Abstract
We examined how two critical constructs, health beliefs and sensation seeking, influence combination antiretroviral therapy adherence in HIV + African Americans, and whether these factors mediate the association between age and adherence. Two hundred and eighty-six HIV + African Americans participated in this observational study. Path analyses revealed that higher levels of a specific health belief, perceived utility of treatment, and lower levels of a sensation seeking component, Thrill and Adventure Seeking, directly predicted optimal adherence. The influence of age on adherence was partially mediated by lower Thrill and Adventure Seeking levels. Depression predicted adherence via perceived utility of treatment and Thrill and Adventure Seeking, whereas current substance abuse and dependence did via Thrill and Adventure Seeking. Poorer neurocognitive function had a direct, adverse effect on adherence. Our findings suggest that supporting the development of more positive perceptions about HIV treatment utility may help increase medication adherence among African Americans. This may be particularly relevant for those with higher levels of depression symptoms, which were directly associated with negative perceptions about treatment. Additionally, clinicians can assess sensation seeking tendencies to help identify HIV + African Americans at risk for suboptimal adherence. Compensatory strategies for medication management may help improve adherence among HIV + individuals with poorer neurocognitive function.
Public Interest Statement
This research sheds light on some of the factors that influence whether HIV + African Americans adhere to their antiretroviral medication regimen. Although it might seem surprising that some HIV + individuals would not adequately adhere to their medication regimens, a number of demographic, sociocultural, cognitive, and psychological factors might explain why this might be. For instance, sociohistorical factors may lead to lower levels of trust in physicians and HIV treatments, particularly in older African Americans, and, thus, suboptimal medication adherence. Our key findings were that younger age, poorer cognitive functioning, depression, substance use, preferences for sensation-eliciting activities, and pessimistic beliefs about HIV treatment predicted suboptimal adherence. Our findings have significant public and individual-level health consequences, as HIV medication adherence is critical to living well with HIV and reducing morbidity and mortality. Improving perceptions about HIV treatment utility may help increase adherence, particularly among African Americans with higher levels of depression symptoms.
Competing interests
The authors declare no competing interest.
1. Introduction
HIV is a mounting health disparity and public health crisis for African Americans (AAs). AAs are disproportionately infected with HIV, constituting nearly half (44%) of the more than 1 million Americans with HIV (Centers for Disease Control and Prevention, [CDC], Citation2013). Advances in the medical treatment of HIV, such as combination antiretroviral therapy (cART), have led to significantly longer life expectancies for those living with HIV. As a result, HIV is becoming increasingly common in older AAs. The prevalence rate of HIV infection among AAs aged 50 years and older is approximately 12 times higher compared to their Caucasian counterparts (CDC, Citation2008).
Older HIV + adults have been shown to better adhere to their medication regimen than younger HIV + adults (e.g. Barclay et al., Citation2007). However, many older HIV + patients face challenges that can contribute to treatment nonadherence, such as co-morbid illnesses (e.g. Roland & Havlir, Citation2003), complex medication regimens, and neurocognitive decline (e.g. Hinkin et al., Citation2002). Older AAs in particular may be at increased risk for poor cART adherence due to socioculturally influenced pessimistic beliefs about HIV treatment. Siegel, Karus, and Schrimshaw (Citation2000) found that HIV + AA men aged 50–67 years were more likely than their Caucasian counterparts to endorse doubt about their physicians’ ability to competently manage their HIV medication regimen. Unfortunately, there is minimal research on factors that are associated with cART adherence in AAs across the life span.
Two additional factors that may influence medication adherence in HIV + populations are health beliefs and sensation seeking. Although health beliefs (e.g. Sayegh & Knight, Citation2012) and sensation seeking (e.g. Szrek, Chao, Ramlagan, & Peltzer, Citation2012) have been shown to predict various health-related behaviors and outcomes in diverse populations, their potential influences on cART adherence have received inadequate attention in HIV + AAs. There has been even less attention to the influence of age on cART adherence in AAs, and whether its influence on adherence is mediated by these factors. Below, we discuss the extant literature on the influence of these and other key variables on HIV medication adherence.
1.1. Health beliefs
Health beliefs have long been studied in the context of various health-related behaviors and outcomes, including those pertaining to HIV (e.g. Barclay et al., Citation2007). The Health Belief Model (HBM; e.g. Rosenstock, Citation1974) was originally developed as a conceptual framework for understanding why people do or do not pursue a wide range of health-related behaviors. The HBM proposes that a person’s resolution to pursue a health-related behavior, such as adhering to cART regimens, is contingent on that person’s perceptions of: (1) susceptibility to the condition, or belief in the credibility of the diagnosis; (2) severity of the condition itself, or not receiving treatment for it; (3) utility of treatment, pertaining to beliefs in the efficacy of various actions (e.g. taking medications) that can reduce disease threat; and (4) barriers to taking action or complying with treatment that may reduce susceptibility to or severity of the condition. Before an individual takes action, the anticipated perceived benefits must outweigh the anticipated perceived barriers.
Few studies have examined the role of health beliefs on cART adherence in HIV + populations. In a racially and ethnically diverse (predominately AA) sample of HIV + males, Durvasula et al. (Citation2002) found that increased levels of perceived utility of treatment (PUT) and intentions to adhere were related to better adherence. On the other hand, perceived barriers to treatment were associated with poorer adherence in HIV + females. A qualitative study by Malcolm, Ng, Rosen, and Stone (Citation2003) found that beliefs in the effectiveness of medication, conceptually similar to PUT, predicted the best levels of cART adherence in a mixed minority sample.
1.2. Age, ethnicity, and health beliefs
Demographic variables including age have direct effects on health beliefs (e.g. Rosenstock, Citation1974) and, consequently, health-related behaviors (e.g. Deeks, Lombard, Michelmore, & Teede, Citation2009). Nonetheless, research on age differences in the effects of health beliefs on cART adherence in HIV + individuals is lacking. Barclay et al. (Citation2007) examined the influence of age on aspects of the HBM and other potentially important predictors of HIV medication adherence in a sample of younger (<50 years old) and older (≥50 years old) HIV + adults. Interestingly, PUT was the only health belief that was significantly associated with poorer cART adherence in the younger sample, whereas poorer neurocognitive function was the sole predictor of suboptimal adherence in the older group.
The lack of reported association between PUT and adherence in older adults in the aforementioned study is somewhat perplexing as PUT is a health belief that is likely more salient among older compared to younger adults. Given the association of increasing age with medical problems, older adults are more likely to have had more beneficial medical treatments themselves (Bernstein et al., Citation2003) and witnessed successful medical treatments in peers compared to younger adults, which may in part reflect increased levels of PUT in addition to their medical vulnerability. Thus, it could be hypothesized that PUT would also predict adherence in older HIV + adults, though this topic has not yet been examined in AA samples. As many older AAs were directly affected by or recall living in a time when unscrupulous clinical studies such as the Tuskegee Syphilis Experiment were conducted on AAs, they may likely have especially lower levels of trust in the health care system and providers (e.g. Guinan, Citation1993). Consequently, they may be more likely to have pessimistic perceptions of cART as being a useful or effective treatment.
1.3. Sensation seeking
Risky behaviors such as injecting drugs/needle-sharing and unprotected sex are strongly associated with HIV infection, and research suggests that those more likely to historically engage in such risky behaviors are less cART adherent (e.g. Wilson et al., Citation2002). Sensation seeking is a trait defined by the pursuit of diverse, new, complex, and intense experiences and sensations as well as the inclination to take physical, legal, social, and monetary risks for the sake of those experiences and sensations (Zuckerman, Citation1994). Numerous studies have reported associations between sensation seeking and risky behaviors, including unsafe sexual behaviors associated with HIV transmission risk (e.g. McCoul & Haslam, Citation2001).
Zuckerman, Eysenck, and Eysenck (Citation1978) operationalized this construct with the Sensation Seeking Scale (SSS). It is composed of four subscales, one of which, Thrill and Adventure Seeking (TAS), was employed in the current study. TAS taps the desire to engage in risky, unusual, and exhilarating activities, regardless of whether they are actually pursued. There is no research to our knowledge on the influence of sensation seeking on cART adherence in HIV + individuals. However, sensation seeking traits have been shown to predict poor medication compliance for other conditions such as depression (Ekselius, Bengtsson, & von Knorring, Citation2000).
1.4. Age, ethnicity, and sensation seeking
Sensation seeking levels have been shown to decline precipitously with age (e.g. Zuckerman et al., Citation1978), a finding that has also been demonstrated in the four SSS subscales, especially TAS. Additionally, sensation seeking has been associated with continued sexual risk behaviors in predominately AA HIV + samples (e.g. Gonzalez et al., Citation2005). Although sensation seeking has been linked with risk-taking behaviors related to HIV transmission, research on the associations between age, sensation seeking, and cART adherence is lacking. It is plausible that individuals with sensation seeking preferences who contract HIV may be at increased risk for poor cART adherence, given the association of sensation seeking tendencies and risky behaviors. Clarifying whether sensation seeking is a risk factor for poor adherence in AAs may help clinicians identify patients who are more likely to exhibit poor cART adherence in this disproportionately HIV-infected population.
1.5. Substance use
Active substance use is known to predict poor cART adherence in HIV-infected individuals. For example, a longitudinal study of predominately HIV + AAs revealed that 93% of participants who met DSM-IV diagnostic criteria for current drug abuse or dependence demonstrated poor adherence compared to 65% of those who did not meet criteria (Hinkin et al., Citation2004). Substance use can indirectly influence adherence through associated neurocognitive decline (Volkow et al., Citation2001), psychosocial deficits (Reback, Larkins, & Shoptaw, Citation2003), and worsening of psychiatric problems (Hinkin, Castellon, Atkinson, & Goodkin, Citation2001). Substance use has also been frequently associated with sensation seeking (e.g. McCoul & Haslam, Citation2001) and may also result in negativistic health beliefs regarding HIV treatment benefits. In summary, current substance use disorders (SUDs) appear to be a key predictor of cART adherence through several mechanisms, including health beliefs and sensation seeking.
1.6. Depression
Depression is the most common neuropsychiatric syndrome in HIV + individuals and is nearly twice as common in this group compared to HIV-negative individuals (Ciesla & Roberts, Citation2001). The influence of depression on both poorer adherence to and earlier cessation of cART treatment is generally well established (e.g. Gonzalez, Batchelder, Psaros, & Safren, Citation2011). However, some studies have not found a relation between these variables (e.g. Barclay et al., Citation2007). One possible explanation for this inconsistency is that the influence of depression on cART adherence may be mediated by other variables that are not always assessed in studies, including health beliefs and sensation seeking. Specifically, depression is associated with hopelessness, negative thoughts, and apathy, which may result in pessimistic health beliefs regarding treatment utility and thus poorer cART adherence. In addition, the sad mood, fatigue, anhedonia, and diminished energy associated with depression may be negatively associated with sensation seeking preferences, which may in turn predict cART adherence.
1.7. Neurocognition
Neurocognition plays a key role in cART adherence in HIV-infected individuals, particularly in older adults. Prior studies have demonstrated that lower levels of neurocognitive function (e.g. Barclay et al., Citation2007) and neurocognitive impairment (i.e. t score < 40; Hinkin et al., Citation2002) are often the strongest predictor of reduced cART adherence in older HIV + adults. Furthermore, the association between neurocognition and cART adherence has been shown to be reciprocal in that lower levels of neurocognitive functioning lead to poorer cART adherence, and poorer adherence often results in declines in neurocognition (Ettenhofer, Foley, Castellon, & Hinkin, Citation2010). Research has not yet focused on the influence of neurocognition on cART adherence in older, HIV + participants of AA background specifically.
1.8. Hypotheses
In summary, cART adherence in HIV + populations is clearly complex and multifactorial in nature, with age, substance use, depression, neurocognition, health beliefs, and sensation seeking all representing likely contributors to adherence. As much of the prior research in this area has focused on few or even individual predictors, we sought to model the simultaneous influence of the combination of the abovementioned variables on cART adherence in a sample of HIV + AAs across the lifespan. Figure provides an illustration of our hypothesized model and depicts the predicted associations among the variables of interest in our study.
Figure 1. Hypothesized path model of predictors of combination antiretroviral therapy adherence in HIV-positive African Americans.
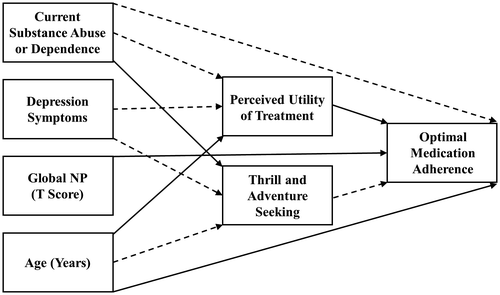
Consistent with prior work, we hypothesized that: (1) health beliefs (e.g. higher levels of PUT), lower sensation seeking levels, older age, the absence of current SUDs, and better overall neurocognitive functioning would have direct effects on optimal cART adherence. For our key hypotheses, we anticipated the following mediation effects: (2) the influence of increasing age on optimal cART adherence would be partially mediated by both HIV-related health beliefs and (3) lower sensation seeking levels; (4) among participants with current SUDs, health beliefs related to lower PUT levels and higher sensation seeking levels would predict poor cART adherence; and (5) depression would predict poor adherence via both health beliefs and sensation seeking.
2. Materials and methods
2.1. Participants
We recruited 286 AA female and male HIV + participants aged 18 years or older from 15 Greater Los Angeles (13 Los Angeles County, 1 Orange County, and 1 Riverside County) community agencies specialized in the provision of service to HIV + individuals through the use of fliers posted in university-affiliated medical center infectious disease clinics. Those who expressed interest in participation were referred to study personnel for screening and possible enrollment. To be eligible for the study, participants had to be prescribed self-administered highly active cART for HIV. Exclusion criteria included history of traumatic brain injury with loss of consciousness exceeding 60 min, active seizure disorders, other non-HIV-related neurological diseases, psychotic spectrum disorders, and HIV-associated central nervous system opportunistic infections and neoplasm. For the current study, only individuals with self-reported AA ethnicity were included in the analyses. Table presents demographic and other characteristics of this study’s sample.
Table 1. Descriptive statistics of participants and key variables
2.2. Procedure
Institutional review board panels at the University of California, Los Angeles and Veterans Administration, Greater Los Angeles Healthcare System approved all research methods and procedures. We obtained written informed consent from all participants via a consent form and a verbal summary of the key points from the consent form by study personnel, after which we invited participants to have any questions or concerns addressed. Participants completed the study protocol in-person at the research team’s offices. Trained and supervised psychometrists conducted the psychiatric interviews, neuropsychological testing, and questionnaire administration (in paper-and-pencil format) and provided participants with instruction on how to have their medication adherence tracked for this study (described below). At the one-month follow-up session, when we obtained medication adherence information, participants received $80 in payment for participation in this portion of the study.
2.3. Measures
2.3.1. Exogenous variables
We included age (in years) as a key exogenous demographic variable to determine how health beliefs and sensation seeking may mediate its role in cART adherence. We assessed for current (i.e. past 12 months) substance abuse and dependence (yes or no) using the corresponding modules from the Structured Clinical Interview for the DSM-IV. Trained psychometrists conducted the interviews under the supervision of a licensed clinical psychologist. We operationalized depression symptoms over the past 2 weeks as total scores (range: 0–63) from the self-administered, 21-item Beck Depression Inventory-II (BDI-II; Beck, Steer, & Brown, Citation1996). Participants rated each symptom on a scale of 0–3, with higher scores indicative of greater severity of depressive symptomatology. Numerous studies have provided evidence for this measure’s excellent reliability and ability to adequately distinguish individuals with depression from those without depression (e.g. Beck et al., Citation1996), including in AA patients (e.g. Dutton et al., Citation2004). In terms of internal consistency reliability, Cronbach’s alpha (α) for the BDI-II in this sample was 0.91.
Additionally, trained psychometrists administered neuropsychological tests under the supervision of a board-certified neuropsychologist. Participants completed one of two similar fixed neuropsychological test batteries (Battery 1 [n = 127] and Battery 2 [n = 159]) assessing a total of six domains (information processing speed, learning and memory, verbal fluency, attention and working memory, executive function, and motor function). Although there were slight variations across the batteries (e.g. first vs. second edition of one test), any nonoverlapping tests were very similar and primarily tapped the same main neurocognitive function. See Ettenhofer et al. (Citation2009) for the tests included in each battery and normative data sources.
We calculated demographically corrected t scores (M = 50, SD = 10) from raw test scores using published normative data. We calculated a global neurocognitive t score by adding the individual test t scores and dividing this sum by the number of tests administered (see Heaton et al., Citation1995 for a detailed description of this approach).
2.3.2. Mediating variables
We measured health beliefs with the Adherence Determination Questionnaire (ADQ; DiMatteo et al., Citation1993), which is based on the HBM and consists of 38 short statements rated on a 1 (strongly disagree) to 5 (strongly agree) Likert scale. Seven subscales are derived from this measure, which include: (1) interpersonal aspects of care and relationship with treating professionals; (2) PUT; (3) perceived severity of illness; (4) perceived susceptibility to illness progression; (5) subjective norms (i.e. belief that family and friends support treatment plan adherence); (6) intentions to comply with treatment; and (7) barriers to and support for treatment. As the ADQ is multidimensional, we examined the bivariate correlations of each subscale with our cART adherence outcome to assist us with determining which subscale(s) to include in our model. Only three of the seven subscales (PUT, intentions to comply with treatment, and barriers to and support for treatment) had statistically significant (p < 0.05) correlations with adherence. PUT was most strongly correlated with the adherence outcome variable (r = 0.18, p < 0.01) based on both the magnitude of its correlation coefficient and its significance level. Furthermore, PUT has also been shown in other studies to be important in cART adherence (e.g. Barclay et al., Citation2007; Durvasula et al., Citation2002). Therefore, we included this particular health belief in our analyses. This subscale is derived from a total of eight items, which include, “My treatment plan is too much trouble for what I get out of it” and “I’ll be just as healthy if I avoid my treatment plan.” Corcoran and Fischer (Citation2013) reported that the validity of this scale has been found to be fair to good in several studies based on its subscales’ associations with other adherence characteristics. They also stated that other studies have reported fair to good internal consistency reliability, with α values ranging from 0.63 to 0.94 and a median α of 0.76. The α values in this sample were 0.78 for the entire ADQ and 0.81 for the PUT subscale.
We measured sensation seeking and its four component subscales with the SSS Form V (Zuckerman et al., Citation1978), a 40-item, forced-choice format scale whose constructs are presumed to contribute to risk preferences. Form V of the SSS represents an updated version of the scale in which several items that had become dated or irrelevant to current generations were modified. We ultimately selected the TAS subscale for inclusion in our analyses primarily based on its statistically significant bivariate correlation (r = −0.15, p = 0.02) with optimal cART adherence in this sample in contrast to the other subscales and total SSS scores. In addition, TAS is viewed as highly predictive of risk-taking behaviors in other areas (e.g. Zuckerman, Citation1994), including risky and exhilarating behaviors that may likely be related to HIV-related risk behaviors. Furthermore, TAS has recently been shown to predict risk-taking preferences using both behavioral and neuroimaging measures (Kruschwitz, Simmons, Flagan, & Paulus, Citation2012). Finally, the TAS subscale in particular has been demonstrated to show age-associated declines (e.g. Zuckerman et al., Citation1978), and mediators of the role of age in adherence were a key focus of this study. Of note, although prior studies have found gender differences in sensation seeking (e.g. Zuckerman, Citation1994), with men generally reporting higher levels, gender was not significantly correlated with sensation seeking in the current study and was thus not included as a predictor of TAS in our model. The TAS subscale is defined by 10 items indicating a desire to engage in risky and exhilarating activities with items including, “I often wish I could be a mountain climber” and “I would like to try parachute jumping.” The construct validity and reliability of the SSS Form V have been well established (see Zuckerman, Citation1994 for a review). The α values in this sample were 0.71 for the entire SSS scale and 0.67 for the TAS subscale.
2.3.3. Dependent variable
We objectively measured HIV medication adherence over approximately 30 days using the Medication Event Monitoring System (MEMS), which employs a pressure-activated microprocessor in the medication bottle cap to automatically record the time, date, and duration of bottle opening. We downloaded this information from the bottle cap to a computer after approximately 1 month. To determine which specific medications’ adherence rates would be tracked using the MEMS, we gave priority to protease inhibitors, followed by nucleoside reverse transcriptase inhibitors, nonnucleoside reverse transcriptase inhibitors, and nucleotide reverse transcriptase inhibitors. We calculated medication adherence, as derived from the MEMS data, as percent of doses taken in relation to total number of prescribed doses. To optimize the accuracy of MEMS data, we instructed participants to open the MEMS cap only when taking a dose, refill the bottle at a time when they usually took a dose, and not utilize pill boxes or remove multiple pills at one time for later ingestion. At the one-month follow-up, study personnel had the opportunity to ask participants if they ever took the designated medication without the use of the MEMS bottle, and corrections were made accordingly. Additionally, to account for the possibility of bottle openings that were unlikely to be associated with an actual dosing event, we adjusted MEMS data as follows: only a single MEMS cap opening was tallied for every 2-h period; the number of MEMS cap openings was reduced as needed to the total number of prescribed daily doses; and MEMS cap openings conducted by the research staff for administrative reasons were not counted. We operationalized optimal medication adherence as ≥ 90% adherent over the one-month study period and poor adherence as < 90% adherent over this time, consistent with prior studies on cART adherence (e.g. Hinkin et al., Citation2007). The one-month timeframe for tracking adherence has been used in several prior HIV studies (e.g. Barclay et al., Citation2007; Hinkin et al., Citation2002).
2.4. Statistical method
To assess the multivariate normality of the data, we first evaluated the data for univariate skewness and kurtosis, with skew > 3 and kurtosis > 10, suggestive of nonnormality (Kline, Citation2013). We then tested the data for multivariate kurtosis, a critically important assumption of structural equation modeling. Bentler (Citation2005) stated that Mardia’s normalized estimate of multivariate kurtosis values > 5 are suggestive of nonnormally distributed data. Finally, we assessed for multivariate outliers based on examination of Mahalanobis distance (D2) values. Byrne (Citation2010) indicated that outliers will have D2 values that stand distinctively apart from other D2 values.
As previously noted, we examined the statistical significance of the bivariate correlations between the key mediating variables of interest (i.e. health beliefs and the SSS total and subscale scores) and cART adherence to help us determine which specific mediating variables from the multidimensional ADQ and SSS scales to examine. We tested our path models in AMOS 17.0 using Markov chain Monte Carlo methods, given the binary nature of the dependent variable (Byrne, Citation2010). We examined the statistical significance of estimated path coefficients and various goodness-of-fit statistics for the models as a whole, including χ2 to degrees of freedom ratio (χ2/df), comparative fit index (CFI), and root mean square error of approximation (RMSEA). Models with a better fit generally have higher CFI and lower χ2/df and RMSEA values. Adequate fit was indicated by CFI values greater than 0.95 and RMSEA values less than 0.06 (e.g. Schreiber, Nora, Stage, Barlow, & King, Citation2006). In addition, posterior predictive P values around 0.50 are suggestive of a plausible model, whereas values toward the extremes of 0 or 1 indicate an implausible model (Lee & Song, Citation2003). If models demonstrated adequate fit yet had nonsignificant path coefficients, we deleted nonsignificant paths in the interest of parsimony and assessed for changes in fit before accepting the reduced models as the final models (Byrne, Citation2010). To test for significant changes in fit, we assessed for statistically significant (p < 0.05) Δχ2 difference values (Byrne, Citation2010), ΔCFI values greater than 0.01 (Cheung & Rensvold, Citation2002), and negative Δdeviance information criterion (DIC) values (Spiegelhalter, Best, Carlin, & van der Linde, Citation2002). Finally, to evaluate the adequacy of our sample size, we examined Hoelter’s (Citation1983) critical N (CN) for the 0.05 level, with values greater than 200 suggestive of a large enough sample and values below 75 deemed unacceptably low.
3. Results
Table presents descriptive statistics for the key variables of interest. The data did not show evidence of univariate skewness or kurtosis or multivariate kurtosis (Mardia’s normalized estimate of multivariate kurtosis = −0.56). In addition, we did not find evidence of multivariate outliers based on examination of D2 values.
Our first hypothesized path model had excellent model fit statistics (χ2/df = 0.83, CFI = 1.00, RMSEA = 0.00, RMSEA 90% confidence interval [CI; 0.00, 0.06], posterior predictive p = 0.50, and DIC = 6,052.57) and was suggestive of sufficient sample size (CN = 669). We then estimated a reduced model after deleting nonsignificant paths from the originally proposed model in the interest of parsimony. The three deleted nonsignificant paths were: (1) current SUDs PUT; (2) current SUDs optimal medication adherence; and (3) age PUT. The fit statistics of the reduced, final model also suggested that the sample size was adequate (CN = 512) and that the data fit the model very well (χ2/df = 1.00, CFI = 1.00, RMSEA = 0.00, RMSEA 90% CI [0.00, 0.06], posterior predictive p = 0.50, and DIC = 6,050.92). The fit statistics did not significantly differ from those of the first model (Δχ2: p = 0.23; ΔCFI = 0.00; ΔDIC = −1.65).
Figure illustrates the final path model along with standardized path coefficients (β).
Figure 2. Final path model (with standardized path coefficients) of predictors of combination antiretroviral therapy adherence in HIV-positive African Americans.
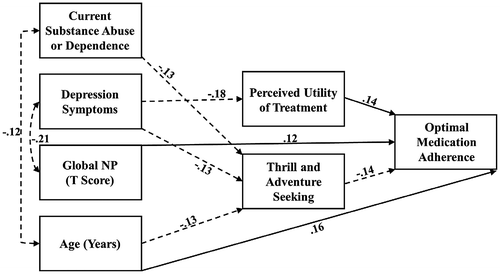
Table presents the unstandardized regression coefficients for both models.
Table 2. Unstandardized regression coefficients estimated from fully recursive first and reduced path models predicting optimal medication adherence (N = 286)
Results from the final model provided Hypothesis 1 with mixed support. As anticipated, higher levels of PUT and lower levels of TAS had direct effects on optimal adherence (PUT Optimal Medication Adherence: β = 0.14; and TAS Optimal Medication Adherence: β = −0.14). Additionally, increasing age and better neuropsychological test performance both had direct effects on optimal cART adherence (Age [Years] Optimal Medication Adherence: β = 0.16; and Global Neuropsychological Test Performance [t score] Optimal Medication Adherence: β = 0.12). However, current SUDs did not directly influence adherence.
In terms of our key hypotheses, Hypothesis 2 was not supported, as the influence of age on cART adherence was not mediated by PUT (i.e. no path from age PUT). However, Hypothesis 3 was supported. The influence of age on adherence was partially mediated by TAS (Age [years] TAS: β = −0.13; and TAS Optimal Medication Adherence: β = −0.14). Specifically, increasing age predicted lower levels of TAS, and lower TAS levels were related to optimal adherence. Regarding Hypothesis 4, the influence of current SUDs on cART adherence was fully mediated by TAS as anticipated but not PUT, with SUDs being negatively associated with TAS (Current SUDs TAS: β = −0.13; and TAS Optimal Medication Adherence: β = −0.14). Finally, as anticipated (Hypothesis 5), the role of depression symptoms in adherence was fully mediated by both PUT and TAS (Depression Symptoms PUT: β = −0.18; and PUT Optimal Medication Adherence: β = 0.14; Depression Symptoms to TAS: β = −0.13; and TAS Optimal Medication Adherence: β = −0.14). Higher levels of depressive symptomatology were related to lower PUT and TAS levels, which were in turn associated with cART adherence.
4. Discussion
The primary aim of the current study was to determine whether the influence of increasing age on optimal cART adherence is mediated by treatment-specific health beliefs (i.e. PUT) and sensation seeking (i.e. TAS) in a sample of HIV + AAs. This study examined the concomitant potential effects of age, health beliefs, and sensation seeking on adherence. Although age was not associated with health beliefs (i.e. PUT) in our model, findings from this study add to the extant literature on the benefits of increasing age on cART adherence by highlighting the partially mediating role of sensation seeking in this association among AAs. Sensation seeking (and TAS in particular) is known to decrease with age (e.g. Zuckerman et al., Citation1978). In this study, the decrease in TAS associated with increasing age was related to better adherence. The influence of sensation seeking on adherence has also been understudied in the literature, and our findings suggest both that this construct may serve as an important indicator for cART adherence in HIV + AAs and that age differences are present in this association.
In line with the HBM, our results showed that higher PUT levels directly predicted better cART adherence. In the absence of beliefs about the benefits of treatment, individuals would be unlikely to adhere to treatment. However, we did not find that PUT had a mediating effect on the relation between age and adherence. This finding is somewhat consistent with the findings of Barclay and colleagues (Citation2007) who also failed to find a significant predictive role of PUT on adherence in their sample of HIV + adults aged 50 years and older. Age is posited to have an influence on various HBM components (e.g. Rosenstock, Citation1974) such that increasing age may be associated with higher PUT levels. However, it is possible that older AAs, who lived during an era in which unethical, experimental studies were conducted on individuals in their ethnic group, may harbor higher levels of suspiciousness and mistrust of the health care system (e.g. Guinan, Citation1993) and may thus be less likely to perceive medications including cART as useful. They may also consequently have lower levels of trust in and satisfaction with their health care providers and prescribed medications, which have both been associated with cART adherence (e.g. Siegel et al., Citation2000). Therefore, the role of increasing age on PUT among HIV + AAs may be attenuated by these kinds of factors. Although these data do not include information on the ethnic background of the medication-prescribing medical providers, it is plausible that the majority of participants were prescribed cART by Caucasian providers. The vast majority (approximately 73.7%) of medical providers in the US identified as Caucasian in 2008. In contrast, only 3.8% of physicians identified as AA (Boukus, Cassil, & O’Malley, Citation2009).
We anticipated the findings regarding the influence of depression on cART adherence, as depression is associated with symptoms that may lead to diminished or maladaptive views of treatment utility, such as apathy, hopelessness, and cognitive distortions. Additionally, the anhedonia, fatigue, and reduced energy levels often seen in depressive disorders would likely be linked with lower TAS levels, as found in this study. This finding provides some clarification to the mixed findings in the literature regarding the effects of depression on cART adherence. While some of the disparity in findings across studies may be due to methodological and sample differences, it may be that variables that mediate the relationship between depression and adherence such as PUT and sensation seeking have not been previously examined.
We did not anticipate that current SUDs would be associated with lower TAS levels. One possible explanation for this finding is that sensation seeking likely plays a more salient role in the earlier stages of substance use when the resulting intoxication is new and exciting (Zuckerman, Citation1983). This finding suggests that sensation seeking is associated with occasional or experimental substance use, as well as the initiation versus maintenance of use. For example, both present and former smokers have been shown to have similar sensation seeking levels, and sensation seeking levels differ only between those who never smoked versus those who smoked to any degree (e.g. von Knorring & Oreland, Citation1985). This finding is concordant with the notion that sensation seeking may affect the adoption of substance use but does not necessarily directly relate to active SUDs. Furthermore, with regard to TAS, the items composing this scale tap activities that generally require motivation, energy, physical fitness, and stamina. In retrospect, it is not surprising that individuals with current clinical diagnoses of SUDs may no longer endorse TAS subscale items to pre-SUD levels, especially for those with current substance dependence. We should note that several possible reasons other than sensation seeking may lead to substance use initiation, particularly in populations at great risk for substance use as a coping strategy in the face of exposure to a wide array of discriminatory and traumatic experiences.
Our findings have several clinical implications. For example, clinicians may identify HIV + AAs at risk for poorer adherence by examining patients’ PUT regarding cART and TAS preferences and intervene with these individuals by, for example, providing psychoeducation about the benefits of their medications. Psychoeducation should be provided in a culturally sensitive fashion, as some individuals, especially older AAs, may have less trust of the health care system and providers (e.g. Guinan, Citation1993). Regarding age, it appears that younger AA individuals, and particularly those with higher TAS preferences, might also be at increased risk of poor cART adherence and may benefit from similar interventions. Individuals with higher levels of TAS may be identified through the use of the SSS, which is a relatively fast, inexpensive, patient-administered tool in comparison to other measures of risk-taking (e.g. computerized gambling tasks). To increase cART adherence in HIV + AAs with depression, clinicians may benefit from conducting interventions aimed at helping patients reframe negative or maladaptive thoughts about PUT. Clinical interventions may also target depressive symptoms that are likely linked with lower levels of PUT (e.g. hopelessness, negative thought patterns, and apathy). Cognitive-behavioral therapy may be particularly well suited to help individuals reframe negative thoughts about treatment utility and thereby improve adherence. Finally, HIV + individuals with poorer neurocognitive function, particularly in the domains of memory, executive function, and attention, would likely benefit from compensatory strategies to assist them with cART management and adherence, such as the use of pill boxes, reminder systems, or the assistance of others.
Although this sample was partially composed of HIV + AAs with current SUDs, this characteristic may in fact be representative of the larger HIV + population. The percentage of individuals in our study who met diagnostic criteria for current SUDs (25.99%) is nearly identical to national estimates in the larger US HIV/AIDS population (23.9%; Substance Abuse & Mental Health Services Administration, Center for Behavioral Health Statistics & Quality, Citation2010). SUDs are common in this group in part as a result of the relation between injection drug use and HIV transmission. As such, these findings are likely generalizable to other HIV + AAs. Adding to the generalizability of our findings is the inclusion of older HIV + AA adults (aged 50 years and older), who constitute a rapidly growing number of individuals in this population living with HIV (CDC, Citation2008). Limiting the generalizability of our findings are the constricted geographic location from which the study sample was recruited (i.e. Greater Los Angeles) and the relatively low annual household income of the sample. Future research should examine predictors of cART adherence in AAs using more geographically, socioeconomically, and ethnically diverse samples. This sample was also primarily composed of men (78.70%). Nonetheless, these findings may very well generalize to AA females, as the percentage of women in our sample exceeded the estimated base rate (11%) of women living with HIV in Los Angeles County (Division of HIV & STD Programs, Los Angeles County Department of Public Health, Citation2012).
We also highlight that these data are cross-sectional in nature, and we cannot make conclusions regarding causality. Additionally, we lacked statistical power to test for group differences in younger versus older adults, given the relatively small number of participants older than 50 years (n = 52). Finally, it was infeasible to include other known predictors of medication adherence both in the interest of parsimony and due to limitations on statistical power.
In summary, we found that age is protective against poor cART adherence among HIV + AAs, and this relationship is partially mediated by declines in TAS associated with older age. We also demonstrated that higher PUT and lower TAS levels directly predicted better adherence. Future research on other possible mediators of the association between age and cART adherence among diverse groups is warranted, given the graying of the ethnically diverse HIV + population.
Cover image
Source: Authors.
Additional information
Funding
Notes on contributors
Philip Sayegh
Philip Sayegh’s program of research focuses on the intersection of aging, culture, and cognition, with an emphasis on neuromedical illnesses and health behaviors and outcomes in ethnic minorities. His prior research has examined how cultural values and the stress and coping process affect the mental and physical health of family dementia caregivers, as well as cultural influences on the assessment and diagnosis of dementia across ethnic groups. The research reported in this paper is directly related to his broader research program. It examines the influence of age and neurocognitive, psychological, and culturally influenced predictors of an important health behavior, antiretroviral medication adherence, among HIV + African Americans. All co-authors have research interests in a wide range of HIV-related issues, including HIV-risk reduction, the neurocognitive, neurobehavioral, and neuroanatomical sequelae of HIV infection, predictors of medication adherence (e.g. substance use, psychopathology, age, and regimen complexity), and ethnic disparities in HIV treatment.
References
- Barclay, T. R., Hinkin, C. H., Castellon, S. A., Mason, K. I., Reinhard, M. J., Marion, S. D., … Durvasula, R. S. (2007). Age-associated predictors of medication adherence in HIV-positive adults: Health beliefs, self-efficacy, and neurocognitive status. Health Psychology, 26, 40–49. doi:10.1037/2F0278-6133.26.1.40
- Beck, A. T., Steer, R., & Brown, G. K. (1996). Beck depression inventory-II (BDI-II) manual (2nd ed.). San Antonio, TX: Psychological Corporation.
- Bentler, P. M. (2005). EQS 6 structural equations program manual. Encino, CA: Multivariate Software.
- Bernstein, A. B., Hing, E., Moss, A. J., Allen, K. F., Siller, A. B., & Tiggle, R. B. (2003). Health care in America: Trends in utilization. Hyattsville, MD: National Center for Health Statistics. Retrieved from http://www.cdc.gov/nchs/data/misc/healthcare.pdf
- Boukus, E., Cassil, A., & O’Malley, A. S. (2009). A snapshot of US physicians: Key findings from the 2008 health tracking physician survey. Washington, DC: Center for Studying Health System Change. Retrieved from http://www.hschange.com/CONTENT/1078/
- Byrne, B. M. (2010). Structural equation modeling with AMOS: Basic concepts, applications, and programming (2nd ed.). New York, NY: Taylor & Francis Group.
- Centers for Disease Control and Prevention. (2008). HIV/AIDS among persons aged 50 and older [Website]. Retrieved from http://www.cdc.gov/hiv/pdf/library_factsheet_HIV_among_PersonsAged50andOlder.pdf
- Centers for Disease Control and Prevention. (2013). HIV in the United States: At a glance [Website]. Retrieved from http://www.cdc.gov/hiv/statistics/basics/ataglance.html
- Cheung, G. W., & Rensvold, R. B. (2002). Evaluating goodness-of-fit indexes for testing measurement invariance. Structural Equation Modeling: A Multidisciplinary Journal, 9, 233–255. doi:10.1207/S15328007SEM0902_5
- Ciesla, J. A., & Roberts, J. E. (2001). Meta-analysis of the relationship between HIV infection and risk for depressive disorders. The American Journal of Psychiatry, 158, 725–730. doi:10.1176/2Fappi.ajp.158.5.725
- Corcoran, K., & Fischer, J. (2013). Measures for clinical practice and research, Volume 2: A sourcebook: Adults (5th ed.). New York, NY: Oxford University Press.
- Deeks, A., Lombard, C., Michelmore, J., & Teede, H. (2009). The effects of gender and age on health related behaviors. BMC Public Health, 9, 213. doi:10.1186/1471-2458-9-213
- DiMatteo, M. R., Hays, R. D., Gritz, E. R., Bastani, R., Crane, L., Elashoff, R., … Marcus, A. (1993). Patient adherence to cancer control regimens: Scale development and initial validation. Psychological Assessment, 5, 102–112. doi:10.1037/1040-3590.5.1.102
- Division of HIV and STD Programs, Los Angeles County Department of Public Health. (2012). 2012 Annual HIV surveillance report [Website]. Retrieved from http://publichealth.lacounty.gov/wwwfiles/ph/hae/hiv/2012AnnualHIVSurveillanceReport.pdf
- Durvasula, R. S., Mason, K. I., Thrasher, D., Ropacki, M., Farchione, T., Stefaniak, M., … Hinkin, C. H. (2002, July). Differential predictors of HAART adherence as a function of gender. Poster session presented at the Proceedings of the 14th World AIDS Conference, Barcelona. Abstract No. WePeB5823.
- Dutton, G. R., Grothe, K. B., Jones, G. N., Whitehead, D., Kendra, K., & Brantley, P. J. (2004). Use of the Beck depression inventory-II with African American primary care patients. General Hospital Psychiatry, 26, 437–442. doi:10.1016/j.genhosppsych.2004.06.002
- Ekselius, L., Bengtsson, F., & von Knorring, L. (2000). Non-compliance with pharmacotherapy of depression is associated with a sensation seeking personality. International Clinical Psychopharmacology, 15, 273–278. doi:10.1097/00004850-200015050-00004
- Ettenhofer, M. L., Foley, J., Castellon, S. A., & Hinkin, C. H. (2010). Reciprocal prediction of medication adherence and neurocognition in HIV/AIDS. Neurology, 74, 1217–1222. doi:10.1212/WNL.0b013e3181d8c1ca
- Ettenhofer, M. L., Hinkin, C. H., Castellon, S. A., Durvasula, R., Ullman, J., Lam, M., … Foley, J. (2009). Aging, neurocognition, and medication adherence in HIV infection. American Journal of Geriatric Psychiatry, 17, 281–290. doi:10.1097/JGP.0b013e31819431bd
- Gonzalez, J. S., Batchelder, A. W., Psaros, C., & Safren, S. A. (2011). Depression and HIV/AIDS treatment nonadherence: A review and meta-analysis. Journal of Acquired Immune Deficiency Syndromes, 58, 181–187. doi:10.1097/QAI.0b013e31822d490a
- Gonzalez, R., Vassileva, J., Bechara, A., Grbesic, S., Sworowski, L., Novak, R. M., … Martin, E. M. (2005). The influence of executive functions, sensation seeking, and HIV serostatus on the risky sexual practices of substance-dependent individuals. Journal of the International Neuropsychological Society, 11, 121–131. doi:10.1017/S1355617705050186
- Guinan, M. E. (1993). Black communities’ belief in “AIDS as genocide:” A barrier to overcome for HIV prevention. Annals of Epidemiology, 3, 193–195. doi:10.1016/1047-2797(93)90136-R
- Heaton, R. K., Grant, I., Butters, N., White, D. A., Kirson, D., Atkinson, J. H., … Abramson, I. (1995). The HNRC 500—Neuropsychology of HIV infection at different disease stages. Journal of the International Neuropsychological Society, 1, 231–251. doi:10.1017/S1355617700000230
- Hinkin, C. H., Barclay, T. R., Castellon, S. A., Levine, A. J., Durvasula, R. S., Marion, S. D., … Longshore, D. (2007). Drug use and medication adherence among HIV-1 infected individuals. AIDS and Behavior, 11, 185–194. doi:10.1007/s10461-006-9152-0
- Hinkin, C. H., Castellon, S. A., Atkinson, J. H., & Goodkin, K. (2001). Neuropsychiatric aspects of HIV infection among older adults. Journal of Clinical Epidemiology, 54, S44–S52. doi:10.1016/S0895-4356(01)00446-2
- Hinkin, C. H., Castellon, S. A., Durvasula, R. S., Hardy, D. J., Lam, M. N., Mason, K. I., & Stefaniak, M. (2002). Medication adherence among HIV+ adults: Effects of cognitive dysfunction and regimen complexity. Neurology, 59, 1944–1950. doi:10.1212/2F01.WNL.0000038347.48137.67
- Hinkin, C. H., Hardy, D. J., Mason, K. I., Castellon, S. A., Durvasula, R. S., Lam, M. N., & Stefaniak, M. (2004). Medication adherence in HIV-infected adults: Effect of patient age, cognitive status, and substance abuse. AIDS, 18, S19–S25. doi:10.1097/00002030-200401001-00004
- Hoelter, J. W. (1983). The analysis of covariance structures: Goodness-of-fit indices. Sociological Methods & Research, 11, 325–344. doi:10.1177/0049124183011003003
- Kline, R.B. (2013). Principles and practice of structural equation modeling (2nd ed., pp. 46–74). New York, NY: Guilford.
- Kruschwitz, J. D., Simmons, A. N., Flagan, T., & Paulus, M. P. (2012). Nothing to lose: Processing blindness to potential losses drives thrill and adventure seekers. NeuroImage, 59, 2850–2859. doi:10.1016/j.neuroimage.2011.09.048
- Lee, S. Y., & Song X. Y. (2003). Bayesian analysis of structural equation models with dichotomous variables. Statistics in Medicine, 22, 3073–3088. doi:10.1002/sim.1544
- Malcolm, S. E., Ng, J. J., Rosen, R. K., & Stone, V. E. (2003). An examination of HIV/AIDS patients who have excellent adherence to HAART. AIDS Care, 15, 251–261. doi:10.1080/0954012031000068399
- McCoul, M. D., & Haslam, N. (2001). Predicting high risk sexual behaviour in heterosexual and homosexual men: The roles of impulsivity and sensation seeking. Personality and Individual Differences, 31, 1303–1310. doi:10.1016/2FS0191-8869/2800/2900222-1
- Reback, C. J., Larkins, S., & Shoptaw, S. (2003). Methamphetamine abuse as a barrier to HIV medication adherence among gay and bisexual men. AIDS Care, 15, 775–785. doi:10.1080/09540120310001618621
- Roland, M. E., & Havlir, D. V. (2003). Responding to organ failure in HIV-infected patients. The New England Journal of Medicine, 348, 2279–2281. doi:10.1056/NEJMp030074
- Rosenstock, I. M. (1974). Historical origins of the health belief model. Health Education & Behavior, 2, 328–335. doi:10.1177/109019817400200403
- Sayegh, P., & Knight, B. G. (2012). Cross-cultural differences in dementia: The Sociocultural Health Belief Model. International Psychogeriatrics, 25, 517–530. doi:10.1017/2FS104161021200213X
- Schreiber, J. B., Nora, A., Stage, F. K., Barlow, E. A., & King, J. (2006). Reporting structural equation modeling and confirmatory factor analysis results: A review. The Journal of Educational Research, 99, 323–337. doi:10.3200/JOER.99.6.323-338
- Siegel, K., Karus, D., & Schrimshaw, E. W. (2000). Racial differences in attitudes toward protease inhibitors among older HIV-infected men. AIDS Care: Psychological and Socio-medical Aspects of AIDS/HIV, 12, 423–434. doi:10.1080/2F09540120050123828
- Spiegelhalter, D. J., Best, N. G., Carlin, B. P., & van der Linde, A. (2002). Bayesian measures of model complexity and fit. Journal of the Royal Statistical Society: Series B (Statistical Methodology), 64, 583–639. doi:10.1111/1467-9868.00353
- Substance Abuse and Mental Health Services Administration, Center for Behavioral Health Statistics and Quality. (2010). The NSDUH report: HIV/AIDS and substance use [Website]. Retrieved from http://www.samhsa.gov/data/2k10/HIV-AIDS/HIV-AIDs.htm
- Szrek, H., Chao, L.-W., Ramlagan, S., & Peltzer, K. (2012). Predicting (un)healthy behavior: A comparison of risk-taking propensity measures. Judgment and Decision Making, 7, 716–727. PMCID:PMC3846348
- Volkow, N. D., Chang, L., Wang, G. J., Fowler, J. S., Leonido-Yee, M., Franceschi, D., … Miller, E. N. (2001). Association of dopamine transporter reduction with psychomotor impairment in methamphetamine abusers. The American Journal of Psychiatry, 158, 377–382. doi:10.1176/appi.ajp.158.3.377
- von Knorring, L., & Oreland, L. (1985). Personality traits and platelet monoamine oxidase in tobacco smokers. Psychological Medicine, 15, 327–334. doi:10.1017/S0033291700023606
- Wilson, T. E., Barrón, Y., Cohen, M., Richardson, J., Greenblatt, R., Sacks, H. S., & Young, M. (2002). Adherence to antiretroviral therapy and its association with sexual behavior in a national sample of women with human immunodeficiency virus. Clinical Infectious Diseases, 34, 529–534. doi:10.1086/338397
- Zuckerman, M. (1983). Sensation seeking: The initial motive for drug abuse. In E. H. Gottheil, K. A. Druley, T. E. Skoloda, & H. M. Waxman (Eds.), Etiological aspects of alcohol and drug abuse (pp. 202–220). Springfield, IL: Charles C. Thomas.
- Zuckerman, M. (1994). Behavioral expressions and biosocial bases of sensation seeking. New York, NY: Cambridge University Press.
- Zuckerman, M., Eysenck, S. B. J., & Eysenck, H. J. (1978). Sensation seeking in England and America: Cross-cultural, age, and sex comparisons. Journal of Consulting and Clinical Psychology, 46, 139–149. doi:10.1037/0022-006X.46.1.139