Abstract
Recommended psychological treatment for obsessive-compulsive disorder (OCD) is cognitive behavioral therapy (CBT) including exposure with response prevention (ERP). Previous studies have identified few consistent predictors of both short- and long-term treatment outcomes in CBT for OCD. The aim of the current study was to investigate the relation between the combined burden of three post-treatment predictors (subclinical symptoms of OCD, anxiety, and depression) and long-term treatment outcome. A total of 97 patients with a diagnosis of OCD from an outpatient clinic in Norway completed a concentrated four-day group treatment of ERP for OCD. Results revealed that patients in the high-risk group had 2.5 times the risk (odds ratio = 10.1) of having OCD at follow-up compared to patients in the low risk group. Pre-treatment levels of depression, anxiety and OCD were not significantly related to follow-up status. The results indicate an advantage of a combined post-treatment predictor model over single pre-treatment predictors. Furthermore, we argue that the intensive four-day treatment format provides a unique setting for investigating predictors of treatment outcome.
Public Interest Statement
Obsessive-compulsive disorder (OCD) is a psychiatric disorder characterized by intrusive thoughts (obsessions) which provoke strong anxiety. Patients try to control anxiety with rituals (compulsions). Recommended psychological treatments for OCD are cognitive behavioral therapy and exposure therapy. However, about 30% of patients do not benefit fully from treatment. We set out to investigate whether subclinical levels of anxiety, depression, and OCD symptoms at the end of treatment could predict long term treatment outcome. Our study revealed that 72% of patients with no risk factors succeeded in the long run, while only 17% of the patients with all tree risk factors succeeded. These results suggest that therapists should monitor these risk factors in order to avoid patients relapsing.
Competing Interests
The authors declare no competing interest.
1. Introduction
Cognitive behavior therapy (CBT) including exposure with response prevention (ERP) typically yields clinically significant change for 60–70% of the patients (Öst, Havnen, Hansen, & Kvale, Citation2015; Öst, Riise, Wergeland, Hansen, & Kvale, Citation2016; Skapinakis et al., Citation2016). Numerous studies have suggested a variety of predictors of treatment outcome in CBT and ERP for obsessive-compulsive disorder (OCD), with pre-treatment levels of depression, comorbid anxiety and OCD-severity among the most common. A review from 2008 suggests that severe depression pre-treatment, as opposed to mild or moderate depression, is related to treatment outcome (Keely, Storch, Merlo, & Geffken, Citation2008). However, the finding is not consistent (Knopp, Knowles, Bee, Lovell, & Bower, Citation2013; Olatunji, Davis, Powers, & Smits, Citation2013). In a review by Knopp et al. (Citation2013), one third of the included studies found a significant relation between anxiety pre-treatment and treatment outcome, but 2/3 did not, so again findings diverge. In the same review one third of the included studies reported a significant relation between baseline OCD severity and treatment outcome (Knopp et al., Citation2013). Age and gender have also been found to be related to effect sizes in CBT for OCD (Öst et al., Citation2015), but not consistently (Olatunji et al., Citation2013).
In sum, the results are inconsistent or conflicting, and so far no consistent predictors for treatment response have been identified (Keely et al., Citation2008; Knopp et al., Citation2013). However, a study using post-treatment levels of OCD-symptoms as predictor for long-term treatment outcome shows promising results. This study indicated that patients with full remission of OCD had a success rate of 70%, whereas patients with partial remission had a success rate of merely 45% (Eisen et al., Citation2013). On the other hand, Eisen and colleagues (Citation2013) did not investigate the combined effect of several predictors, and only about half of the participants (52%) received CBT. Post-treatment levels of symptoms have also been found to predict follow-up status in studies of depression and bipolar disorder (Judd et al., Citation2008; Kiosses & Alexopoulos, Citation2013). One possible explanation for the better predictive value of post-treatment score is that clinical aspects, such as anxiety and depression, are addressed directly or indirectly during treatment of OCD, and one could expect less change in such symptoms after treatment termination. In light of this, we propose that post-treatment variables should be better predictors of long term treatment outcome than pre-treatment predictors.
Previous research has been unable to pinpoint stable single predictors of treatment outcome. Research on resilience suggests a cumulative stress model, where elevated levels of stress increase the risk for onset and maintenance of psychological problems (Rutter, Citation2001). Symptoms of depression, anxiety and OCD have all been linked to increased levels of stress as well as functional impairment (Eisen et al., Citation2006; Mendlowicz & Stein, Citation2000; Pruessner, Hellhammer, Pruessner, & Lupien, Citation2003). Furthermore, even subthreshold levels of psychiatric symptoms have been linked to increased impairment (Eisen et al., Citation2013; Grabovich, Lu, Tang, Tu, & Lyness, Citation2010; Olfson et al., Citation1996). In line with this, the combined influence of several predictors should be stronger than the effects of single predictors.
Previous predictor studies have displayed several shortcomings. For instance different treatments have been offered in different predictor studies (CBT vs. SSRI; in-patients vs. out-patients etc.), making it difficult to compare findings. Furthermore, therapist competency is often not controlled for. In a newly developed treatment format (Havnen, Hansen, Haug, Prescott, & Kvale, Citation2013; Havnen, Hansen, Öst, & Kvale, Citation2014, Citation2017), CBT is delivered during four consecutive days, which dramatically reduces unwanted variability in predictor studies, such as extra-therapeutic factors (e.g. effects of time, life events, and therapist competency), through shortening the treatment time period and thorough therapist training (see closer description of the treatment format below). This approach yields significant acute (one week post treatment) changes in approximately 80% of the patients, with 66% being classified as recovered at follow-up, and with practically no patients terminating the treatment prematurely (Havnen et al., Citation2013, Citation2014). The four-day format thus provides a unique opportunity to study long-term treatment response.
In the current study we wanted to investigate if a composite reflecting the combined effect of residual symptoms of OCD, anxiety and depression at post-treatment predicts long-term treatment outcome. The study was conducted with treatment-seeking patients from an ordinary specialist healthcare clinic which yields high ecological validity. We expected that even subclinical levels of these factors could represent vulnerability for worse outcome. We also expected that the combined power of all three predictors would be stronger than single predictors’ individual effects in relation to OCD at follow-up because of the increased burden and possible functional impairment caused by them. The identification of strong predictors for treatment outcome is important because it can be used to guide individual tailoring of treatment, and to guide future search for consistent predictors of outcome. The study is unique in the sense that it uses an intensive group therapy of four days instead of a more traditional treatment format, the use of post-treatment predictors instead of pre-treatment predictors, and that it looks at several risk factors combined as opposed to looking at single predictors.
2. Method
2.1. Participants and procedure
The current study is part of a standard quality assurance procedure in an outpatient OCD clinic in Norway. The clinic is part of the ordinary specialist healthcare, where all OCD patients in a catchment area of about 400,000 inhabitants are referred to the OCD-clinic via their general practitioner and local psychiatric department. The patients are granted admission to the OCD-Team if their OCD is considered severe enough to need treatment. Using the Mini International Neuropsychiatric Interview (Sheehan et al., Citation1998), trained therapists at the OCD-Team assessed diagnostic status of the patients. Patients were offered treatment if they fulfilled the diagnostic criteria of OCD (American Psychiatric Association, Citation2000). The four-day treatment format was not offered if the patient was psychotic, suicidal, or had ongoing drug abuse. Furthermore, the patients had to be fluent in Norwegian. Patients were urged to discontinue any use of anxiolytics prior to treatment. The rationale for doing so was that patients should not use anxiolytics to regulate their anxiety while doing exposure treatment. A total of 32 treatment groups were offered between July 2012 and February 2016.
As treatment was delivered in a routine care clinic and patients had the right to be granted treatment within 12 weeks after referral, waiting-list was not an option. Patients were offered participation in the groups whenever there was an open slot. Due to seasonal variation in referrals, group size ranged from three to seven patients per group. During the actual period, 15 patients who were initially offered participation in a treatment group did not participate for various reasons. One patient due to substance abuse, one patient needed treatment for an eating disorder, two became sick as the group was about to start and one was unable to participate because of a work situation. About 11 patients did not participate in the first open slot because of sickness or equivalent reasons, but later participated in another group. No patients dropped out during the four-day treatment. In the current paper, only patients who had responded to all relevant questionnaires and interviews were included.
A total of 97 patients (72.2% females) who completed the concentrated treatment were included. Age ranged from 18 to 68 years (M = 31.45, SD = 10.38). A total of 43 patients (44%) had one or more comorbid psychiatric diagnosis, of which the most common were depression (n = 12, 12.4% of total sample), generalized anxiety disorder (n = 10, 10.3%), and social anxiety disorder (n = 5, 5.2%). Forty patients (41.2%) reported having received treatment previously for their OCD.
All patients received the Bergen concentrated exposure treatment (cET). We only outline the treatment briefly here, and refer to Havnen et al. (Citation2013, Citation2014) for more thorough descriptions. The four-day format has been labelled individual treatment in a group setting, since the patient-therapist ratio is 1:1. This allows for individually tailored and therapist assisted treatment while simultaneously taking advantage of the group setting where the patients can observe change in others and offer support. The first day of treatment involves psychoeducation and exposure planning. The second and third days are assigned to therapist-assisted exposure. On the fourth day, the patients discuss “lessons learnt”, and plan self-exposure for the next three weeks. An open trial reports that 77% of the patients recovered following cET (defined by Yale-Brown Obsessive Compulsive Scale (Y-BOCS) score of 14 or below, as well as a reduction of at least 10 points on the Y-BOCS from pre to post-treatment), and 65.7% of the patients were classified as recovered at 6 month follow-up (Havnen et al., Citation2014). Furthermore, drop-out rates and rates of people declining treatment have been reported to be remarkably low for the four-day treatment format; zero patients dropped out and no patients declined treatment in the pilot-study (Havnen et al., Citation2013).
2.1.1. Therapist competency
All therapists were clinical psychologists or psychiatrists with extensive OCD-relevant clinical experience (ranging from <1 to 30 years). All had undergone a comprehensive training and certification in cET. The training comprises a multiple-choice exam, hands-on clinical training, and supervision from experienced cET therapists.
2.1.2. Measures
Pre-treatment measures were filled out by the patients before treatment initiation and returned to their therapist when patients attended their screening interview. Post-treatment self-report measures were completed by the patients one week after termination of the four-day treatment (i.e. after one week of self-exposure at home) and returned to the clinic in pre-paid envelopes. Since the current study is based on an unselected treatment-seeking patient sample, all measures were collected as part of standard quality improvement. Due to this, some of the instruments for anxiety and depression were replaced with briefer screening instruments half-way through the current time-frame. The measures were used at pre-treatment, post-treatment, and 6-months follow-up.
2.1.2.1. OCD symptom severity
A clinician assessed patients before treatment using the Y-BOCS interview (Goodman, Price, Rasmussen, Mazure, Fleischmann, et al., Citation1989). An independent assessor conducted Y-BOCS interviews by phone one week after termination of treatment and at follow-up (3 and 6 months post-treatment). All clinicians completing the Y-BOCS interview were specifically trained in the procedure. The Y-BOCS interview has been shown to have good psychometric properties, and to be sensitive to change after treatment (Goodman, Price, Rasmussen, Mazure, Delgado, et al., Citation1989; Goodman, Price, Rasmussen, Mazure, Fleischmann, et al., Citation1989).
The Y-BOCS 6-month score was used as indicator of long-term treatment response. We merged data from the two Y-BOCS follow-up assessments, using the 6-month follow-up data when available (n = 79) and supplementing with 3-month follow-up data when 6-month follow-up (n = 18) were unavailable. A paired-samples t-test showed no significant difference between the 3-month scores and the 6-month scores (t(65) = −1.33, p = .19, mean difference = −.95).
2.1.2.2. Anxiety
The patients who participated in the first treatment groups (about half of the patients) filled out Beck Anxiety Inventory (BAI; Beck, Epstein, Brown, & Steer, Citation1988). The patients who participated in the remaining groups filled out the Generalized Anxiety Disorder 7-item scale (GAD-7; Spitzer, Kroenke, Williams, & Löwe, Citation2006). This is a briefer self-report measure which has shown good reliability and validity (Löwe et al., Citation2008). Both GAD-7 and BAI has been proved to yield a good measurement of anxiety (Beck & Steer, Citation1991; Spitzer et al., Citation2006). Furthermore, the correlation between GAD-7 and BAI has been reported to be strong (r = .72), supporting the assumption that the two measures assess similar aspects of anxiety (Spitzer et al., Citation2006).
2.1.2.3. Depression
Patients from about half of the treatment groups filled out Beck Depression Inventory, revised version (BDI-II; Beck, Steer, & Brown, Citation1996), which has been shown to have good psychometric properties (Titov et al., Citation2011). The second half of patients filled out the Patient Health Questionnaire (PHQ-9; Spitzer, Kroenke, Williams, & Patient Health Questionnaire Primary Care Study Group, Citation1999), which gives a briefer measure of depressive symptoms. The PHQ-9 has been shown to be an adequate screening instrument for depression both in medical settings (Gilbody, Richards, Brealey, & Hewitt, Citation2007) and in the general population (Martin, Rief, Klaiberg, & Braehler, Citation2006), and has been found to correlate strongly with BDI-II (r = .72–.77; Kung et al., Citation2013; Titov et al., Citation2011).
2.1.2.4. Cut-off criteria for predictor variables
Cut-off points were set to differentiate a dichotomous high risk vs. low-risk group variable where the low-risk group showed none or very mild symptoms, whereas the high risk group showed mild to severe symptoms at post-treatment. The cut-off criteria also had to yield a similar proportion of high risk and low risk participants when using either the BAI or the GAD-7, as well as for the BDI-II and the PHQ-9.
The cut-off criteria were set to 9 for GAD-7 (Spitzer et al., Citation2006) and BAI (Julian, Citation2011), corresponding to mild anxiety. With these cut-off criteria, 56.8% of the patients who had answered the BAI and 54.7% of the patients who had filled out GAD-7 were classified as “at risk”.
For the PHQ-9 the cut-off criterion was set to 12 (Löwe et al., Citation2004), and for BDI-II it was set to 13 (Salkind, Citation1969), corresponding to mild depression. With these criteria, 34.1% of the patients who filled out BDI-II, and 37.7% of the patients who filled out the PHQ-9 were classified as “at risk”.
For symptoms of OCD the cut-off criterion was set to 11 on the Y-BOCS, i.e. mild OCD (between asymptomatic and cut-off suggested by Fisher & Wells, Citation2005). This cut-off criterion placed 47.4% of the patients in the “at risk” group.
Patients were defined as “high risk” if they scored above cut-off on all three scales (anxiety, depression and Y-BOCS post-treatment), and “low risk” if they scored below cut-off on at least one of the scales. Details of mean scores and standard deviations on Y-BOCS, BDI, BAI, PHQ-9 and GAD-7 are displayed in Table .
Table 1. Measures of psychiatric symptoms
2.2. Statistical analyses
The maximum amount of missing items per questionnaire for this sample was 2. Missing items were replaced with the average score on the questionnaire for the individual patient.
Treatment status at follow-up was based on Y-BOCS scores. Clinical meaningful change was investigated using criteria recommended by Mataix-Cols et al. (Citation2016), which states that a patient can be considered in remission with a Y-BOCS score of ≤12, and that a change in Y-BOCS score of ≥35% can be considered a significant treatment response. In order to assess the predictors individually we used three chi-square (χ2) tests checking whether high vs. low-scorers on depression, anxiety and Y-BOCS post-treatment were related to remission status at follow-up (i.e. Y-BOCS above or at/below 12).
A multiple regression analysis was also used to investigate the predictive effects of continuous variables (age, gender and post-treatment scores on anxiety, depression and OCD) on Y-BOCS follow-up scores. To incorporate different measures of depression and anxiety in the analysis, depression and anxiety scores were converted to z-scores before running the analysis. We controlled for age and gender in all regression analyses, based on the findings that age and gender might be significantly related to effect sizes in studies of CBT for OCD (Öst et al., Citation2015).
To further investigate the relationship between predictors and follow-up status, we ran three logistic regression analyses. Logistic regression analyses were chosen because it provides a way of dealing with the different measures applied in the clinic. Through dichotomizing the variables we aimed at equalizing the different predictors so that it would be possible to combine them into single predictors (anxiety and depression), yielding better power than the different measures by themselves. Furthermore, the use of dichotomous cut-off criteria might be easier and more intuitive to apply in the clinic compared to continuous variables for identifying patients at risk. The questionnaires are often used in this way in the clinic as the forms have cut-offs for mild, moderate and severe levels of distress. In the first logistic regression analysis, age, gender and all three risk factors were entered separately as independent variables. Y-BOCS-score at follow-up (above or at/below 12) was entered as dependent variable. In the second logistic regression analysis, age, gender and the aforementioned new variable (“high risk” vs. “low risk” patients’) were entered as independent variables, and again categorical Y-BOCS score at follow-up was entered as dependent variable. Then we ran the second analysis again, this time excluding all patients who had not recovered post-treatment (i.e. Y.BOCS > 12) to make sure the results in the second regression analysis were not a product of non-recovered patients scoring high on the Y-BOCS both post-treatment and at follow-up. We converted odds ratio to risk ratio using online software (http://clincalc.com).
We then investigated patients who relapsed vs. patients who remained in remission with t-tests, to find out whether there was a significant difference in demographic factors (age and gender) as well as risk factors for relapsed vs. non-relapsed patients. Relapse was defined using criteria suggested by Mataix-Cols et al. (Citation2016); the patient initially responded to treatment (defined by a Y-BOCS score of 12 or lower) and no longer met this criteria at follow-up (i.e. a Y-BOCS score of 13 or higher). This procedure was not performed for responders (i.e. failing to meet the 35% reduction criteria after initial response and remission) as suggested by Matiax-Cols and colleagues (Citation2016), as this applied for only two patients.
Finally, to examine whether post-treatment predictors were truly better related to follow-up status, we investigated whether pre-treatment levels of depression (measured with PHQ-9), anxiety (measured with GAD-7) and OCD (measured with Y-BOCS) were related to Y-BOCS scores at follow-up using bivariate correlations. A hierarchical linear regression analysis was then run to investigate the effects of age, gender, anxiety pre-treatment (measured with GAD-7), depression pre-treatment (measured with PHQ-9) and Y-BOCS pre-treatment on Y-BOCS score at follow-up. The pre-treatment measures were not analyzed using logistic regression analyses as described above, as all patients scored above cut-off on OCD before treatment. T-tests were used to investigate whether mean scores on pre-treatment characteristics (age, gender, Y-BOCS scores, GAD-7 scores and PHQ-9 scores) were different for remission vs. non-remission (i.e. Y-BOCS ≤ 12) post-treatment.
3. Results
Nearly 90% (87.6% (n = 85)) of the patients responded to treatment (i.e. had a reduction in symptoms of 35% or more from pre- to post-treatment). The corresponding figure for follow-up was 74.2%. Seventy-two patients (74.2%) were in remission (Y-BOCS ≤ 12) post-treatment, and 56.7% had symptom remission at follow-up. Mean Y-BOCS scores at pre- and post-treatment and follow-up are displayed in Table .
When applying the cut-off criteria as described above for the risk factors, 46 patients (47.4%) scored above cut-off on OCD (Y-BOCS ≥ 11), 54 (55.7%) scored above cut-off on anxiety and 35 (36.1%) scored above cut-off on depression post-treatment. When counting number of risk factors, 25 patients had no risk factors, 27 patients had 1 risk factor, 27 patients had 2 risk factors and 18 patients had all three risk factors. In other words, 18 patients were in the “high risk group”, displaying all three risk factors, while 79 patients were in the “low risk” group, displaying 2 or less risk factors. In the last logistic regression analysis where non-responders were removed from the analysis, 8 patients were classified as “high risk” and 64 patients were classified as “low risk”.
Three χ2 tests were performed to investigate the relation between “at risk” scores on depression, anxiety and Y-BOCS post-treatment and remission at follow-up. We found a significant relation between OCD follow-up status and depression post-treatment (χ2 (1, N = 97) = 8.53, p = .003), anxiety post-treatment (χ2 (1, N = 97) = 5.37, p = .02) as well as Y-BOCS score post-treatment (χ2 (1, N = 97) = 6.23, p = .013). All three risk factors were significant as single predictors of long-term outcome.
A multiple regression analysis was also used to investigate the predictive effect of continuous variables (i.e. age, gender and post-treatment scores on anxiety, depression and OCD) on Y-BOCS scores at follow-up. The results of the regression indicated the predictors explained 23% of the variance (R2 = .23, F(5,91) = 5.53, p < .01). However, the only significant predictor was Y-BOCS post treatment (p < .01). Neither; age (p = .09), gender (p = .42), anxiety post-treatment (p = .09) nor depression post-treatment (p = .87) significantly contributed to the model.
A logistic regression analysis was used to investigate the predictive effects of age, gender, and all three risk factors separately for remission status at follow-up. Age (p = .072), gender (p = .59) and anxiety scores post-treatment (p = .48) were not significantly related to remission status at follow-up. Symptoms of depression (p < .05) and OCD (p < .05), on the other hand, were significantly related to remission at follow-up. All logistic regression analysis results are presented in Table .
Table 2. Summary of logistic regression analysis for post-treatment variables predicting symptom remission at follow-up
A second logistic regression analysis investigated the effects of low vs. high-risk patients for remission status at follow-up. Age and gender were again added to the regression. Whereas age (p = .21) and gender (p = .39) were not significant contributors to the model, having three risk factors (i.e. high scores on depression, anxiety and OCD post-treatment) was significantly associated with worse outcome (p < .01). As can be seen in Table , this yielded an odds ratio of 10.09. Converting this odds ratio to risk ratio gave a risk ratio of 2.46. Figure displays graphically how the number of risk factors lowered treatment success rate.
Figure 1. Remission rates at follow-up decrease with each added post-treatment risk factor.
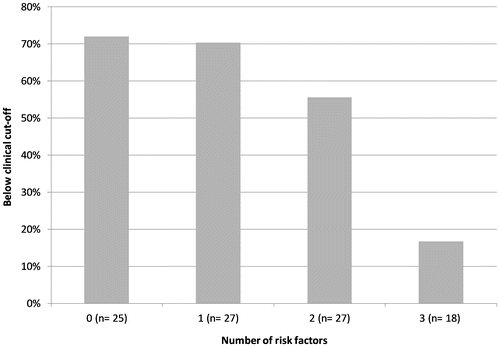
Then we reran the second logistic regression analysis, excluding the patients who had not recovered post-treatment (n = 25). Age (p = .07) and gender (p = .71) were not significant contributors to the model. Having all three risk factors was significantly related to worse outcome (p < .05), again with a high odds ratio (8.71; risk ratio 2.65).
We then investigated relapsed vs. non-relapsed patients as defined above, excluding patients who did not respond to treatment initially (i.e. Y-BOCS > 12 post-treatment). Twenty-five patients relapsed between post-treatment and follow-up. Mean change in Y-BOCS score from post-treatment to follow-up for the relapse group was 8.76 (SD = 6.75). Average Y-BOCS score at follow-up was 6.87 for the non-relapsed group and 17.40 for the relapsed group, and the difference was significant (t(72) = 9.88, p < .001). The corresponding figures for pre-treatment and post-treatment Y-BOCS scores were 25.30 and 7.94 for the non-relapsed group and 24.24 and 8.64 for the relapsed group. Neither pre-treatment scores (t(72) = .91, p = .37), nor post-treatment scores (t(72) = −.86, p = .39) were significantly different between the two groups. T-tests revealed no differences in age (t(72) = 1.65, p = .10) nor gender (t(72) = .26, p = .80) between the relapse vs. non-relapse patients. However, the relapse group had significantly more risk-factors post-treatment (M = 1.52, median = 2, mode = 2, SD = .1.16) compared to the non-relapse group (M = .94, median = 1, mode = 0, SD = .89), t(72) = 2.38, p < .05).
To examine whether post-treatment predictors were better related to follow-up status than pre-treatment predictors, we investigated the correlation between pre-treatment levels of depression, anxiety, and OCD and Y-BOCS scores at follow-up. Neither symptoms of depression (r = −.02, n = 65, p = .88), anxiety (r = −.07, n = 67, p = .57) nor OCD (r = −.02, n = 97, p = .88) at pre-treatment were significantly correlated with follow-up status. We ran a hierarchical regression analysis to investigate the relation further. The regression equation was not significant (F(5,58) = .97, p = .45). Neither age (p = .14), gender (p = .94), anxiety pre-treatment (p = .09), depression pre-treatment (p = .25) nor OCD pre-treatment (p = .71) were significantly related to follow-up status. In summary, none of the risk factors pre-treatment were significantly related to follow-up status.
Having investigated pre-treatment predictors for follow-up status, we finally investigated pre-treatment predictors of post-treatment status. Independent sample t-tests were used to investigate whether mean scores on pre-treatment characteristics were different for patients in remission vs. not in remission post-treatment. Whereas age (t(95) = .12, p = .90), OCD (t(95) = −.08, p = .28), anxiety (t(65) = .13, p = .89) and depression (t(63) = 1.09, p = .28) were not significantly different for remission vs. non-remission, gender was significantly different for the two groups (t(95) = −2.12, p < .05), with a larger proportion of men in the non-remission group (44%) compared with the remission group (22%).
4. Discussion
We investigated the relation between post-treatment risk factors and OCD symptom severity at follow-up using an unselected sample of treatment-seeking OCD patients receiving cET in the specialist health care. Nearly 90% of the patients responded to treatment initially, and 74.2% were in remission (Y-BOCS ≤ 12) post-treatment. The corresponding numbers for follow-up status were 74.2% responders and 56.7% in remission. Our results showed that patients with mild to moderate symptoms on measures of OCD-symptom severity, depression and anxiety at post-treatment had 2.5 times the risk (odds ratio = 10.1) of having OCD at follow-up. The results still held true when removing non-recovered patients from the analysis (odds ratio = 8.71). As can be seen in Figure , each additional risk factor seems to add risk for reduced treatment outcome. Patients who relapsed had significantly more risk factors post-treatment than non-relapsed patients. Pre-treatment levels of anxiety, depression and OCD, however, were not significantly related to Y-BOCS score at follow-up.
Previous studies have been inconsistent regarding the relation between pre-treatment levels of anxiety, depression and OCD-symptoms and treatment outcome (Keely et al., Citation2008; Knopp et al., Citation2013). The current study shows that pre-treatment predictors have weak predictive validity. However, the study revealed a strong relation between post-treatment predictors and long-term treatment outcome. Our study encourages the use of post-treatment predictors for long-term outcome. Eisen and colleagues (Citation2013) found that patients with full remission of OCD symptoms had a success rate of 70%, while patients with partial remission had a 40% success rate. The corresponding figures in our study indicated that patients with 0 risk factors had a 72.0% success rate, while the patients with 3 risk factors had a success rate of 16.7% (see Figure ). Furthermore, in our study the treatment was better controlled, as all patients received the four-day format delivered by trained therapists, while in the study by Eisen and colleagues, only 52% of the patients received CBT. In the study by Eisen et al., 36 patients had full remission and 47 patients had partial remission, and 59% relapsed. In our study, more people responded to treatment, less people relapsed and the predictive model proved stronger. Findings from the current study therefore replicates the study by Eisen et al. and extends it by showing that it is also important to monitor for symptoms of anxiety and depression in patients with OCD.
Furthermore, the study revealed a strong predictive effect of the combined burden of several post-treatment predictors. Our results underscore the importance of using several predictors as opposed to single predictors when investigating treatment outcome for psychiatric disorders, which are often complex and multifaceted. This is in line with research on the cumulative stress model from the resilience literature, which states that cumulative levels of stress increases the risk of maintenance of psychological problems (Rutter, Citation2001). While the patient might cope in face of moderate depression alone, the combined effects of depression, anxiety and OCD-symptoms could overwhelm the patient and might contribute to the higher OCD severity scores found in the high risk group. Several studies have investigated pathways through which stress can influence health (Rod, Grønbaek, Schnohr, Prescott, & Kristensen, Citation2009; Steptoe, Citation1991). For instance, Steptoe (Citation1991) and Rod and colleagues (Citation2009) highlight how stress can influence health related behaviors, such as smoking, alcohol use and regular exercise, thus possible worsening the burden for the patient.
Finally, our study supports the use of cut-off criteria for estimating risk of relapse as the multiple regression analysis using continuous variables were not able to identify the risk of having residual symptoms. We also argue the use of cut-off criteria is intuitive and easy to apply in the clinic to identify patients at risk of relapsing.
4.1. Strengths and limitations
One important limitation concerns the use of two different measures for anxiety (BAI and GAD-7) as well as for depression (BDI-II and PHQ-9). This was a result of the current study being part of the quality assurance procedures at an outpatient clinic in its early stages. We made careful steps to make the measures as equal as possible through matching the cut-off criteria and using categorical analyses. This allowed us to get a bigger sample, thus overall strengthening the analyses. Post-hoc we ran the main logistic regression analysis again, with only (1) PHQ-9, GAD-7 and Y-BOCS as risk factors, and (2) BDI, BAI and Y-BOCS as risk factors, both analyses controlling for age and gender. In the first analysis, the risk factors came out significantly related to follow-up status (p < .01), whereas in the second they did not (p = .10). Hence, GAD-7 and PHQ-9 might be better predictors of follow-up status and pick up different aspects of anxiety and depression than BDI and BAI. However, only seven patients were classified as “high risk” in the BDI/BAI analysis, making the sample size too small to draw firm conclusions. Another limitation concerns the urge of discontinuing use of anxiolytics prior to treatment. This was not monitored formally, and the discontinuation might possibly increase symptoms pre-treatment, representing a confounding variable.
Previous reports of the four-day treatment format have indicated that hardly any patients decline treatment, and that there are very few drop-outs (Havnen et al., Citation2013), yielding a sample with high ecological validity. Since the intervention is so concentrated, it reduces unwanted variability related to external factors confounding short-term treatment outcome. Also, since the intervention is clearly potent as indicated by nearly 90% of the patients being classified as responders post-treatment, it represents an ideal setting for studying post-treatment predictors for long-term outcome. Future studies should investigate whether our findings also hold true in a standard CBT format.
5. Conclusion and implications
The study found a strong association between the combined effects of post-treatment scores of depression, anxiety and OCD-symptoms and high Y-BOCS scores at follow-up. This collection of symptoms could indicate vulnerability for relapse. Our results indicate that clinicians should be aware of patients displaying these residual symptoms at the end of the treatment period, and maybe pay special attention to these patients in the period following treatment. We recommend future research to investigate alternative treatments for non-responders and patients with relapse, for example treatment with d-cycloserine and exposure therapy combined (Kvale & Hansen, Citation2016). We also recommend future research to use both pre- and post-treatment predictors when investigating predictors of treatment outcome.
Funding
The authors received no direct funding for this research.
Additional information
Notes on contributors
Silje Elisabeth Hasmo Holm
Silje Elisabeth Hasmo Holm is a clinical psychologist and PhD candidate in Helse Vest, Norway.
Bjarne Hansen
Bjarne Hansen is a clinical psychologist and associate professor at the University of Bergen.
Gerd Kvale
Gerd Kvale is a professor at the University of Bergen and the leader of the OCD-Team in Helse Bergen.
Thomas Eilertsen
Thomas Eilertsen is a clinical psychologist working in Helse Vest.
Torun Grøtte
Torun Grøtte is a PhD candidate at NTNU, Trondheim, Norway.
Stian Solem
Stian Solem is an associate professor at NTNU.
The OCD-Team in Helse Bergen offers evidence based therapy to patients with OCD. The team integrates clinical practice and research and has published several papers about the four-day intensive treatment. The current paper investigates the treatment format further, investigating predictors of treatment outcome. A list of research publications can be found at https://helse-bergen.no/ocd-teamet/publications.
References
- American Psychiatric Association. (2000). Diagnostic and statistical manual of mental disorders DSM-IV-TR (4th ed.). Washington, DC: Author.
- Beck, A. T., Epstein, N., Brown, G., & Steer, R. A. (1988). An inventory for measuring clinical anxiety: Psychometric properties. Journal of Consulting and Clinical Psychology, 56(6), 893–897. doi:10.1037/0022-006x.56.6.893
- Beck, A. T., & Steer, R. A. (1991). Relationship between the Beck anxiety inventory and the Hamilton anxiety rating scale with anxious outpatients. Journal of Anxiety Disorders, 5(3), 213–223. doi:10.1016/0887-6185(91)90002-B
- Beck, A. T., Steer, R. A., & Brown, G. K. (1996). Beck depression inventory-II. San Antonio, TX: Psychological Corporation.
- Eisen, J. L., Mancebo, M. A., Pinto, A., Coles, M. E., Pagano, M. E., Stout, R., & Rasmussen, S. A. (2006). Impact of obsessive-compulsive disorder on quality of life. Comprehensive Psychiatry, 47(4), 270–275. doi:10.1016/j.comppsych.2005.11.006
- Eisen, J. L., Sibrava, N. J., Boisseau, C. L., Mancebo, M. C., Stout, R. L., Pinto, A., & Rasmussen, S. A. (2013). Five-year course of obsessive-compulsive disorder: Predictors of remission and relapse. The Journal of Clinical Psychiatry, 74(3), 233–239. doi:10.4088/JCP.12m07657
- Fisher, P. L., & Wells, A. (2005). How effective are cognitive and behavioral treatments for obsessive–compulsive disorder? A clinical significance analysis. Behaviour Research and Therapy, 43(12), 1543–1558. doi:10.1016/j.brat.2004.11.007
- Gilbody, S., Richards, D., Brealey, S., & Hewitt, C. (2007). Screening for depression in medical settings with the Patient Health Questionnaire (PHQ): A diagnostic meta-analysis. Journal of General Internal Medicine, 22(11), 1596–1602. doi:10.1007/s11606-007-0333-y
- Goodman, W. K., Price, L. H., Rasmussen, S. A., Mazure, C., Delgado, P., Heninger, G. R., & Charney, D. S. (1989). The Yale-Brown obsessive compulsive scale: II. Validity. Archives of General Psychiatry, 46(11), 1012–1016. doi:10.1001/archpsyc.1989.01810110054008
- Goodman, W. K., Price, L. H., Rasmussen, S. A., Mazure, C., Fleischmann, R. L., Hill, C. L., … Charney, D. S. (1989). The Yale-Brown obsessive compulsive scale: I. Development, use, and reliability. Archives of General Psychiatry, 46(11), 1006–1011. doi:10.1001/archpsych.1989.01810110048007
- Grabovich, A., Lu, N., Tang, W., Tu, X., & Lyness, J. M. (2010). Outcomes of subsyndromal depression in older primary care patients. The American Journal of Geriatric Psychiatry, 18(3), 227–235. doi:10.1097/jgp.0b013e3181cb87d6
- Havnen, A., Hansen, B., Haug, E. T., Prescott, P., & Kvale, G. (2013). Intensive group treatment of obsessive-compulsive disorder: A pilot study. Clinical Neuropsychiatry, 10(3), 48–55.
- Havnen, A., Hansen, B., Öst, L.-G., & Kvale, G. (2014). Concentrated ERP delivered in a group setting: An effectiveness study. Journal of Obsessive-Compulsive and Related Disorders, 3(4), 319–324. doi:10.1016/j.jocrd.2014.08.002
- Havnen, A., Hansen, B., Öst, L.-G., & Kvale, G. (2017). Concentrated ERP delivered in a group setting: A replication study. Behavioural and Cognitive Psychotherapy, 1–7. doi:10.1017/s1352465817000091
- Judd, L. L., Schettler, P. J., Akiskal, H. S., Coryell, W., Leon, A. C., Maser, J. D., & Solomon, D. A. (2008). Residual symptom recovery from major affective episodes in bipolar disorders and rapid episode relapse/recurrence. Archives of General Psychiatry, 65(4), 386–394. doi:10.1001/archpsyc.65.4.386
- Julian, L. J. (2011). Measures of anxiety: State-Trait Anxiety Inventory (STAI), Beck Anxiety Inventory (BAI), and Hospital Anxiety and Depression Scale-Anxiety (HADS-A). Arthritis Care & Research, 63(11), 467–472. doi:10.1002/acr.20561
- Keely, M. L., Storch, E. A., Merlo, L. J., & Geffken, G. R. (2008). Clinical predictors of response to cognitive-behavioral therapy for obsessive–compulsive disorder. Clinical Psychology Review, 28(1), 118–130. doi:10.1016/j.cpr.2007.04.003
- Kiosses, D. N., & Alexopoulos, G. S. (2013). The prognostic significance of subsyndromal symptoms emerging after remission of late-life depression. Psychological Medicine, 43(2), 341–350. doi:10.1017/s0033291712000967
- Knopp, J., Knowles, S., Bee, P., Lovell, K., & Bower, P. (2013). A systematic review of predictors and moderators of response to psychological therapies in OCD: Do we have enough empirical evidence to target treatment? Clinical Psychology Review, 33(8), 1067–1081. doi:10.1016/j.cpr.2013.08.008
- Kung, S., Alarcon, R. D., Williams, M. D., Poppe, K. A., Moore, M. J., & Frye, M. A. (2013). Comparing the Beck Depression Inventory-II (BDI-II) and Patient Health Questionnaire (PHQ-9) depression measures in an integrated mood disorders practice. Journal of Affective Disorders, 145(3), 341–343. doi:10.1016/j.jad.2012.08.017
- Kvale, G., & Hansen, B. (2016, August 26). Translational approach to the understanding and treatment of obsessive-compulsive disorder (OCD). Can d-cycloserine enhance and stabilize the treatment-response in relapsed and non-responding OCD-patients? Retrieved December 21, 2016, from https://clinicaltrials.gov/ct2/show/study/NCT02656342#contacts
- Löwe, B., Decker, O., Müller, S., Brähler, E., Schellberg, D., Herzog, W., & Herzberg, P. Y. (2008). Validation and standardization of the Generalized Anxiety Disorder Screener (GAD-7) in the general population. Medical Care, 46(3), 266–274. doi:10.1097/mlr.0b013e318160d093
- Löwe, B., Spitzer, R. L., Gräfe, K., Kroenke, K., Quenter, A., Zipfel, S., … Herzog, W. (2004). Comparative validity of three screening questionnaires for DSM-IV depressive disorders and physicians’ diagnoses. Journal of Affective Disorders, 78(2), 131–140. doi:10.1016/s0165-0327(02)00237-9
- Martin, A., Rief, W., Klaiberg, A., & Braehler, E. (2006). Validity of the brief Patient Health Questionnaire mood scale (PHQ-9) in the general population. General Hospital Psychiatry, 28(1), 71–77. doi:10.1016/j.genhosppsych.2005.07.003
- Mataix-Cols, D., de la Cruz, L. F., Nordsletten, A. E., Lenhard, F., Isomura, K., & Simpson, H. B. (2016). Towards an international expert consensus for defining treatment response, remission, recovery and relapse in obsessive-compulsive disorder. World Psychiatry, 15(1), 80–81. doi:10.1002/wps.20299
- Mendlowicz, M. V., & Stein, M. B. (2000). Quality of life in individuals with anxiety disorders. American Journal of Psychiatry, 157(5), 669–682. doi:10.1176/appi.ajp.157.5.669
- Olatunji, B. O., Davis, M. L., Powers, M. B., & Smits, J. A. (2013). Cognitive-behavioral therapy for obsessive-compulsive disorder: A meta-analysis of treatment outcome and moderators. Journal of Psychiatric Research, 47(1), 33–41. doi:10.1176/appi.focus.130219
- Olfson, M., Broadhead, W. E., Weissman, M. M., Leon, A. C., Farber, L., Hoven, C., & Kathol, R. (1996). Subthreshold psychiatric symptoms in a primary care group practice. Archives of General Psychiatry, 53(10), 880–886. doi:10.1001/archpsyc.1996.01830100026004
- Öst, L.-G., Havnen, A., Hansen, B., & Kvale, G. (2015). Cognitive behavioral treatments of obsessive–compulsive disorder. A systematic review and meta-analysis of studies published 1993–2014. Clinical Psychology Review, 40, 156–169. doi:10.1016/j.cpr.2015.06.003
- Öst, L.-G., Riise, E. N., Wergeland, G. J., Hansen, B., & Kvale, G. (2016). Cognitive behavioral and pharmacological treatments of OCD in children: A systematic review and meta-analysis. Journal of Anxiety Disorders, 43, 58–69. doi:10.1016/j.janxdis.2016.08.003
- Pruessner, M., Hellhammer, D. H., Pruessner, J. C., & Lupien, S. J. (2003). Self-reported depressive symptoms and stress levels in healthy young men: Associations with the cortisol response to awakening. Psychosomatic Medicine, 65(1), 92–99. doi:10.1097/01.psy.0000040950.22044.10
- Rod, N. H., Grønbaek, M., Schnohr, P., Prescott, E., & Kristensen, T. (2009). Perceived stress as a risk factor for changes in health behaviour and cardiac risk profile: A longitudinal study. Journal of Internal Medicine, 266(5), 467–475. doi:10.1111/j.1365-2796.2009.02124.x
- Rutter, M. (2001). Psychosocial adversity: Risk, resilience and recovery. In J. M. Richman & M. W. Fraser (Eds.), The context of youth violence: Resilience, risk, and protection (pp. 13–41). London: Praeger.
- Salkind, M. R. (1969). Beck depression inventory in general practice. The Journal of the Royal College of General Practitioners, 18(88), 267–271 . Retrieved from http://bjgp.org/content/bjgp/18/88/267.full.pdf
- Sheehan, D., Lecrubier, Y., Sheehan, K. H., Amorim, P., Janavs, J., Weiller, E., … Dunbar, G. (1998). The Mini-International Neuropsychiatric Interview (M.I.N.I.): The development and validation of a structured diagnostic psychiatric interview for DSM-IV and ICD-10. Journal of Clinical Psychiatry, 59(Suppl. 20), 22–33.
- Skapinakis, P., Caldwell, D. M., Hollingworth, W., Bryden, P., Fineberg, N. A., Salkovskis, P., & Churchill, R. (2016). Pharmacological and psychotherapeutic interventions for management of obsessive-compulsive disorder in adults: A systematic review and network meta-analysis. The Lancet Psychiatry, 3(8), 730–739. doi:10.1016/s2215-0366(16)30069-4
- Spitzer, R. L., Kroenke, K., Williams, J. B., & Löwe, B. (2006). A brief measure for assessing generalized anxiety disorder: The GAD-7. Archives of Internal Medicine, 166(10), 1092–1097. doi:10.1001/archinte.166.10.1092
- Spitzer, R. L., Kroenke, K., Williams, J. B., & Patient Health Questionnaire Primary Care Study Group. (1999). Validation and utility of a self-report version of PRIME-MD: The PHQ primary care study. JAMA, 282(18), 1737–1744. doi:10.1001/jama.282.18.1737
- Steptoe, A. (1991). Invited review: The links between stress and illness. Journal of Psychosomatic Research, 35(6), 633–644. doi:10.1016/0022-3999(91)90113-3
- Titov, N., Dear, B. F., McMillan, D., Anderson, T., Zou, J., & Sunderland, M. (2011). Psychometric comparison of the PHQ-9 and BDI-II for measuring response during treatment of depression. Cognitive Behaviour Therapy, 40(2), 126–136. doi:10.1080/16506073.2010.550059