Abstract
The Internet permits students to share course evaluations with millions of people, and recent research suggests that students who read these evaluations form expectations about instructors’ competence, attractiveness, and capability. The present study extended past research investigating these expectations by (1) exposing participants to actual Ratemyprofessors.com (RMP) evaluations, (2) presenting the evaluations on a computer screen to simulate real-world exposure, and (3) assessing the effects of the evaluations on standard outcomes from the pedagogy literature. Results of Study 1 revealed that participants exposed to a positive evaluation rated an instructor as more pedagogically skilled, personally favorable, and a better lecturer than participants who read a negative evaluation. Study 2 replicated these findings and found that participants who read a positive evaluation reported being more engaged in the lecture and scored higher on an unexpected quiz taken one week later than those who read a negative evaluation. Engagement, however, did not mediate the relationship between evaluations and performance. Given these results, instructors might consider reviewing their RMP ratings to anticipate the likely expectations of incoming students and to prepare accordingly. However, research that further enhances the realism of this study is needed before specific recommendations for corrective action can be suggested.
Public Interest Statement
Ratemyprofessors.com has emerged as an indispensable source of information for students trying to decide whether to take a class from a professor. Favorable and unfavorable evaluations provided by students from multiple countries populate the website. How do these evaluations affect prospective students? In two studies, students received either a favorable or unfavorable evaluation said to have been retrieved from Ratemyprofessors.com. They then watched a recorded lecture from the ostensible professor and evaluated her skill, personality, and course difficulty (Study 1) and their engagement in the lecture and a measure of performance one week later (i.e. a pop quiz; Study 2). Students’ impressions after the lecture mirrored the favorability of the evaluation and affected their performance. Specifically, students with poorer impressions judged the professor more negatively, reported being less involved in the lecture, and scored worse on the pop quiz. Long-term effects of this technology on educational outcomes merit further study.
1. Introduction
Traditionally, only instructors and the institutions that employ them have had access to the results of students’ teaching evaluations. Consequently, course and instructor reputations among students were largely a function of the “grapevine”, with future students asking former students what they thought of a particular class and professor. This meant that student expectations, if they were formed at all, were shaped by small convenience samples providing verbal evaluations based on idiosyncratic criteria.
With the advent of the Internet, however, websites have emerged that permit students to share evaluations of teachers and courses with millions of other students online. Ratemyprofessors.com (RMP), for example, was created in 1999 and has become a widely used source of course and instructor evaluations. According to their website, Ratemyprofessors.com is “the largest online destination for professor ratings” with “more than 17 million ratings, 1.6 million professors and over 7,000 schools”. They also claim that “RateMyProfessors.com [is] the highest trafficked site for quickly researching and rating professors, colleges and universities across the United States, Canada and the United Kingdom. More than 4 million college students each month are using Rate my Professors” (Ratemyprofessors.com, Citation2017). Given the broad popularity of RMP and its potentially significant impact on student expectations, course enrollment, and learning outcomes, social science and education researchers have begun to investigate the effects of this largest of online teaching evaluation resources on student expectations (Boswell, Citation2016; Klein, Citation2017; Murray & Zdravkovic, Citation2016).
Three general findings have emerged from this research. First, scholars have discovered that participants reviewing RMP ratings pay particular attention to evaluators’ comments about professors’ personal characteristics, such as physical attractiveness and competence (Mahmoud, Mihalcea, & Abernathy, Citation2016; Mendez & Mendez, Citation2016; Rosen, Citation2017). Second, they have found that participants who read glowing ratings of a professor expect the teacher to be more competent and effective, while participants who read negative evaluations expect poor performance (Kowai-Bell, Guadagno, Little, & Preiss, Citation2011; Sohr-Preston, Boswell, McCaleb, & Robertson, Citation2016). Finally, researchers have discovered that these expectations impact not only student perceptions of the professor’s teaching, but may affect student learning as well (Edwards, Edwards, Shaver, & Oaks, Citation2009; Lewandowski, Higgins, & Nardone, Citation2012; Westfall, Millar, & Walsh, Citation2016).
The present study drew upon these findings to investigate potential perceptual and behavioral effects of student expectations that might be formed by their review of glowing or poor ratings of a professor on RMP. Study 1 investigated the perceptual confirmation of student expectations of a strong or poor teacher based on their review of either positive or negative RMP ratings. In Study 2, we examined the effects of students’ expectations of a strong or poor teacher developed from RMP ratings on two student behaviors: (1) engagement and (2) performance on an examination of the material covered in a lecture.
2. Study 1
2.1. Introduction
Psychologists have long known that teachers and students form expectations of each other and that these expectations can influence beliefs, attitudes, and perceptions (e.g. Jussim & Harber, Citation2005). A preponderance of these studies examines the formation of teacher expectations of students and the effects of these expectations on teachers’ perceptions of students’ motivation, effort, and ability (e.g. Reynolds, Citation2007). A smaller, but growing program of research considers the ways in which students form impressions of their instructors and investigates the effects of these impressions on student perceptions of teachers and the effectiveness and quality of their teaching. One source of student expectations that has been frequently studied by researchers is word-of-mouth evaluations that students are exposed to prior to any contact with the teacher. Specifically, researchers have investigated the extent to which exposure to either positive or negative word-of-mouth evaluations lead students to expect and perceive (i.e. perceptually confirm) effective or poor teaching (Kowai-Bell et al., Citation2011; see also Li & Wang, Citation2013).
Three recent studies have examined the extent to which positive or negative word-of-mouth evaluations affect student perceptions of a professor and his or her teaching. Edwards, Edwards, Qing, and Wahl (Citation2007) found that student participants who reviewed positive RMP-type ratings and then watched a video recording of the instructor teaching a class perceived the instructor to be significantly more physically attractive and credible than participants who reviewed either negative ratings or received no information about the instructor. Edwards and Edwards (Citation2013) replicated these results and added the finding that participants who watched the teaching video after reviewing mixed-ratings of the professor (both positive and negative evaluations) rated the professor’s credibility and attractiveness significantly lower than participants in a positive rating condition. Participants in the mixed-ratings condition did not differ significantly from a control condition in which no ratings were viewed. Lewandowski and his colleagues (Citation2012) had participants rate a professor they observed teaching in either a video-recorded or live teaching session using the same criteria as the RMP ratings: easiness, helpfulness, clarity, and interest. The researchers added three other items because they saw comments reflecting these attributes so regularly on the RMP website, which were: humorous, entertaining, and willingness to take a course taught by this professor. In both the recorded and live teaching conditions, Lewandowski et al. (Citation2012) found that teachers in the positive condition were judged to be easier, clearer, and more helpful, interesting, humorous, and entertaining than those in the negative condition. Moreover, participants were more willing to take a course taught by a teacher in the positive than in the negative condition.
The purpose of Study 1was to conceptually replicate and extend these findings. Like the previous studies, we exposed participants to either positive or negative RMP ratings of a professor prior to having them watch a video recording of the professor teaching a class, whose teaching they evaluated immediately afterward. What differentiates Study 1 from the previous research is: (1) we used actual RMP comments that focused on the personal characteristics of the professor, which previous research shows attract the most attention (Mahmoud et al., Citation2016; Rosen, Citation2017; Sohr-Preston et al., Citation2016), to capture the voice, content, and candor of real student comments and to shape participants’ expectations; (2) we had participants review the ratings on a computer screen exactly as they would appear on the RMP website, rather than on a paper handout (e.g. Edwards et al., Citation2009), to capture the typical exposure of prospective students; and (3) we extended the evaluations provided by participants to include important dimensions investigated by other researchers to supplement previous research findings. Consistent with previous research, we expected participants who received positive RMP ratings to evaluate the teacher’s performance more favorably along all assessed dimensions than participants who received negative RMP ratings.
2.2. Method
Studies 1 and 2 were reviewed and approved by the university Institutional Review Board prior to data collection and all participants were treated in accordance with the American Psychological Association’s ethical guidelines.
2.2.1. Participants
Sixty-four participants were recruited from introductory psychology courses to take part in Study 1. Fifty-one percent of the participants were female. Eighty-eight percent of the participants self-identified as white/Caucasian, 8% as Latino/Hispanic, 2% as Asian, and 3% as Pacific Islander. The ethnic homogeneity of the sample may restrict the generalizability of the findings. Moreover, given that the ethnicity and gender of the majority of the participants matched the gender and ethnicity of the instructor, there may have been a potential for unconscious bias in the sample. The average age of participants was 20.4 (SD = 2.25) with 38% identifying themselves as freshmen, 24% as sophomores, 25% as juniors, and 13% as seniors. Forty-six percent of respondents indicated that they used the RMP website every semester to evaluate all of their potential instructors, and another 22% reported that they used the website every semester to evaluate a few of their instructors. Only 6% of participants indicated that they never used RMP and another 6% reported using it only a few times. Seventy-nine percent of the participants indicated that they found the RMP ratings they reviewed to be “helpful” or “very helpful”. A check comparing gender, ethnicity, age, year in school, and RMP use and helpfulness between participants in the positive and negative conditions revealed no differences between the two groups on these dimensions.
2.2.2. Procedure
Participants reported to a computer lab on campus at an appointed time. Upon arrival, each participant was seated at a semi-private computer station and was given a consent form to read and sign. A researcher then read a script to the participants, which informed them that the university Faculty Development Office (a fictional entity) was interested in studying the impressions students form about teachers. Participants were asked to adopt the role of a student who was required to complete a diversity course as part of the university’s general education requirements. Specifically, they were asked to imagine that there was only one section of one course available that would fulfill that requirement, Psychology of Gender. The course was taught by a professor they did not know and had not heard anything about, so to make an informed decision about enrolling in the course, the students looked up the professor’s ratings on RMP and decided to sit in on one period of her current semester class.
After hearing this overview, participants opened a web page that brought up a screen shot of the RMP ratings for the professor. The page was randomly assigned to be either a positive or negative evaluation (each two pages in length). A timer revealed that participants looked at the positive (n = 34) and negative (n = 30) evaluations for 57 and 58 s, respectively. Following their review of the RMP ratings, participants opened a video window and, wearing headphones for privacy, watched a 12-min teaching session on sex stereotypes that was supposedly taught by the professor whose ratings they just reviewed. The video page was timed so that participants could not advance to the next page until after the playback ended, which ensured that all participants watched the full clip.
After the clip ended, participants completed a 16-item questionnaire that assessed their perceptions of the professor and the quality of her teaching. They also answered several demographic questions, items examining their personal RMP usage, and questions about whether they recognized the professor in the video clip or had taken Psychology of Gender before. Upon completion of the questions, the website displayed a written debriefing that fully explained the purposes of the study. After reading the debriefing, participants were thanked for their participation and excused.
2.2.3. Materials
2.2.3.1. RMP ratings
To make the RMP ratings as realistic as possible, we reviewed the Ratemyprofessors.com evaluations of 100 psychology faculty from 10 comparable universities. First, we checked to see whether both very low and very high ratings of psychology faculty were common. We found a wide range of comments and average rating scores, including many very high (e.g. 4.8 on a five-point scale) and many very low (e.g. 1.5). Believing that extreme averages might raise participants’ suspicion about the authenticity of the ratings used in the study, we selected an average overall rating for the instructor that would be a reasonably positive score in the positive condition (4.2) and likewise for the negative condition (1.8).
Each of the comments were taken verbatim from actual comments about psychology professors found on RMP. In previous research, the comments used were fabricated by the researchers to emphasize features of the teacher and course that were the focus of the study (e.g. Edwards et al., Citation2007; Edwards & Edwards, Citation2013; Lewandowski et al., Citation2012). Although fabricating the content of comments may be useful for producing a clean experimental design, it removes a layer of authenticity that is characteristic of computer-mediated word-of-mouth communication. Therefore, we opted for a more ecologically valid approach and selected comments from actual students that were consistent with the numerical rating for the professor in each condition. A key criterion for the selection of comments was that they included information about the personal characteristics of the professor. Consequently, selected comments emphasized the professor’s personal qualities, such as her being enthusiastic vs. boring, open vs. aloof, or kind-hearted vs. mean-spirited.
Comments in the positive condition consisted of seven positive comments, two neutral comments, and one rating with no comment, whereas comments in the negative condition included seven negative comments, two neutral comments, and one rating with no comment. When the comment taken from RMP was positive in tone, a negative version of the same comment was created for the negative condition by changing the positive words to negative words. So, the statement, “This is the BEST professor I have ever had!” was changed to “This is the WORST professor I have ever had!” Positive comments were created from negative comments using the same method. The same neutral comments, which simply described features of the class like the kinds of tests or assignments that were required, were used in both conditions.
We then created two fictional professor profiles on RMP, one for Dr Kathy Baker that was positive and one that was negative. We uploaded the individual ratings and comments to each profile, and the RMP website automatically calculated the three category rating averages and the overall rating average that were predetermined. Screen shots from the RMP website were then uploaded to Qualtrics survey webpages.
It is important to note that previous studies on the expectancy effects of word-of-mouth teaching evaluations, like those found on RMP, have employed printed stimulus materials to create the expectation of a strong or poor instructor (Edwards et al., Citation2007, Citation2009; Edwards & Edwards, Citation2013; Feldman & Prohaska, Citation1979; Lewandowski et al., Citation2012). This method provides a clean presentation of the independent variable that is high in internal validity. In the present study, participants viewed the stimulus materials on a computer in the precise format used by RMP. This difference is important because researchers have found that reading online content, with its advertisements, pop-up windows, and sidebar information, is more distracting than reading print content and thus may be less likely to be comprehended and remembered (Carr, Citation2010). Using stimulus materials that were identical to the format and content structure of RMP profiles allowed us to test the reliability of previous findings by conducting a stronger test of the hypotheses. To the extent that participants were affected by the profiles in predictable ways, despite the presence of distracting content, the reliability of previous research findings would be strengthened.
2.2.3.2. Manipulation check
To test the extent to which the positive and negative profiles of Dr Baker successfully conveyed a favorable or unfavorable impression of her teaching, 38 students read and evaluated the online profiles. Three five-point Likert-type items were embedded in the evaluation that assessed the favorability/unfavorability of Dr. Baker as a teacher: (1) The ratings were favorable toward Dr. Baker, (2) I felt the ratings gave a poor impression of the professor (reverse scored), and (3) The ratings were generally positive. Participants were randomly assigned to view either the positive or negative profile. The data were analyzed by first calculating a mean score for the three favorability items and then entering this as the dependent variable in an independent samples t-test. A statistically significant difference between the means was found, t(36) = 10.42, p < 0.001, d = 3.38, confirming that the positive profile (M = 4.2, SD = 0.47) was more positive than the negative profile (M = 2.37, SD = 0.61). The means for all other items were not significantly different. This indicates that our manipulation of the profiles was successful in conveying a favorable or unfavorable impression of Dr Baker.
2.2.3.3. Video clip
The video recording used in this study was developed in previous research (Reber, Downs, & Peterson-Nelson, Citationin press). We chose a teaching video in which the teacher used an expository pedagogy because it is the method of teaching that is most familiar to students and best fits their preferred learning style (Wittwer & Renkl, Citation2008). In the video, the instructor taught sex stereotypes primarily by defining key terms and reviewing the results of research in a typical lecture format. The camera focused on the teacher during the entire lecture, although the backs of some of the students’ heads were visible and their voices could be heard when questions were asked or answered. The teacher’s real name was never used.
2.2.3.4. Teaching assessment
The 16 items used to assess participants’ perceptions of Dr Baker and the quality of her lesson were taken from previous research. Six items were taken from Feldman and Prohaska’s (Citation1979) teaching assessment, three of which assessed the quality of the lesson taught (lesson difficulty, interest, and effectiveness) and three of which examined personal qualities of the teacher (competence, intelligence, and likability). Five items were taken from Silva et al. (Citation2008; see also Davison and Price (Citation2009) and assessed participants’ perceptions of the teacher’s ability to stimulate student interest, her organization, enthusiasm and knowledge of the material, and the likelihood that the participant would recommend the teacher to other students.
We also included the four ratings used by RMP: perceived overall quality and clarity of the instruction, and helpfulness and easiness of the teacher and class. Finally, for the purposes of this study, an item was included that examined how likely participants would be to sign up for the Psychology of Gender course this instructor taught. All assessments were made using five-point Likert-type scales that were anchored by the criterion of interest (e.g. “not at all helpful” vs. “completely helpful”).
2.3. Results
2.3.1. Data screening
An initial screening of the data found that one participant in the positive condition recognized the instructor. The data for that participant were removed. There were no missing data for any of the 16 items that assessed teaching or for the demographic and RMP usage items for the remaining 63 participants.
2.3.2. Data reduction
To assess the extent to which the data might be reducible to meaningful scales, the 16 items of the teaching assessment were entered into a principle components analysis (PCA) with varimax rotation. The analysis produced a rotated three-component solution that accounted for 71% of the variance in the pattern matrix. Component 1, on which six items loaded (all loadings > 0.30), accounted for 29.3% of the variance. Component 2, on which five items loaded, accounted for 24.5% of the variance, and Component 3, on which three items loaded, accounted for 17% of the variance.
As Table indicates, the six items that loaded on Component 1 all clearly relate to the teacher’s pedagogical skill and do so with high reliability. The five items that loaded on Component 2 all touch upon the teacher’s personal attributes, and also do so with good reliability. Finally, the three items that loaded on the third component all relate to the quality of the lecture participants observed and do so with good reliability as well. Two items concerning the effectiveness of the lecture and the teacher’s helpfulness did not load onto any one component and were analyzed as individual items only.
Table 1. Means and standard deviations for teaching assessment items organized by RMP evaluation content
2.3.3. Significance tests
Scale scores for the pedagogical skill, personal attributes, and lecture quality scales were computed by summing their respective items and dividing by the number of items in each scale. These scores, as well as the individual effectiveness and helpfulness items, were then submitted as the dependent variables to a one-way multiple analysis of variance (MANOVA, conducted to protect against Type I error in the individual analysis of variance (ANOVA) tests), with RMP rating condition (positive or negative) as a between-subjects factor. Prior to conducting the MANOVA, Pearson correlation coefficients were calculated for all the DVs and were found to be in the acceptable “moderate” range (Meyers, Gampst, & Guarino, Citation2006). Additionally, the Box’s M statistic of 13.86 was not significant (p = 0.63), which indicated that the covariance matrices of the DVs were assumed to be equal across conditions. Still, given the slightly different sample sizes, the more conservative Pillai’s Trace statistic was selected for the MANOVA test.
The MANOVA revealed a significant effect for RMP rating condition on student perceptions across all five DVs, Pillai’s Trace = 0.24, multivariate F(5, 58) = 3.67, p = 0.006, η2 = 0.24. Before conducting follow-up ANOVAs, each DV was tested for homogeneity of variance, which confirmed that all five of the DVs satisfied Levene’s test (p > 0.05). A series of follow-up analyses of variance were then conducted on the five DVs. Results showed that subjects who received a positive evaluation of Kathy Baker judged her pedagogical skill to be significantly better (M = 3.70, SD = 0.89) than those who received a negative evaluation (M = 2.93, SD = 0.78), F(1, 62) = 13.57, p < 0.001, d = 0.92. Similarly, the former group judged her personal attributes to be significantly more favorable (M = 4.15, SD = 0.73) than the latter group (M = 2.93, SD = 0.78), F(1, 62) = 4.56, p = 0.04, d = 0.54, and perceived the quality of her lecture to be significantly better in the former (M = 4.25, SD = 0.68) than in the latter condition (M = 3.83, SD = 0.72), F(1, 62) = 5.58, p = 0.02, d = 0.59. Though the means for the individual items of lesson effectiveness and teacher helpfulness both differed in the predicted direction, the follow-up ANOVAs were not significant, F’s(1, 62) < 1.98, p’s > 0.17.
2.4. Discussion
The results of Study 1 provide convincing evidence that subjects’ judgments of a teacher and her lecture were strongly affected by the RMP ratings they read. Importantly, the communication of this information was conveyed in the words of actual RMP users, complete with misspellings and poor grammar, rather than in simulated comments created by the researchers. Moreover, the information was delivered in the same online format that is typically accessed by RMP consumers, which included all the color and potentially irrelevant information (e.g. instructor “hotness”) found on the website, rather than on sheets of paper containing the comments in isolation. Despite devoting less than a minute to reading the information in a busier, potentially more distracting format, subjects honed in on the relevant information and were led to view the instructor and the lecture in a favorable or unfavorable way. Students who observed an identical teacher and lecture after reading a favorable, as opposed to an unfavorable, evaluation perceived the instruction to be more interesting and stimulating. In addition, they judged her to be more competent, intelligent, enthusiastic, knowledgeable, and organized, and they perceived the lecture to be clearer and easier to understand. Effect size estimates confirm that these effects were moderate to large. Thus, this research confirms and extends the findings of others (Edwards et al., Citation2007, Citation2009; Edwards & Edwards, Citation2013; Lewandowski et al., Citation2012), providing further evidence of beliefs being perceptually confirmed in the minds of perceivers as a result of mere exposure to pre-interaction information.
Given the clear evidence of students’ perceptual confirmation of expectations that were formed by their review of RMP ratings, there is good reason to suspect that they may also behave in a manner that is consistent with their expectations. Study 2 examined this hypothesis by investigating two relevant student behaviors: (1) engagement in the lecture and (2) performance on an examination. We outline the study and our hypotheses next.
3. Study 2
3.1. Introduction
Years of social psychological research show that perceptual confirmation of expectations goes hand in hand with behavioral confirmation (Snyder & Stukas, Citation1999). For example, teacher expectations of students’ personal attributes and capacity for learning can affect the teacher’s behaviors toward students, just as it does the teacher’s perceptions of the students (Jussim & Harber, Citation2005; Reynolds, Citation2007). Similarly, student expectations of a poor or good teacher can affect the behaviors of students in a manner that is consistent with their perceptions (Feldman & Prohaska, Citation1979). Three recent studies have examined the extent to which students’ review of RMP-type ratings create favorable or unfavorable expectations of a professor that influence students’ behaviors during and after their observation of the professor teaching a class.
The first study (Edwards, Bresnahan, & Edwards, Citation2008) assessed the extent to which humorous, positive RMP-type ratings would affect participants’ affective learning and motivation to learn. Though not behavioral measures per se, these behavioral precursors were higher when compared to a non-humorous, positive condition and a control condition. A second study (Edwards et al., Citation2009) compared participants reviewing non-humorous, positive RMP-type ratings to participants reviewing negative or no ratings on measures of cognitive and behavioral learning. Results indicated that participants in the positive rating condition scored significantly higher than other participants on an immediate recall test of cognitive learning and on behavioral learning (the latter operationalized as participants’ perceived likelihood of engaging in behaviors recommended by the instructor, such as eating healthier foods). The third study (Lewandowski et al., Citation2012) assessed only cognitive learning using an immediate recall test of the information covered in a lecture that was either live or on video. Results of both the live and video teaching conditions failed to show evidence of a difference in cognitive learning between participants reviewing positive or negative RMP-type ratings.
This emerging body of research suggests that students’ performance might be affected by how they perceive their teachers, although the results are not consistent. Study 2 built upon this literature and Study 1 by examining the behavioral consequences of expectations gained through computer-mediated word-of-mouth teaching evaluations in at least two distinct ways. First, in contrast with previous research, we implemented a delayed, unexpected recall test to examine participant retention of cognitive learning. Second, we examined the impact of expectations on participant engagement with the lecture and assessed engagement as a potential mediator between participant expectations and their perceptual and learning outcomes.
3.1.1. Unexpected delayed recall test
With regard to the implementation of a delayed, unexpected recall test, memory researchers have demonstrated that long-term retention of learning involves a different set of memory components and activities than those involved in immediate learning (Rose & Craik, Citation2012). Thus, it is a common practice to implement delayed assessments to gain more comprehensive evidence of cognitive learning. As discussed above, previous research has thus far assessed only immediate learning and done so with mixed results (Edwards et al., Citation2009; Lewandowski et al., Citation2012). Lewandowski et al., who failed to find an effect, suggest that “for some students, it would have been better to measure retention after they had time to study and reflect on the material. Thus, results in this study may have been different if students’ knowledge of the lecture was assessed at a later date” (p. 120). Edwards et al., who found an effect, also acknowledge the limitations of an immediate recall examination and state that “future research could remedy these shortcomings by employing a delayed posttest of cognitive learning” (p. 384). Therefore, we employed a delayed examination to see if differences in cognitive learning would emerge over time.
Importantly, we did not forewarn participants that they would be quizzed over the lecture material. It is possible that such a forewarning would have affected participants in positive and negative expectation conditions differently, such that those in the positive condition may have reflected on the material more and tried to do better on a subsequent test (which is an interesting empirical question in and of itself; see Lewandowski et al., Citation2012; Oeberst & Lindner, Citation2015). Our intent, however, was to test participants with a “pop quiz” to see if the expectation of the teacher, independent of an expected assessment, would produce a behavioral difference between conditions. It stands to reason, given the lack of study and reflection, that cognitive learning might be weakest when an unanticipated assessment is employed. We felt that obtaining differences between the conditions under these circumstances would be a particularly strong test of our hypotheses. Thus, we hypothesized that participants in the positive expectation condition would score higher on the unexpected delayed recall test than participants in the negative expectation condition.
3.1.2. Participant engagement
Study 2 also builds upon and enhances previous research by examining participant engagement during the lecture as a potential behavioral mediating variable of learning outcomes. Engagement is defined as “active involvement, commitment, and concentrated attention, in contrast to superficial participation, apathy, or lack of interest” (Newmann, Wehlage, & Lamborn, Citation1992, p. 11). Based on their review of research, Fredricks, Blumenfeld, and Paris (Citation2004) conclude that engagement is a multidimensional psychological construct consisting of affective (e.g. interest), behavioral (e.g. attention), and cognitive (e.g. understanding) components. Researchers also note that student engagement is influenced by a number of contextual factors, including a sense of a supportive and invested instructor (Klem & Connell, Citation2004). Finally, research shows that student engagement has a significant impact on student learning as measured by performance on examinations (Carini, Kuh, & Klein, Citation2006), as well as on student perceptions of the instructor and the course (Skinner & Belmont, Citation1993). This body of research suggests that student expectations of a poor or strong teacher might influence their engagement in a lecture that is taught by that teacher, which may in turn have an effect on their perceptions of the teacher, the lecture itself, and on their learning of the material.
There is precedent for using a mediation model to shed light on the process by which RMP-induced expectations might influence learning. Edwards et al. (Citation2009) examined the extent to which affective learning might act as a potential mediator between expectations and cognitive learning, and their results indicated a partial mediation between participants’ attitude toward the class content and their cognitive learning, suggesting that the relationship between positive RMP ratings (but not negative ratings) and cognitive learning was mediated by the positive affect participants developed as a result of their expectations of a good teacher. Noting the limitations of this partial finding, including the lack of evidence of a behavioral change, Edwards et al. called for future research on mediation, suggesting that “there may also be a direct causal relationship between expectations and learning outcomes, and/or additional mediating variables that were not accounted for in this experiment” (p. 383).
We designed Study 2 to investigate whether there may be a direct causal relationship between expectations and learning and to examine potential mediators of the relationship, namely: engagement in the lecture and perceptions of teacher and lecture quality. We theorized that expectations of a teacher may affect a student’s engagement in the classroom (e.g. higher expectations lead to more engagement). This engagement might then affect a student’s perceptions of the teacher’s pedagogical skill, personal attributes, and lecture quality (e.g. more engagement produces more favorable perceptions), which would affect student performance (e.g. more favorable perceptions lead to better performance), possibly as a result of affective factors (Edwards et al., Citation2009). Therefore, we predicted that participants in the positive expectation condition would report more engagement in the lecture than participants in the negative expectation condition. Further, we predicted that more engagement in the lecture would produce more favorable perceptions of the teacher and the lecture, which would then produce a higher score on the pop quiz.
3.2. Method
3.2.1. Participants
One hundred and three students were recruited from undergraduate psychology courses (positive expectation n = 49, negative expectation n = 54). Fifty-seven percent of the participants were female. Ninety-four percent identified their race as white/Caucasian, 4% as Latino/Hispanic, and 2% as Asian. All but 7% were single. The racial/ethnic homogeneity of the sample may limit the generalizability of the findings of this study. The average age of participants was 19.79 years (SD = 2.82), with 45% of the sample identifying as freshmen, 27% as sophomores, 20% as juniors, and 8% as seniors. When asked about their use of the RMP website, 42% indicated that they used RMP ratings to evaluate all their professors every semester, whereas 27% used the ratings to evaluate a few of their professors every semester. Seventy-nine percent indicated that they found RMP ratings to be “helpful” or “very helpful”. A check on any potential differences between participants in the positive and negative conditions on demographic and RMP usage and helpfulness dimensions showed no significant variation between groups.
3.2.2. Procedure
The procedure for Study 2 was identical to the procedure used in Study 1 with two exceptions. First, we added two questions that assessed the extent to which participants felt that they paid attention to the lecture and showed interest in the instruction to the 16-item teaching assessment instrument. These items allowed us to examine the participants’ self-perceived engagement. Second, we administered a 10-item examination of the material covered in the lecture exactly one week after the study. Given the short duration of the lecture and how little information could be covered in 12 min, the examination was limited to seven multiple-choice questions and three true/false items that were selected from a test bank that accompanied the textbook from which the lecture material was taken. The format of these questions is consistent with that used in previous research (Edwards et al., Citation2009; Lewandowski et al., Citation2012).
We told participants that there would be a second part to the study one week after the initial session, but we did not tell them that they would be tested on the material or give them any other clues about the purpose of the second session. One week later, we sent participants a link to a Qualtrics survey URL and instructed them to answer questions about the lecture. This delayed and unexpected testing method allowed us to capitalize on the finding that differences in student retention, which are often not detectable immediately following a lecture, tend to emerge following a one- or two-week period when the effects of retention decay have set in, and students who have not learned the material as deeply demonstrate reduced retention, whereas those who have learned the material more deeply demonstrate better retention (e.g. Fazio, Agarwal, Marsh, & Roediger, Citation2010).
3.2.3. Materials
We used the same script, RMP ratings, video recording, and teaching assessment from Study 1. The two items that were added to the assessment that evaluated participants’ engagement in the lecture followed the same five-point Likert-type response format as the 16 items that made up the teaching assessment. As with those items, the left side of each scale was anchored by “not at all ________” and the right side by “completely __________”. The retention test, the items examining the participants’ engagement, and the three teaching assessment scales used in Study 1 constituted the dependent variables in this study.
3.2.4. Video length
Previous research using a video recorded lecture suggests that the length of the video may impact participant learning. Edwards et al. (Citation2009) used a 10-min video in their study, whereas Lewandowski et al. (Citation2012) used a 5-min video. The results from these two studies do not match, specifically in regard to learning outcomes. Lewandowski et al. question whether the short length of their video impacted their results, noting that “it is possible that the five-minute video lecture did not present enough material to effectively measure learning” (p. 12). Given this possibility, we looked at the attention span literature for guidance about the ideal video length. Research in this field indicates that college student attention spans in a class lecture follow a predictable pattern in which the first 3–5 min are needed for students to settle down and begin paying attention. Then, student attention will ebb and flow for the remainder of the lecture, with a general decline beginning around 10 min into the lecture (Middendorf & Kalish, Citation1996). Given this pattern, we concluded that the 12-min lecture video from Study 1 was appropriate for this study because it allowed for a couple minutes of settling in and then 10 min of observation that would take place when participants are most likely paying the closest attention.
3.3. Results
We calculated bivariate correlations between participants’ teaching assessments (teacher pedagogical skill, teacher personal attributes, lecture quality), their engagement, and their retention test performance to assess the degree of multicollinearity among the DVs. All but one of the variables had moderate correlations with all the other variables (r’s > 0.54), dictating the use of MANOVA to initiate our analysis. The Box’s M-value of 20.67 was not statistically significant (p = 0.57) suggesting that the covariance matrices across all groups were equal, and because the group sizes were not equivalent, we used the more conservative Pillai’s Trace as the MANOVA test statistic. The one-way MANOVA tested the hypothesis that a significant difference between the positive and negative RMP conditions would emerge in an omnibus test of all measures in the predicted direction. Results confirmed the hypothesis, Pillai’s Trace = 0.84, multivariate F(6, 85) = 2.62, p = 0.02, η2 = 0.16.
3.3.1. Teaching assessment
Having obtained a significant omnibus effect, we conducted three follow-up ANOVAs with the teaching assessment scales as the dependent variables. All three scales satisfied the conditions of Levene’s test of the equality of the variances. The means and standard deviations for the three scales are listed in Table . As in Study 1, results showed that subjects who received a positive evaluation of Kathy Baker judged her pedagogical skill to be significantly better (M = 3.56, SD = 0.87) than those who received a negative evaluation (M = 3.10, SD = 0.91), F(1, 101) = 8.49, p = 0.005, d = 0.52. Moreover, the former group judged her personal attributes to be significantly more favorable (M = 3.96, SD = 0.67) than the latter group (M = 3.65, SD = 0.75), F(1, 101) = 4.56, p = 0.047, d = 0.44, and perceived the quality of her lecture to be significantly better in the former (M = 4.21, SD = 0.64) than in the latter condition (M = 3.90, SD = 0.78), F(1, 101) = 4.95, p = 0.03, d = 0.44.
Table 2. Means and standard deviations for study 2 dependent variables organized by RMP evaluation content
3.3.2. Engagement
The two items that assessed participants’ engagement were moderately correlated (r = 0.46), so we combined them to form a single index and submitted the index as the dependent variable to a follow-up ANOVA. Consistent with our expectations, the results of the ANOVA revealed that the two groups differed significantly in the predicted direction, F(1, 101) = 6.38, p = 0.01, d = 0.44, with participants in the positive evaluation condition (M = 3.89, SD = 0.64) reporting being significantly more engaged in the lecture than those in the negative evaluation condition (M = 3.52, SD = 0.87).
3.3.3. Retention
The 10 questions that constituted cognitive learning were scored, and a total number of correct answers was tallied for each participant. Three participants from the positive rating condition and eight from the negative rating condition did not complete the examination. This attrition reduced the number of participant scores that could be evaluated from 103 to 92 (46 positive/46 negative), an acceptable attrition rate of 11% (Valentine & McHugh, Citation2007). The result of a follow-up ANOVA that compared the learning scores between participants who reviewed positive RMP ratings (M = 7.15, SD = 1.48) and those exposed to negative RMP ratings (M = 6.39, SD = 1.14) revealed a significant difference between groups, F(1, 90) = 6.41, p = 0.01, d = 0.53. Thus, participants who reviewed a positive RMP evaluation not only judged the instruction to be better and reported being more engaged in the lecture, but they also remembered more of the lecture one week later as evidenced by a nearly 10% higher score on the pop quiz.
3.3.4. Path analysis
We constructed a mediation model that examined the extent to which participants’ expectation of a poor or strong teacher led them to perceive their own behavior (i.e. engagement) differently as they observed the teaching video, which might then affect their perceptions of the teacher’s behavior and their retention of the material taught. Figure shows the results of this analysis.
Figure 1. Path analysis of the direct and indirect effects of viewing positive or negative teacher evaluations (condition) on participants’ ratings of their own engagement, the teacher’s pedagogical skills, personal attributes, and lecture quality, as well as participants’ performance on a retention test.
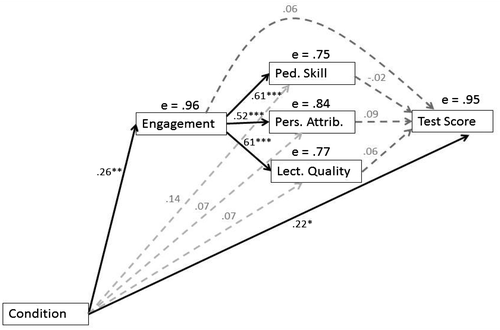
We found evidence that viewing positive or negative evaluations directly affected retention scores (β = 0.22, p < 0.05). As predicted, participants with a more favorable expectation of the teacher performed better on a pop quiz than participants with a lower expectation. Contrary to our hypothesis, however, this relationship was not mediated by engagement or perceptions. Participants’ self-perceived engagement did not significantly predict scores on the retention test, nor did participants’ perceptions of the lecture and teacher. Nevertheless, as predicted, the relationships between expectation condition and participants’ perceptions of pedagogical skill, personal attributes, and lecture quality were mediated by engagement. As Figure shows, the paths between condition and engagement (β = 0.26, p = 0.01), engagement and pedagogical skills (β = 0.61, p < 0.001), engagement and personal attributes (β = 0.52, p < 0.001), and engagement and lecture quality (β = 0.61, p < 0.001) were statistically significant. Thus, consistent with the findings of previous research, our results revealed that viewing positive or negative evaluations significantly affected participants’ self-perceived engagement in the lecture, which in turn significantly affected the participants’ ratings of pedagogical skills, teacher personal attributes, and lecture quality. Unexpectedly, these factors did not significantly affect performance. We tested the significance of indirect paths using bootstrapping procedures with 1,000 samples. None of the indirect paths were statistically significant as all bootstrapped unstandardized 95% confidence intervals for indirect paths included zero.
3.4. Discussion
As in Study 1, we found that positive and negative expectations of a teacher affected students’ perceptions of pedagogical skill, personal attributes, and lecture quality. In addition and as predicted, we found that self-perceived engagement mediated the relationship such that when participants expected a good teacher, they reported showing more interest in the lesson and paying more attention to her, which caused them to view her and her lecture more favorably. When they expected a poor teacher, they reported showing less interest and attention and, as a result, judged her and her lecture more unfavorably.
Contrary to our prediction, participants’ engagement did not mediate the relationship between expectations and performance on the examination. Rather, the link between expectations and performance was direct, a finding that Edwards et al. (Citation2009) suggested may be true in some cases. This null finding is not without precedent, as Edwards et al. found that positive affect mediated the relationship between expectations and learning only when students had a favorable impression of the teacher. Clearly, future research is needed to understand whether our failure to find mediation is a result of theoretical issues (i.e. the failure to include theoretically relevant mediating constructs) or something else. Nevertheless, the results of Study 2 show that participants’ expectations of a poor or strong teacher, which were developed from their review of positive or negative RMP ratings, had behavioral consequences for the participants. Participants were less engaged in a lecture when they expected a poor teacher and this led them to perceive fewer positive skills and attributes and rate the lecture as being of poorer quality than participants who expected an effective teacher and were more engaged in the lecture as a result. The findings also reveal that participants’ retention of the material was directly impacted by their expectation of a strong or weak teacher, regardless of differences in their engagement during the lecture.
4. General discussion
The two studies that comprised the present investigation conceptually replicated and extended the findings of previous studies that have examined the potential effects of positive and negative RMP ratings on student perceptions and behaviors.
4.1. Replication
We felt it necessary and important to test the reliability of the findings of previous studies in light of a recent emphasis on replication within the field of psychology (Fabrigar & Wegener, Citation2016). Both Studies 1 and 2 produced results that were consistent with the findings of previous research. Like Edwards et al. (Citation2007, Citation2009), Edwards and Edwards (Citation2013) and Lewandowski and colleagues (Citation2012), we found that exposing students to RMP evaluations that were positive or negative produced a significant difference in perceptions of a professor and her/his lecture. Study 2 also replicated Edwards et al.’s (Citation2009) finding of a difference in cognitive learning, with participants exposed to positive RMP ratings scoring higher on a recall test than participants exposed to negative ratings. This replication was particularly important because Lewandowski and his colleagues failed to demonstrate this same outcome in their replication of the Edwards et al. study.
4.2. Extension
Studies 1 and 2 also extended the findings of previous research to broaden and strengthen disciplinary understanding of this important phenomenon. In Study 1, we found that using positive and negative comments posted by students on RMP and focused on the personal attributes of the professor, as opposed to general comments fabricated by researchers, shaped expectations sufficiently to influence the perception of a professor and her teaching. We also showed that presenting ratings on a computer screen exactly as they would appear on the RMP website produced the positive or negative perceptions that have been found in previous studies employing paper and pencil ratings. The third extension was that the dependent variable used in Study 1 included additional dimensions that have been used in previous relevant studies, specifically pedagogical skills, teacher attributes, and lecture quality. Finding that perceptual confirmation of expectations developed by exposure to RMP ratings manifested itself on these tried and true measures enhances the reliability and validity of the dependent variables used in this program of research.
Study 2 further extended the findings of previous research by investigating two important behavioral outcomes. First, we found that differences in test performance observed in the Edwards et al. (Citation2009) study of immediate effects were also observed one week after the lecture when the test was unexpected. This broadens our understanding of the effects of RMP ratings on student learning by showing that both immediate and delayed learning differ according to the valence of the ratings the participants reviewed prior to a lecture. Given that retention of learning (i.e. delayed learning) is a chief objective of higher education, this new finding is critically important.
A second behavioral outcome that was confirmed in Study 2 and extends the findings of previous research was that the participants who reviewed glowing ratings of the professor paid more attention and were more interested in the lecture than participants who reviewed negative RMP evaluations. This increased engagement led to higher ratings of the teacher’s pedagogical skill, personal attributes, and quality of the lecture. Future research on the processes underlying this mediation is needed, but one possibility is that the increased engagement may have heightened students’ sensitivity to the professor’s competence and skill in teaching the material effectively. Students who were less engaged, on the other hand, may not have noticed evidence of effective teaching, making it easier for them to rely on the ratings they reviewed prior to the lecture rather than the actual teaching they observed.
Despite its impact on student perceptions, engagement did not mediate students’ performance on the unexpected recall test taken one week later. This may indicate that the engaged students’ attention and interest were focused primarily on those attributes of the professor and her lecturing ability that were evaluated in the RMP ratings rather than the content of the lecture. It is also possible that student engagement may not have had a mediating effect on test performance because the test was given one week after the lecture was observed after the immediate effects of the engagement may have worn off. Future research will need to examine engagement in relation to immediate learning outcomes and compare that model to the delayed retention model in order to test this possibility.
4.3. Limitations
Although this study strengthened the validity and reliability of this program of research by replicating and extending the procedure, analyses, and findings of previous studies, it did not overcome all of the limitations inherent in any investigation of this phenomenon. The participants did not naturally come across the ratings as they would if they were researching RMP ratings of professors for actual classes they might take in the future. Also, despite using real student comments and developing ratings that are realistic and similar to those found on RMP, some artificiality in the ratings was inescapable. Finally, the participants watched a short recorded lecture immediately after reviewing the RMP ratings, whereas in everyday circumstances, students often review RMP ratings at the time they sign up for classes, which is often well in advance of any observation of teaching. When they do observe, the professor teaching it is live, in the classroom, longer than 12 min, and as students who are already enrolled in the class. Though this study made some advances in the realism of the experiment, future research will need to address these remaining issues. Ultimately, the effects of RMP ratings on students actually enrolled in classes with the professors whose ratings they have reviewed, taking place over the course of a term or semester, with actual grades on the line, will need to be investigated.
Additional information
Funding
Notes on contributors
Jeffrey S. Reber
Jeffrey S. Reber is an associate professor of psychology and interim chair of Criminology at the University of West Georgia. His PhD is in general psychology with a dual emphasis in theoretical/philosophical psychology and applied social psychology. This study was designed and conducted in Reber’s relational psychology research lab which investigates the relational dynamics that form our identities and inform our thoughts, feelings, and behaviors in a variety of contexts, including the college classroom.
Robert D. Ridge
Robert D. Ridge is an associate professor of psychology and chair of the Institutional Review Board at Brigham Young University. His PhD is in psychology with an emphasis in social psychology. He studies media violence and aggression.
Samuel D. Downs
Samuel D. Downs is an assistant professor of psychology at the University of South Carolina, Salkehatchie. His PhD is in psychology with an emphasis in applied social psychology. He studies relationships using theoretical methods informed by a hermeneutic perspective.
References
- Boswell, S. S. (2016). Ratemyprofessors is hogwash (but I care): Effects of Ratemyprofessors and university-administered teaching evaluations on professors. Computers in Human Behavior, 56, 155–162.10.1016/j.chb.2015.11.045
- Carini, R. M., Kuh, G. D., & Klein, S. P. (2006). Student engagement and student learning: Testing the linkages. Research in Higher Education, 47(1), 1–32.10.1007/s11162-005-8150-9
- Carr, N. (2010). The shallows: What the internet is doing to our brains. New York, NY: W. W. Norton & Co.
- Davison, E., & Price, J. (2009). How do we rate? An evaluation of online student evaluations. Assessment & Evaluation in Higher Education, 34, 51–65.10.1080/02602930801895695
- Edwards, C., Bresnahan, K., & Edwards, A. (2008). The influence of humorous positive computer-mediated word-of-mouth communication on student motivation and affective learning. Texas Speech Communication Journal, 33, 1–8.
- Edwards, A., & Edwards, C. (2013). Computer-mediated word-of-mouth communication: The influence of mixed reviews on student perceptions of instructors and courses. Communication Education, 62, 412–424.10.1080/03634523.2013.800217
- Edwards, A., Edwards, C., Shaver, C., & Oaks, M. (2009). Computer-mediated word-of-mouth communication on ratemyprofessors.com: Expectancy effects on student cognitive and behavioral learning. Journal of Computer-Mediated Communication, 14, 368–392.
- Edwards, C., Edwards, A., Qing, Q., & Wahl, S. T. (2007). The influence of computer-mediated word-of-mouth communication on student perceptions of instructors and attitudes toward learning course content. Communication Education, 56, 255–277.10.1080/03634520701236866
- Fabrigar, L. R., & Wegener, D. T. (2016). Conceptualizing and evaluating the replication of research results. Journal of Experimental Social Psychology, 66, 68–80.10.1016/j.jesp.2015.07.009
- Fazio, L. K., Agarwal, P. K., Marsh, E. J., & Roediger III, H. L. (2010). Memorial consequences of multiple-choice testing on immediate and delayed tests. Memory & Cognition, 38, 407–418.10.3758/MC.38.4.407
- Feldman, R. S., & Prohaska, T. (1979). The student as pygmalion: Effect of student expectation on the teacher. Journal of Educational Psychology, 71, 485–493.10.1037/0022-0663.71.4.485
- Fredricks, J. A., Blumenfeld, P. C., & Paris, A. (2004). School engagement: Potential of the concept, state of the evidence. Review of Educational Research, 74, 59–109.10.3102/00346543074001059
- Jussim, L., & Harber, K. D. (2005). Teacher expectations and self-fulfilling prophecies: Knowns and unknowns, resolved and unresolved controversies. Personality and Social Psychology Review, 9, 131–155.10.1207/s15327957pspr0902_3
- Klein, B. D. (2017). On the development and application of a framework for understanding the properties of information quality of online reputation systems. Journal of the Midwest Association for Information Systems, 2017, 35–51.
- Klem, A. M., & Connell, J. P. (2004). Relationships matter: Linking teacher support to student engagement and achievement. Journal of School Health, 74, 262–273.10.1111/josh.2004.74.issue-7
- Kowai-Bell, N., Guadagno, R. E., Little, T. E., & Preiss, N. (2011). Rate my expectations: How online evaluations of professors impact students’ perceived control. Computers in Human Behavior, 27, 1862–1867.10.1016/j.chb.2011.04.009
- Lewandowski, G. W., Higgins, E., & Nardone, N. N. (2012). Just a harmless website? An experimental examination of RateMyProfessors.com’s effect on student evaluations. Assessment & Evaluation in Higher Education, 37, 987–1002.10.1080/02602938.2011.594497
- Li, C., & Wang, X. (2013). The power of eWOM: A re-examination of online student evaluations of their professors. Computers in Human Behavior, 29, 1350–1357.10.1016/j.chb.2013.01.007
- Mahmoud, A., Mihalcea, R., & Abernathy, J. (2016). Analysing Ratemyprofessors evaluations across institutions, disciplines, and cultures: The tell-tale signs of a good professor. Social Informatics, 10046, 438–453.
- Mendez, J. M., & Mendez, J. P. (2016). Student inferences based on facial appearance. Higher Education, 71(1), 1–19.10.1007/s10734-015-9885-7
- Meyers, L. S., Gamst, G. C., & Guarino, A. J. (2006). Applied multivariate research: Design and interpretation. Thousand Oaks, CA: Sage.
- Middendorf, J., & Kalish, A. (1996). The “change-up” in lectures. The Teaching and Learning Forum, 5(2), 1–5.
- Murray, K. B., & Zdravkovic, S. (2016). Does MTV really do a good job of evaluating professors? An empirical test of the internet site RateMyProfessors.com. Journal of Education for Business, 91, 138–147.10.1080/08832323.2016.1140115
- Newmann, F. M., Wehlage, G. G., & Lamborn, S. D. (1992). The significance and sources of student engagement. In F. Newmann (Ed.), Student engagement and achievement in American secondary schools (pp. 11–39). New York, NY: Teachers College Press.
- Oeberst, A., & Lindner, I. (2015). Unannounced memory tests are not necessarily unexpected by participants: Test expectation and its consequences in the repeated test paradigm. Cognitive Processing, 16, 269–278.10.1007/s10339-015-0663-3
- Ratemyprofessors.com. (2017). About Ratemyprofessors.com. Retrieved from www.ratemyprofessors.com/About.jsp
- Reber, J. S., Downs, S. D., & Peterson-Nelson, J. A. (in press). Effects of three pedagogies on learning outcomes in a psychology of gender lecture: A quasi-experimental study. Teaching of Psychology, 44, 134–144.
- Reynolds, D. (2007). Restraining golem and harnessing Pygmalion in the classroom: A laboratory study of managerial expectations and task design. Academy of Management Learning & Education, 6, 475–483.10.5465/AMLE.2007.27694947
- Rose, N. S., & Craik, F. I. (2012). A processing approach to the working memory/long-term memory distinction: Evidence from the levels-of-processing span task. Journal of Experimental Psychology, 38, 1019–1029.
- Rosen, A. S. (2017). Correlations, trends and potential biases among publicly accessible web-based student evaluations of teaching: A large-scale study of RateMyProfessors.com data. Assessment & Evaluation in Higher Education, 29, 1–14.10.1080/02602938.2016.1276155
- Silva, K. M., Silva, F. J., Quinn, M. A., Draper, J. N., Cover, K. R., & Munoff, A. A. (2008). Rate my professor: Online evaluations of psychology instructors. Teaching of Psychology, 35, 71–80.10.1177/009862830803500202
- Skinner, E. A., & Belmont, M. J. (1993). Motivation in the classroom: Reciprocal effects of teacher behavior and student engagement across the school year. Journal of Educational Psychology, 85, 571–581.10.1037/0022-0663.85.4.571
- Snyder, M., & Stukas Jr., A. A. (1999). Interpersonal processes: The interplay of cognitive, motivational, and behavioral activities in social interaction. Annual Review of Psychology, 50, 273–303.10.1146/annurev.psych.50.1.273
- Sohr-Preston, S. L., Boswell, S. S., McCaleb, K., & Robertson, D. (2016). Professor gender, age, and “hotness” in influencing college students’ generation and interpretation of professor ratings. Higher Learning Research Communications, 6(3), 1–23.
- Valentine, J. C., & McHugh, C. M. (2007). The effects of attrition on baseline comparability in randomized experiments in education: A meta-analysis. Psychological Methods, 12, 268–282.10.1037/1082-989X.12.3.268
- Westfall, R., Millar, M., & Walsh, M. (2016). Effects of instructor attractiveness on learning. The Journal of General Psychology, 143, 161–171.10.1080/00221309.2016.1200529
- Wittwer, J., & Renkl, A. (2008). Why instructional explanations often do not work: A framework for understanding the effectiveness of instructional explanations. Educational Psychologist, 43, 49–64. doi:10.1080/00461520701756420