Abstract
In this study, we analyze the interaction between smartphones and their users as contingencies of reinforcement underpinning social behavior. We posit the introduction of a nudge: an environmental intervention meant to guide behavior that can be easily avoided in a social context. Our experiment takes us to an Italian pub with the hypothesis that a simple environmental factor (a basket featuring a social cue) will contribute to a reduction in digital social interactions in favor of physical social interactions. Data were collected employing a momentary time sampling where we recorded an increase in estimated time with no smartphone interactions and a decrease in estimated time with all the customers seated at one table using their smartphones in the experimental condition. These results were significant and suggest that the nudge was effective at reducing smartphone use among the patrons. Moreover, the estimates of these digital interactions were shorter for the statistical unit when compared to the control. Together, the results of study demonstrate that a nudge can reduce smartphone use in contexts of social interaction. However, it may be difficult to sustain alternative behavior without providing consequences that reinforce its future occurrences.
PUBLIC INTEREST STATEMENT
Although the use of smartphones has improved several aspects of our lives, their overuse can lead to social and psychological difficulties. In this experiment, we nudged the customers of an Italian pub to be more social with each other, and less through their smartphones. Although they were always free to choose, our results showed that introducing a smartphone basket with a social message decreased the use of smartphones. The basket in which the customers were nudged to place their smartphones served as an antecedent for shifting the contingencies of their behavior in a social setting: from their smartphones to their friends or colleagues seated at the same table. To our knowledge, this study is the first of its kind to introduce a digital detoxing nudge in the context of the Italian society. Moreover, we discuss the importance of analyzing contingencies of behavior as we design nudging interventions in social settings.
1. Introduction
1.1. Smartphone use and society
The spread and function of smartphones have changed dramatically in recent years, bearing important psychological (e.g., smartphone seduction and compulsive use), social (e.g., reduced quality of conversations) and political (e.g., polarization) issues (Peeples, Citation2018; see also Berenguer et al., Citation2017; Petrovčič et al., Citation2018). In countries characterized by high smartphone penetration (Gimpel et al., Citation2014) and in which smartphone adoption rates approached the late majority (Kim et al., Citation2014), the spread of smartphones is not necessarily bound to increase. Nevertheless, it is likely that smartphones will continue to become more ubiquitous and the time we spend on them will also increase, which may pose the risk of developing an addiction. Furthermore, smartphone adoption rates have increased dramatically over the past decade as it nears market saturation. The function of this technology has also increased, resulting in consumers of smartphones spending more time on their devices. We expect both trends to persist as the devices provide value for its users. Similar to other handheld devices, smartphones have shifted from being mere tools of communication to indispensable and inclusive technological devices. For example, they had strong impacts on societal mobility (Khan et al., Citation2020), personal identity (Damaševičius et al., Citation2016), information (Motamedi & Choe, Citation2015), commodity transactions (Marforio et al., Citation2014), and human relationships (Enez Darcin et al., Citation2016; Rhiu & Yun, Citation2018).
About half of the world’s population uses a smartphone, and about two-thirds own a mobile phone (We Are Social, Citation2017). The use of smartphones has penetrated almost all our daily life activities (Lee et al., Citation2014), which may suggest that digital users prefer browsing the Internet and using Web applications on their smartphones, rather than on their personal computers. Among the social media applications, Facebook is currently the most popular platform “with over 2.7 billion monthly active users as of the second quarter of 2020” (Clement, Citation2020). Moreover, there has been an exponential increase in the use of the instant text, image and video messaging applications, such as WhatsApp, Messenger, Instagram and Snapchat (We Are Social, Citation2017; Patmanthara et al., Citation2019). These and other social media applications are particularly interesting because they provide immediate and virtually endless access to social reinforcement (e.g., instant messaging, immediate and frequent feedback—“likes”). We argue that access to social reinforcement can not only sustain smartphone use at a steady rate but increase it further by introducing positive socially mediated consequences.
Although the widespread use of smartphones produces advantages in several domains, their overuse may produce negative consequences for physical and mental health that put individual wellbeing at stake (David et al., Citation2018). Previous research has found that regular and prolonged glances at smartphone screens (e.g., checking whether any incoming calls or messages were received) may lead to compulsive smartphone use (Bianchi & Phillips, Citation2005; Takao et al., Citation2009). For example, Oulasvirta et al. (Citation2012) showed that the participants in their research checked their smartphones 34 times a day, not because of a real need but rather of a rooted habit. From a behavioral perspective, this phenomenon can be termed as a behavioral addiction (Marks, Citation1990), which is characterized by a preference for immediate over delayed gratification (see also Rachlin, Citation2000).
Moreover, the overuse of smartphones was shown to heighten psychological distress (Chesley, Citation2005) and lead to other health issues, such as sleep disturbances and depression (Thomée et al., Citation2007, Citation2011). The term technostress intends the tension that end-users experience due to information and communication overload (Brod, Citation1984; Ragu-Nathan et al., Citation2008). The overuse of smartphones in social and recreational contexts can be associated with a reduction in the quality and quantity of social interactions (Geser, Citation2006). Engaging in a detoxification is one of the measures that may help smartphone users get back in the driver’s seat of their digitally mediated social interactions. Although it may sound as an overly invasive form of behavioral control, this is not the case for both choice and the availability of reinforcement are retained.
1.2. Aims of the study
The present study addresses contextual factors underlying and sustaining smartphone use behavior and how they can be manipulated to nudge more social interactions and less digital interactions whenever social reinforcement is available (i.e., in a social setting). We resort to the nudging framework and toolkit, which makes use of environmental cues to steer behavior and achieve better outcomes (Thaler & Sunstein, Citation2008). Nudging represents a promising paradigm for implementing social change. For example, it has been resorted to for increasing compliance (e.g., of handwashing; Dai, Milkman, Hofmann, & Staats, 2015) and persuading alternative choice behavior (e.g., reducing littering in the streets of Copenhagen; in Halpern, 2015). However, nudging is an extremely flexible approach that can be implemented for virtually all attempts to influence behavior as long as it is deployed for the “good” of people (see Thaler, Citation2018).
The primary aim of this study is to reduce the overuse of smartphones in social contexts by testing out a nudging exploratory study. The overuse of smartphones and other technologies poses not only a threat to our wellbeing but alters our social lives to the point of potentially harming society altogether (Montag et al., Citation2016, p. 1). Although it may not be possible to remove this source of reinforcement, nor should it be desirable, we submit to explore how behavior may change as a function of the environmental cues: that is, without changing the consequences of smartphone use, which leads to another issue. In fact, our secondary aim is to provide an analysis and discussion of the ubiquitous role of social reinforcement in this and other nudging studies that otherwise tend to focus uniquely on the role of the antecedents of behavior in the behavioral contingency (see Skinner, Citation1953).
The research question underlying this experimental work was further divided into three research hypotheses. They are herein sequentially addressed from data collection to presentation of results: (i) is there a significant difference in the estimated percentage of time during which none of the users seated at the same table spent interacting with their smartphones due to the experimental manipulation? Outcome 1 represents a measure of this question. Conversely, we asked whether (ii) there is a significant difference during which all the customers seated at the same table spent interacting with their smartphones? The first two outcomes represent a measure of these hypotheses (i.e., (1) no-smartphone ratio and (2) all-smartphone ratio, respectively). Furthermore, we asked whether (iii) there is a significant difference in the longest consecutive estimated period during which none of the customers interacted with their smartphones. This was an additional measure to the first research hypothesis and related specifically to the continuity of the experimental manipulation. The outcome associated with this hypothesis was (3) longest no-smartphone period.
The following sections of this study are organized as follows. First, we present the state of the art of the conceptual frameworks that guided the present work and underpins the nudging approach. This section goes beyond introducing the classical contributions of cognitive processes underlying decision-making and the modification of the environment in which choices are made in at least two ways. First, it puts forward an analysis of the behavior of smartphone use as a function of its reinforcing social consequences, which may not be avoided. Second, it expands our understanding of nudging by considering it as an antecedent term in the contingency (e.g., similar to a setting or motivating stimulus).
Next, we present the materials, methods and results of the exploratory study for reducing smartphone use. The discussion section links our findings to the broader literature and takes up some ethical concerns that ought to be presented in a transparent way whenever nudging experiments are resorted to. Furthermore, we discuss possible limitations of our experiment, which should be addressed in subsequent replications. Lastly, the conclusions contain some further thoughts on the possibility and desirability of evoking forms of alternative social behavior.
2. State of the art: nudging in the behavioral contingency
2.1. Studying choice as cognitive processes
Smartphone use represents an instance of behavior: in its most simple form, it is the result of a (recurrent) choice between using and not using the smartphone. Whether it is possible to significantly influence the pervasive and dysfunctional use of smartphones by manipulating the contextual factors encompassing smartphone use and overuse behavior is an empirical question. However, nudging is a relatively novel approach that includes concepts and methods drawn from behavioral economics. Some of the contributions that led to the formulation of nudging include the study of cognitive processes involved in decision-making and choice behavior. Specifically, the heuristics and bias program (Tversky & Kahneman, Citation1974, Citation1983) is the closest precursor for the development of the nudging research program. According to this program, users and consumers deviate systematically from taking best choices that entail optimal outcomes. For example, the extent to which information is representative, available, and may serve as an anchor to eventually adjust can exert a strong and systematic influence on our perceptions and decision-making processes in the presence of uncertain events (Tversky & Kahneman, Citation1974). Some examples of heuristics include rules of thumb (Todd, Citation2001) and mental shortcuts (Kahneman, Citation2011) for processing information that is relevant to a given choice. They have an important evolutionary role for the survival of our species (e.g., fleeing from potential threatening predators), but they often lead to “good enough” and not best choice (i.e., satisficing; see Simon, Citation1956).
These concepts were later reviewed and developed in a dual process theory that contraposed two systems: the first (System 1) based on effortless intuition and the second (System 2) of deliberate reasoning (Stanovich & West, Citation2000; see also Kahneman, Citation2003). According to this dualistic view, excessive smartphone use behavior and other types of additions may be considered a form of autopilot-steered behavior, which is more under the control of a System 1-like processing system: it is automatic and effortless (Kahneman, Citation2011). Conversely, the choice of using the smartphone only when it is useful or appropriate may be considered a process mediated by System 2, which is more reflective and effortful. Thus, the overuse of smartphone is considered a form of counterproductive behavior insofar as it affects negatively people’s life and activities. For example, checking the smartphone screen several times or for a prolonged time are two examples of counterproductive behavior. Behavior is maintained by certain positive and immediate socially mediated consequences associated with smartphone use (e.g., liking a picture, posting on a public news feed, etc.). However, negative delayed consequences may occur with a degree of uncertainty: some of these may pose a risk, such as developing a behavioral addiction (Gigerenzer, 2015; Stanovich & West, Citation2000).
Counterproductive behaviors may be explained in terms of psychological predispositions or cognitive biases that can be referred to any systematic error in decision-making and choice behavior (e.g., Angner & Loewenstein, 2007; Camerer, 1999; Kahneman, Slovic, & Tversky, 1982). The tension between the choice of giving in to the impulse and the choice of resisting the temptation to act on the smartphone is liable to bias depending on the arrangement of the behavioral contingencies underlying that choice. In other words, it is the relation between context and behavior that maintains the choice to either use or refrain from using our smartphones. Thus, counterproductive behaviors may be studied by taking a distance from any underlying cognitive system (e.g., dual-process theory or processes of judgement under uncertainty) and focusing instead on their behavioral principles (e.g., Hursh, 1980; Skinner, Citation1953).
2.2. Concepts and questions of nudging as a technology
Nudging refers to an empirical approach for changing behavior as a function of its contextual features, rather than by changing the economic incentives of choosing one course of action or another (Thaler & Sunstein, Citation2008; see also Sunstein, Citation2014; Thaler, Citation2018). Thaler and Sunstein (Citation2008) defined a nudge as “any aspect of the choice architecture that alters people’s behavior in a predictable way without forbidding any options or significantly changing their economic incentives” (p. 6).
This approach centered on the environment sustaining choice behavior is shared with a behavior analytic approach to choice. Behavior analysis is a natural science concerned with the functional relations of some classes of variables. The understanding that users may overuse their smartphones has a limited pragmatic value per se. Conversely, the focus is herein on the understanding of the context in which a certain behavior occurs (Moderato & Copelli, 2010). A behavioral contingency depicts an “if … then” temporal relationship between a target behavior and its antecedents and consequences (Mechner, 2008; see also Houmanfar & Rodrigues, 2006). This relation was first formalized by Skinner (Citation1953) and, in its most simple formulation, is comprised of three terms: an antecedent term, a behavior, and its consequence(s). Thus, behavior represents a function and is maintained by its effects on the encompassing environment. According to a recent review study, the structure of behavior matters in the analysis of behavior (e.g., Sandaker et al., Citation2019). Behavioral topography refers to what a behavior looks like and has an operational value in applied settings. In case of smartphone use, there are different forms of interacting with one’s smartphone: pushing buttons, holding it to the ear, swiping, reading, dictating and so on. However, what classifies it as interaction is the function of that behavior.
The function of a behavior refers to the process that derives from the interaction between an individual and the environment, which are united in a reciprocal relation (Moderato & Copelli, 2010). For example, the overuse of smartphones or the risk of developing an addiction to smartphone use may be maintained by access to social attention, public recognition, and by social contexts in which other users also overuse their smartphones. In turn, this may lead to a negative cycle of dysfunctional practices. Thus, there are two (but possibly more) concurrently available schedules of reinforcement that sustain the overuse of smartphones. One of them programs for direct and positive social consequences, which is what maintains behavior in the short term. The other schedule, which is often overshadowed by the former, programs for long-term negative effects that are possibly both social (e.g., deteriorating relationships) and carry health implications for the agent, such as stress and fatigue. More generally, smartphone overuse may also be viewed as an issue people have with balancing short-term versus long-term consequences at the point of choice. As in the case of other forms of addiction, it is more difficult and demanding to steer choice behavior after the first negative consequences appear, when the agent has already lost control over his or her behavior (Anonymous, personal communication, 8 September 2020).
The introduction of a stimulus that may help the agent discriminate between choices is aimed at signaling to the agent that there are different consequences available. These depend on the choice taken in the present, but their effects may manifest themselves at different points in the future. This duality represents the starting point for expanding on a three- to four-term contingency analysis of smartphone use. Whereas the three-term contingency model is made of a behavior preceded by an antecedent and followed by a consequence (see Skinner, Citation1953), the four-term model adds the term motivating operation before the three-term contingency (Michael, Citation1982). The motivating operation acts on a person’s motivation to attain certain consequences and is able to affect behavioral contingencies in a (more) powerful way (Langthorne & McGill, Citation2009).
2.3. Defining nudging as a behavior analytic technology
A stimulus is any physical, organic or social event that can be studied directly or through a specific tool. A stimulus may have an eliciting or a discriminative function: in the former case, it automatically produces a certain response. In the latter case, it sets the occasion for behavior to occur and is referred to as a discriminative stimulus (SD) (see Catania, Citation2013). For example, the notification produced by the smartphone that a new message was received may serve as an SD insofar as it signals that reinforcement is available upon reaching out to it and operating its screen (e.g., the pleasure of hearing from a distant friend or learning that a delivery of a parcel was notified). Conversely, elicited responses are involuntary reactions that happen automatically whenever a stimulus is presented (Moderato & Copelli, 2010), such as an increase in heart rate upon the notification of an incoming call from an unknown foreign number (e.g., it may be an unforeseen opportunity of professional collaboration or an attempt to extort personal information).
In the four-term contingency model, a motivating operation (MO) intends any environmental variable that alters the reinforcing effectiveness of a stimulus, object, or event, and alters the frequency of the behavior that has been reinforced (Michael, Citation1988; see also, Citation1982, Citation1993). Thus, the concepts of SD and MO share common features, but remain distinct. The main difference between them is that an SD evokes behavior due to past correlations with an increased availability of reinforcement, whereas an MO can increase behavior when reinforcement is not available (i.e., even without the notifications of a new incoming message). When analyzing the effects of stimulus manipulation and control in nudging research, SD and MO may be considered in similar functional terms: they both comprise antecedent elements in the three- or four-term contingency.
After introducing terms in the previous paragraph, it can now be maintained that a nudge comprises an SD or an MO depending on whether reinforcement is available following smartphone use or any other target behavior. The techniques used in nudging interventions are typically focused on the manipulation of antecedents. Although they usually have a more reinforcing value compared to consequences that lead to future better outcomes (Reed et al., Citation2013), nudges do not directly target immediate consequences of behavior. Nevertheless, Rachlin (Citation2015) suggested that a nudge may help bring behavior from the control of immediate and concrete gratification to delayed and abstract reinforcers. Thus, a nudge can discontinue the smoker’s behavior by emphasizing the possible negative consequences of lung cancer over the instant gratification of a smoke. Similarly, it can discontinue the smartphone addict’s behavior by emphasizing the possible negative consequences of oxidative stress over the instant gratification of a social media notification.
Similar to an SD or an MO, the nudge deployed in this study comprises a manipulation of the antecedents of the behavior of interacting with one’s smartphone. The aim of the nudge is to help users to come in contact with possible positive natural consequences of their behavior, by arranging their choice environment accordingly. Furthermore, this arrangement is able to affect the likelihood of future occurrences of similar behavior, regardless of their topography (e.g., decreasing the use of smartphones in contexts in which social reinforcement is available from other sources, such as at a party). Notwithstanding, it needs be noted that the nudging intervention described in the present study does not program for any direct manipulation of behavioral consequences and we maintain that neither do other nudging interventions.
3. Methods
3.1. Setting and study design
The experiment was conducted in a pub located in the city center of Milan, Italy. The pub was located in a notoriously attractive and crowded area next to the channels, which is one of the most popular aperitif destinations in the city and has a high frequency of visitors. The size of the establishment was similar to other pubs in the surroundings and we estimate that it could seat around 60 customers during ordinary opening hours. Most of the income of the pub was drawn from the sale of drinks, which included access to a food buffet in the evening. Thus, the pub represented an elective social context in which families, friends, colleagues, and acquaintances were able to enjoy food and beverages during their leisure time. In fact, the experiment took place during the evening hours, from 19:00 to 23:00. Moreover, the choice of implementing the intervention in a pub was due to the experimental setting: it retained a high ecological validity given by the simultaneous availability of reinforcement whether provided by other people or mediated by the smartphones.
The setting and design were arranged to reduce as much as possible any direct influence of the observers on the customers of the pub in a naturalistic setting. No personal or sensitive data were collected, including age and gender, although it was observed that the pub was mostly frequented by younger adults and both genders were represented in a balanced way. This decision was supported by the guarantee of fully protecting the privacy of the customers and compliance to the ethical guidelines of the research organization that supported this study. Furthermore, the time intervals for the observations would not have allowed to collect with enough accuracy this information in addition to the behavior of smartphone use. The time sampling procedure is described in the next section on the design of the study.
The study consisted of a pre/post simulated design with 2 independent conditions, and the same tables were observed during both the control and the experimental conditions. The independent variable was the introduction of nudge to influence, without forcing, an alternative choice behavior. We presented a smartphone basket (whose characteristics and function are described in the next section) in the experimental condition, whereas no manipulation was made in the control condition.
3.1.1. Target behaviors: definition and recording procedures
The dependent variable was the use of smartphones, measured as the number of people who interacted with their smartphone divided by the number of people seated at a table and expressed in percentage. For example, if four customers were seated at a table and two of them interacted with their smartphone, the percentage was 50%. Next, these data were converted into statistical units (SUs) following the criteria described in the data and statistical analysis section. SUs are arbitrarily identified statistical measures comprising groups: they varied as the composition of the groups changed (i.e., how many people were seated at the same table), and they were able to convey smartphone use beyond the level of single user–smartphone interactions, which comprised target behaviors.
Because of the nature of these behaviors that are characterized by a high frequency, we chose to perform their observation and recording according to a sampling procedure, based on time. Time sampling refers to a variety of methods for observing and recording behavior during intervals or at specific moments in time (Cooper et al., Citation2007). A momentary time sampling (MTS) was used as a proxy of the period of time in which customers were using or not their smartphones. According to an MTS procedure, each of the two deployed observers recorded whether a behavior occurred within the time interval established during the design of the intervention. We chose relatively short time intervals (5 seconds) in order to counterbalance some of the limits of the MTS. In fact, although MTS may not be the best method to observe short behaviors, adopting short monitoring time intervals and increasing the total number of observation periods may partially correct for these limitations.
Nevertheless, the choice of resorting to an MTS procedure was four-fold. First, target behaviors are usually prolonged in time (e.g., calls, social media messaging, internet browsing, games, etc.), although one-time behaviors (e.g., tap the smartphone screen to check notifications; check the time on the screen, etc.) were also included during the observation. The MTS procedure is more reliable whenever measuring behaviors that are extended over time and variable insofar as their topography is concerned (i.e., retaining their function). For example, interacting with one’s smartphone normally occurs over time and in different ways, which include reading, texting, browsing; however, these behaviors share a common function. Second, MTS is suitable for measuring the behavior of the individuals in a group. Specifically, the groups consisted of the customers seated at each table and included in the observation during both the experimental and control conditions. Third, MTS does not require continuous observation (LeBlanc et al., Citation2020). Being a sampling procedure, it is particularly effective at estimating the occurrence of a behavior and its sensitivity to change over longer time spans (Fiske & Delmolino, Citation2012). Fourth, MTS is less intrusive compared with other time sampling methods. Throughout the duration of the experiment, the two observers were seated in the pub as disguised customers. There were low chances of them being noticed, for they were sitting at one of the tables that were least visible from the other customers, and they ordered food and drinks like all other customers in the pub. In sum, it is unlikely that their presence influenced smartphone use behavior among the customers in both conditions.
3.2. Materials
The following materials were used: an observation grid, an audio file, and a smartphone basket. The first two allowed to record the extent to which the customers interacted with their smartphone; the third (smartphone basket) represented the independent variable of the experiment.
Observation grid. We developed an observation grid to record the extent of user–smartphone interaction; it is displayed in . The grid was identical for both control and experimental conditions. It included 30 rows for each observation and 6 columns for each observed table. The 6 columns were split in two: the left part was reserved for recording the number of customers seated at each table and the right part for recording how many of them were interacting with their smartphones. The operational criteria for including and excluding target behaviors of smartphone interaction, although topographically different, are listed in : target behaviors in the left column and non-target behaviors in the right column.
Figure 1. Observation grid
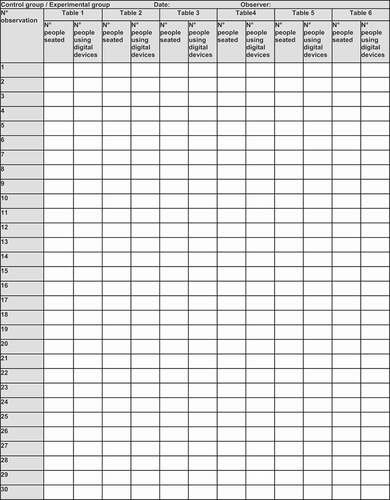
Table 1. List of target and non-target behaviors for recording smartphone use
Audio file. An audio file containing the MTS was recorded to help both independent observers keep track of time and pace for registering the data in the observation grid. The recording stated the table number at the start of the observation period for each of the six tables included in the observations. Thus, when the observers heard the number one, they were prompted to observe the first table included in the observation. When hearing two, they observed the second table, and so on. The count continued this way until number six was reached, which prompted the observation of the sixth table and, thereafter, a pause of five seconds was included. This comprised a completed string of observation, which was repeated in the audio file for as many times as there were rows in the observation grid (i.e., 30).
Smartphone basket. We prepared six identical wooden baskets and placed them in the middle of each of the six tables included in the observations in the experimental condition. displays a lateral view and a view from above of one of the baskets. They featured the picture of a smartphone and a slogan that prompted the customers to place their smartphones into the basket as a proof of social commitment. The translation of the slogan from Italian to English is: “Are you really social? #LayItDown”. The baskets could be reached effortlessly and were divided into six compartments that could conveniently store up to 6 smartphones because each table could seat up to six customers.
3.3. Procedure and analyses
The observations were conducted once a week, on the same day, during two consecutive weeks. The first observation comprised the control condition, while the second observation comprised the experimental condition. Two independent observers sequentially recorded the use of smartphones by the customers seated at each of the six observed tables. For each condition, the observers were assigned five observation grids. For each row, they were instructed to record the number of customers seated at each table and how many of them were interacting with their smartphones in the appropriate columns until an observation grid was complete. depicts an example of how the completion of an observation string (i.e., a row) would have looked like, based on the number of people and smartphone use. Furthermore, it shows the progress based on the audio file and whether there was agreement between the two observers.
Observations were divided into time sample intervals of 5 seconds each. The audio file containing the time sample intervals was played by the observers through earphones. This helped them progressing from the observation of one table to the next in synchrony and maintaining the predetermined sequence. Observation periods (i.e., the contiguous observation of each of the 6 tables) lasted 30 seconds, with a pause of 5 seconds between the end of one period and the beginning of the following one. Thus, each table was observed with a time latency of 35 seconds. The total duration of the audio file was 17 minutes and 30 seconds, and this was the time needed to complete the data collection on one observation grid. The criteria specifying which tables to observe included: (a) an unobstructed view of the observed table from where the observer was seated and (b) the occupancy of at least 3 tables, with at least 2 customers at each observed table. Whenever these criteria were not met, the observers were instructed to leave an empty cell in the observation grid, indicating a missing data point.
3.4. Data and statistical analyses
Raw data, represented by each row in the observational grid (see ), were collapsed into SUs. These were comprised of the data recorded from consecutive observations of the same group of customers seated at one table, in terms of size and composition. Thus, any change in the number of people seated at one table or in their composition caused a change in the SUs. For example, if during three consecutive observations the observers recorded that four people were seated at “table one”, and during the following observation, they recorded that only two people were seated at the same table, it follows that the SU also changed. Moreover, when the number of customers seated at a table was not recorded for more than three consecutive observations (i.e., the table was empty), the SU also changed.
A threshold was established according to the following criteria: (a) observations comprising SUs with 3 or less consecutive observations and (b) observations comprising SUs composed of only 1 customer were excluded from the data analysis. Because there is a lack of literature on the use of MTS to record smartphone use behavior in naturalistic settings, we agreed on these criteria as the optimal way to have the chance to observe the frequency of smartphone use as truthfully as possible in the setting of a pub. In fact, smaller SUs may have led to underestimate smartphone use frequencies and larger SUs may have led to overestimate them. Furthermore, this decision was supported by preliminary phases of testing with the experimental setup and procedure that preceded the collection of data.
In order to account for the reliability of the collected data, we calculated the inter-observer agreement (IOA), expressed in percentage. This is a measure of the degree to which two or more independent observers report the same observation after measuring the same event (Cooper et al., Citation2007). The main benefits of relying on this measure of accuracy and reliability include an increased confidence that target behavior are recorded and that any change of behavior between conditions are due to the independent variable rather than to data collection. In both these cases, the IOA is expected to be high (Cooper et al., Citation2007).
Categorical data are presented as n (%) (e.g., use of smartphones within SUs); continuous data as median (e.g., the estimated percentage of time) and interquartile range because the data present a non-normal distribution. A 95% confidence interval (CI) indicates uncertainty around the estimates. In order to analyze whether the likelihood that the customers did not use their smartphones was different between control and experimental conditions, we applied Chi-square tests using Fisher’s exact test (when the number of cases within a cell was less than 5 unit). We used a Mann–Whitney test to analyze if the estimated percentage of time was different in the two conditions. The alpha level was set at 0.05. Analyses were conducted using Statistical Package for Social Science (SPSS), version 22.
4. Results
First, we present the results of the IOA. Both in the control and experimental conditions, the IOA of the number of the registered customers seated at each of the 6 observed tables ranged between 96% and 100%. In the control condition, the IOA of the frequency of smartphone use ranged between 86% and 97%. In the experimental condition, this measure ranged between 93% and 100%. Overall, the IOA was excellent and indicated almost perfect agreement between the two independent observers.
Next, we present the results of the composition of the SUs. In total, 74 SUs were observed: 47 (63.5%) of them belonged to the control condition and 27 (36.5%) to the experimental condition. In the control condition, 29 SUs were composed of 2 customers (61.7%), 9 SUs of 3 customers (19.1%), and 9 SUs of 4 customers (19.1%). In the experimental condition, 15 SUs were composed of 2 customers (55.6%), 3 SUs of 3 customers (11.1%), and 9 SUs of 4 customers (33.3%). There was no difference between the two groups in this distribution (Chi-square (2) = 2.21; p = .331). The median time spent by the customers seated at the tables included in each SU was similar in the two conditions (U = 626.50; p = .928). It was calculated in 5 minutes and 15 seconds (interquartile range = 7 minutes and 35 seconds) in the control condition, and 6 minutes and 25 seconds (interquartile range = 8 minutes and 45 seconds) in the experimental condition.
The presentation of results follows the three outcomes for operationalizing and measuring smartphone use among the customers. The first outcome indicates that no customers were observed while interacting with their smartphones. It refers to the estimated percentage of time with no smartphone use per SU. The second outcome indicates that all customers were observed while interacting with their smartphones. It refers to the estimated percentage of time with smartphone use by all customers per SU. The third outcome indicates the longest time without smartphone interaction. It refers to the maximum consecutive estimated period within an SU during which none of the customers interacted with their smartphones. The remainder of this section reports the results of each outcome, according to the same order in which they were presented.
Outcome 1: no-smartphone ratio. The estimated percentage of time with no smartphone use among any of the customers included in a SU was significantly higher (U = 454.5; p = .038) in the experimental condition (median = 98.5%; interquartile range = 25%) than in the control condition (median = 75%; interquartile range = 50%). Specifically, 22 SUs (46.8%) in the control condition and 19 SUs (70.4%) in the experimental condition did not feature any smartphone use during 80% or more of the observations. These results are illustrated in the left hand-side of . The likelihood that the customers did not use their smartphones was significantly higher in the experimental condition, when the nudge was present, than in the control condition (Chi-square (1) = 3.85; p = .05: OR = 2.70; 95% CI = 1.04–7.32).
Figure 4. Results of outcome 1 (no-smartphone ratio) and outcome 2 (all smartphone ratio)
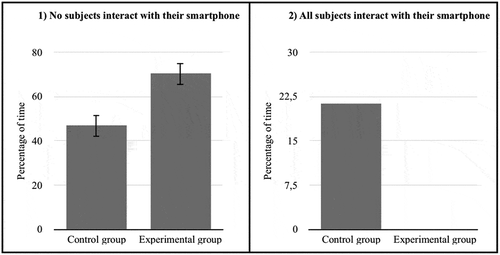
Outcome 2: all smartphone ratio. The estimated percentage of time during which all the customers included in a specific SU interacted with their smartphones was significantly higher (Chi-square (1) = 6.64; p = .010: OR = 19.36 CI = 1.0.9–341.65) in the control condition (21.3% (N = 10)) than in the experimental condition (0%—right hand-side of ) in which the smartphone basket was on the tables.
Outcome 3: longest no-smartphone period. The maximum consecutive estimated percentage of time within a SU in which none of the customers interacted with their smartphones was similar in the two conditions (U = 502.00; p = .393). No significant difference was recorded, as displayed in . The longest no-smartphone period amounted to 3 minutes and 30 seconds (interquartile range = 6 minutes and 31 seconds) in the control condition, and 4 minutes and 39 seconds (interquartile range = 8 minutes and 59 seconds) in the experimental condition.
5. Discussion
Based on the data of this study, it seems that the nudge was effective at reducing smartphone use. However, these results need to be interpreted with caution because of the wide confidence intervals. The introduction of a smartphone basket on the table “invited” potential smartphone users to seek alternative topographies of social behavior as a function of their consequences. The customers of the pub were nudging them to be more social with one another rather than through their smartphones. Technically put, we aimed at replacing the control exerted by smartphone-mediated social reinforcement with the social reinforcement available from other people surrounding the user. In practice, this meant introducing a commitment device (see Rachlin, Citation2015) (i.e., the nudge-basket) that would emphasize the availability of social reinforcement: introducing positive consequences that are able to maintain and increase social behavior (see Skinner, Citation1953). In a way, the customers in our experiment may have agreed that it is what they wanted before entering the pub, although we did not ask them (we would have compromised the tenets of this study, if we did).
Supposedly, we may assume that the customers were drawn to the pub for enjoying each other’s company before using their smartphones. Thus, the customers may have agreed to committing themselves to a better course of action, similarly to how Rachlin and Green (Citation1972) “nudged” rats to commit to better choices, years before the term nudging was first presented. They introduced an earlier point of choice in their experiment that would grant the rats longer access to food than they would have without this commitment device. However, they had to wait longer for experiencing the effects of the larger consequence, whereas on most trials the preference was for smaller-sooner consequences. This is a basic principle that suits human preferences, too (e.g., Green & Myerson, Citation2004; Rachlin & Jones, Citation2008).
One of the reasons that may justify resorting to nudges for improving choices is the alignment of the choosers’ preferences that preceded the intervention with their attainment thanks to the nudge, which works for the better of the choosers as “judged by themselves” (Sunstein, Citation2018). Put differently, Reijula and Hertwig (Citation2020) introduced the term self-nudging as a collection of “empowering interventions that enable people to design and structure their own decision environments” (p. 1). The authors distilled these two important features of nudging research in a more economic term that intends both preserving self-control over the attainment of possible consequences in the future and the autonomy to choose in the present. The results based on Outcome 1 and 2 seem to support the case for designing choice environments free form punishment for using smartphones or engaging in other potentially counterproductive behaviors. Furthermore, this approach would also remove more severe health and socioeconomic costs associated with treatment, whenever counterproductive behavior develops into self-harm behavior (e.g., rehabilitation of addiction behavior). Specifically, the youngest users represent a sensible target of better and more sustainable alternatives for seeking social reinforcement while preventing smartphone overuse and its related health and social risks.
However, some form of control exerted on behavior by the encompassing environment is unavoidable, suggesting that freedom is simply unachievable (Skinner, Citation1971). In other words, the context needs be arranged in one way or another and this is likely to influence our choices and decision-making processes. Thus, it is impossible to avoid the influence of certain environmental features on our behavior and how they shaped our learning histories. For example, increased smartphone use in a given period such as in the pub could be due to deprivation from smartphone use in the preceding hours (e.g., while at work or school), which works as a MO to increase the attractiveness of the (social) reinforcement deriving from it (see also Laraway et al., Citation2003). Furthermore, this could be one instance of several user–smartphone interactions that have been repeated over time and in similar conditions throughout the lifetime of the customer (i.e., a learning history). However, the nudging intervention in this study allowed the customers to experience the natural consequences of their behavior, rather than imposing them. Possibly, this may produce long-lasting behavior change, enduring after the nudge is withdrawn.
There was an increase in time in the experimental condition during which social interactions were smartphone-free (outcome 1). Furthermore, a complementary measure of all-smartphone ratio decreased to zero from the control to the experimental condition, which suggests that it is possible to replace smartphone-mediated social reinforcement with social reinforcement occurring from physical proximity. As the customers could attain both depending on their preferred source, this calls for an increased attention to the ecological validity of the current experimental setup. Although nudging is a concept and a technology derived from theories of bounded rationality (see Simon, Citation1983), it addresses behavior and not necessarily its underpinning processes. Nudging was suggested as a possible correction from systematic errors in cognitive processes underlying choice behavior. However, we developed further the term in relation to its environmental consequences: from a behavior analytic standpoint, nudges affect behavioral contingencies, for they add or amend the antecedent terms (i.e., SD or MO). In social settings, they signal changes in the availability of (social) reinforcement.
This study is just one small but effective step towards understating these mechanisms and designing choice environments that preserve wellbeing, more than they may harm it, such as in the case of technostress among smartphone over-users. To our knowledge, it is the first of its kind in Italy, for it describes a digital detoxing intervention in a recreational social setting based on the principles of nudging. Within the Italian context, it builds on some of the conceptual work of Gui et al. (Citation2017) that addressed the raising threat of deteriorating social relationships options and digital information overflow. The authors developed the definition of digital wellbeing by including the interpersonal level represented by the community to individual smartphone–user interactions. In yet another study, a representative Italian sample was interviewed and reported the discounting of physical relationship in terms of wellbeing and satisfaction whenever smartphones were concurrently available (Rotondi et al., Citation2017). As the authors highlighted that time spent with friends was worth less among smartphone users, our study explores how this perception can be changed by changing the behavioral contingencies of smartphone use.
Nudging rests on the philosophical underpinning termed “libertarian paternalism”, which captures the dual aspect of both free and forced choices and the ethical implications that need be considered when extending its applications to policymaking. Nudging is paternalistic because the environment of choice is deliberately designed to guide users’ choice is a predetermined direction. This direction is usually for the wellbeing of the users and should not clash with their values (e.g., in the case of compulsive smartphone use, there should be agreement on the aim on the desirability of reducing it). Nevertheless, nudging is also libertarian, which defines the modalities in which this objective wants to be pursued. In sum, libertarian paternalism is an attempt of reconciling the two and sets the grounds for solving complex societal problems, one bit of them at a time (Rebonato, Citation2012). For an intervention to be regarded as a nudge, it must not preclude people’s freedom of choice between alternative options. Notwithstanding, it guides people’s choices in the direction proposed by the choice architect (i.e., the designer of a nudging intervention), retaining the possibility to avoid the “gentle push” without burdens or difficulties (Hansen, Citation2017; Thaler & Sunstein, Citation2008). Choice architecture refers to the natural or artificial arrangement of the physical and social contingencies of reinforcement (e.g., Simon & Tagliabue, Citation2018). It represents the environmental context for choice behavior to occur. Hence, choice architecture may not be avoided, inasmuch is must be arranged one way or another, and this is likely to influence choice accordingly (Sunstein, Citation2015). Furthermore, the reader familiar with the concept of affordances of Gibson (Citation1966) may be brought to find similarities with the architecture of choice, and a comparison between these perspectives might be extremely promising. However, although Gibson’s theory of affordances is partially consistent with the concept of choice architecture, the latter does not deal with perception. Conversely, the purpose of choice architecture concerns the pragmatic presentation of choices and their effects on modelling the agents’ behavior. Because the comparison of both theoretical underpinnings reaches beyond the scope of this study, the discussion of these commonalities deserves more ample space in a subsequent conceptual work.
As previously noted, another feature of nudging smartphone reduction was that the customers’ choices were not forced in any way, for the only experimental manipulation concerned the environment or context. In fact, nudges do not entail the imposition of (economic) incentives (or penalties) on alternative choice behavior; on the contrary, they are easy cheap to avoid (Thaler & Sunstein, Citation2008). Nevertheless, it does not seem clear where the cutoff lies, nor how this may be operationalized (e.g., Marchiori et al., Citation2015). We recommend that nudging research be less arbitrary concerning its definition and empirical scope, and that there is more consistency between theory and its applications (Tagliabue et al., Citation2019). Some nudging experiments have notably manipulated the consequences of behavior, providing incentives; they reached beyond the mere manipulation of contextual variables preceding the behavior. For example, Allcott (Citation2011) provided a smiley face whenever electricity users were more energy-efficient than their neighbors were. Hence, the smiley face had reinforcing value towards a desirable behavior and contributed to the success of creating an alternative behavior. However, it may be debated whether this intervention was consistent with the definition of a nudge (see also Hansen, Citation2017). It is widely accepted that positive reinforcement is preferable and preferred than resorting to punishment and penalties (Skinner, Citation1938, Citation1953; see also Crow, Citation2017; Daniels & Daniels, Citation2004). Thus, direct manipulation of consequences should be aimed at positively reinforcing target choice behavior.
Another possible source of alternative behavior may have been represented by the slogan on the side of the smartphone basket. In this case, whether the smartphone was placed inside or outside the basket would not have mattered much to the potential user, for the normative message may have sufficed to steer the choice of not using it. The slogan displayed on the smartphone basket, prompted the customers of the pub to abstain from using their smartphones for the period of time in which they were seated at their table and be social in reality, rather than on the media. Indeed, the textual prompt “Are you really social? #LayItDown” was specifically designed to make more salient for the customers the chance to spend their time at the pub interacting with other people instead of using their digital devices. It is possible that this verbal cue may have been perceived as a construed paternalistic approach, although in its mildest form.
The results of the third outcome of this study (longest no-smartphone period) showed that although there was a difference between control and experimental groups, this was not significant. This may have broader implications that are shared with other nudging interventions and concern the sustainability of target behaviors over time. The manipulation of contingencies is seen as neither negative nor positive. The redesign of the environment ought to be as free as possible from aversive stimuli (Skinner, Citation1971). The idea that behavior is shaped by the context in which it occurs represents a guiding principle of nudge theory. Functional analyses and explanations of choice behavior may not succeed whether executed in isolation from its contextual variables, and counterproductive (or irrational) behaviors may be predicted and prevented. For example, dual-process theories wherein System 1 and System 2 are embedded (Kahneman, Citation2011; Tversky & Kahneman, Citation1974) may enhance the understanding of antecedent contingencies of behavior, which may otherwise not be as appealing to a broader audience if termed SD and MO. However, they bear the risk of adhering to these labels to such an extent that they may assume a function of a causal explanation underlying choice. To this extent, behavior analysis may be a more parsimonious approach of the study of human behavior, allowing the operationalization of concepts and processes.
Lastly, because of the low cost of the intervention and the flexibility of the experimental design, the observations could be extended to different geographical and cultural contexts in order to test the generalizability of our findings. In fact, the use and abuse in some cases of smartphone seem to be a worldwide phenomenon and problem. This is not confined within the borders of a specific country or region although it may be argued that smartphone use may have different consequences depending on the cultural framework in which it occurs; and so may the conditions for technostress to occur and the extent to which societies are designed to foster it, such as between developed and least developed countries.
5.1. Limitations
Despite the statistical significance of two of the three outcomes, this study is characterized by some limitations, which are discussed in the remainder of this section. First, the experiment featured a relatively small sample size of observed SUs. We recommend that forthcoming studies that make use of the same procedure herein described increase the number of customers in both conditions. For example, this may be achieved by modifying the layout of the pub in a way that more tables are concurrently in sight of the observers, employing more observers, and extending the observation periods. Furthermore, it may be possible to resort to video recording in countries and settings in which the law and scientific ethical committees permit setting up cameras inside the premises of commercial activities open to the public. In our experiment, it was not permitted to perform video recordings in the pub for the duration of this study due to privacy laws. Direct observations represented a less precise data collection method than recording on camera. This limitation was partially addressed by having two independent observers and measuring their agreement. However, it was not possible to record all possible interactions of the customers with their smartphones, nor to detect precisely enough gender and age information.
Recently developed MTS technologies include less error-prone procedures when engaging in data collection, which may be resorted to in subsequent studies. For example, interval recording applications (such as, e.g., Intervals ABAFootnote1 and Behavior Observation Made EasyFootnote2) can assure more rigorous control, homogenize data, and control for human errors to a more confident degree than traditional agent-based technology. Although we do not suggest to necessarily increase smartphone use to record behavioral data on apps while warning against the risks of smartphone overuse, we maintained to raise awareness on some of the possible risks of an inappropriate use of smartphones. Furthermore, these risks can be extended to other handheld technological devices affecting our lifestyles (e.g., tables, notebooks, smart watches, etc.), whose analysis was not included in this study but shares similarities insofar as they may provide further alternative sources of mediated social reinforcement. Notwithstanding, it needs be stated that the aim of this study and its authors is not to express disapproval of a legitimate use of smartphones.
Third, the MTS may have led to slightly overestimate (but also underestimate) the duration of the behaviors compared with other recording procedures (Cooper et al., Citation2007, p.113; see also Ary & Suen, Citation1983). However, the target behaviors of this study included smartphone interactions that are usually prolonged, such as calls or internet browsing. Moreover, we decided to use short time intervals in order to observe the behaviors of the customers with a higher frequency. In turn, this increased our chances of completing more observations in a given period of time. In sum, we maintain that this strategy increased our chances of detecting the target behaviors.
Fourth, for the primary measures of this study concerned user–smartphone interactions, we neglected user–user interactions (i.e., customers’ interpersonal, verbal and non-verbal, exchanges). Thus, an interesting and informative measure of increased precision might address whether there is any inverse correlation between user-smartphone and user–user interactions. Given the experimental setup, it was not possible to discriminate between possible sources of choice behavior control. The interaction of simultaneous conditions may have been necessary to achieve significance of our findings, but only a stricter empirical procedure may provide enough clarity. Running a component analysis may establish whether the effectiveness of the intervention (i.e., the controlling variable) was due to the smartphone basket alone, the social message printed on the side of the basket, or their combined effect. Previous nudging studies have pointed to how social norms suffice to prompt behavior modification (e.g., Aldrovandi et al., Citation2015; Allcott, Citation2011; Kallbekken & Sælen, Citation2013). However, they are most effective whenever communicated in a timely fashion and perceived as salient (Behavioural Insights Team, Citation2011).
6. Conclusions
This study emphasized how a nudging intervention may comprise a concept and technology to prevent and overcome disruptive social behavior. The overuse of smartphones becomes a counterproductive behavior insofar as it contingently related not only to contiguous negative social consequences but also delayed and possible health hazards (e.g., oxidative stress and technostress).
Similar to other examples of counterproductive behavior that may turn into behavioral addictions (e.g., substance abuse, gambling, workaholism, etc.), choice behavior represents a tension between the availability of consequences that are immediate and certain versus delayed and abstract (Rachlin, Citation2015). For example, smartphone use provides virtually endless sources of social reinforcement contingent on liking, texting, and other forms of social media presence. However, oxidative and technostress are only an abstract possibility that may or may not manifest itself in the not-too-near future. We advanced the claim of defining nudging in behavioral analytic terms, rather than according to their possibly underlying dichotomous cognitive processes, which are represented by System 1 and System 2 (Kahneman, Citation2011). Although the latter is more effortful and can consider the long term and potentially negative consequences of smartphone use, it is possible that smartphone use behavior is steered by System 1. This educative nudges are called for, in order to increase people’s own power of agency (Sunstein, Citation2016, p. 124). Behavioral antecedent terms, such as an SD or an MO, are effective for both altering and maintaining desired behavior. In several cases (e.g., warnings, social approval, norms, etc.), nudging interventions already include the exploitation of their effects.
Biologist E. O. Wilson famously declared, “The real problem of humanity is the following: We have Paleolithic emotions, medieval institutions and godlike technology.”Footnote3 While our study successfully nudged patrons to be really social, rather than digitally social, this is just a hack for the growing concern that surrounds technology companies’ impact on its consumers’ well-being. Until developers of these technologies fully consider the consequences of their products exploiting our Paleolithic psychology and impulses, we believe nudges can be implored. We hope this study will spark a dialogue for patches that can be used to address our obsolete, Paleolithic hardware.
Declaration of interest statement
The experiment described in the present paper was originally featured in and has been substantially revised from the 7th chapter of the first author's doctoral thesis, which can be found at the following link: https://apeiron.iulm.it/handle/10808/26204#.XqaqQpMzY1I. The authors declare that they have no conflict of interests.
Ethical approval
All procedures performed in studies involving human customers were in accordance with the ethical standards of the institutional and/or national research committee and with the 1964 Helsinki declaration and its later amendments or comparable ethical standards. All authors have read and agreed to the published version of the manuscript.
Acknowledgements
The authors wish to thank the team of Nudge Italia for helping with the design and implementation of the intervention.
Additional information
Funding
Notes on contributors
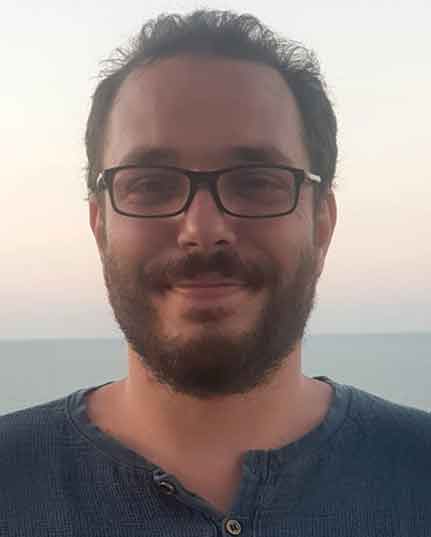
Massimo Cesareo
Massimo Cesareo is a psychologist. He holds a PhD in communications and markets: economy, marketing and creativity at IULM University. He is the coordinator of Nudge Italia, a special interest group of IESCUM - Istituto Europeo per lo Studio del Comportamento Umano, and choice architect for a Better Place.
Marco Tagliabue
Marco Tagliabue is associate professor at the faculty of Health Sciences at Oslo Metropolitan University. He is a psychologist and a member of the Cultural Selection and Behavioral Economics Lab. He funded and manages the Norwegian Nudging Network. His research interests include sustainable choice behavior and cooperation from a cultural perspective.
Annalisa Oppo
Annalisa Oppo is a psychologist, psychotherapist, and biostatstician. She is tenured lecturer in general psychology and methodology at Sigmund Freud University and researcher at IESCUM.
Paolo Moderato
Paolo Moderato is full professor in general psychology at IULM University. He is founder and president of IESCUM.
Notes
1. Christopher Mays (2019). elocinSoft (4.0.5) [Mobile application software]. https://itunes.apple.com/us/app/intervals-aba-interval-recording/id431231950?mt=8.
2. EdPsychLA. (2017). Zhanna Shekhtmeyster (1.7) [Mobile application software]. https://itunes.apple.com/us/app/behavior-observation-made-easy/id1281984900?mt=8.
3. Debate at the Harvard Museum of Natural History, Cambridge, MA, 9 September 2009.
References
- Aldrovandi, S., Brown, G. D., & Wood, A. M. (2015). Social norms and rank-based nudging: Changing willingness to pay for healthy food. Journal of Experimental Psychology. Applied, 21(3), 242–22. https://doi.org/10.1037/xap0000048
- Allcott, H. (2011). Social norms and energy conservation. Journal of Public Economics, 95(9), 1082–1095. https://doi.org/10.1016/j.jpubeco.2011.03.003
- Ary, D., & Suen, H. K. (1983). The use of momentary time sampling to assess both frequency and duration of behavior. Journal of Behavioral Assessment, 5(2), 143–150. https://doi.org/10.1007/BF01321446
- Behavioural Insights Team. (2011). Annual update 2010–11. Cabinet Office Behavioural Insights Team, London, UK. https://www.gov.uk/government/uploads/system/uploads/attachment_data/file/60537/Behaviour-Change-Insight-Team-Annual-Update_acc.pdf
- Berenguer, A., Goncalves, J., Hosio, S., Ferreira, D., Anagnostopoulos, T., & Kostakos, V. (2017). Are smartphones ubiquitous?: An in-depth survey of smartphone adoption by seniors. IEEE Consumer Electronics Magazine, 6(1), 104–110. https://doi.org/10.1109/MCE.2016.2614524
- Bianchi, A., & Phillips, J. G. (2005). Psychological predictors of problem mobile phone use. Cyber Psychology & Behavior, 8(1), 39–51. https://doi.org/10.1089/cpb.2005.8.39
- Brod, C. (1984). Technostress: The human cost of the computer revolution. Addison-Wesley.
- Catania, C. A. (2013). Learning (5th ed ed.). Sloan.
- Chesley, N. (2005). Blurring boundaries? Linking technology use, spillover, individual distress, and family satisfaction. Journal of Marriage and Family, 67(5), 1237–1248. https://doi.org/10.1111/j.1741-3737.2005.00213.x
- Clement, J. (2020, August 10). Number of monthly active Facebook users worldwide as of 2nd quarter 2020 (in millions). Statista. https://www.statista.com/statistics/264810/number-of-monthly-active-facebook-users-worldwide/#:~:text=How%20many%20users%20does%20Facebook,network%20ever%20to%20do%20so
- Cooper, J., Heron, T., & Heward, W. (2007). Applied behavior analysis (2nd ed. ed.). Pearson/Merrill-Prentice Hall.
- Crow, R. (2017). Nudge & boost for better living: A semi-autobiographical novel on using the ABCs of human behavior. CreateSpace.
- Damaševičius, R., Maskeliūnas, R., Venčkauskas, A., & Woźniak, M. (2016). Smartphone user identity verification using gait characteristics. Symmetry, 8(10), 100. https://doi.org/10.3390/sym8100100
- Daniels, A. C., & Daniels, J. E. (2004). Performance management: Changing behavior that drives organizational performance. Performance Management Publications.
- David, M. E., Roberts, J. A., & Christenson, B. (2018). Too much of a good thing: Investigating the association between actual smartphone use and individual well-being. International Journal of Human–Computer Interaction, 34(3), 265–275. https://doi.org/10.1080/10447318.2017.1349250
- Enez Darcin, A., Kose, S., Noyan, C. O., Nurmedov, S., Yılmaz, O., & Dilbaz, N. (2016). Smartphone addiction and its relationship with social anxiety and loneliness. Behaviour & Information Technology, 35(7), 520–525. https://doi.org/10.1080/0144929X.2016.1158319
- Fiske, K., & Delmolino, L. (2012). Use of discontinuous methods of data collection in behavioral intervention: Guidelines for practitioners. Behavior Analysis in Practice, 5(2), 77–81. https://doi.org/10.1007/BF03391826
- Geser, H. (2006). Is the cell phone undermining the social order?: Understanding mobile technology from a sociological perspective. Knowledge, Technology & Policy, 19(1), 8–18. https://doi.org/10.1007/s12130-006-1010-x
- Gibson, J. J. (1966). The senses considered as perceptual systems. Allen and Unwin.
- Gimpel, G., Sudzina, F., & Petrovcikova, K. (2014). Mobile ICT acceptance in late adopter countries. 2014 International Conference on Mobile Business, 3, London, United Kingdom. https://aisel.aisnet.org/icmb2014/3
- Green, L., & Myerson, J. (2004). A discounting framework for choice with delayed and probabilistic rewards. Psychological Bulletin, 130(5), 769–792. https://doi.org/10.1037/0033-2909.130.5.769
- Gui, M., Fasoli, M., & Carradore, R. (2017). “Digital well-being”. Developing a new theoretical tool for media literacy research. Italian Journal of Sociology of Education, 9(1), 155–173. https://doi.org/10.14658/pupj-ijse-2017-1-8
- Hansen, P. G. (2016). The definition of nudge and libertarian paternalism: Does the hand fit the glove? European Journal of Risk Regulation, 7(1), 155–174. https://doi.org/10.1017/s1867299x00005468
- Kahneman, D. (2003). A perspective on judgment and choice: Mapping bounded rationality. American Psychologist, 58(9), 697–720. https://doi.org/10.1037/0003-066X.58.9.697
- Kahneman, D. (2011). Thinking, fast and slow. Farrar, Straus and Giroux.
- Kallbekken, S., & Sælen, H. (2013). ‘Nudging’ hotel guests to reduce food waste as a win–win environmental measure. Economics Letters, 119(3), 325–327. https://doi.org/10.1016/j.econlet.2013.03.019
- Khan, N. A., Habib, M. A., & Jamal, S. (2020). Effects of smartphone application usage on mobility choices. Transportation Research Part A: Policy and Practice, 132, 932–947. https://doi.org/10.1016/j.tra.2019.12.024
- Kim, D., Chun, H., & Lee, H. (2014). Determining factors of college students’ smartphone adoption. Journal of the Association for Information Science and Technology, 65(3), 578–588. https://doi.org/10.1002/asi.22987
- Langthorne, P., & McGill, P. (2009). A tutorial on the concept of the motivating operation and its importance to application. Behavior Analysis in Practice, 2(2), 22–31. https://doi.org/10.1007/BF03391745
- Laraway, S., Snycerski, S., Michael, J., & Poling, A. (2003). Motivating operations and terms to describe them: Some further refinements. Journal of Applied Behavior Analysis, 36(3), 407–414. https://doi.org/10.1901/jaba.2003.36-407
- LeBlanc, L. A., Lund, C., Kooken, C., Lund, J. B., & Fisher, W. W. (2020). Procedures and accuracy of discontinuous measurement of problem behavior in common practice of applied behavior analysis. Behavior Analysis in Practice, 13(2), 411–420. https://doi.org/10.1007/s40617-019-00361-6
- Lee, Y. K., Chang, C. T., Lin, Y., & Cheng, Z. H. (2014). The dark side of smartphone usage: Psychological traits, compulsive behavior and technostress. Computers in Human Behavior, 31, 373–383. https://doi.org/10.1016/j.chb.2013.10.047
- Marchiori, D., De Ridder, D., Veltkamp, M., & Adriaanse, M. (2015). What is in a nudge: Putting the psychology back in nudges. European Health Psychologist, 17(1), 546. https://doi.org/10.1111/spc3.12297
- Marforio, C., Karapanos, N., Soriente, C., Kostiainen, K., & Capkun, S. (2014, February 22). Smartphones as practical and secure location verification tokens for payments. Network and Distributed System Security Symposium, 14, San Diego, CA (pp. 23–26). https://doi.org/10.14722/ndss.2014.23165
- Marks, I. (1990). Behavioural (non-chemical) addictions. British Journal of Addiction, 85(11), 1389–1394. https://doi.org/10.1111/j.1360-0443.1990.tb01618.x
- Michael, J. (1982). Distinguishing between discriminative and motivational functions of stimuli. Journal of the Experimental Analysis of Behavior, 37(1), 149–155. https://doi.org/10.1901/jeab.1982.37-149
- Michael, J. (1988). Establishing operations and the mand. The Analysis of Verbal Behavior, 6(1), 3–9. https://doi.org/10.1007/bf03392824
- Michael, J. (1993). Establishing operations. The Behavior Analyst, 16(2), 191–206. https://doi.org/10.1007/BF03392623
- Montag, C., Walla, P., & Koller, M. (2016). Carpe diem instead of losing your social mind: Beyond digital addiction and why we all suffer from digital overuse. Cogent Psychology, 3(1), 1157281. https://doi.org/10.1080/23311908.2016.1157281
- Motamedi, S., & Choe, P. (2015). Smartphone information displays when reading news in Persian and English languages. International Journal of Human–Computer Interaction, 31(6), 427–439. https://doi.org/10.1080/10447318.2015.1038129
- Oulasvirta, A., Rattenbury, T., Lingyi, M., & Raita, E. (2012). Habits make smartphone use more pervasive. Personal and Ubiquitous Computing, 16(1), 105–114. https://doi.org/10.1007/s00779-011-0412-2
- Patmanthara, S., Febiharsa, D., & Dwiyanto, F. A. (2019, October 3). Social media as a learning media: A comparative analysis of Youtube, WhatsApp, Facebook and Instagram utilization. International Conference on Electrical Electronics and Information Engineering, Denpasar, Bali, Indonesia (pp. 183–186). https://doi.org/10.1109/ICEEIE47180.2019.8981441
- Peeples, L. (2018, December 14). Can’t put down the phone? How smartphones are changing our brains — and lives. NBC News.The National Broadcasting Company (NBC). https://www.nbcnews.com/mach/science/surprising-ways-smartphones-affect-our-brains-our-lives-ncna947566
- Petrovčič, A., Slavec, A., & Dolničar, V. (2018). The ten shades of silver: Segmentation of older adults in the mobile phone market. International Journal of Human–Computer Interaction, 34(9), 845–860. https://doi.org/10.1080/10447318.2017.1399328
- Rachlin, H. C. (2000). The science of self-control. Harvard University Press.
- Rachlin, H. C. (2015). Choice architecture: A review of why nudge: The politics of libertarian paternalism. Journal of the Experimental Analysis of Behavior, 104(2), 198–203. https://doi.org/10.1002/jeab.163
- https://doi.org/10.1002/mde.2714
- Rachlin, H. C., & Green, L. (1972). Commitment, choice and self-control. Journal of the Experimental Analysis of Behavior, 17(1), 15–22. https://doi.org/10.1901/jeab.1972.17-15
- Rachlin, H. C., & Jones, B. A. (2008). Social discounting and delay discounting. Behavioral Decision Making, 21(1), 29–43. https://doi.org/10.1002/bdm.567
- Ragu-Nathan, T., Tarafdar, M., Ragu-Nathan, B., & Tu, Q. (2008). The consequences of technostress for end users in organizations: Conceptual development and empirical validation. Information Systems Research, 19(4), 417–433. https://doi.org/10.1287/isre.1070.0165
- Rebonato, R. (2012). Taking liberties: A critical examination of libertarian paternalism. Palgrave Macmillan.
- Reed, D. D., Niileksela, C. R., & Kaplan, B. A. (2013). Behavioral economics: A tutorial for behavior analysts in practice. Behavior Analysis in Practice, 6(1), 34–54. https://doi.org/10.1007/BF03391790
- Reijula, S., & Hertwig, R. (2020). Self-nudging and the citizen choice architect. Behavioural Public Policy, 1–31. https://doi.org/10.1017/bpp.2020.5
- Rhiu, I., & Yun, M. H. (2018). Exploring user experience of smartphones in social media: A mixed-method analysis. International Journal of Human–Computer Interaction, 34(10), 960–969. https://doi.org/10.1080/10447318.2018.1471572
- Rotondi, V., Stanca, L., & Tomasuolo, M. (2017). Connecting alone: Smartphone use, quality of social interactions and well-being. Journal Of Economic Psychology, 63, 17–26. https://doi.org/10.1016/j.joep.2017.09.001
- Sandaker, I., Couto, K. C., & de Carvalho, L. C. (2019). Selection at three levels of organization: Does structure matter? Behavior and Social Issues, 28(1), 221–228. https://doi.org/10.1007/s42822-019-00020-z
- Simon, C., & Tagliabue, M. (2018). Feeding the behavioral revolution: Contributions of behavior analysis to nudging and vice versa. Journal of Behavioral Economics for Policy, 2(1), 91–97. http://sabeconomics.org/wordpress/wp-content/uploads/JBEP-2-1-13.pdf
- Simon, H. A. (1956). Rational choice and the structure of the environment. Psychological Review, 63(2), 129–138. https://doi.org/10.1037/h0042769
- Simon, H. A. (1983). Reason in human affairs. Stanford University Press.
- Skinner, B. F. (1938). The behavior of organisms: An experimental analysis. Appleton-Century.
- Skinner, B. F. (1953). Science and human behavior. Free Press.
- Skinner, B. F. (1971). Beyond freedom and dignity. Knopf/Random House.
- Stanovich, K. E., & West, R. F. (2000). Individual differences in reasoning: Implications for the rationality debate? Behavioral and Brain Sciences, 23(5), 645–665. https://doi.org/10.1017/S0140525X00003435
- Sunstein, C. R. (2014). Nudging: A very short guide. Journal of Consumer Policy, 37(4), 583–588. https://doi.org/10.1007/s10603-014-9273-1
- Sunstein, C. R. (2015). The ethics of nudging. Yale Journal on Regulation, 32(2), 413–450. Retrieved March 13, 2019 from https://digitalcommons.law.yale.edu/yjreg/vol32/iss2/6
- Sunstein, C. R. (2016). People prefer system 2 nudges (kind of). Duke Law Journal, 66, 121–168. https://papers.ssrn.com/sol3/papers.cfm?abstract_id=2731868
- Sunstein, C. R. (2018). “Better off, as judged by themselves”: A comment on evaluating nudges. International Review of Economics, 65(1), 1–8. https://doi.org/10.1007/s12232-017-0280-9
- Tagliabue, M., Squatrito, V., & Presti, G. (2019). Models of cognition and their applications in behavioral economics: A conceptual framework for nudging derived from behavior analysis and relational frame theory. Frontiers in Psychology, 10(2418). https://doi.org/10.3389/fpsyg.2019.02418
- Takao, M., Takahashi, S., & Kitamura, M. (2009). Addictive personality and problematic mobile phone use. Cyberpsychology & Behavior, 12(5), 501–507. https://doi.org/10.1089/cpb.2009.0022
- Thaler, R. H. (2018). Nudge, not sludge. Science, 361(6401), 431. https://doi.org/10.1126/science.aau9241
- Thaler, R. H., & Sunstein, C. R. (2008). Nudge: Improving decisions about health, wealth, and happiness. Yale University Press.
- Thomée, S., Eklöf, M., Gustafsson, E., Nilsson, R., & Hagberg, M. (2007). Prevalence of perceived stress, symptoms of depression and sleep disturbances in relation to information and communication technology (ICT) use among young adults – an explorative prospective study. Computers in Human Behavior, 23(3), 1300–1321. https://doi.org/10.1016/j.chb.2004.12.007
- Thomée, S., Härenstam, A., & Hagberg, M. (2011). Mobile phone use and stress, sleep disturbances, and symptoms of depression among young adults - a prospective cohort study. BMC Public Health, 11(66). https://doi.org/10.1186/1471-2458-11-66
- Todd, P. T. (2001). Heuristics for decision and choice. International Encyclopedia of the Social & Behavioral Sciences. Elsevier. https://www.sciencedirect.com/referencework/9780080430768/international-encyclopedia-of-the-social-and-behavioral-sciences
- Tversky, A., & Kahneman, D. (1974). Judgment under uncertainty: Heuristics and biases. Science, 185(4157), 1124–1131. https://doi.org/10.1126/science.185.4157.1124
- Tversky, A., & Kahneman, D. (1983). Extensional versus intuitive reasoning: The conjunction fallacy in probability judgment. Psychological Review, 90(4), 293–315. https://doi.org/10.1037/0033-295X.90.4.293
- We Are Social. (2017). Digital in 2017: Global overview - We Are Social. We Are Social & Hootsuite. Retrieved September 5, 2017 from https://wearesocial.com/special-reports/digital-in-2017-global-overview