. (1994). Caveats on a process-tracing measure and a remedy. Journal of Behavioral Decision Making, 7, 103–117. doi:10.1002/bdm.3960070203
- Boyce, T., Dixon, A., Fasolo, B., & Reutskaja, E. (2010). Choosing a high-quality hospital: The role of nudges, scorecard design and information. Retrieved from http://www.kingsfund.org.uk/sites/files/kf/field/field_publication_file/Choosing-high-quality-hospital-role-report-Tammy-Boyce-Anna-Dixon-November2010.pdf
- Brucks, M. (1988). Search monitor: An approach for computer-controlled experiments involving consumer information search. Journal of Consumer Research, 15, 117–121.10.1086/jcr.1988.15.issue-1
- Bryant, L. D., Bown, N., Bekker, H. L., & House, A. (2007). The lure of ‘patient choice’. The British Journal of General Practice, 57, 822–826.
- Burge, P., Devlin, N., Appleby, J., Rohr, C., & Grant, J. (2004). Do patients always prefer quicker treatment? Applied Health Economics and Health Policy, 3, 183–194. doi:10.2165/00148365-200403040-00002
- Chaberny, I. F., Sohr, D., Rüden, H., & Gastmeier, P. (2007). Development of a surveillance system for methicillin-resistant staphylococcus aureus in German hospitals. Development, 28, 446–452.
- Cline, R. J. W., & Haynes, K. M. (2001). Consumer health information seeking on the Internet: The state of the art. Health Education Research, 16, 671–692.10.1093/her/16.6.671
- Cook, G. J., & Swain, M. R. (1993). A computerized approach to decision process tracing for decision support system design. Decision Sciences, 24, 931–952. doi:10.1111/j.1540-5915.1993.tb00497.x
- Coulter, A. (2010). Do patients want a choice and does it work? BMJ, 341, 973–975. doi:10.1136/bmj.c4989
- Crié, D., & Chebat, J.-C. (2013). Health marketing: Toward an integrative perspective. Journal of Business Research, 66, 123–126.
- Damman, O. C., Hendriks, M., Rademakers, J., Delnoij, D. M. J., & Groenewegen, P. P. (2009). How do healthcare consumers process and evaluate comparative healthcare information? A qualitative study using cognitive interviews. BMC Public Health, 9(423). doi:10.1186/1471-2458-9-423
- Damman, O. C., Spreeuwenberg, P., Rademakers, J., & Hendriks, M. (2012). Creating compact comparative health care information: What are the key quality attributes to present for cataract and total hip or knee replacement surgery? Medical Decision Making, 32, 287–300. doi:10.1177/0272989x11415115
- de Cruppé, W., & Geraedts, M. (2011). How do patients choose a hospital for elective surgery? Bundesgesundheitsblatt - Gesundheitsforschung - Gesundheitsschutz, 54, 951–957. doi:10.1007/s00103-011-1320-3
- de Groot, I., Otten, W., Smeets, H., & Marang-van de Mheen, P. (2011). Is the impact of hospital performance data greater in patients who have compared hospitals? BMC Health Services Research, 11, 214. doi:10.1186/1472-6963-11-214
- de Groot, I. B., Otten, W., Dijs-Elsinga, J., Smeets, H. J., Kievit, J., & Marang-van de Mheen, P. J. (2012). Choosing between hospitals: The influence of the experiences of other patients. Medical Decision Making, 32, 764–778. doi:10.1177/0272989x12443416
- Dealey, C. (2005). The factors that influence patients’ choice of hospital and treatment. British Journal of Nursing, 14, 576–579.10.12968/bjon.2005.14.10.18109
- Dietrich, M., & Lindenmeier, J. (2009). Standardized quality information and provider choice—Results of an experimental study on hospital quality reports. Zeitschrift für Betriebswirtschaft, 79, 869–896.10.1007/s11573-009-0269-2
- Dijs-Elsinga, J., Otten, W., Versluijs, M. M., Smeets, H. J., Kievit, J., Vree, R., … Marang-van de Mheen, P. J. (2010). Choosing a hospital for surgery: The importance of information on quality of care. Medical Decision Making, 30, 544–555. doi:10.1177/0272989x09357474
- Dixon, A., Robertson, R., Appleby, J., Burge, P., Devlin, N., & Magee, H. (2010). Patient choice: How patients choose and how providers respond. King’s Fund. Retrieved from http://www.kingsfund.org.uk/sites/files/kf/Patient-choice-final-report-Kings-Fund-Anna_Dixon-Ruth-Robertson-John-Appleby-Peter-Purge-Nancy-Devlin-Helen-Magee-June-2010.pdf
- Dixon, S. (2010). Report on the national patient choice survey—February 2010 England. Retrieved from https://www.gov.uk/government/publications/report-on-the-national-patient-choice-survey-england-february-2010
- Drevs, F. (2013). How patients choose hospitals: Using the stereotypic content model to model trustworthiness, warmth and competence. Health Services Management Research, 26, 95–101. doi:10.1177/0951484813513246
- Drevs, F., & Hinz, V. (2013). Who chooses, who uses, who rates: The impact of agency on electronic word-of-mouth about hospitals stays. Health Care Management Review, 39, 223–233. doi:10.1097/HMR.1090b1013e3182993b3182996a
- Drevs, F., Tscheulin, D. K., & Lindenmeier, J. (2012). Do patient perceptions vary with ownership status? A study of nonprofit, for-profit, and public hospital patients. Nonprofit and Voluntary Sector Quarterly., 43, 164–184. doi:10.1177/0899764012458179
- Dutta-Bergman, M. J. (2004). Primary sources of health information: Comparisons in the domain of health attitudes, health cognitions, and health behaviors. Health Communication, 16, 273–288.10.1207/S15327027HC1603_1
- Elwyn, G., Edwards, A., Kinnersley, P., & Grol, R. (2000). Shared decision making and the concept of equipoise: The competences of involving patients in healthcare choices. British Journal of General Practice, 50, 892–899.
- Emmert, M., Maryschok, M., Eisenreich, S., & Schöffski, O. (2009). Websites to assess quality of care: Appropriate to identify good physicians? Gesundheitswesen, 71, e18–27.10.1055/s-0028-1103288
- Emmert, M., Sander, U., & Pisch, F. (2013). Eight questions about physician-rating websites: A systematic review. Journal of Medical Internet Research, 15. doi:10.2196/jmir.2360
- Erdem, S. A., & Harrison-Walker, L. J. (2006). The role of the internet in physician–patient relationships: The issue of trust. Business Horizons, 49, 387–393. doi:10.1016/j.bushor.2006.01.003
- Ericsson, K. A., & Simon, H. A. (1980). Verbal reports as data. Psychological Review, 87, 215–251. doi:10.1037/0033-295x.87.3.215
- Fischer, S. (2014). Project “Weisse Liste”—A German best practice example for online provider ratings in health care. In S. Gurtner & K. Soyez (Eds.), Challenges and opportunities in health care management (pp. 339–346). Wiesbaden: Springer.
- Flynn, K. E., Smith, M. A., & Vanness, D. (2006). A typology of preferences for participation in healthcare decision making. Social Science & Medicine, 63, 1158–1169. doi:10.1016/j.socscimed.2006.03.030
- Focus. (2012). Deutschlands große Klinikliste [Germany’s biggest list of hospitals]. München: Focus Magazin Verlag GmbH.
- Ford, J. K., Schmitt, N., Schechtman, S. L., Hults, B. M., & Doherty, M. L. (1989). Process tracing methods: Contributions, problems, and neglected research questions. Organizational Behavior and Human Decision Processes, 43, 75–117.10.1016/0749-5978(89)90059-9
- Frank, R. G. (2004). Behavioral economics and health economics (National Bureau of Economic Research Working Paper Series, Working paper 10881). Retrieved from http://www.nber.org/papers/w10881
- Frewer, L. J., Howard, C., Hedderley, D., & Shepherd, R. (1996). What determines trust in information about food-related risks? Underlying psychological constructs. Risk Analysis, 16, 473–486. doi:10.1111/j.1539-6924.1996.tb01094.x
- Gafni, A., Charles, C., & Whelan, T. (1998). The physician–patient encounter: The physician as a perfect agent for the patient versus the informed treatment decision-making model. Social Science & Medicine, 47, 347–354. doi:10.1016/S0277-9536(98)00091-4
- G-BA. (2014). Hospital quality report. Retrieved from http://www.english.g-ba.de/special-topics/quality/report/
- Geraedts, M., Schwartze, D., & Molzahn, T. (2007). Hospital quality reports in Germany: Patient and physician opinion of the reported quality indicators. BMC Health Services Research, 7, 157. doi:10.1186/1472-6963-7-157
- Gigerenzer, G., & Goldstein, D. G. (1996). Reasoning the fast and frugal way: Models of bounded rationality. Psychological Review, 103, 650–669.10.1037/0033-295X.103.4.650
- Goldstein, D. G., & Gigerenzer, G. (2002). Models of ecological rationality: The recognition heuristic. Psychological Review, 109, 75–90.10.1037/0033-295X.109.1.75
- Griffin, D., Gonzalez, R., & Varey, C. (2001). The heuristics and biases approach to judgment under uncertainty. In A. Tesser & N. Schwarz (Eds.), Blackwell handbook of social psychology: Intraindividual processes (Vol. 1, pp. 207–235). Malden, MA: Blackwell.
- Halasyamani, L. K., & Davis, M. M. (2007). Conflicting measures of hospital quality: Ratings from “hospital compare” versus “best hospitals”. Journal of Hospital Medicine, 2, 128–134. doi:10.1002/jhm.176
- Halm, E. A., Lee, C., & Chassin, M. R. (2002). Is volume related to outcome in health care? A systematic review and methodologic critique of the literature. Annals of Internal Medicine, 137, 511–520. doi:10.7326/0003-4819-137-6-200209170-00012
- Haustein, T., Gastmeier, P., Holmes, A., Lucet, J.-C., Shannon, R. P., Pittet, D., & Harbarth, S. (2011). Use of benchmarking and public reporting for infection control in four high-income countries. The Lancet Infectious Diseases, 11, 471–481. doi:10.1016/S1473-3099(10)70315-7
- Hesse, B. W., Nelson, D. E., Kreps, G. L., Croyle, R. T., Arora, N. K., Rimer, B. K., & Viswanath, K. (2005). Trust and sources of health information. Archives of Internal Medicine, 12/26, 2618–2624. doi:10.1001/archinte.165.22.2618
- Hibbard, J. H., & Peters, E. (2003). Supporting informed consumer health care decisions: Data presentation approaches that facilitate the use of information in choice. Annual Review of Public Health, 24, 413–433. doi:10.1146/annurev.publhealth.24.100901.141005
- Hibbard, J. H., Slovic, P., & Jewett, J. J. (1997). Informing consumer decisions in health care: Implications from decision-making research. The Milbank Quarterly, 75, 395–414. doi:10.1111/1468-0009.00061
- Hogarth, R. M. (1975). Decision time as a function of task complexity. In D. Wendt & C. A. J. Vlek (Eds.), Utility, probability, and human decision making (pp. 321–338). Dordrecht: Riedel.10.1007/978-94-010-1834-0
- Huerta, T. R., Hefner, J. L., Ford, E. W., McAlearney, A. S., & Menachemi, N. (2014). Hospital website rankings in the United States: Expanding benchmarks and standards for effective consumer engagement. Journal of Medical Internet Research, 16, e64. doi:10.2196/jmir.3054
- Huesch, M. D., Currid-Halkett, E., & Doctor, J. N. (2014). Public hospital quality report awareness: Evidence from national and Californian internet searches and social media mentions, 2012. BMJ Open, 4, doi:10.1136/bmjopen-2013-004417
- Huneke, M. E. (1996). Comparing consideration set formation and choice: An empirical investigation of information processing (Unpublished doctoral dissertation). Iowa, IA: University of Iowa.
- Huppertz, J. W., & Carlson, J. P. (2010). Consumers’ use of HCAHPS ratings and word-of-mouth in hospital choice. Health Services Research, 45, 1602–1613. doi:10.1111/j.1475-6773.2010.01153.x
- Jacoby, J., Chestnut, R. W., Weigl, K. C., & Fisher, W. (1976). Prepurchase information acquisition: Description of a process methodology, research paradigm, and pilot investigation. In B. B. Anderson (Ed.), Advances in Consumer Research (Vol. 3, pp. 306–314). Cincinnati, OH: Association for Consumer Research.
- Jasper, J., & Shapiro, J. (2002). Mousetrace: A better mousetrap for catching decision processes. Behavior Research Methods, Instruments, & Computers, 34, 364–374.
- Johnson, E. J., & Payne, J. W. (1985). Effort and accuracy in choice. Management Science, 31, 395–414.10.1287/mnsc.31.4.395
- Johnson, E. J., Payne, J. W., Schkade, D. A., & Bettman, J. R. (1986). Monitoring information processing and decisions: The Mouselab system ( Unpublished manuscript, pp. 1–53). Center for Decision Studies, Fuqua School of Business, Duke University. Retrieved from http://www.dtic.mil/cgi-bin/GetTRDoc?AD=ADA205963
- Jung, K., Feldman, R., & Scanlon, D. (2011). Where would you go for your next hospitalization? Journal of Health Economics, 30, 832–841. doi:10.1016/j.jhealeco.2011.05.006
- Kahn, B. E., Greenleaf, E., Irwin, J. R., Isen, A. M., Levin, I. P., Luce, M. F., … Young, M. J. (1997). Examining medical decision making from a marketing perspective. Marketing Letters, 8, 361–375. doi:10.1023/a:1007979923514
- Kahneman, D., Slovic, P., & Tversky, A. (1974). Judgment under uncertainty. Cambridge, MA: Cambridge University Press.
- Klayman, J. (1982). Simulations of six decision strategies: Comparisons of search patterns, processing characteristics, and response to task complexity (Unpublished doctoral dissertation). University of Chicago, Chicago, IL.
- Kreps, G. L. (2012). The maturation of health communication inquiry: Directions for future development and growth. Journal of Health Communication, 17, 495–497.10.1080/10810730.2012.685802
- Laverty, A. A., Dixon, A., & Millett, C. (2013). Do patients’ information requirements for choice in health care vary with their socio-demographic characteristics? Health Expectations. doi:10.1111/hex.12086
- Leister, J., & Stausberg, J. (2007). Why do patients select a hospital? Journal of Hospital Marketing & Public Relations, 17, 13–31.
- Lubalin, J. S., & Harris-Kojetin, L. (1999). What do consumers want and need to know in making health care choices? Medical Care Research and Review, 56, 67–102.10.1177/107755899773743873
- Marang-van de Mheen, P. J., Dijs-Elsinga, J., Otten, W., Versluijs, M., Smeets, H. J., van der Made, W. J., & Kievit, J. (2010). The importance of experienced adverse outcomes on patients’ future choice of a hospital for surgery. Quality & Safety in Health Care, 19. doi:10.1136/qshc.2008.031690
- Marewski, J. N., & Gigerenzer, G. (2012). Heuristic decision making in medicine. Dialogues in clinical neuroscience, 14, 77–89.
- Marshall, M. N., Shekelle, P. G., Leatherman, S., & Brook, R. H. (2000). The public release of performance data: What do we expect to gain? a review of the evidence. JAMA, 283(14), 1866–1874. doi:10.1001/jama.283.14.1866
- McCullough, T., & Dodge, H. R. (2002). Understanding the role consumer involvement plays in the effectiveness of hospital advertising. Health Marketing Quarterly, 19, 3–20. doi:10.1300/J026v19n03_02
- McGuckin, M., Waterman, R., & Shubin, A. (2006). Consumer attitudes about health care-acquired infections and hand hygiene. American Journal of Medical Quality, 21, 342–346. doi:10.1177/1062860606291328
- Merle, V., Germain, J. M., Tavolacci, M. P., Brocard, C., Chefson, C., Cyvoct, C., … Czernichow, P. (2009). Influence of infection control report cards on patients’ choice of hospital: Pilot survey. Journal of Hospital Infection, 71, 263–268. doi:10.1016/j.jhin.2008.11.025
- Moser, A., Korstjens, I., van der Weijden, T., & Tange, H. (2010a). Patient’s decision making in selecting a hospital for elective orthopaedic surgery. Journal of Evaluation in Clinical Practice, 16, 1262–1268. doi:10.1111/j.1365-2753.2009.01311.x
- Moser, A., Korstjens, I., van der Weijden, T., & Tange, H. (2010b). Themes affecting health-care consumers’ choice of a hospital for elective surgery when receiving web-based comparative consumer information. Patient Education and Counseling, 78, 365–371. doi:10.1016/j.pec.2009.10.027
- Niehues, S. M., Emmert, M., Haas, M., Schöffski, O., & Hamm, B. (2012). The impact of the emergence of internet hospital rating sites on patients’ choice: A quality evaluation and examination of the patterns of approach. International Journal of Technology Marketing, 7, 4–19. doi:10.1504/ijtmkt.2012.046435
- OECD. (2012). Health at a glance: Europe 2012. OECD Publishing. Retrieved from http://dx.doi.org/10.1787/9789264183896-en
- OECD. (2013). Health at a glance 2013: OECD indicators. OECD Publishing. Retrieved from http://dx.doi.org/10.1787/health_glance-2013-en
- Payne, J. W. (1976). Task complexity and contingent processing in decision making: An information search and protocol analysis. Organizational Behavior and Human Performance, 16, 366–378. doi:10.1016/0030-5073(76)90022-2
- Payne, J. W., Bettman, J. R., Coupey, E., & Johnson, E. J. (1992). A constructive process view of decision making: Multiple strategies in judgment and choice. Acta Psychologica, 80, 107–141. doi:10.1016/0001-6918(92)90043-D
- Payne, J. W., Bettman, J. R., & Johnson, E. J. (1993). The adaptive decision maker. New York, NY: Cambridge University Press.10.1017/CBO9781139173933
- Payne, J. W., & Ragsdale, E. E. K. (1978). Verbal protocols and direct observation of supermarket shopping behavior: Some findings and a discussion of methods. Advances in Consumer Research, 5, 571–577.
- Peters, E., Dieckmann, N., Dixon, A., Hibbard, J. H., & Mertz, C. K. (2007). Less is more in presenting quality information to consumers. Medical Care Research and Review, 64, 169–190. doi:10.1177/10775587070640020301
- Petersen, U., Kaminski, A., & Jackson, A. (2007). Analyse aktueller Klinikführer: Was bringen sie dem Patienten? [Analysis of current hospital guides: What is their value for patients?] Das Krankenhaus, 11, 1112–1117.
- Pfeiffer, J., Meißner, M., Brandstätter, E., Riedl, R., Decker, R., & Rothlauf, F. (2014). On the influence of context-based complexity on information search patterns: An individual perspective. Journal of Neuroscience, Psychology, and Economics, 7, 103–124. doi:10.1037/npe0000021
- Pierce, P. F. (1996). When the patient chooses: Describing unaided decisions in health care. Human Factors: The Journal of the Human Factors and Ergonomics Society, 38, 278–287. doi:10.1177/001872089606380208
- Pierce, P. F., & Hicks, F. D. (2001). Patient decision-making behavior. Nursing Research, 50, 267–274.10.1097/00006199-200109000-00003
- Rademakers, J., Nijman, J., Brabers, A. E. M., de Jong, J. D., & Hendriks, M. (2014). The relative effect of health literacy and patient activation on provider choice in the Netherlands. Health Policy, 114, 200–206. doi:10.1016/j.healthpol.2013.07.020
- Reisen, N., Hoffrage, U., & Mast, F. W. (2008). Identifying decision strategies in a consumer choice situation. Judgment and Decision Making, 3, 641–658.
- Riedl, R., Brandstätter, E., & Roithmayr, F. (2008). Identifying decision strategies: A process-and outcome-based classification method. Behavior Research Methods, 40, 795–807.10.3758/BRM.40.3.795
- Roh, C.-Y., & Moon, M. J. (2005). Nearby, but not wanted? The bypassing of rural hospitals and policy implications for rural health care systems. Policy Studies Journal, 33, 377–394. doi:10.1111/j.1541-0072.2005.00121.x
- Rosén, P., Anell, A., & Hjortsberg, C. (2001). Patient views on choice and participation in primary health care. Health Policy, 55, 121–128. doi:10.1016/S0168-8510(00)00122-6
- Russo, J. E., & Rosen, L. D. (1975). An eye fixation analysis of multialternative choice. Memory and Cognition, 3, 267–276.10.3758/BF03212910
- Scammon, D. L., Keller, P. A., Albinsson, P. A., Bahl, S., Catlin, J. R., Haws, K. L., … Schindler, R. M. (2011). Transforming consumer health. Journal of Public Policy & Marketing, 30, 14–22. doi:10.1509/jppm.30.1.14
- Schmücker, D. J. (2002). IDM visual processor (Appendix to doctoral disseration). Universität Lüneburg, Lüneburg.
- Schulte-Mecklenbeck, M., Kühberger, A., & Ranyard, R. (2011a). The role of process data in the development and testing of process models of judgment and decision making. Judgment and Decision Making, 6, 733–739.
- Schulte-Mecklenbeck, M., Murphy, R. O., & Hutzler, F. (2011b). Flashlight—Recording information acquisition online. Computers in Human Behavior, 27, 1771–1782. doi:10.1016/j.chb.2011.03.004
- Schulte-Mecklenbeck, M., & Neun, M. (2005). WebDiP: A tool for information search experiments on the world-wide web. Behavior Research Methods, 37, 293–300. doi:10.3758/BF03192697
- Schulz, P. J., & Nakamoto, K. (2013). Health literacy and patient empowerment in health communication: The importance of separating conjoined twins. Patient Education and Counseling, 90, 4–11. doi:10.1016/j.pec.2012.09.006
- Schwartz, L. M., Woloshin, S., & Birkmeyer, J. D. (2005). How do elderly patients decide where to go for major surgery? Telephone interview survey. BMJ, 331, 1–7. doi:10.1136/bmj.38614.449016.DE
- Sehgal, A. R. (2010). The role of reputation in US news & world report’s rankings of the top 50 American hospitals. Annals of Internal Medicine, 152, 521–525. doi:10.7326/0003-4819-152-8-201004200-00009
- Shah, A. K., & Oppenheimer, D. M. (2008). Heuristics made easy: An effort-reduction framework. Psychological Bulletin, 134, 207–222.10.1037/0033-2909.134.2.207
- Shah, J., & Dickinson, C. L. (2010). Establishing which factors patients value when selecting urology outpatient care. British Journal of Medical and Surgical Urology, 3, 25–29. doi:10.1016/j.bjmsu.2009.10.003
- Siciliani, L., & Hurst, J. (2003). Explaining waiting times variations for elective surgery across OECD countries (Vol. 38). OECD Economic Studies. Retrieved from http://www.oecd.org/eco/growth/35028282.pdf
- Simon, H. A. (1959). Theories of decision-making in economics and behavioral science. The American Economic Review, 49, 253–283.
- Simon, H. A. (1990). Invariants of human behavior. Annual Review of Psychology, 41(1), 1–20.10.1146/annurev.ps.41.020190.000245
- Statistisches Bundesamt. (2013). Grunddaten der Krankenhäuser 2012. Wiesbaden. Retrieved from https://www.destatis.de/DE/Publikationen/Thematisch/Gesundheit/Krankenhaeuser/GrunddatenKrankenhaeuser2120611117004.pdf
- Svenson, O. (1979). Process descriptions of decision making. Organizational Behavior and Human Performance, 23, 86–112.10.1016/0030-5073(79)90048-5
- Tabatabai, M. (1998). Investigation of decision making process: A hypermedia approach. Interacting with Computers, 9, 385–396. doi:10.1016/S0953-5438(97)00009-X
- Tai, W.-T. C., Porell, F. W., & Adams, E. K. (2004). Hospital choice of rural medicare beneficiaries: Patient, hospital attributes, and the patient-physician relationship. Health Services Research, 39, 1903–1922. doi:10.1111/j.1475-6773.2004.00324.x
- Tang, L. (2012). The patient’s anxiety before seeing a doctor and her/his hospital choice behavior in China. BMC Public Health, 12, 1121. doi:10.1186/1471-2458-12-1121
- Varkevisser, M., & van der Geest, S. (2007). Why do patients bypass the nearest hospital? An empirical analysis for orthopaedic care and neurosurgery in the Netherlands. The European Journal of Health Economics, 8, 287–295. doi:10.1007/s10198-006-0035-0
- Varkevisser, M., van der Geest, S. A., & Schut, F. T. (2012). Do patients choose hospitals with high quality ratings? Empirical evidence from the market for angioplasty in the Netherlands. Journal of Health Economics, 31, 371–378. doi:10.1016/j.jhealeco.2012.02.001
- Victoor, A., Delnoij, D. M. J., Friele, R. D., & Rademakers, J. (2012). Determinants of patient choice of healthcare providers: A scoping review. BMC Health Services Research, 12, 272. doi:10.1186/1472-6963-12-272
- Vonberg, R.-P., Sander, C., & Gastmeier, P. (2008). Consumer attitudes about health care acquired infections: A German survey on factors considered important in the choice of a hospital. American Journal of Medical Quality, 23, 56–59. doi:10.1177/1062860607310915
- Weisse Liste. (2013). Weisse Liste—Wegweiser im Gesundheitswesen: Wir geben Orientierung [Weisse Liste—Guide for German Public Health]. Retrieved from http://www.weisse-liste.de/
- Weisse Liste. (2014). Methode Krankenhaussuche [Methodology for hospital search]. Retrieved from http://www.weisse-liste.de/krankenhaussuche.4028.de.html
- Wendt, C. (2009). Mapping European healthcare systems: A comparative analysis of financing, service provision and access to healthcare. Journal of European Social Policy, 19, 432–445. doi:10.1177/0958928709344247
- Willemsen, M. C., & Johnson, E. J. (2005). MouselabWEB: Monitoring information acquisition processes on the web. Retrieved from http://www.mouselabweb.org/
- Wilson, C., Woloshin, S., & Schwartz, L. M. (2007). Choosing where to have major surgery. Archives of Surgery, 142, 242–246. doi:10.1001/archsurg.142.3.242
- Zwijnenberg, N. C., Hendriks, M., Damman, O. C., Bloemendal, E., Wendel, S., de Jong, J. D., & Rademakers, J. (2012). Understanding and using comparative healthcare information; the effect of the amount of information and consumer characteristics and skills. BMC Medical Informatics and Decision Making, 12, 101. doi:10.1186/1472-6947-12-101
Appendices
The attributes in Appendix A were derived from a comprehensive literature analysis of patient information and decision behavior, and contain relevant formal and informal information cues a patient is likely to use when selecting a hospital. Appendix B discusses why the attributes presented in Appendix A are appropriate attributes for a decision-making experiment in a hospital choice scenario that is based in Germany. Moreover, based on a literature review, in Appendix B we discuss scientific literature related to the preference variables, attributes, and possible utility values. The cues are divided into two categories: Easily accessible formal information on provider characteristics and informal information originating from the patient’s social environment.