Abstract
Most value-based decision-making (VBDM) tasks instruct people to make value judgements about stimuli using wording relating to consumption, however in some contexts this may be inappropriate. This study aims to explore whether variations of trial wording capture a common construct of value. This is a pre-registered experimental study with a within-subject design. Fifty-nine participants completed a two-alternative forced-choice task where they chose between two food images. Participants completed three blocks of trials: one asked which they would rather consume (standard wording), one asked which image they liked more, and one asked them to recall which image they rated higher during a previous block. We fitted the EZ drift-diffusion model to the reaction time and choice data to estimate evidence accumulation (EA) processes during the different blocks. There was a highly significant main effect of trial difficulty, but this was not modified by trial wording (F = 2.00, p = .11, ηp2 = .03, BF10 = .05). We also found highly significant positive correlations between the EA rates across task blocks (rs > .44, ps < .001). The findings provide initial validation of alternative wording in VBDM tasks that can be used in contexts where it may be undesirable to ask participants to make consummatory judgements.
PUBLIC INTEREST STATEMENT
Existing tasks used to explore value-based decision-making (VBDM) often require people to make consummatory judgements, however there are particular research contexts where this may be inappropriate. This study explored how robust VBDM tasks are to minor alterations in trial wording that do not require people to think about their momentary desire to consume objects depicted in pictures. Results showed that differences in evidence accumulation (EA) rates were equivalent across variations of trial wording. This study contributes towards initial validation of alternative trial wording that can be appropriate to implement in future research where it is undesirable to ask participants to make consummatory judgements, such as research into VBDM in people who are in recovery from addiction.
1. Introduction
In everyday life, people are confronted with multiple choices which range from trivial (e.g. whether to drink tea or coffee) to important (e.g. whether to say yes or no to a marriage proposal). Value-based decision-making (VBDM) is a theoretical framework that posits, on average, a person makes decisions based upon the things that they value (Berkman et al., Citation2017; Levy & Glimcher, Citation2012; Rangel et al., Citation2008). Based on this account, the overall value for each response option is calculated through a dynamic integration of different sources of value which incorporate the anticipated positive and negative consequences. The computation of the overall value is essential: it enables a person to compare and subsequently choose the response option with the highest overall value (Berkman et al., Citation2017).
Computational models, such as the drift-diffusion model (DDM; Ratcliff & McKoon, Citation2008), are widely used because of their ability to parameterise the internal cognitive components of decision-making. The DDM takes behavioural data (response time (RT) and choice accuracy) from two-alternative forced choice (2AFC) tasks as input, and then through a principled reconciliation, recovers decision parameters including evidence accumulation (EA) rate (also known as the ‘drift rate’, the rate at which momentary evidence is accumulated), response threshold (response caution represented in the speed-accuracy trade-off that a participant maintains), and non-decision time (encoding of stimuli and response execution; Stafford et al., Citation2020). The assumption that evidence accumulates noisily until it reaches a threshold for responding underlies the DDM (Ratcliff et al., Citation2016) which has been implemented across various domains of decision-making, including value-based (Berkman et al., Citation2017; Krajbich et al., Citation2010; Polanía et al., Citation2014).
In typical VBDM tasks (e.g. Polanía et al., Citation2014) participants initially make value judgements about a set of images, and in a subsequent 2AFC task they select the image they rated higher in value as quickly as possible. This experimental procedure generates the behavioural data that is necessary for the DDM to parameterise the internal processes of decision-making because it measures RT (the speed at which participants respond) and choice accuracy (whether the participant chose in accordance with their previous value ratings). The majority of existing VBDM tasks rely on the strength of desire to consume a commodity as a reflection of value (Krajbich et al., Citation2010; Mormann et al., Citation2010; Polanía et al., Citation2014; Tusche & Hutcherson, Citation2018): participants are initially instructed to rate and subsequently make choices about food images according to how much they would like to consume the food depicted in the image at the end of the experiment. However, the exact terminology used within the trials is often not made explicitly clear.Footnote1
Conceptual work (Copeland et al., Citation2021; Field et al., Citation2020) extended VBDM to recovery from addiction and outlined a number of hypotheses that await empirical testing. More recently, the application of computational models has been advocated to improve methodological rigour in the field of addiction (Pennington et al., Citation2021). However, methodological considerations—such as trial wording—have impeded the implementation of this research. This is because asking people in recovery to make consummatory judgements about substance-related images could be unethical (e.g. triggering desire to consume a substance could jeopardise recovery) or cause discomfort for people trying to abstain. Variations of trial wording, such as ‘which do you like more?’, could be less aversive; however, no research has explored whether variations of wording in VBDM tasks do indeed capture a common construct of value.
Given the lack of a standard methodology and the ambiguity in some existing research, it is important to explore how sensitive VBDM tasks are to alterations in wording. To explore this, we investigated whether behavioural data from the same task—but with variations of wording other than ‘which would you rather consume’—reflect a coherent construct of value as captured by EA rates. Design, hypothesis, and analysis strategy were pre-registered before data collection commenced (https://aspredicted.org/2tm3s.pdf). Our hypotheses were:
There will be no significant differences in EA rates for food images when participants complete the VBDM task with the trial wording ‘which would you rather consume?’ versus ‘which do you like more?’ and ‘which did you rate higher?’. Specifically, we hypothesise A) no significant main effect of trial wording on EA rates, and B) no significant interaction between trial wording and trial difficulty.
There will be a ‘difficultyFootnote2 effect’ such that EA rates for food images will significantly decrease alongside increasing difficulty level, and this will be consistent regardless of trial wording.
To complement these pre-registered hypotheses, we also predicted that there would be significant correlations (>.7) between EA the rates across trial wording conditions.
2. Methods
Design
This was an experimental study with a within-subject design. The dependent variable was the EA rate (estimated by fitting the DDM to RT and accuracy data from the VBDM task). Independent variables were trial wording (‘consume’, ‘like’, and ‘recall’ variants, see below) and trial difficulty (easy, medium, and hard). There is not a G*Power function to conduct a power analysis for a two-way repeated measure ANOVA, but we offered study participation to 60 participants based on heuristics (Lakens, Citation2021). However, existing research was considered only as a guide, and we recruited a larger amount than is the norm in this field (see Table S1 in supplementary materials).
Participants
We recruited 60 participants through Prolific (https://www.prolific.co/) but removed data from 1 participant who failed all attention checks in line with the preregistered exclusion criteria (see supplementary materials for detail on attention checks). Our total sample therefore comprised 59 participants (33 females and 26 males) and ages ranged from 19 to 66 years old (M = 35.08, SD = 12.78). Inclusion criteria were age ≥18 years, current residence in the United Kingdom, having no dietary restrictions (e.g. being vegetarian/vegan), not following any diet (e.g. Weight Watchers), and having ≥95% approval rate from previous Prolific participations. The University of Sheffield research ethics committee approved the study,Footnote3 and all participants gave informed consent. Recruitment took place in November 2020 and participants were reimbursed with £6.25 Prolific credit for their time.
Materials
2.1. Pictorial stimuli
The 30 food images used in this study were taken from an image database (CROCUFID: Toet et al., Citation2019) which is accompanied by valence ratings. There are a variety of images: the food depicted varied from being fresh to moulded, rotten, and partly consumed. This meant that images could be selected in order to solicit differential value judgements (standardised CROCUFID images are available from the OSF repository at https://osf.io/5jtqx; see supplementary materials for images used in this study).
2.2. Brief self-report questions
Participants answered demographic questions (age and gender) and their current level of hunger using a visual analogue scale that ranged from 0 (I am not hungry at all) to 10 (very hungry). The mean hunger level was 4.49 (SD = 2.61).
Procedure
Participants completed the study online which took an average of 28.45 minutes (SD = 14.29). Participants first completed self-report questions prior to completing the image-rating phase and the VBDM task (both programmed in PsychoPy and hosted on Pavlovia; Peirce et al., Citation2019).
2.3. Image-rating phase
Participants viewed 30 food images and made value judgements about them by placing each of the images into one of the four boxes using a computer mouse to indicate how much they would like to consume the food depicted in the image ‘right now’, ranging from: ‘A lot’, ‘A little bit’, ‘Not really’, and ‘Not at all’. Participants were instructed to rate all 30 images while assigning at least five to each value category. Subsequently, five images were randomly selected from each value category for use in the VBDM task.
2.4. VBDM task
To begin, five images randomly selected from each of the four value categories were displayed in the centre of the screen for 3 seconds each, followed by a 500 ms fixation cross, in order to remind participants about how they had ranked the images and to show them which subset of images had been randomly selected for use in the task. Participants subsequently completed the 2AFC trials. In each trial, two images appeared (one on the left and one on the right), and participants were instructed to press one of the two computer keys (‘Z’ for left and ‘M’ for right) to select one of the images as quickly as possible (see ). Participants first completed a practice block consisting of six trials. In the real task, there were three blocks of trials and in each block, participants were asked to think about the images in a different way. Specifically, the trial instructions varied between ‘which would you rather consume?’ (‘consume’ condition), ‘which do you like more?’ (‘like’ condition) and ‘which did you rate higher?’ (‘recall’ condition). Block order was randomised, with 150 trials in each, making 450 trials in total with a short break after every 50 trials. Difficulty levels across trials were varied, in that the difference in ratings between the two images could be 1, 2, or 3 (hard, medium, and easy choices, respectively). In each trial, there was a correct answer, and whether this appeared on the left or the right of the screen was random. Participants were given a maximum of 4 seconds to respond in each trial, responses outside of this window were classed as ‘miss trials’ as commonly used in VBDM tasks (e.g. Polanía et al., Citation2014).
Figure 1. Example trials in the VBDM task.Note. Trial wording varied between three blocks from ‘which would you rather consume?’, ‘which do you like more?’, and ‘which did you rate higher?’. Participants were instructed to press a key to select either of the images (‘Z’ for left, ‘M’ for right). Participants had up to 4 seconds to make their decision per trial, and each trial was followed by a 500 ms fixation cross located in the centre of the screen.
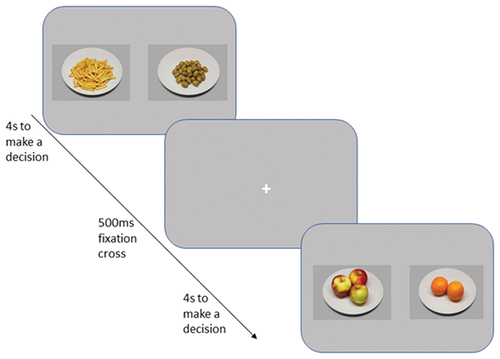
Data preparation and analysis
On the VBDM task, ’miss trials’ (responses exceeding 4 seconds) were removed (0.24%) as well as trials that were under 300 ms (0.03%) as these are likely to be fast guesses (Ratcliff et al., Citation2006) which resulted in the overall removal of 0.27% of trials.
There are a wide variety of sequential sampling models that are based on the common underlying assumption that decisions arise from a noisy accumulation-to-threshold process (Bogacz et al., Citation2006; Busemeyer et al., Citation2019). A prominent example is the DDM (Ratcliff & McKoon, Citation2008), of which there are also variations, but no general consensus of which model is optimal to use. We fitted the EZ-DDM (Wagenmakers et al., Citation2007) to RT and accuracy data from the VBDM task. This simplified version of the DDM is a powerful tool (Van Ravenzwaaij et al., Citation2017) that allows researchers to overcome the complexity of the parameter fitting procedure of the ‘full’ DDM (Stafford et al., Citation2020). Crucially, research has demonstrated that relatively simple models such as the EZ-DDM yield comparatively accurate and robust inferences when compared to more complex model fitting approaches (Dutilh et al., Citation2019; Lin et al., Citation2020). The EZ-DDM takes the mean correct RT, variance of correct RT, and response accuracy as input and produces three key parameters, which are drift rate (v), boundary separation (a), and non-decision time (Ter). We estimated the parameters for each participant in each condition and for each difficulty level.
Two-way repeated measure ANOVA and correlational analyses were used to analyse EA rates in accordance with our primary hypotheses. We calculated Bayes factors in JASP using default priorsFootnote4 (version 0.16; JASP Team, Citation2021) because we hypothesised specifically that trial wording would not affect EA rates and this method allows us to quantify evidence in favour of the null hypothesis beyond p-values (Wagenmakers et al., Citation2018). We used common cut-offs for interpretation (Jeffreys, Citation1961) with Bayes factors greater than 3 or else lower than 0.3, representing evidence in favour of the experimental and null hypotheses, respectively. All other analyses were conducted in RStudio version 4.0.2 (R Core Team, Citation2020). We did not make any pre-registered hypotheses about other DDM outputs (response thresholds and non-decision times), but these exploratory analyses are reported in the supplementary materials.
3. Results
3.1. Preregistered analyses
EA rates were analysed using a two-way repeated measure ANOVA with trial wording (3: ‘consume’; ‘like’; ‘recall’) and trial difficulty (3: easy; medium, hard) as within-subject variables. There was a significant main effect of difficulty, F(2, 116) = 286.34, p < .001, ηp2 = .83, with the Bayes factor indicating extreme evidence in favour of the experimental hypothesis (BF10 > 100), but not of trial wording, F(2, 116) = 2.81, p = .06, ηp2 = .05, with the Bayes factor indicating moderate evidence in favour of the null hypothesis (BF10 = .19). Furthermore, there was no significant interaction between trial wording and trial difficulty, F(3.39, 196.47) = 2.00, p = .11, ηp2 = .03, with the Bayes factor indicating strong evidence in favour of the null hypothesis (BF10 = .05).Footnote5
Post-hoc tests for the significant main effect of difficultyFootnote6 (applying the Holm-Bonferroni correction to p-values for multiple comparisons) revealed that EA rates in the easier trials (M = 2.53, SD = .82) were significantly higher compared to medium trials (M = 1.98, SD = .73; p < .001) and hard trials (M = 1.19, SD = .50; p < .001). Furthermore, EA rates on medium trials were significantly higher compared to EA rates on hard trials (p < .001). Overall, as shown in , these findings demonstrate that EA rates increased as trial difficulty decreased, and this was not modified by trial wording.
Figure 2. Mean evidence accumulation (EA) rates split by trial difficulty and trial wording.Notes. Light blue (circle) represents EA rates with the wording ‘which would you rather consume’, orange (triangle) represents EA rates with the wording ‘which do you like more’, and dark blue (square) represents EA rates with the wording ‘which did you rate higher’. Error bars represent the standard error of the mean (SE).
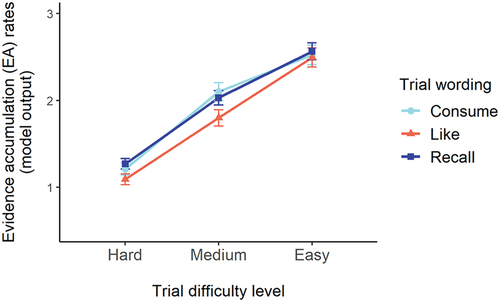
We conducted Pearson’s correlation coefficient analyses to explore the direction, strength, and significance of the relationships between the EA rates across the different trial wording blocks. As shown in , these correlational analyses revealed highly significant positive correlations between the EA rates in all three blocks (all ps < .001; consume and recall wording, r(57) = .68, p < .001; consume and like wording, r(57) = .46, p < .001; recall and like wording, r(57) = .44, p < .001).
Figure 3. Scatterplots to show correlations between the EA rates during the three blocks of trials.Notes. On the left is the correlation between the EA rates during the block of trials with ‘which would you rather consume’ and the block of trials with ‘which did you rate higher’. In the middle is the correlation between the EA rates during the block of trials with ‘which would you rather consume’ and the block of trials with ‘which do you like more’. On the right is the correlation between the EA rates during the block of trials with ‘which did you rate higher’ and the block of trials with ‘which do you like more’. The grey dashed line represents the line of equality. Shaded areas represent the 95% confidence interval.
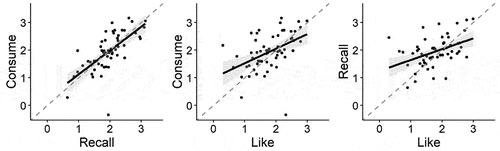
4. Discussion
We explored whether a coherent construct of value was captured by variations of trial wording that do not require participants to think about their momentary desire to consume objects depicted in pictures. As hypothesised, EA rates significantly increased alongside decreasing trial difficulty, and this was not modified by trial wording. Contrary to our hypothesis, there was a trend towards a main effect of trial wording on EA rates, regardless of trial difficulty, reflective of lower EA rates when participants considered how much they ‘liked’ the images compared to when asked to indicate how much they wanted to consume the food depicted or to recall which they had rated higher previously. This effect however was sensitive to the software used to fit the DDM, and it fell short of statistical significance when data were analysed using the EZ-DDM in accordance with our pre-registered analysis plan. Finally, the EA rates across the different blocks were significantly positively correlated with each other, although the coefficients were not as large (>0.70) as we predicted (Abma et al., Citation2016).
These findings are important because the majority of VBDM tasks require participants to make choices across trials about their strength of desire to consume a commodity (Krajbich et al., Citation2010; Mormann et al., Citation2010; Polanía et al., Citation2014; Tusche & Hutcherson, Citation2018), and our findings suggest that variations of trial wording are viable alternatives that can capture value-based choices. Importantly, the variations in trial wording are interpreted to be viable alternatives as opposed to completely identical substitutes because the correlations between the EA rates varied in strength across comparisons and the main effect of trial wording approached statistical significance. Furthermore, in line with other research, the EA rates increased as trial difficulty decreased (Polanía et al., Citation2014). This establishment of a ‘difficulty effect’, regardless of the wording of the value-based question on that block of trials, is important because it demonstrates that the way that participants were responding during the 2AFC trials was compatible with the value judgments that they had made during the image-rating task.
The core focus of this study was EA rates because this parameter is hypothesised to represent the value (Field et al., Citation2020); however, analyses on other decision parameters derived from the EZ-DDM (response thresholds and non-decision times) are presented in the supplementary materials. An implication of this research is that variations of trial wording used in this study may be appropriate to implement in future research contexts whereby it is undesirable to ask participants to make consummatory judgements, such as those in recovery from addiction (Copeland et al., Citation2021; Field et al., Citation2020). It may be less aversive for people in recovery to express preferences in relation to how much they like an image rather than in relation to their desire to consume the item depicted; indeed, similar procedures have been implemented with people with addiction in clinical settings (Moeller & Stoops, Citation2015). A limitation of this study is that the EZ-DDM model fitting approach (Wagenmakers et al., Citation2007) precluded the fixing of decision parameters across conditions; however, when we used alternative software (the fast-dm-30; Voss et al., Citation2015) that enabled us to fix decision parameters, the main effect of trial wording on EA rates became statistically significant (see supplementary materials). A broader issue is that alternative, more complex models may provide a better fit to behavioural data and thereby be more sensitive to the effects of trial wording (Colas, Citation2017)—an important topic for future research. A further limitation is that participants made value ratings and were reminded of these, prior to completion of the 2AFC task which may have inadvertently affected participant behaviour. Future research could explore the impact of all combinations of initial value ratings and subsequent wording of 2AFC trials using a full factorial design to investigate the robustness of the findings reported in this study.
To conclude, this study was an exploration into the sensitivity of VBDM tasks to minor alterations in trial wording. Results demonstrated robust evidence that differences in EA rates (sensitivity to whether trials are easy, medium, or hard determined by participants’ own value ratings) were equivalent across the variations of trial wording. EA rates were affected by trial wording, however this was confined to absolute EA rates, was not robust, and appeared sensitive to the software used to fit the DDM. This study contributes towards an initial validation of alternative wording that can be appropriate to implement in future research in which it is undesirable to ask participants to make consummatory judgments about the stimuli, such as research into VBDM in people who are in recovery from addiction.
Correction
This article was originally published with errors, which have now been corrected in the online version. Please see Correction (http://dx.doi.org/10.1080/23311908.2022.2086707)
Open Scholarship
This article has earned the Center for Open Science badges for Open Data and Preregistered. The data and materials are openly accessible at https://researchbox.org/505 and https://aspredicted.org/2tm3s.pdf.
Supplemental Material
Download MS Word (413.2 KB)Acknowledgements
We would like to thank Adriel Chua for his help programming the online task. We would also like to thank the Editor and the two anonymous reviewers for their constructive feedback on earlier versions of the manuscript.
Disclosure statement
No potential conflict of interest was reported by the author(s).
Data availability statement
Data and analysis scripts are available and can be found on researchbox: https://researchbox.org/505
Supplementary material
Supplemental data for this article can be accessed online at https://doi.org/10.1080/23311908.2022.2079801
Additional information
Funding
Notes on contributors
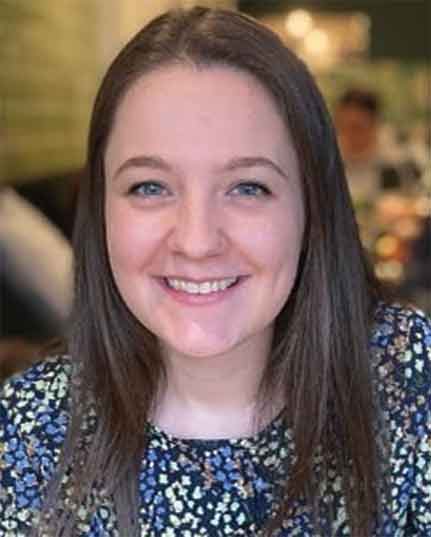
Amber Copeland
Amber Copeland is a PhD student, and in her PhD project she is applying computational models of value-based decision-making (VBDM) to investigate the potential mechanisms that underlie behaviour change and recovery from addiction.
Tom Stafford
Dr Tom Stafford studies learning and decision making. Much of his research looks at risk and bias, and their management, in decision making. He is also interested in skill learning, using measures of behaviour informed by work done in computational theory, robotics and neuroscience. More recently a strand of his research looks at complex decisions, and the psychology of reason, argument and persuasion.
Matt Field
Professor Matt Field conducts research into the psychological mechanisms that underlie alcohol problems and other addictions. He is particularly interested in the roles of decision-making and impulse control in addiction, recovery, and behaviour change more broadly.
Notes
1. See Table S1 in supplementary materials for a comparison of methods across different studies (including whether precise trial wording is included within the manuscript).
2. Difficulty is operationalised as the difference in value rating between the two images presented on each trial of the VBDM task. Trials are categorised into the following difficulty levels: hard (difference of 1), medium (difference of 2), or easy (difference of 3).
3. Ethical approval number: 033881.
4. r scale fixed effects = 0.5.
5. As requested by an anonymous reviewer, we repeated these analyses using alternative software—the fast-dm-30 (Voss et al., Citation2015)—which enabled us to explore if trial wording would affect EA rates after fixing response thresholds across difficulty conditions and non-decision times across all conditions (i.e. per participant). These analyses are reported in the supplementary materials, but in brief we found that the main effect of trial wording became statistically significant when analysed with the fast-dm-30, with a Bayes factor indicative of moderate evidence in favour of the experimental hypothesis (BF10 = 4.18); contrasting with moderate evidence in favour of the null (BF10 = .19) when the EZ-DDM was used.
6. See supplementary materials for post-hoc tests on the main effect of trial wording and interaction between trial wording and trial difficulty.
References
- Abma, I. L., Rovers, M., & van der Wees, P. J. (2016). Appraising convergent validity of patient-reported outcome measures in systematic reviews: Constructing hypotheses and interpreting outcomes. BMC Research Notes, 9(1), 226. https://doi.org/10.1186/s13104-016-2034-2
- Berkman, E. T., Hutcherson, C. A., Livingston, J. L., Kahn, L. E., & Inzlicht, M. (2017). Self-control as value-based choice. Current Directions in Psychological Science, 26(5), 422–10. https://doi.org/10.1177/0963721417704394
- Bogacz, R., Brown, E., Moehlis, J., Holmes, P., & Cohen, J. D. (2006). The physics of optimal decision making: A formal analysis of models of performance in two-alternative forced-choice tasks. Psychological Review, 113(4), 700–765. https://doi.org/10.1037/0033-295X.113.4.700
- Busemeyer, J. R., Gluth, S., Rieskamp, J., & Turner, B. M. (2019). Cognitive and neural bases of multi-attribute, multi-alternative, value-based decisions. Trends in Cognitive Sciences, 23(3), 251–263. https://doi.org/10.1016/j.tics.2018.12.003
- Colas, J. T. (2017). Value-based decision making via sequential sampling with hierarchical competition and attentional modulation. PLoS One, 12(10), e0186822. https://doi.org/10.1371/journal.pone.0186822
- Copeland, A., Stafford, T., & Field, M. (2021). Recovery from addiction: A synthesis of perspectives from behavioral economics, psychology, and decision modeling. In D. Frings & I. P. Albery (Eds.), The Handbook of Alcohol Use (pp. 563–579). Academic Press. https://doi.org/10.1016/B978-0-12-816720-5.00002-5
- Dutilh, G., Annis, J., Brown, S. D., Cassey, P., Evans, N. J., Grasman, R. P. P. P., Hawkins, G. E., Heathcote, A., Holmes, W. R., Krypotos, A.-M., Kupitz, C. N., Leite, F. P., Lerche, V., Lin, Y.-S., Logan, G. D., Palmeri, T. J., Starns, J. J., Trueblood, J. S., van Maanen, L., … Donkin, C. (2019). The quality of response time data inference: A blinded, collaborative assessment of the validity of cognitive models. Psychonomic Bulletin & Review, 26(4), 1051–1069. https://doi.org/10.3758/s13423-017-1417-2
- Field, M., Heather, N., Murphy, J. G., Stafford, T., Tucker, J. A., & Witkiewitz, K. (2020). Recovery from addiction: Behavioral economics and value-based decision making. Psychology of Addictive Behaviors, 34(1), 182–193. https://doi.org/10.1037/adb0000518
- JASP Team. (2021). JASP (Version 0.16). https://jasp-stats.org/
- Jeffreys, H. (1961). Theory of probability (3rd ed.). Oxford University Press.
- Krajbich, I., Armel, C., & Rangel, A. (2010). Visual fixations and the computation and comparison of value in simple choice. Nature Neuroscience, 13(10), 1292–1298. https://doi.org/10.1038/nn.2635
- Lakens, D. (2021). Sample size justification. PsyArXiv. https://doi.org/10.31234/osf.io/9d3yf
- Levy, D. J., & Glimcher, P. W. (2012). The root of all value: A neural common currency for choice. Current Opinion in Neurobiology, 22(6), 1027–1038. https://doi.org/10.1016/j.conb.2012.06.001
- Lin, H., Saunders, B., Friese, M., Evans, N. J., & Inzlicht, M. (2020). Strong effort manipulations reduce response caution: A preregistered reinvention of the ego-depletion paradigm. Psychological Science, 31(5), 531–547. https://doi.org/10.1177/0956797620904990
- Moeller, S. J., & Stoops, W. W. (2015). Cocaine choice procedures in animals, humans, and treatment-seekers: Can we bridge the divide? Pharmacology Biochemistry and Behavior, 138, 133–141. https://doi.org/10.1016/j.pbb.2015.09.020
- Mormann, M., Malmaud, J., Huth, A., Koch, C., & Rangel, A. (2010). The drift diffusion model can account for the accuracy and reaction time of value-based choices under high and low time pressure. Judgment and Decision Making, 5(6), 437–449. https://papers.ssrn.com/sol3/papers.cfm?abstract_id=1901533
- Peirce, J., Gray, J. R., Simpson, S., MacAskill, M., Höchenberger, R., Sogo, H., Kastman, E., & Lindeløv, J. K. (2019). PsychoPy2: Experiments in behavior made easy. Behavior Research Methods, 51(1), 195–203. https://doi.org/10.3758/s13428-018-01193-y
- Pennington, C. R., Jones, A., Bartlett, J. E., Copeland, A., & Shaw, D. J. (2021). Raising the bar: Improving methodological rigour in cognitive alcohol research. Addiction, 116(11), 3243–3251. https://doi.org/10.1111/add.15563
- Polanía, R., Krajbich, I., Grueschow, M., & Ruff, C. C. (2014). Neural oscillations and synchronization differentially support evidence accumulation in perceptual and value-based decision making. Neuron, 82(3), 709–720. https://doi.org/10.1016/j.neuron.2014.03.014
- R Core Team. (2020). A language and environment for statistical computing. R Foundation for Statistical Computing. https://www.R-project.org/
- Rangel, A., Camerer, C., & Montague, P. R. (2008). A framework for studying the neurobiology of value-based decision making. Nature Reviews Neuroscience, 9(7), 545–556. https://doi.org/10.1038/nrn2357
- Ratcliff, R., Thapar, A., & McKoon, G. (2006). Aging, practice, and perceptual tasks: A diffusion model analysis. Psychology and Aging, 21(2), 353–371. https://doi.org/10.1037/0882-7974.21.2.353.
- Ratcliff, R., & McKoon, G. (2008). The diffusion decision model: Theory and data for two-choice decision tasks. Neural Computation, 20(4), 873–922. https://doi.org/10.1162/neco.2008.12-06-420
- Ratcliff, R., Smith, P. L., Brown, S. D., & McKoon, G. (2016). Diffusion decision model: Current issues and history. Trends in Cognitive Sciences, 20(4), 260–281. https://doi.org/10.1016/j.tics.2016.01.007
- Stafford, T., Pirrone, A., Croucher, M., & Krystalli, A. (2020). Quantifying the benefits of using decision models with response time and accuracy data. Behavior Research Methods, 52(5), 2142–2155. https://doi.org/10.3758/s13428-020-01372-w
- Toet, A., Kaneko, D., de Kruijf, I., Ushiama, S., van Schaik, M. G., Brouwer, A.-M., Kallen, V., & van Erp, J. B. F. (2019). CROCUFID: A cross-cultural food image database for research on food elicited affective responses. Frontiers in Psychology, 101, 1–21. https://doi.org/10.3389/fpsyg.2019.00058
- Tusche, A., & Hutcherson, C. A. (2018). Cognitive regulation alters social and dietary choice by changing attribute representations in domain-general and domain-specific brain circuits. ELife, 7, e31185. https://doi.org/10.7554/eLife.31185
- van Ravenzwaaij, D., Donkin, C., & Vandekerckhove, J. (2017). The EZ diffusion model provides a powerful test of simple empirical effects. Psychonomic Bulletin & Review, 24(2), 547–556. https://doi.org/10.3758/s13423-016-1081-y
- Voss, A., Voss, J., & Lerche, V. (2015). Assessing cognitive processes with diffusion model analyses: A tutorial based on fast-dm-30. Frontiers in Psychology, 6. https://www.frontiersin.org/article/10.3389/fpsyg.2015.00336; https://www.readcube.com/articles/10.3389/fpsyg.2015.00336
- Wagenmakers, E.-J., Van Der Maas, H. L. J., & Grasman, R. P. P. P. (2007). An EZ-diffusion model for response time and accuracy. Psychonomic Bulletin & Review, 14(1), 3–22. https://doi.org/10.3758/BF03194023
- Wagenmakers, E.-J., Love, J., Marsman, M., Jamil, T., Ly, A., Verhagen, J., Selker, R., Gronau, Q. F., Dropmann, D., Boutin, B., Meerhoff, F., Knight, P., Raj, A., van Kesteren, E.-J., van Doorn, J., Šmíra, M., Epskamp, S., Etz, A., Matzke, D., … Morey, R. D. (2018). Bayesian inference for psychology. Part II: Example applications with JASP. Psychonomic Bulletin & Review, 25(1), 58–76. https://doi.org/10.3758/s13423-017-1323-7