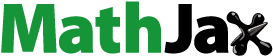
Abstract
One of the interesting methods for conversion of synthesis gas to heavy hydrocarbons is Fischer–Tropsch process. The process has some bottlenecks, such as hot spot formation and low degree of conversion. In this work, computational fluid dynamics technique was used to simulate conversion of synthetic gas and product distribution. Also, hot spot formation in the catalytic fixed-bed reactor was investigated in several runs. Simulation results indicated that hot spot formation occurred more likely in the early and middle part of reactor due to high reaction rates. Based on the simulation results, the temperature of hot spots increased with increase in the inlet temperature as well as pressure. Among the many CFD runs conducted, it is found that the optimal temperature and pressure for Fischer–Tropsch synthesis are 565 K and 20 bar, respectively. As it seems that the reactor shall work very well under optimal conditions, the reaction rates and catalyst duration would simultaneously be maximum .
Public Interest Statement
Nowadays, the energy crisis is a real challenge to human society, since oil sources in the world are declining. A promising alternative approach in this regard is to directly convert natural gas to liquid fuel by means of gas-to-liquid (GTL) technology. Fischer–Tropsch synthesis (FTS) with a complex network of reactions in parallel and/or in series converts synthesis gas into valuable liquid hydrocarbons, clean fuels, and chemical feedstock.
1. Introduction
Nowadays, the energy crisis is a real challenge to human society, since oil sources in the world are declining. A promising alternative approach in this regard is to directly convert natural gas to liquid fuel by means of gas-to-liquid (GTL) technology (Li et al., Citation2009). Fischer–Tropsch synthesis (FTS), with a complex network of reactions in parallel and/or in series, converts synthesis gas into valuable liquid hydrocarbons, clean fuels, and chemical feedstock (Li, Zhang, Zhang, & Cao, Citation2007; Ma, Sun, Ying, & Fang, Citation2009; Pour, Housaindokht, Tayyari, & Zarkesh, Citation2010a; Pour, Zare, & Zamani, Citation2010b; Takassi, Salooki, & Esfandyari, Citation2011). In this process, a wide range of paraffins and olefins (from C1 to C7+) can be synthesized, depending on the operating conditions, catalyst characteristics, and type of the process (Feyzi & Jafari, Citation2012). Because of escalation in the price of crude oil, air pollution, global warming, and resulting climate change, the process is of great interest as a vital alternative for producing clean fuels (Dai & Yu, Citation2008; Forghani, Elekaei, & Rahimpour, Citation2009; Hao, Bai, Xiang, & Li, Citation2009; James, Mesubi, Ako, & Maity, Citation2010; Wan et al., Citation2007). The fixed-bed Fischer–Tropsch process plays a unique role in FTS industrial units, so that a variety of hydrocarbons ranging from methane to wax are produced by this process (Wang, Xu, Li, Zhao, & Zhang, Citation2003). Figure shows a typical FTS plant equipped with fixed-bed reactor. The kinetic scheme used in this paper has some novelties; firstly, the scheme is not a kinetic totally rendered earlier. The kinetic relations are selective from different models. Secondly, we select this kinetic scheme according to our pilot plant and experimental data which is published earlier (Aligolzadeh, Citation2011). In this paper, we focus on the production of C7+ hydrocarbons from reactants, which are plentifully available and compatible with national resources in Iran (natural gas). Hence, the process and operation conditions are set, so that the waxes formation is low.
A heterogeneous fixed-bed reactor was modeled/simulated, so as to evaluate the performance of the reactor, in which study, there was good agreement between simulation and experimental data (Wang et al., Citation2003). Later on, an experimental study on the milli-structured fixed-bed reactor for FTS process was performed, along with mathematical modeling (Knochen, Güttel, Knobloch, & Turek, Citation2010). Rafiq, Jakobsen, Schmid, and Hustad (Citation2011) carried out experimental analysis as well as mathematical modeling of a fixed-bed reactor for FTS process fed with biosynthesis gas. A two-dimensional pseudo-homogeneous model was developed by Rafiq et al. to evaluate the performance of the FTS reactor, the results of which showed that the model predicts experimental data successfully. Computational fluid dynamics (CFD) technique as a powerful device was applied for three-dimensional simulation of hydrogenation of carbon monoxide in a fixed-bed FTS reactor (Miroliaei, Shahraki, Atashi, & Karimzadeh, Citation2012). They discovered that operational conditions extremely affect the product distribution; and, the more temperature increases, the more conversion increases. Moreover, it was found that the selectivity parameter and mass fraction of the products increase in value, as a result of temperature or the ratio of H2/CO increase.
In this work, two-dimensional simulation of GTL fixed-bed reactor is carried out using CFD technique. The commercial CFD solver, FLUENT.6.3, is used for numerically solving the governing equations. Effects of operating pressure and feed temperature on the hot spot formation are investigated. A detailed kinetic scheme is used to estimate the rate of materials generated/consumed during the process once started. Mass fraction of present components, evaluation of heat of reactions, and analysis of hot spot formation and its location, all ascertain the necessity of using a detailed kinetic scheme. In fact, the novelty of present work is limited for incorporating a detailed kinetic scheme into the CFD solver and looking for hot spot formation.
2. Kinetic scheme
In this work, we use the kinetic which best fitted the experimental data at the outlet of GC. Kinetic scheme of Yang et al. is used to predict the rate of reactions and added to the CFD solver (Yang et al., Citation2003):(1)
(1)
(2)
(2)
(3)
(3)
We can consider four steps for these reactions, the following reactions are considered as leading FTS reactions (Yang et al., Citation2003).
First step is adsorption of reactants:(4)
(4)
(5)
(5)
Second step is chain initiation. We assumed that chain growth is initiated by the adsorption of CO on the active sites (Yang et al., Citation2003).(6)
(6)
(7)
(7)
(8)
(8)
(9)
(9)
Third step is chain propagation (Yang et al., Citation2003):(10)
(10)
(11)
(11)
Fourth step is chain termination and desorption of products, termination of the chain growth can occur via several routes for FTS (Yang et al., Citation2003):(12)
(12)
(13)
(13)
where s1 is a vacant catalytic site, on which hydrocarbon can be formed. The reaction rate equations for carbon dioxide, methane, paraffins, and olefins are as follows, and the kinetic parameters are listed in Table . According to water-gas shift reaction, the rate equation for CO2 formation is as follows:(14)
(14)
Table 1. Kinetic parameters used in the present study
where(15)
(15)
The reaction rate for methane, paraffins, and olefins formation are given:(16)
(16)
(17)
(17)
(18)
(18)
where(19)
(19)
(20)
(20)
(21)
(21)
The rate constant in the above equations can be defined according to Arrhenius relation, which is consistent with what CFD solver needs:(22)
(22)
where n is temperature exponent (dimensionless). The aforementioned kinetics has been developed, when using industrial manganese-promoted iron catalyst, including 0.5 wt% Mn and 1.5 wt% Fe. All reactions are defined volumetric type with generalized laminar finite-rate formulation.
3. Governing equations
There are a variety of commercial CFD solvers coded based on the finite element, finite difference, and finite volume methods. In the present study, finite volume method (FLUENT 6.3) was utilized for solving the governing equations.
3.1. Momentum conservation equation
The conservation of momentum is expressed by:(23)
(23)
The equation is transformed to so-called Brinkman equation, which is valid for porous media and cylindrical coordinates, similar to the conditions of FTS catalytic reactor.(24)
(24)
3.2. Mass conservation equation
General equation for conservation of mass, continuity equation, is defined as follows:(25)
(25)
This equation may be simplified if we make the assumption that there is no variation of fluid density with time (steady-state condition), so the instability term (partial derivative of density with respect to time) in the left-hand side of Equation 25 becomes zero. The right-hand side term is likewise zero.(26)
(26)
3.3. Energy conservation equation
Energy conservation equation valid for porous media including conduction flux terms is given by:(27)
(27)
The term on the left-hand side of Equation 27 is the heat transfer due to convection. On the right-hand side, the terms are conduction heat transfer, species enthalpy due to diffusion flux of, for example, species j, heat dissipation, and heat source, respectively. Through the porous medium, conduction flux is calculated using an effective conductivity, as Equation 28 shows. The effective thermal conductivity in the porous medium, keff, is calculated as the volume-weighted average of the fluid and solid conductivity (Shahhosseini, Alinia, & Irani, Citation2009):(28)
(28)
3.4. Species transport equation
The equation governing species transport through the reactor is of course well known:(29)
(29)
Assuming steady-state and irrotational flow (θ direction), Equation 29 diminishes such:(30)
(30)
The intrinsic kinetics scheme is independent of boundary layer effects. But in the species transport equation, we have the terms, including the local velocity, which affects on the mass transfer and conversion, and the component concentration. When the species concentration changes with velocity profile, thus the rate of all reactions alter according to local concentration, as the reaction rate equations dictate. This is why we should consider boundary layer effects on the calculations.
4. Mesh generation and grid dependency
A two-dimensional axisymmetric system (0.046 × 7 m) is considered as the domain of fluid, through which the governing equations should numerically be solved. Two different grids for the mentioned geometry are generated using Gambit software 2.3.2 edition; they have a total number of 84330 and 112440 structured quadrilateral elements, respectively. Primary simulation is carried out by commercial CFD solver, FLUENT (Fluent Inc., 2003) 6.3 edition, for checking grid dependency. Mass fraction of CO versus reactor length for both grids is shown in Figure .
It is clear that the results obtained for both grids are coincident; therefore, the results of coarser grid are as good as finer grid. Consequently, choosing the coarser grid, rather than finer one, would enable solving the problem more quickly. Even the coarser grid is fine enough to capture near the wall treatment (wall effects). The height of first cells adjacent to the wall boundary is fine enough and the height of next cells grows up with a density ratio of 1.2 to capture near the wall treatment. The mesh is designed to be denser near the inlet and outlet boundaries, as one can see in Figure .
5. Boundary conditions
The geometry discussed above is subjected to the following boundary conditions:
The flow velocity was specified as inlet boundary condition (0.55 m/s).
Outflow boundary was considered as outlet boundary condition.
Wall boundary condition was set for other boundaries with zero diffusive flux for all species.
The solver is selected to be pressure-based because of solving incompressible flow. Among several pressure–velocity-coupling algorithms, the well-known SIMPLE algorithm is utilized. Moreover, the second-order upwind scheme is employed to discrete the governing equations due to its high accuracy. The turbulence modeling is passed over throughout the domain because of laminar flow.
6. Operating condition
The temperature at the inlet of the reactor is 553 K and the system works under different pressures (see Table ). The characteristics of the reactor and catalyst are listed in Table .
Table 2. Characteristics of fixed-bed reactor and catalyst
Bed characteristics are estimated through rigorous porous media relations having basic information about size and shape of catalyst. Particle solid is considered spherically 3 mm in diameter and the porosity of the bed including particles is assumed as 0.3894. The term F in Equation 24 symbolizes the momentum source term induced by body forces or here by porous media which is identical to:(31)
(31)
The first term on the right-hand side is engaged in F as viscous loss and the second as inertial loss, where Fi refers to source of ith (x, r or z) momentum equation. The notation |V| shows velocity magnitude everywhere, and D and C are prescribed matrices being able to switch to scalar in case of isotropic environment. For homogeneous porous media, above equation is changed to:(32)
(32)
where α is permeability and C2 is the inertial resistance factor and shows itself as diagonal elements of Dij matrix, where the rest of elements are all zero. In fact, porous media theory is nothing more than a momentum sink on the right-hand side of momentum conservation equations. Other important subject in the porous media formulation is the pressure drop modeling; somehow, there are a few theoretical, empirical, or semi-empirical models for evaluating the pressure drop across porous media. The most used model is the Ergun’s equation, a semi-empirical equation, which correlates the pressure drop with velocity magnitude, as demonstrated beneath (Ergun, Citation1952).(33)
(33)
Or in simpler form:(34)
(34)
Permeability and inertial loss coefficient may theoretically be determined by physical properties of the catalyst and bed porosity. The relevant relations for the aforesaid quantities are:(35)
(35)
Effect of sphericity on the porous pressure drop is not included in Equation 35 holding it to elsewhere (Equation 33); hence, both permeability and inertial loss coefficient are shape independent. Viscous and inertial resistances for the bed are calculated with the help of equations. We get the values of 1.052385e + 08 1/m2 and 12064 1/m, respectively, for viscous and inertial resistances. It must be said that viscous resistance is inversely associated with permeability.
In the present work, all physical properties of feed are assumed to be constant and temperature independent, except for density and viscosity that they are computed by (incompressible flow of an ideal gas).Footnote1 Mass diffusivity is also calculated by kinetic theory (FLUENT6.3., CitationXXXX).
The species mass fraction at the inlet is considered, as one can see in Table . The system is insulated and the heat flux from the wall to environment is zero.
Table 3. Species mass fraction at the inlet
7. Results and discussion
In this section, the results of CFD simulation are compared with experimental data reported by Yang et al., as one can see in Table , showing that the simulation results are in good agreement with experimental data (Yang et al., Citation2003). The last column shows that the maximum relative error obtained is below 6%.
Table 4. Comparison between CFD simulation results with experimental data
Temperature profile as a function of reactor length under different total pressures is displayed in Figure . According to this figure, the temperatures of hot spots and products at the outlet rise as pressure increases. It is observed that the hot spot location occurs almost near the reactor inlet due to high reactants concentration there. The locus of hot spot formation shifts gently to the inside, as a result of decreasing pressure. This conclusion is completely according to what Chapman–Enskog equation represents; penetration rate is directly proportional to the temperature, penetration, and molar flux increase caused by rising temperature, leading to shift in hot spot location to the inside of the reactor (Miroliaei et al., Citation2012). Therefore, considering the key role of the temperature on the product selectivity, it can be expected that rising reactor bulk temperature caused by bulk pressure increase will lead to the production of undesirable heavy linear hydrocarbons.
In the following, four different temperatures were considered to study the effect of inlet temperature on the hot spot formation. The characteristics of flow inlet was reported in literature (Yang et al., Citation2003). Figure shows the effect of inlet temperature on the hot spot formation.
As obvious, the temperature related to hot spot formation increases as inlet temperature rises. It is ascribed to increase in reactants enthalpy in addition to raising reaction rate. Contours of mole fraction for CO and H2 are illustrated in Figure , showing that mole fraction of CO and H2 decreases along the length of the reactor. Increasing pressure and temperature affects to increasing heavy hydrocarbons. We can rationalize this idea by the activation energy of Olefin formation, which is 97.37 kJ mol−1, much smaller than that of paraffin’s (Yang et al., Citation2003), which can interpret the general fact that rising reactor bulk temperature caused by bulk pressure will increase the production of heavy linear hydrocarbons on the Fe–Mn catalysts than the other iron-based catalysts.
Predicted concentration of products through the axis of fixed-bed reactor is shown in Figures –. All contours are extracted from FLUENT6.3 and then depicted by MATLAB 6.5Ed software. In all figures, mole fractions change was depicted on vertical axis and reactor length on horizontal axis.
Figures – indicate that the concentration of products increases by reaction progress along the length of the reactor. It can be attributed to the inhomogeneity in the flow around a catalytic particle, which strongly affects the concentration distribution in the reaction zone (Miroliaei et al., Citation2012).
Although temperature rise has a positive effect on the reactor performance, further increase will cause catalyst to damage because of hot spot formation. Using CFD simulation, optimal operational temperature and pressure for fixed-bed reactor of FTS were obtained as 565 K and 20 bar, respectively. The optimal conditions were found through several CFD runs; however, the reactor works under optimal conditions as long as the pressure and temperature are 565 K and 20 bar. Simulation results demonstrated that C7+ productivity and the rate of production of linear hydrocarbons increase as temperature and pressure rise. In addition, more paraffins with equal carbon chains are produced in comparison with olefins. It is ascribed to higher reaction rate of paraffins compared to olefins.
8. Conclusion
Two-dimensional CFD technique was employed to predict the conversion of synthetic gas (CO and H2) and distribution of products through fixed-bed reactor in the FTS process. Results show that conversion of CO and H2 and the selectivity of C7+ as it seen in Figure increase along the length of reactor. As a consequence of high reaction rates in the early part of the reactor that decrease along the length of the reactor, hot spot formation occurred more likely in the early part and less in the middle part of the reactor. Results show that any increase in the temperature leads to higher reaction rate, but it causes deactivation of catalyst due to hot spot formation. For this reason, there must be some optimal conditions for FTS process, under which the rate of desirable reactions and catalyst duration shall simultaneously be maximum. Among the many CFD runs done, optimal temperature and pressure for fixed-bed reactor of FTS process were acquired as 565 K and 20 bar, respectively.
Nomenclature | ||
g | = | acceleration due to gravity (m s−2) |
F | = | surface tension force components and also body force (N) |
Q | = | mass source term (kg m−3 s−1) |
C | = | molar concentration (kg mol m−3) |
cp | = | heat capacity (J mol−1 K−1) |
h | = | fluid enthalpy (kJ kg−1) |
hj | = | enthalpy of species j (kJ kg−1) |
Jj | = | diffusion flux of species j (kg m−2 s−1) |
k | = | conductivity of fluid phase (W m−1 K−1) |
α | = | permeability (m2) |
keff | = | effective thermal conductivity (W m−1 K−1) |
kf | = | fluid-phase thermal conductivity (W m−1 K−1) |
ks | = | solid medium thermal conductivity (W m−1 K−1) |
P | = | total pressure (bar) |
ra | = | the et rate of production of species (mol g−1 s−1) |
Sf | = | heat source (kJ m−3 s−1) |
T | = | temperature of the reaction system (K) |
t | = | time (s) |
u | = | superficial velocity of liquid phase (m s−1) |
ρf | = | density of fluid phase (kg m−3) |
= | velocity vector (m s−1) | |
τ | = | shear stress (N m−2) |
ε | = | void fraction (–) |
ε | = | porosity of the medium (–) |
= | dynamic viscosity (N s m−2) | |
= | specific weight (kg m−2 s−2) | |
= | porosity of porous layer (–) | |
Ef | = | total fluid energy (kJ) |
Additional information
Funding
Notes on contributors
Hamed Aligolzadeh
Hamed Aligolzadeh received his MSc in Chemical Engineering from Iran University of Science and Technology in Tehran, I.R. Iran. His research interests are GTL technology and CFD simulation.
Ali Jebreili Jolodar
Ali Jebreili Jolodar received his MSc in Chemical Engineering from Mazandaran University of Science and Technology, I.R. Iran. His research interests are GTL and numerical simulation. He manages above 10 national projects in the Research Institute of Petroleum Industry, I.R. Iran.
Rasool Mohammadikhah
Rasool Mohammadikhah is assistant professor of Chemical Engineering and a scientific member of the Research Institute of Petroleum Industry, I.R. Iran. He received his doctorate in Chemical Engineering from University of Tehran in Tehran (2014), I.R. Iran. His academic publications include up to 30 technical papers. His research interests are molecular thermodynamics, molecular dynamic simulation, and CFD simulation.
Notes
1. “Incompressible ideal gas” in the FLUENT 6.3 ed, FLUENT Inc., USA.
References
- Aligolzadeh, H. (2011). CFD simulation of Fischer–Tropsch synthesis fixed bed reactor ( MSc thesis). Iran University of Science and Technology, Tehran.
- Dai, X., & Yu, C. (2008). Characterization and catalytic performance of CeO2-Co/SiO2 catalyst for Fischer–Tropsch synthesis using nitrogen-diluted synthesis gas over a laboratory scale fixed-bed reactor. Journal of Natural Gas Chemistry, 17, 17–23.10.1016/S1003-9953(08)60020-1
- Ergun, S. (1952). Fluid flow through packed columns. Chemical Engineering Progress, 48, 89–94.
- Feyzi, M., & Jafari, F. (2012). Study on iron–manganese catalysts for Fischer–Tropsch synthesis. Journal of Fuel Chemistry and Technology, 40, 550–557.10.1016/S1872-5813(12)60021-8
- FLUENT6.3 ed. (XXXX). FLUENT Inc., USA.
- Forghani, A. A., Elekaei, H., & Rahimpour, M. R. (2009). Enhancement of gasoline production in a novel hydrogen-permselective membrane reactor in Fischer–Tropsch synthesis of GTL technology. International Journal of Hydrogen Energy, 34, 3965–3976.10.1016/j.ijhydene.2009.02.038
- Hao, Q., Bai, L., Xiang, H., & Li, Y. (2009). Activation pressure studies with an iron-based catalyst for slurry Fischer–Tropsch synthesis. Journal of Natural Gas Chemistry, 18, 429–435.10.1016/S1003-9953(08)60134-6
- James, O. O., Mesubi, A. M., Ako, T. C., & Maity, S. (2010). Increasing carbon utilization in Fischer–Tropsch synthesis using H2-deficient or CO2-rich syngas feeds. Fuel Processing Technology, 91, 136–144.10.1016/j.fuproc.2009.09.017
- Knochen, J., Güttel, R., Knobloch, C., & Turek, T. (2010). Fischer–Tropsch synthesis in milli-structured fixed-bed reactors: Experimental study and scale-up considerations. Chemical Engineering and Processing: Process Intensification, 49, 958–964.10.1016/j.cep.2010.04.013
- Li, J., Zhang, J., Zhang, R., & Cao, W. (2009). Nanosized cobalt-based catalyst prepared by supercritical phase condition for Fischer–Tropsch synthesis. Journal of Natural Gas Chemistry, 18, 325–330.10.1016/S1003-9953(08)60110-3
- Li, T., Yang, Y., Tao, Z., Wan, H., An, X., Zhang, C., Xiang, H., & Li, Y. (2007). Effect of sulfate on an iron manganese catalyst for Fischer–Tropsch synthesis. Journal of Natural Gas Chemistry, 16, 354–362.10.1016/S1003-9953(08)60004-3
- Ma, X., Sun, Q., Ying, W., & Fang, D. (2009). Effects of the ratio of Fe to Co over Fe-Co/SiO2 bimetallic catalysts on their catalytic performance for Fischer–Tropsch synthesis. Journal of Natural Gas Chemistry, 18, 232–236.10.1016/S1003-9953(08)60102-4
- Miroliaei, A. R., Shahraki, F., Atashi, H., & Karimzadeh, R. (2012). Comparison of CFD results and experimental data in a fixed bed Fischer–Tropsch synthesis reactor. Journal of Industrial and Engineering Chemistry, 18, 1912–1920.
- Pour, A., Housaindokht, M. R., Tayyari, S. F., & Zarkesh, J.. (2010). Deactivation studies of nano-structured iron catalyst in Fischer–Tropsch synthesis. Journal of Natural Gas Chemistry, 19, 333–340.10.1016/S1003-9953(09)60061-X
- Pour, A., Zare, M., & Zamani, Y. (2010). Studies on product distribution of alkali promoted iron catalyst in Fischer–Tropsch synthesis. Journal of Natural Gas Chemistry, 19, 31–34.10.1016/S1003-9953(09)60025-6
- Rafiq, M. H., Jakobsen, H. A., Schmid, R., & Hustad, J. E. (2011). Experimental studies and modeling of a fixed bed reactor for Fischer–Tropsch synthesis using biosyngas. Fuel Processing Technology, 92, 893–907.10.1016/j.fuproc.2010.12.008
- Shahhosseini, S., Alinia, S., & Irani, M. (2009). CFD simulation of fixed bed reactor in Fischer–Tropsch synthesis of GTL technology. WASET, 60, 585–589.
- Takassi, M. A., Salooki, M., & Esfandyari, M. (2011). Fuzzy model prediction of Co (III)Al2O3 catalytic behavior in Fischer–Tropsch synthesis. Journal of Natural Gas Chemistry, 20, 603–610.10.1016/S1003-9953(10)60240-X
- Wan, H. J., Wu, B. S., An, X., Li, T. Z., Tao, Z. C., Xiang, H. W., & Li, Y. W. (2007). Effect of A12O3 binder on the precipitated iron-based catalysts for Fischer–Tropsch Synthesis. Journal of Natural Gas Chemistry, 16, 130–138.10.1016/S1003-9953(07)60038-3
- Wang, Y. N., Xu, Y. Y., Li, Y. W., Zhao, Y. L., & Zhang, B. J. (2003). Heterogeneous modeling for fixed-bed Fischer–Tropsch synthesis: Reactor model and its applications. Chemical Engineering Science, 58, 867–875.10.1016/S0009-2509(02)00618-8
- Yang, J., Liu, Y., Chang, J., Wang, Y. N., Bai, L., Xu, Y. Y., Xiang, H. W., … Zhong, B. (2003). Detailed kinetics of Fischer–Tropsch synthesis on an industrial Fe–Mn catalyst. Industrial & Engineering Chemistry Research, 42, 5066–5090.10.1021/ie030135o