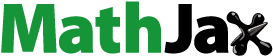
Abstract
This study which was informed by cognitive styles theories was a correlational study that examined the interactive effects of cognitive styles and their power to predict academic performance in Chemistry. Cognitive styles were measured on four dimensions: concrete–abstract, active–reflective, verbal–visual and sequential–global. The study explored how cognitive styles of students and teachers interacted to influence learner performance in Mock and in Kenya Certificate of Secondary Education (KCSE) Chemistry Examinations. The target population was all students in sixty schools elevated to National School status across Kenya’s 47 Counties between 2011 and 2012. Six schools from three counties were randomly selected; from these, one class of Form Four students was randomly selected. 293 students, 6 teachers and 6 Academic Masters were sampled. Data was collected using a Cognitive Styles Inventory, interview guide for Academic Masters and marks record forms. From correlation tests, ANOVA and regression analysis the study revealed that: (1) Three schools had very high levels of student–teacher cognitive styles congruence while the other three had low levels of congruence, (2) Out of the four dimensions of cognitive styles, only the sequential–global dimension was a significant predictor of performance in KCSE Chemistry, (3) The study constructed a regression equation: y′ = 0.904 X1 – 0.525 X2 – 0.583 X3 – 0.23 X4 + 14.794. The equation significantly predicted 62.8% of variance in performance in KCSE Chemistry (y′) based on four regressor variables: performance in Mock (X1), level of student–teacher cognitive styles match (X2), level of learner on the sequential–global scale (X3) and age of respondent (X4).
Public Interest Statement
Differences in the way people think and process information has been a point of discussion for decades. Typically, the manner in which one processes information is manifested outwardly by how one acts and reacts in given situations. This article describes the differences in styles of processing information among secondary school students. Moreover, the study determined the level to which students and their teachers differed in the manner in which they process information. The study went further to use these differences to explain learners’ academic performance. It was found that the differences in information processing can predict 62.8% of performance of a learner. Clearly, information processing (referred to as cognitive styles in this study) impacts on performance. Through proper profiling, students and teachers can be helped to adjust their learning environment and learning activities so that students are not disadvantaged by their natural way of processing information.
Competing interests
The author declares no competing interest.
1. Introduction
Cognitive styles is a term used to describe the way individuals perceive, think and remember information. Cognitive styles as a concept is a component of a larger concept termed as learning styles. Learning styles highlight characteristic cognitive, affective and psychomotor behaviours displayed by an individual as they learn. Learning styles provide an explanation for how any two learners who are taught the same concepts would differ in the way they learn as a result of various stimuli, including: environmental (e.g. noise, temperature and design); emotional (e.g. motivation and persistence); sociological (e.g. pairing and grouping); physical stimuli (e.g. auditory, visual or kinaesthetic); and psychological stimuli (e.g. global or analytic in approach, impulsive or reflective).
Cognitive styles of an individual can be gauged from their response to physical and psychological stimuli, and have both internal and external features. Internally, they are structured contents of thought and experience in one’s mind. Externally, they are outward expressions of thought in terms of flow of logic, arrangement of symbols (writings, drawings etc.), use of language and relations between these facets. Consequently, cognitive styles influence decisions and choices made by both the learner and the teacher during teaching and learning.
According to Evans (Citation2010), cognitive styles of the teacher impact on the various phases of preparation for learning, even before learning begins. The aspects impacted include: organisation of content, choice of resources, choice of tasks, as well as planning for how feedback will be given. During the learning process itself, cognitive styles determine how the lesson is structured, pace of the lesson delivery, interaction, flexibility of the learner and the teacher, as well as how marking and follow-up is done. The differences in the ways the learner and the teacher perceive, think and remember information is thus, the basis for differential performance in a classroom. Some learners therefore grasp the content better as a direct result of the manner in which the teacher presents the content.
Douglas (Citation2003) found that as many as 19 different ways of describing cognitive styles have been identified over the years, all of which consist of bipolar distinctions of thinking and thought processing styles. Some of these distinctions are: The Reflective–Impulsive Style by Kagan, Rosman, Day, Albert, and Phillips (Citation1964), The Hudson (Citation1967) Conveger–Diverger Construct, The Holist–Serialist theory by Pask (Citation1976), Ornstein’s (Citation1973) Hemispherical Lateralisation concept, The Field Dependence–Field Independence model (FI-FD) by Witkin, Oltman, Raskin, and Karp (Citation1971), The Adaption–Innovation Theory by Kirton (Citation1976) and The Wholist–Analytic and Verbal–Imagery Model by Riding and Cheema (Citation1991). Riding and Cheema acknowledged the existence of a plethora of labels used to categorise cognitive styles. However, they noted that there were overlaps in some of the labels and they re-categorised them to limit repetition.
In the present study, the re-categorisation by Riding and Cheema (Citation1991) guided the researcher in selecting labels and definitions of cognitive styles that captured the following dimensions: concrete–abstract, verbal–visual, active–reflective and sequential–global, consideration for intervening variables was also made.
1.1. Statement of the problem
Learners all perceive and process information, however, they linger at different places along the way (McCarthy, Citation1990). This lingering forms the basis for differential performance among learners who have shared the same learning experiences. For this reason, an evaluation of how learners think is paramount in assessing knowledge acquisition. According to Buli-Holmberg, Schiering, and Bogner (Citation2008), many teachers simply assess “how much” rather than “how” the student has learnt. Thus, not many learners are aware of how they acquire and process new information. Furthermore, teachers are also unaware of their own thought processing and that of their students. It follows then that in an effort to get learners to understand, teachers tend to impose their own cognitive styles on learners. Such imposition results in a blockage in knowledge transfer which results in teaching of concepts repeatedly instead of moving to subsequent content. Delayed delivery of content has direct bearing on any future learning considering that when concepts in the syllabus are not adequately covered, some important building blocks are skipped. Learners then enter the next level of learning with a shortfall on requisite skills.
1.2. Purpose of the study
The purpose of this study was to determine the predictive power of cognitive styles of students and teachers on academic performance of the learner in selected National Secondary schools in Kenya.
1.3. Objectives of the study
The study was guided by the following objectives: To
(1) | Describe the cognitive styles of students and teachers. | ||||
(2) | Determine the level to which cognitive styles of students and teachers interact to influence learner performance. | ||||
(3) | Determine the predictive power of cognitive styles on academic performance of learners. |
1.4. Significance of the study
Findings of this study provide a basis for a shift in focus that can enrich the teaching and learning process to improve learner performance. The study shows that indeed there are differences in the psychological conditions of thought (i.e. cognitive styles) among learners. Awareness of these differences may assist the learner to modify their thought and behavioural strategies. As such, they are then able to apply the most effective learning strategies for the learning tasks at hand. For the teacher, the study provides justification for incorporating moderations into teaching and suggests methodologies that address every learner’s individual cognitive style. Such considerations may reduce frustration for both the learner and the teacher as content taught is mastered faster and in a more integrative manner. Teacher training institutions may also use findings of this study to justify the inclusion of cognitive styles as an important concept to be taught to teacher trainees. Finally, this study may inform policy review and changes in practice such as training, testing and supervision in the Ministry of Education, Teachers’ Service Commission (TSC), Kenya National Examination Council (KNEC), The National Commission for Science Technology and Innovation (NACOSTI), the Commission for University Education (CUE) and the Kenya Institute for Curriculum Development (KICD).
1.5. Theoretical and conceptual framework
Riding and Cheema (Citation1991) developed the Wholist–Analytic and Verbal–Imagery Model that describes two dimensions of cognitive styles: the Wholist–Analytic (W-A) dimension and the Verbal–Imagery (VI) dimension. The Wholist–Analytic dimension described how individuals tended to organise information; either in wholes or in discrete parts. The dimension acknowledged that some individuals processed and organised information into its component parts (described as analytics); while others retained a global or overall view of information (described as wholists). An individual’s position on the Wholist–Analytic dimension was determined by the relative speed of processing “matching figure” tasks and “embedded figure” tasks. In the “matching figures” test, the respondent is presented with pairs of geometrical shapes which are to be matched. A relatively fast response to this task would indicate a wholist tendency.
An individual’s style on the Wholist–Analytic (W-A) dimension was then identified by calculating a ratio between speed of processing and the matching figures parts of the test. The analytic learner was described as one who treated a situation as a collection of parts and tended to focus on one or two aspects of the situation at a time to the exclusion of the others. The wholist learner on the other hand, treated a situation as a whole and tended to have an overall perspective and to appreciate its total context. For the wholist, Riding and Cheema (Citation1991) believed there was a danger that the distinction between the parts of the topic may become blurred. For analytics, the separation of the whole into its parts may be focused on at the expense of the others hence its overall importance exaggerated.
According to Riding and Cheema (Citation1991), the Wholist–Analytic dimension interacted with the structure and organisation of contents of instruction. For example, analytics take a structured approach to learning and will prefer information that is set out in a clearly organised way. They may impose order of information, events and experiences, which are not inherently structured, or may attempt to do so in situations where structuring is inappropriate (e.g. in a brainstorming exercise). Wholists on the other hand will not take a structured approach and may therefore need help in imposing a structure on some unstructured situations or experiences. These aspects of W-A dimension are likely to affect preferences for different types of instructional methods (e.g. role play vs. distance learning) and instructional media (e.g. text vs. video).
The second dimension described by Riding and Cheema (Citation1991) was the Verbal–Imagery (V-I) dimension. The V-I dimension described an individual’s habitual mode of representation of information in memory during thinking. Verbalisers consider the information they read, see, or listen to, in words or verbal associations. Imagers, on the other hand, as they read, listen to or consider information, they experience fluent spontaneous and frequent pictorial mental pictures.
According to Riding and Cheema (Citation1991), The V-I dimension interacted with mode of presentation of information (for example textual/verbal of diagrammatic/pictorial modes). It could therefore be anticipated that verbalisers may prefer textual modes of presentation while imagers preferred pictorial and diagrammatic information. For verbalisers, focus tended to be outward towards others; they prefer stimulating environments and the social group in which they find themselves becomes an extension of themselves. We further argued that for imagers, the focus was more inward and they were more passive and contended with a static environment, they were also less socially aware. Thus, to Riding and Cheema, instructional methods and their varying degrees of social interaction accorded would be viewed favourably by different cognitive style groups.
Riding and Cheema’s (Citation1991) model further showed that the two orthogonal dimensions (i.e. the Wholist–Analytic and the Verbal–Imagery) interacted during learning, as shown in Figure . Consequently, a classroom environment would have four types of learners: analytic imagers, analytic verbalisers, wholist imagers and wholist verbalisers.
Figure 1. Wholist–analytic and verbal–imagery model (Riding & Cheema, Citation1991).
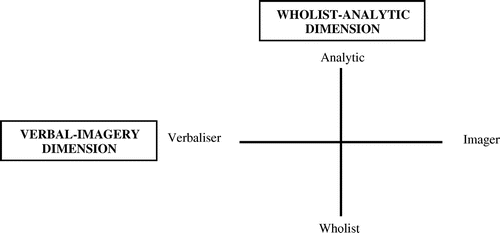
Although Riding and Cheema’s model successfully re-categorised the many cognitive styles labels used by various researchers, it was the opinion of the researcher of the present study that two dimensions proposed by Kolb (Citation1984) did not quite fit the Wholist–Analytic and Verbal–Imagery labels. The present study thus analysed cognitive styles on four dimensions; two proposed by Riding and Cheema (Citation1991) and two by Kolb (Citation1984).
Kolb’s (Citation1984) Theory of Experiential Learning explained the cyclic pattern of all learning. Kolb explored the process associated with making sense of concrete experience. According to him, a learner goes from experience, through reflection and conceptualisation, to experimentation and back to experience. Thus, experiential learning occurs as a direct result of a learner’s participation in learning events. Kolb’s model was built around an understanding of participant’s own experiences and their own reflection about that experience. From the theory of experiential learning, two dimensions of style were identified by Kolb: the concrete–abstract and the active–reflective dimensions.
The concrete–abstract dimension explained how information was taken in or perceived. According to Kolb (Citation1984), concrete learners absorbed information through direct experience, by doing, acting, sensing and feeling. In contrast, the level of abstractness was related to understanding data through reason and intuition. Abstract learners took in information through observation and thinking about what was theoretical or speculative in nature, and they understood and believed what they could not actually see.
The active–reflective dimension accounted for the amount of time and number of incorrect responses given before a learner reached the correct answer. Reflective individuals were characterised by their tendency to focus on accuracy rather than on speed of response, whereas impulsive individuals were characterised by the tendency to focus on speed rather than on careful avoidance of mistakes. In conditions of uncertainty, reflective individuals were likely to approach each new task with a degree of caution, assessing the difficulty of the task to ensure that they responded accurately.
Based on the four dimensions of style described in this sub-section, the researcher structured the measurement of the cognitive styles on the following four dimensions: verbal–visual and sequential–global (which Riding & Cheema, Citation1991 termed as Verbal–Imagery and Wholist–Analytic), concrete–abstract and active–reflective (as described by Kolb, Citation1984).
Based on the Wholist–Analytic and Verbal–Imagery Model by Riding and Cheema (Citation1991), and the Kolb’s (Citation1984) Theory of Experiential Learning, the researcher proposed a conceptual framework (see Figure ) that accounted for cognitive styles, performance and six extraneous variables.
Figure depicts that cognitive styles as a variable (operationalised as Perception and Processing by the learner) has an effect on performance (measured by considering scores obtained on Mock and KCSE Chemistry Examinations) of the learner. It was considered that gender, age, location of school, school culture and the length of contact time between the teacher and the individual learner were likely to influence the interaction between cognitive styles and performance.
2. Literature review
Although there is consensus that cognitive styles have an effect on learner performance, the nature of this effect has resulted in contradictory reports. Differences in how well a student learns and performs in different teaching environments is more marked for students identified as belonging to certain cognitive style categories (Alamolhodaei, Citation2001; Atkinson, Citation2010; Cano, Citation1999; Grimley & Banner, Citation2008; Kai-Ming, Citation1997). Kai-Ming (Citation1997) determined the role of cognitive styles as a moderator variable in students’ performance across a variety of assessment methods. He found out that Field-Independent students tended to perform better than Field-Dependent students across three assessment types. This finding was further supported by Alamolhodaei (Citation2001) whose results supported the hypothesis that students with divergent cognitive styles showed higher performance than convergent ones in pictorial problems. A similar conclusion on importance of cognitive styles was reached by Witkin (Citation1973), Riding and Grimley (Citation1999), Peklaj (Citation2003), Gellel (Citation2005), Atkinson (Citation2010) and Ibrahim and Aljughaiman (Citation2012). The researchers revealed significant differences in performance of various groupings of cognitive styles on academic tasks. Ibrahim and Aljughaiman (Citation2012) and Atkinson (Citation2010) found that there were significantly more wholists and verbalisers among the high performers, while analytics and imagers performed at lower levels. In fact, Atkinson’s findings revealed that 100% of wholists achieved a score above the mean of the sample, while only 50% of analytics managed the same. The Atkinson (Citation2010) study went further and captured the effect of interaction of cognitive styles as they related with performance. Significant interaction was found between two cognitive style categories and performance: Wholist verbalisers and analytic imagers; the mean for wholist verbalisers was almost double that of analytic imagers. Analytic imagers were found to have achieved poorest results and had benefited least from the learning experience provided.
When considering influence of cognitive styles on performance, other variables such as gender, age and culture must be considered. The gender differences model by Maccoby and Jacklin (Citation1974), stipulated vast psychological differences between males and females. This was in stark contrast to the gender similarities model supported by Hyde (Citation2005). Hyde stipulated that men and women, boys and girls were alike on most, but not all, psychological variables. Findings by Maccoby and Jacklin (Citation1974) are further sharply contrasted in subsequent studies by Brandon, Jordan, and Higa (Citation1995) and Kenney-Benson, Pomerantz, Ryan, and Patrick (Citation2006) who found that girls had higher grades than boys in math and that, girls’ grades increased over time while boys’ grades remained the same. Further a study by Zhang (Citation2007) showed that the manner in which student–teacher styles match related to student achievement varied as a function of academic discipline. In addition, Samms (Citation2010) demonstrated that where the cognitive styles gap (difference in style) was small between the teacher and the learner, the learners did not explore resources effectively and they showed less effort.
In a later study, Grimley and Banner (Citation2008) attempted to determine whether cognitive styles could predict performance. They investigated the interplay between working memory, cognitive styles and overt behaviour to ascertain predictors of General Certificate of School Education (GCSE) achievement. A computer-administered task was given where each participant was tested individually to determine amount of time spent on verbal questions as opposed to time spent on visual questions. Working memory was also assessed on a simple task that required recall of order and colour of objects presented. Using a One-Way ANOVA it was revealed that analytic verbalisers had the best GCSE performance, followed by analytic imagers, wholist imagers and finally wholist verbalisers with the least score.
Further, stepwise multiple regression was run, with score on GCSE as the dependent variable. Predictor variables included were: two dimensions of cognitive styles (wholist–analytic and verbal–imagery), working memory, behaviour scores and the interactions of each of the four variables. The regression analysis generated a model that had five significant variables: behaviour, working memory, verbal imagery by working memory interaction, verbal imagery by wholist–analytic interaction and working memory by wholist–analytic interaction. The model was a good fit, accounting for 58% of the variance in GCSE score.
Findings on the predictive power of cognitive styles are supported by the findings by Zhang (Citation2007). Zhang ran multiple regression analysis to determine the predictive power of student–teacher style match/mismatch on performance on three academic disciplines: Mathematics, Physics and Public administration. Results showed that among Mathematics students, no significant correlation was found between student–teacher style match/mismatch and performance. Among Public administration students, prediction was found to be significant on legislative style. Among Physics students it was found that a mismatch (not match) between students and teachers in judicial and legislative styles were related to student achievement.
Zhang (Citation2007) further conducted Stepwise Multiple Regression analysis to determine whether performance differences were evident among specific content areas in the three subjects. For Physics, prediction was found to be significant on statistical mechanics (R2 = 17, β weight .41) and on electrodynamics (R2 = 17, β = .41). The researcher concluded that thinking styles match between students and teacher mattered; however, it varied not only at the level of academic discipline (e.g. prediction was significant in Physics but not in Mathematics) but also at the level of specific subject matter (e.g. in statistical mechanics and electrodynamics which are both specific subject matter in Physics).
The study by Zhang (Citation2007) acknowledged that there were several variables (age, sex and personality) which could have affected the relationship between student–teacher styles match and academic performance. Zhang advised that in future studies, researchers should embed these variables to allow for more comprehensive analysis. Arguably, with the relevant predictor variables in place, cognitive styles as a variable can be a worthy predictor of the variance in performance. The insights from the foregoing studies guided the researcher in the methodology applied to select the predictor variables for academic performance and intervening that could impact on the prediction. Based on the findings in the reviewed literature that the significance of prediction was subject-specific, the researcher only focused on academic performance in Chemistry.
The reviewed studies present a good progression from identifying dimension of cognitive style, profiling cognitive styles of students and teachers, matching learner styles to teacher styles and relating cognitive styles to achievement. However, none of the researchers in these studies made consideration for the fact that both internal and external examinations are administered in schools and thus student–teacher styles match may lend more intricate angles to the analysis of achievement. This study particularly looked at the student–teacher cognitive styles match and the resultant variation in performance of students on both Mock Examinations and in the National Examination.
In the description of cognitive styles in the studies reviewed, some researchers described and measured cognitive styles on only one dimension. Other researchers such as Riding and Cheema (Citation1991), with Wholist–Analytic and Verbal–Imagery dimensions; and Gregorc (Citation1982) with the Concrete–Abstract and Sequential–Random dimensions, measured cognitive styles on two dimensions. In the present study, the researcher used four dimensions to determine cognitive styles: concrete–abstract, verbal–visual, active–reflective and sequential–global.
Further, this study considered differential achievement of students across six schools to account for differences in school culture. Additionally, the prediction model constructed in the present study was done more comprehensively than any previous models with consideration for student–teacher cognitive styles match in two separate examinations (Mock and KCSE), and a range of other extraneous variables. The fact that the reviewed studies presented contradictory findings and that a substantially larger percentage of studies on cognitive styles were carried out in countries outside Africa, and in only one institution by the various researchers, was good motivation for the researcher to join in the conversation with reference to several schools in Kenya.
3. Methodology
3.1. Research design
The study was a correlational study that aimed to profile students’ and teachers’ cognitive styles and to relate the profiles to the learners’ academic performance. The variable “cognitive styles” was operationalised as an embodiment of preferred ways of acquiring new knowledge (perception) and using it (processing).
Cognitive style profiling resulted in categorisation of the learner and the teacher on four dimensions: active or reflective, visual or verbal, abstract or concrete and sequential or global. The dependent variable was performance which was measured by scores in Mock and KCSE Chemistry Examinations. The following intervening variables were taken into account: gender, age, location of the school, school culture and length of student–teacher interaction.
3.2. Participants
The study was carried out across Kenya in secondary schools that were elevated to National School status in 2011 and 2012. The population for the study comprised all Form Four students taking Chemistry in secondary schools, all teachers of Chemistry and all Academic Masters in 60 newly elevated National Schools in Kenya. Three counties were chosen as follows: Trans-Nzoia, Bungoma and Kwale. Two schools were selected from each county to make a total of six schools. One Form Four stream was randomly sampled for participation. A total sample of 342 participants (330 students, 6 chemistry teachers and 6 academic masters) was selected.
All the six schools in the sample were single gender schools; 3 boys’ schools and 3 for girls’ schools. 52% of the student respondents were male while 48% were female. It was found that 95% of the students joined their respective schools in Form 1, only 5% joined either in Form 2, Form 3 or Form 4. The mean age of students in the six schools was 17.66 years. Mean age varied from 17.23 to 18.07 years in the various schools. A One-Way ANOVA test revealed that there was a significant mean difference in age within the schools (F = 5.66 (5,293), p < .05). An LSD Post hoc test showed that difference could mainly be attributed to students in School 4 and 5 who were older. Furthermore, it was determined that the school cultures in the sampled schools were comparable given that specific variables (admission criteria, location of school, co-curricular activities and test-taking training programme) did not differ greatly among the schools. School culture as a variable was also controlled for by selecting only National Schools which reduced the infrastructural and programmatic differences among the schools.
3.3. Measures and procedure
Three instruments were used in this study:
(1) | A Cognitive Styles Inventory that comprised of 32 objective questions that queried preferences in perception and processing. | ||||
(2) | An interview schedule for Academic Masters that explored school culture (including admission criteria, location of school, co-curricular activities and test-taking training programs). | ||||
(3) | Marks Record Form—designed to record results from Mock tests and KCSE Examinations. |
A pilot study was carried out on 36 students in one school outside of the sampled counties. The reliability indices for the four dimensions of style were all above the 0.50 threshold; all instruments were also peer reviewed and were reviewed by experts. Study data was collected between 2013 and 2014. All participant signed informed consent form after a briefing of the study. We further adhered to the principles of voluntary participation, anonymity, confidentiality and honesty. Data was analysed both qualitatively and quantitatively.
4. Findings and discussions
4.1. Performance in mock chemistry examinations
The Mock Examinations are regional examinations done in the second term of the academic year in preparation for the national examinations to be done in the third term. Typically, each County offers its own examinations to schools within it. As shown in Table , performance in Mock Chemistry Examinations in the six schools averaged at a grade point of 4.79 (Grade C Minus), out of a possible maximum score of 12 points. The highest score was from School 1 with 7.21 points (Grade C Plus) while the lowest was School 6 with 3.50 points (Grade D Plus).
Table 1. Descriptives—mean performance in mock examinations
A One-way ANOVA test was run to determine whether performance in Mock Chemistry among the six schools was significantly different, the results showed that the six schools differed significantly in performance F = 18.455 (5,269), p < .05. Post hoc analysis showed that School 1 was significantly different (p < .05) from all the sampled schools except School 2 (p > .05). Performance in School 6 differed significantly with that of all the schools except School 5.
4.2. Performance in Kenya certificate of secondary school examination (KCSE) chemistry examinations
KCSE is the national examination administered in the third term to all Form Four students across Kenya at the end of their four-year learning cycle. Performance in KCSE Chemistry Examinations among the sampled students was comparatively higher (grade C Plus of 7.41 points) than performance in Mock Chemistry (Grade C with 4.79 points). As seen in Table , the highest score was recorded from School 1 that posted an average grade of A Minus (10.34 points) and the lowest was from School 4 that with a grade D Plus (4.08 points). A One-Way ANOVA test determined that performance was significantly different among the schools (F = 35.244, n = 293, p < .05). Post hoc analysis revealed that with a mean of 10.34, School 1 was significantly different from all the other five schools. In fact, difference in performance between schools was significant for all schools except between School 2 and 3 and between School 5 and 6.
Table 2. Descriptives—mean performance in KCSE chemistry
4.3. Cognitive styles profiles of students and teachers
Cognitive styles of each student and each teacher were determined on four dimensions of cognitive styles: concrete–abstract, active–reflective, verbal–visual and sequential–global. Each participant’s preferred style was measured on a self-report scale of 0 to 8 on each of the four dimensions.
4.3.1. Cognitive styles of students
On the concrete–abstract dimension, 85% of all the learners were found to be concrete learners while 15% were abstract learners. On the active–reflective dimension, it was determined that 74% of the learners were active learners while 36% were reflective. On the verbal–visual dimension, most students (74%) were visual learners, only 26% were found to be verbal learners. The sequential–global dimension revealed that students were predominantly sequential learners (82%) and only 18% were global learners (see Table ).
Table 3. Cognitive styles of students
The finding that there were more concrete learners in a classroom supports the findings of Franzoni and Assar (Citation2009) and Santo, Soares, Silveira, and Oliveira (Citation2015) that there were more sensing learners (also categorised as concrete learners) in their samples than there were intuitive learners (also categorised as abstract learners). Further, the present study partly concurred with findings of Aljojo (Citation2012) who found that undergraduate students were concrete in approach while postgraduate students and lecturers predominantly applied the abstract style.
The high prevalence of concrete learners in the present study can be explained by the Theory of Cognitive Development advanced by Piaget in Wadsworth (Citation1971) who described how thought processes evolved from simple reflexes to sophisticated internal mental structures. According to Piaget, with advancement in age, individuals become progressively more adept at logical thinking and at employing reflective abstraction. It was not surprising then that the learners in this study were found to be predominantly concrete, as opposed to older students such as post graduate learners and lecturers whom Aljojo (Citation2012) found to be more abstract.
The finding of high prevalence of the active learners corroborates that of Franzoni and Assar (Citation2009) who demonstrated that there were more active learners than reflective ones in the classroom. This study finding also supported that of Aljojo (Citation2012) who established that among undergraduates and postgraduate students, as well as among lecturers, preference was portrayed for the active style. Santo et al. (Citation2015) also found that on the active–reflective scale 83% of students in the face to face study mode and 57% of those in the distance learning mode were active learners. Arguably, learners across various institutions mostly applied an active style when learning, as was the case with the students in the present study.
In the present study, we further found that a majority of the learners (average of 74%) in the six schools were visual. This finding was in agreement with those of Franzoni and Assar (Citation2009), Russell (Citation2010), Atkinson (Citation2010), Aljojo (Citation2012), as well as with those of Santo et al. (Citation2015) who all found that their respondents’ styles on the verbal–visual dimension, were highly skewed towards visual learning. Difference in findings was however noted with the findings of Grimley (Citation2007) and also with those of Grimley and Banner (Citation2008) who found that among their respondents, verbalisers and visualisers had about equal prevalence of 50% each. Nevertheless, no contradictory findings were reported by the various authors, thereby cementing the conclusion that learners in most classrooms are typically visual.
The finding that majority of learners in the sample were sequential supports the findings of Grimley (Citation2007), Grimley and Banner (Citation2008), Franzoni and Assar (Citation2009), Russell (Citation2010), Evans (Citation2010) and those of Santo et al. (Citation2015) who all found that the distribution of cognitive styles of students on the sequential–global dimension was skewed towards the analytic (also called sequential) category. The findings of this study, however, differed with those of Atkinson (Citation2010) who found that global learners formed 59% of the sample while sequential learners consisted 41% of the sample. Although the Aljojo (Citation2012) study revealed that among postgraduates and lecturers, preference was for global learning (78 and 76%, respectively), he also found that among undergraduates, 52% of students had preference for sequential learning. The findings of the present study therefore were in agreement with the findings of majority of researchers who reported that there were more sequential learners than there were global learners in a typical classroom.
Russell (Citation2010) cautioned that age of the learner had to be considered when analysing learner approach to wholistic–analytic content. In his study, Russel revealed that among his sample, 70% of the 52–65 age group were categorised as sequential, while only 5% were found to be wholists. This finding was reflective of the fact that age was a significant variable when considering the wholist–analytic dimension. This fact was taken into consideration in the present study, thereby justifying the inclusion of age and cognitive styles in the prediction of performance.
4.3.2. Cognitive styles of teachers
As shown in Table , it was observed that: (1) All 6 teachers were concrete, with an average score of 3 out of 8 on the concrete–abstract scale, (2) Five teachers (83.3%) were active, with an average of 3, (3) 5 teachers (83.3%) were visual; with an average of 6, (4) 5 teachers (83.3%) were sequential, with an average of 3.
Table 4. Profile of cognitive styles of chemistry teachers
Evidently, the cognitive styles of these teachers did not vary much from each other, in fact, the cognitive styles of the teachers in School 1, 4, 5 and 6 were exactly the same. This finding could be explained by the fact that the sample was limited to only Chemistry teachers. These respondents had previously been students of Chemistry for many years before becoming trainee teachers and finally becoming teachers of Chemistry. They also had many years of experience as Chemistry teachers. The length of time that the teachers had spent learning and teaching the subject was likely to predispose them to certain ways of thinking that led to the evidenced similarities.
In this study, it was assumed that the teacher teaches in the same manner in which s/he preferred to learn. For instance, a teacher who found it easy to understand a diagram would commonly use diagrams to teach his/her learners. Over a long period of time, the learner would acquire this skill and with that came the crystallisation of the learner’s cognitive style.
As can be observed from Table , the Chemistry teacher in School 6 was a highly concrete teacher. The lesson for such a teacher would often be dominated by experimentation and observation. As much as Chemistry requires concreteness, the teacher would have to often revert to abstraction to clearly explain what was being observed. The present study also found that teachers were predominantly active, visual and sequential. The Chemistry teacher needs to be able to use diagrams, models and formulae to successfully deliver content to the learner, thus a visual teacher may deliver Chemistry content more effectively. On the other hand, being an active teacher (as opposed to a reflective one) may not lend itself well to teaching of Chemistry since being active implied impulsivity and being greatly influenced by the social environment around the teacher. However, considering that 74% of students were also found to be active, the teacher may have been able to present content in a manner that appealed to majority of learners, thereby improving their performance.
On the sequential–global dimension, the finding that teachers were predominantly sequential could be advantageous to the teachers and the learners. Sequential style inclines the teacher towards delivering content in a step-by-step manner before building up to a global representation. Working sequentially also makes it easy for the teacher to engage learners in analytic tasks. Such progressive movement likely allows the student to finally come to a conclusion about the integrated whole and make personal meaning of the content without necessarily being given the connection by the teacher.
The findings on the cognitive profiles of teachers in this study differed on two scales from the findings of Santo et al. (Citation2015) who found that cognitive styles among instructors were predominantly abstract, visual, active and global. However, it must be noted that even though the Santo et al. (Citation2015) study found that the respondents were abstract and global (unlike in the present study where they were found to be concrete and sequential), the participants were lecturers and tutors from a private institution. They were also from a different cultural setting, and being lecturers may have had different teaching approaches as compared to those of secondary school teachers. Further, as pointed out by Witkin (Citation1973), it is reasonable to find a heavy concentration of more sequential teachers in the mathematics–science domain, they are also more directive in instruction than wholist (also called global) teachers who prefer interpersonal engagement and democratic approaches to learning.
4.4. Congruence in student–teacher cognitive styles
To determine impact of cognitive styles on performance it was necessary to narrow down the analysis to the teacher and the individual student. A 100% match meant that the student–teacher styles matched on all four scales (e.g. teacher is concrete, active, verbal and sequential, and the student displays the same four characteristics), 75% meant they matched on three out of the four dimensions, a 50% match meant they matched on two dimensions, 25% meant they matched on only one dimension and 0% match meant student–teacher styles did not match at all on all the four dimensions. Results summarised in Table showed that three schools (School 4, School 5 and School 6) registered very high numbers of students who had 100% match in cognitive styles with their teachers (51, 42 and 39%, respectively) in contrast to School 1, School 2 and School 3 that had 8, 12 and 2%, respectively.
Table 5. Percentage match in student–teacher cognitive styles
The sharp divide in level of student–teacher match in cognitive styles thus resulted in Schools 1, 2 and 3 standing out from Schools 4, 5 and 6. According to Samms (Citation2010), when there are small cognitive style gaps between the teacher and the learner, learners are not likely to explore their learning resources effectively and they show less effort. Although Samms (Citation2010) reported negative impact, other studies (Cafferty, Citation1980; Rochford & Mangino, Citation2006) reported positive effect of teacher–student styles match on performance, while Spoon and Schell (Citation1998) reported no impact at all. On the other hand Zhang (Citation2007) reported both positive and negative effect of styles match depending on the subject matter being tested. For this reason, we found it prudent to further explore whether the levels of congruence reported significantly impacted on learner performance.
4.5. Predictive power of cognitive styles
Having established that cognitive styles of learners and teachers interacted to influence performance, the study sought to establish the predictive power of cognitive styles on student performance in Chemistry. A prediction model with eight predictor variables was tested using Multiple Regression analysis. With performance in KCSE Chemistry Examinations as the outcome variable, the predictor variables were: performance in Mock Chemistry Examinations, level of congruence in teacher–student cognitive styles, sex and age of the learner, and the learner’s level on the four scales of cognitive styles (concrete–abstract, active–reflective, verbal–visual and sequential–global). The model was used to test the following hypothesis: Ho: Cognitive styles cannot significantly predict learner performance. Table presents descriptive statistics for the dependent variable and the eight predictor variables.
Table 6. Descriptive statistics—variables for the prediction model
The correlation matrix in Table shows the Pearson rxy between the nine variables. Congruence correlated significantly with all other variables; thereby implying that it was a highly significant variable in this model. Performance in KCSE Chemistry correlated significantly with all variables except level on verbal–visual scale, level on sequential–global scale and sex of the student. For Mock, the correlations were all significant except for the verbal–visual and the sequential–global scales. The highest correlation index was between performance in Mock and performance in KCSE (rxy = .735). Also observed were some negative correlation indices which indicated that as the measure on the given independent variable increased, the measure on the second variable decreased. For example, high student–teacher cognitive styles match resulted in low performance in Mock (rxy = −.140) showing an inverse relation.
Table 7. Correlation between predictor variables
Prediction was as shown in Figure .
Figure shows a scatterplot of the eight regressor variables in a simplified the equation:
where x stands for all the eight variables together.
The prediction was further summarised in Table ; the R value (R = .802) is the multiple correlation coefficient which showed that the prediction was high since the maximum correlation value was 1.000. The adjusted R2 = .631, this meant that together the eight independent variables accounted for 63.1% of the variability in performance in KCSE Chemistry Examinations.
Table 8. Multiple regression model summary
From Table , the F-test revealed that the model was a good fit for prediction
Table 9. Significance of regression model
There was a significant linear regression. Beta coefficients for three out of the eight predictor variables were non-significant predictors; these were level on the concrete–abstract, active–reflective and verbal–visual scales. On the other hand, the learner’s age, sex, their level on the sequential–global scale, their level of congruence with teacher’s cognitive styles and performance in Mock Chemistry Examinations significantly predicted performance in KCSE Chemistry Examinations.
Using the unstandardised coefficients on Table , the equation for the multiple regression line was constructed as:
Table 10. Significance of independent variables in prediction
where CA = Concrete–Abstract, AR = Active–Reflective, VV = Verbal–Visual, SG = Sequential–Global.
Having established the facts above, the non-significant variables were removed from the model and the regression analysis was re-run to exhibit the strongest effects. A new regression equation was structured that could predict KCSE performance of a learner (y) based on four regressor variables: performance in Mock (X1), level of student–teacher cognitive styles match (X2), level of learner on the sequential–global scale (X3) and age of respondent (X4).
The regression equation can significantly predict 62.8% of performance. Accordingly, the null hypothesis was rejected and it was concluded that cognitive styles can significantly predict learner performance. This finding of the predictive power of cognitive styles was not very different from that found by Grimley and Banner (Citation2008) who developed a model that accounted for 58% of the variance in GCSE score. The Grimley and Banner model had five predictor variables: behaviour, working memory, verbal imagery by working memory interaction, verbal imagery by wholist–analytic interaction and working memory by wholist–analytic interaction. Cognitive styles could, therefore, be used to predict academic performance but, as was cautioned by Zang (Citation2007), the prediction varied not only depending on academic discipline but also depending on the specific subject matter being tested.
4.6. Conclusions and implications
The model developed in this study can be used to objectively diagnose learner weakness by applying techniques of test appraisal with the aim of making analysis of test results more individually useful to each learner. In the Kenyan evaluation system, most institutions use both formative and summative evaluation techniques. Theoretically, formative evaluation is meant to help learners to diagnose their weaknesses and correct them in preparation for the summative evaluation. However, more often than not, the formative score is taken as a cumulative assessment score and is then totalled up with the end-of-term summative score. In essence then, the learner is not given a fair chance to be assessed formatively.
Rather than wait for the learner to fail an examination then intervene, this model is an attestation of the fact that an early diagnosis of cognitive styles can be done to help learners and teachers to adjust accordingly. This can be done early as learner joins secondary school in Form 1. Going forward the teacher and the individual learner can work together to ensure that the learner does not get too comfortable or even worse, languish in his/her cognitive comfort zones. The learner can then be challenged early in their learning cycle to adapt new and/or various learning strategies for the various learning tasks presented to them.
Ultimately, this study concluded with the following facts:
(1) | The cognitive styles of about 89% of students matched those of their teachers to a level of 50% and above; about 25% of students had a 100% match in cognitive styles with their respective teachers, 32% had a 75% match, 31% had a 50% match, 11% had a 25% match and less than 1% had a 0% match. | ||||
(2) | Performance in both Mock and KCSE Chemistry Examinations did not differ significantly among students in the six schools; School 1 stood out as a high performer in both examinations. | ||||
(3) | Out of the four dimensions of style, only the sequential–global scale that showed significance in the prediction model. This could be explained with the reasoning that the predictor variables were being considered in terms of interaction with other variables, thereby making the sequential–global dimension a more formidable predictor in interaction. | ||||
(4) | regression equation was structured that could predict KCSE performance of a learner (y) based on four regressor varibales: performance in Mock (X1), level of student–teacher cognitive styles match (X2), level of learner on the sequential–global scale (X3) and age of respondent (X4). |
The regression equation can significantly predict 62.8% of performance.
Findings of this study support the Four Matrix (4MAT) theory by McCarthy (Citation1990) that cognitive preferences do exist, they can be delineated and that they do have instructional impact on both the learner and the teacher. The findings also support those of Cafferty (Citation1980) Kai-Ming (Citation1997), Cano (Citation1999), Alamolhodaei (Citation2001), Grimley and Banner (Citation2008) and Atkinson (Citation2010) who underscored the importance of cognitive styles in learner performance. The relationship between cognitive styles and performance as has been established in this study can be used to objectively pinpoint systematic pedagogical weaknesses which are likely to undermine performance for certain categories of learners. In the Kenyan evaluation system, most institutions use both formative and summative evaluation techniques. Theoretically, formative evaluation is meant to help learners to diagnose their weaknesses and correct them in preparation for the summative evaluation. However, more often than not the formative score is taken as a cumulative assessment score and is then totalled up with the summative score. In essence then, the learner is not given a fair chance to be assessed formatively.
Although the findings of this study are compelling, it should be noted that given the constraints of time and availability of funds, we sampled six out of 60 schools that had been elevated to National School status in Kenya between 2011 and 2012. Since the study was limited to this category of schools, generalisation to any other school outside of this grouping should be done with caution. Further, the study used self-reports to measure cognitive styles of students and teachers; unlike methods such as direct observation, self-reporting has some inherent weakness. In self-reporting, there was a likelihood that a respondent could have overestimated, underestimated or even misreported on the constructs under study.
5. Recommendations
The following were the study recommendations for practice:
(1) | Early in the learners’ educational cycle, teachers should (i) determine their own cognitive styles and (ii) get to know their learners’ cognitive styles to enrich the teaching–learning process. | ||||
(2) | Depending on the content matter being taught, teachers and learners both need to shift from their cognitive comfort zones so that they can consciously explore and adopt a variety of learning strategies. | ||||
(3) | Teacher training institutions should include the concept of cognitive styles in the teacher training curriculum. |
Recommendations for further research
(1) | Being that this study only sampled Chemistry as a subject, it is suggested that in further research, educational psychologists should explore the effect of congruence in cognitive styles on performance of the learner in a range of subjects. Further, in profiling cognitive styles, researchers should use objective methods (rather than self-reports) to determine the styles. |
Funding
The authors received no direct funding for this research.
Additional information
Notes on contributors
Lusweti Sellah
Lusweti Sellah, PhD, is a lecturer in the Department of Educational Psychology and Special Needs at Pwani University, Kenya. Her research interests are in educational research and evaluation, with a specific interest in objectivity and quality in educational measurement. Prof Mondoh Helen is the Director of Quality Assurance and Kwena Jacinta, PhD, is the Dean of the School of Education at the same University, their interests lie in improvement of quality of education. This study speaks to the need to validly measure academic performance and to improve the quality of measurement techniques. On the basis that people process information differently, this study brought to the fore the fact that learning activities and assessment techniques may have biases that predispose some learners to failure that is preventable.
References
- Alamolhodaei, H. (2001). Convergent/divergent cognitive styles and mathematics problem solving. Journal of Science and Mathematics Education in South East Asia, 24(2), 102–107.
- Aljojo, N. M. (2012). Teacher assisting and subject adaptive material system: An Arabic adaptive learning environment (Thesis submitted in partial fulfilment of the requirements of Doctor of Philosophy degree in the School of Computing). University of Portsmouth.
- Atkinson, S. (2010). A comparison of pupil learning and achievement in computer aided learning and traditionally taught situations with special reference to cognitive style and gender issues. International Journal of Experimental Education Psychology, 24(5), 659–679.
- Brandon, P., Jordan, C., & Higa, T. A. (1995). Why Hawaii girls outperform Hawaii boys: The influence of immigration and peer culture. In P. Rogers & G. Kaiser (Eds.), Equity in mathematics education: Influences of feminism and culture (pp. 99–108). London: Taylor & Francis Group.
- Buli-Holmberg, J., Schiering, M., & Bogner, D. (2008). Awareness of thinking and feelings as a natural process for every learner: Implications for guidance. REOP, 19(2), 123–137.
- Cafferty, E. I. (1980). An analysis of student performance based upon the degree of match between the educational cognitive style of the teacher and the educational cognitive style of the students. Retrieved from http://www.digitalcommons.unl.edu/dissertations/AA18101213
- Cano, J. (1999). The relationship between learning styles, academic major, and academic performance of college students. Journal of Agriculture Education, 40(1), 30–37.
- Douglas, A. T. (2003). Cognitive style and learning strategies. Retrieved May 20, 2010, from Downloaded 20/05/2010 http://www.monografias.com/trabajos16/learning-styles/learning-styles.shtml
- Evans, C. (2010). Exploring the relationship between cognitive style and teaching style. International Journal of Experimental Education Psychology, 24(4), 509–530.
- Franzoni, A. L., & Assar, S. (2009). Student learning styles adaptation method based on teaching strategies and electronic media. Educational Technology and Society, 12(4), 15–29.
- Gellel, A. (2005). Can cognitive style predict scholastic performance? A study of 13 year olds. A paper presented at the 11th biennial conference of the European Association for Research on Learning and Instruction, Nicosia, Cyprus.
- Grimley, M. (2007). An exploration of the interaction between speech rate, gender, and cognitive style in their effect on recall. International Journal of Experimental Education Psychology, 27(3), 401–417.
- Gregorc, A. F. (1982). Gregorc style delineator. Maynard, MA: Gabriel Systems.
- Grimley, M., & Banner, G. (2008). Working memory, cognitive style, and behavioural predictors of GCSE exam success. International Journal of Experimental Education Psychology, 28(3), 341–351.
- Hudson, L. (1967). Contrary imaginations; a psychological study of the English Schoolboy. Harmondsworth: Penguin.
- Hyde, J. S. (2005). The gender similarities hypothesis. American Psychologist, 60(6), 581–592.10.1037/0003-066X.60.6.581
- Ibrahim, U. M., & Aljughaiman, A. (2012). The relationship between cognitive styles, Sternberg’s triarchic ability test, and Ranen’s standard progressive matrices in gifted students. Retrieved November 26, 2016, from http://aljughaiman.net/wp-content/uploads/2012/06/COGNITIVE...pdf
- Kagan, J., Rosman, B. L., Day, D., Albert, J., & Phillips, W. (1964). Information processing in the child: Significance of analytic and reflective attitudes. Psychological Monographs: General and Applied, 78(1), 1–37.10.1037/h0093830
- Kai-Ming, A. A. (1997). Cognitive style as a factor influencing performance of business students across various assessment techniques. Journal of Managerial Psychology, 12(4), 243–250.10.1108/02683949710174847
- Kenney-Benson, G. A., Pomerantz, E. M., Ryan, A. M., & Patrick, H. (2006). Sex differences in math performance: The role of children’s approach to schoolwork. Developmental Psychology, 42(1), 11–26.10.1037/0012-1649.42.1.11
- Kirton, M. (1976). Adaptors and innovators: A description and measure. Journal of Applied Psychology, 61(5), 622–629.10.1037/0021-9010.61.5.622
- Kolb, D. A. (1984). Experiential learning inventory; A self-description of preferred learning modes. Boston, MA: McBer.
- Maccoby, E. E., & Jacklin, C. (1974). The psychology of sex differences. Palo Alto, CA: Stanford University Press.
- McCarthy, B. (1990). Using the 4MAT system to bring learning styles to schools. Retrieved March 13, 2013, from http://www.ascd.org/ASCD/pdf/journals/ed_lead/el_199010_mccarthy.pdf
- Ornstein, R. E. (1973). The nature of human consciousness. San Francisco. CA: The Viking Press.
- Pask, G. (1976). Styles and strategies of learning. British Journal of Educational Psychology, 46, 128–148.10.1111/bjep.1976.46.issue-2
- Peklaj, C. (2003). Gender, abilities, cognitive style and student achievement in cooperative learning. Horizons of Psychology, 12(4), 9–22.
- Riding, R. J., & Cheema, I. (1991). Cognitive Styles – An overview and integration. Educational Psychology, 11, 193–215.10.1080/0144341910110301
- Riding, R. J., & Grimley, M. (1999). Cognitive Styles and learning from multimedia 11-year-old children. British Journal of Educational Psychology, 30, 43–56.
- Rochford, R. A., & Mangino, C. (2006). Are you teaching the way your students learn? Radical Pedagogy, 8(1), 19.
- Russell, A. J. (2010). The effect of learner variables cognitive styles on learning performance in a vocational training environment. Education Psychology: An International Journal of Experimental Educational Psychology, 17(1–2), 195–208.
- Samms, C. L. (2010). Relationship between dissimilar cognitive styles, use of coping behaviour and use of learning strategies (A dissertation submitted in fulfillment of requirements for the degree of Doctor of Philosophy for Louisiana State University).
- Santo, E., Soares, C. Q., Silveira, E. C., & Oliveira, R. (2015). A learning styles comparative study from high level students in face to face and distance education. Debate Universitario, 2314–1530.
- Spoon, J. C., & Schell, J. W. (1998). Aligning student learning styles with instructor teaching styles. Journal of Industrial Teacher Education, 35(2), 41–56. Retrieved from http://scholar.lib.vt.edu/ejournals/JITE/v35n2/spoon.html.
- Wadsworth, B. J. (1971). Piaget’s theory of cognitive development: An introduction for students of psychology and education. New York, NY: McKay.
- Witkin, H. A. (1973). The role of cognitive styles in academic performance and in teacher-student relations. A paper presented at the Cognitive Styles, Creativity and Higher Education symposium. Montreal, Canada. Nov, 1972.
- Witkin, H., Oltman, P., Raskin, E., & Karp, S. (1971). A manual for the group embedded figures test. Palo Alto, CA: Consulting Psychologists Press.
- Zhang, L. (2007). Does student-teacher thinking style match/mismatch matter in students’ achievement? International Journal of Experimental Education Psychology, 26(3), 395–409.