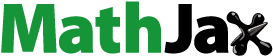
Abstract
The efficacy of assistive devices used during walking is often measured as a reduction in metabolic cost. Metabolic cost is typically assessed within a day or on multiple days, yet the benefit of performing within-day vs. between-day metabolic assessments is unknown. The purpose of this study was to determine the within-day minimal detectable change of standard measures of physiologic performance using a conventional portable metabolic system (K4b2 Cosmed, Rome, Italy), and compare these to between-day values. Twenty healthy adults completed two identical data collection sessions on separate days. In each session they performed three bouts of treadmill walking interspersed with three bouts of rest while oxygen consumption (), carbon dioxide production, and heart rate were measured. Intraclass correlation coefficients (ICC) and minimal detectable change values were calculated for non-resting within-day, as well as all between-day comparisons. All within-day measures were clinically reliable (ICC > 0.96), while between-day measures were generally less reliable (ICCs > 0.82). Within-day minimal detectable change values (walking heart rate = 4.9 bpm; gross
= 0.80 mL/kg/min; net
= 0.80 mL/kg/min; cost of transport = 0.022 J/Nm) were about half as large as between-day values. The results of this study suggest that, where possible, physiologic changes should be assessed within a single day of testing to maximize ability to detect small changes in performance.
Public Interest Statement
A key component of the design and prescription of medical devices that assist in walking is a thorough understanding of how patients will benefit from their use. Reducing the energy patients must expend to walk is often a primary goal of such devices. In order to accurately determine if this goal is being met, it is important for researchers to separate everyday changes in energy expenditure from changes caused by using the device. This work aims to provide a standard against which changes in energy expenditure within one day of testing can be measured. With this benchmark, researchers will have a better understanding of which assistive devices result in meaningful reductions in energy expenditure. This will allow for more rigorous assessments of assistive walking devices, ultimately providing physicians and patients with a more thorough understanding of their benefits.
1. Introduction
A reduction in metabolic cost is one of the primary goals of assistive devices used during walking such as prostheses (Herr & Grabowski, Citation2012; Houdijk, Pollmann, Groenewold, Wiggerts, & Polomski, Citation2009; Major, Twiste, Kenney, & Howard, Citation2014; Segal et al., Citation2012; Zelik et al., Citation2011), orthoses (Balaban et al., Citation2007; Menotti, Laudani, Damiani, Mignogna, & Macaluso, Citation2014; Menotti, Laudani, Damiani, Orlando, & Macaluso, Citation2014; Zissimopoulos, Fatone, & Gard, Citation2007), or exoskeletons (Malcolm, Derave, Galle, & De Clercq, Citation2013; Sawicki & Ferris, Citation2008, Citation2009). The efficacy of such devices is often tested within a single day (Balaban et al., Citation2007; Houdijk et al., Citation2009; Malcolm et al., Citation2013; Menotti, Laudani, Damiani, Mignogna, et al., Citation2014; Menotti, Laudani, Damiani, Orlando, et al., Citation2014; Segal et al., Citation2012; Zelik et al., Citation2011; Zissimopoulos et al., Citation2007) or across multiple days where acclimation to the device is required (Major et al., Citation2014; Sawicki & Ferris, Citation2008, Citation2009). Typical changes observed in energetic measures range from 3 to 8% for prostheses (Herr & Grabowski, Citation2012; Major et al., Citation2014; Segal et al., Citation2012; Zelik et al., Citation2011), 2 to 12% for orthoses (Balaban et al., Citation2007; Menotti, Laudani, Damiani, Orlando, et al., Citation2014; Zissimopoulos et al., Citation2007), or 7 to 15% for exoskeletons (Malcolm et al., Citation2013; Sawicki & Ferris, Citation2008, Citation2009).
Metabolic device evaluation studies have typically utilized portable metabolic units such as the Cosmed K4b2 system (Cosmed, Rome, IT). The between-day reliability and/or minimal detectable change (MDC) of several physiologic measures associated with the K4b2 system in various populations have been previously reported (Darter, Rodriguez, & Wilken, Citation2013; Stookey et al., Citation2013; Thomas, Buckon, Schwartz, Sussman, & Aiona, Citation2009; Welch, Strath, & Swartz, Citation2015). Although comparisons are often made within a single day of testing, the reliability and MDC of energetic measures collected within a single day during treadmill walking are not known. Given the benefit of collecting data on a single day where factors such as hydration and fitness are more controlled, we would expect the consistency of these measures to improve. Therefore, the purpose of this study was to calculate within-day MDCs for several physiological measurements, including heart rate, oxygen consumption, and cost of transport, using a K4b2 system, and compare these to between-day values. Establishing within-day MDCs provides a benchmark by which investigators can determine true changes in human performance and allow for more precise assessments of assistive devices used during walking.
2. Methods
2.1. Participants
Twenty healthy adults (9 females, 11 males) were recruited using flyers and an electronic community registry (UMClinicalStudies.org). Their mean (SD) age, height, weight and BMI were 30 (15) years, 1.74 (0.08) m, 72.4 (11.3) kg, and 23.8 (2.8) kg/m2, respectively (see supplemental material for additional details). All subjects provided written informed consent prior to participation in this institutionally approved study.
2.2. Experimental protocol
Participants performed two identical test sessions in the morning at least one day apart (14 (13) days). Participants were instructed to abstain from caffeine, avoid strenuous exercise, and fast for at least 4 h prior to testing.
A K4b2 portable metabolic system was used to measure the rate of oxygen consumption (, mL/kg/min), carbon dioxide production (
, mL/kg/min), and heart rate. This system consists of a mask, heart-rate monitor, and a collection unit that wirelessly transmits data to a laptop where it can be observed in real time (Figure (A)). First, participants were asked to remain seated and still for at least 5 min to obtain metabolic cost measures for seated rest. Then, they walked on a treadmill at a speed normalized to leg length and approximated a comfortable walking speed (average speed = 1.22 (0.03) m/s) (Gates, Wilken, Scott, Sinitski, & Dingwell, Citation2012). Participants walked for a minimum of 5 min, or until they reached steady-state, which was identified in real-time by visually observing a plateau in
(average time = 9.1 (1.98) min; Figure (B)). Once this plateau was reached, participants walked for an additional 3 min to obtain metabolic cost measures for steady-state walking. This process was then repeated such that all participants completed three bouts of walking which were interrupted by 5 min bouts of seated rest.
Figure 1. Experimental Setup: (A) Oxygen consumption and CO2 production were measured using a COSMED K4b2 system. (B) Oxygen consumption data for a representative subject over one day of testing
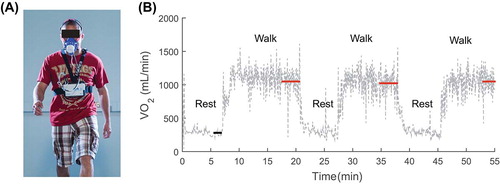
Steady-state walking intervals were later objectively determined using MATLAB (MathWorks, Natick, MA, USA, v.R2014b). Steady-state was defined as a 3 min interval wherein the minute average and
varied by <10%, and the average respiratory quotient
was between 0.7 and 1.0 and varied by <5% (McClave et al., Citation2003; Reeves, Davies, Bauer, & Battistutta, Citation2004). We began by assessing the last 3 min of walking. The interval was then iteratively shifted earlier by one breath until all steady-state criteria were met.
Performance measures included gross (mL/kg/min), net
(gross
− seated rest
: mL/kg/min), cost of transport (COT, J/Nm), and heart rate (HR, bpm). Seated rest
was calculated as the average
over the last 90 s of the first resting bout on each day and was included only in between-day analyses. Metabolic rate was estimated from
and
according to standard formulae (Brockway, Citation1987). COT, defined as the energy cost of moving 1 kg of body mass 1 m (J/N m), was calculated by dividing net metabolic rate by walking speed (Mian, Thom, Ardigo, Narici, & Minetti, Citation2006).
2.3. Statistical analysis
Intraclass correlation coefficients (ICCs) were calculated to assess reliability within- and between-days (Portney & Watkins, Citation2009). Specifically, we used an ICC3,k model for absolute agreement. Within-day ICCs were calculated for the first day of testing. Between-day ICCs were calculated from averages across bouts for each day. Only variables with a significant F-test (p < 0.05) had a sufficiently heterogeneous group for the ICC to be valid (Portney & Watkins, Citation2009). ICC > 0.90 indicated reasonable agreement for clinical measures, ICC > 0.75 indicated good agreement, while ICC < 0.75 indicated poor to moderate agreement (Portney & Watkins, Citation2009). Pooled SD and ICCs were used to estimate the standard error of the measure (SEM) according to the equation:(1)
(1)
where SDpooled is the pooled standard deviation across testing bouts or days.
Subsequently, minimal detectable change (MDC95) values were estimated for each variable according to the equation (Weir, Citation2005):(2)
(2)
where 1.96 corresponds to a 95% confidence level. MDCs are defined as the smallest change in a measure that exceeds random variation (Lassere, van der Heijde, & Johnson, Citation2001), and are often used to quantify the limits of expected variation in a measure. Bland-Altman plots comparing daily average values were used to identify proportional bias (Bland & Altman, Citation1999). Pearson’s correlations were performed to determine if testing separation, subject age, or subject weight were correlated with percent difference in performance measures across days for each subject. All statistical analyses were performed in SPSS version 22 (IBM Corp., Armonk, NY, USA).
3. Results
All 20 subjects were included in the analysis. Data from one subject (S08) was lost for one session on day two of testing due to equipment malfunction. F-tests were significant for all variables (p < 0.01), indicating sufficient heterogeneity. No proportional bias was observed in any measure (see Supplemental material).
All within-day and one between-day measure (walking HR) indicated clinically meaningful reliability (ICC > 0.96; Table ). Between-day measures had good reliability (ICC > 0.82), except for seated rest , which had poor reliability (ICC = 0.65).
Table 1. Physiologic measures between- and within-day for treadmill walking
The within-day MDCs were about half the magnitude of between-day MDCs (Table ). The MDC for walking heart rate exhibited the smallest comparative difference, with a within-day MDC of 4.9 bpm, which was about 30% less than its between-day MDC of 7.1 bpm (Table ).
The percent change across days for energetic measures were not significantly correlated with the days between testing (p ≥ 0.24), subject age (p ≥ 0.17), or subject weight (p ≥ 0.54) for any performance measure (Table ).
Table 2. Pearson’s correlations for energetic measures and subject details
4. Discussion
The purpose of this study was to determine within-day MDCs for various energetic measures of walking performance and compare these to between-day values. All within-day measures were deemed clinically reliable (ICC > 0.96). Between-day comparisons of physiologic measurements generally exhibited good agreement (ICC > 0.82), but were less reliable than within-session measures. Therefore, consistent with our expectations, the K4b2 system was able to reliably detect smaller changes in physiologic measures for comparisons made within a day than comparisons made across different testing days.
The results for between-day reliability were consistent with those reported previously on healthy adults using the K4b2 system (Darter et al., Citation2013) and another portable metabolic system (Blessinger et al., Citation2009). While seated rest and walking HR MDCs in the present study were 3–4 bpm lower than those previously reported (Darter et al., Citation2013), between-day oxygen consumption MDCs were roughly 0.86 and 0.61 mL/kg/min higher than previously reported values (Darter et al., Citation2013) for gross and net , respectively. This discrepancy may be the result of a larger separation between collection days in the current study. In the present study, testing days were separated by an average of two weeks, compared to five days of average separation in other studies (Blessinger et al., Citation2009; Darter et al., Citation2013). The longer between-day interval in the present study may have allowed for greater changes in fitness, body weight, stress levels, or other factors known to influence energetic measurements (Donahoo, Levine, & Melanson, Citation2004).
Consistent with prior studies (Darter et al., Citation2013; Thomas et al., Citation2009), resting was less reliable than walking
. Littlewood et al. (Citation2002) suggested that the K4b2 device is highly variable when acquiring resting energetic measurements, and may only be suitable for measuring the energetics of activity (Littlewood et al., Citation2002). When comparing the Cosmed system to the Deltatrac II, a metabolic cart specifically designed to measure resting energetics, Littlewood et al. (Citation2002) revealed a mean bias for REE and VO2 of 268 ± 702 kcal/day and 51.6 ± 125.6 mL/min, respectively. The exact reason for this discrepancy is not well understood, as its design is similar to devices that have been reported to produce consistently accurate resting measures (Littlewood et al., Citation2002). However, supine rest, longer and stricter fasting periods, and resting prior to data collection have been shown to improve the between and within-day reliability of resting measures obtained with the Cosmed K4b2 system (Welch et al., Citation2015). These stricter resting protocols should be used if resting measures are of particular importance to a study.
There are several limitations to this study. As mentioned above, the protocol may have limited our ability to determine accurate energetic measures for seated rest. To obtain more reliable measures we could have had participants rest for longer periods of time. This may have improved the reliability for both seated rest and net VO2. Second, although participants were asked to abstain from exercise and to fast and avoid caffeine, we have no way of determining whether they actually did these things. Failure to adhere to these guidelines could cause increased variability from day-to-day. This would be true for any study unless participants were observed over extended periods of time in the laboratory. Third, there was a wide variation in subject characteristics and range of time between testing sessions. Testing days were separated by an average of two weeks and the age range of subjects tested was large (19–65 years). These factors were not correlated with changes in any measure across days, however. Although this may increase the variability of the data, testing a wide variety of subjects with varying lengths of testing separation increases the generalizability of the results.
In conclusion, the K4b2 system is able to detect small changes in physiologic measurements within a day. Within-day MDCs were about half the size of between-day values. These results emphasize the benefit of using within-day methodological designs when feasible as the ability to determine differences in metabolic cost dramatically improves.
Funding
This work was supported by the National Center for Advancing Translational Sciences of the National Institutes of Health [grant number 2UL1TR000433]. Deanna H. Gates is supported by the Eunice Kennedy Shriver National Institute of Child Health & Human Development of the National Institutes of Health [grant number K12HD073945].
Declaration
The content is solely the responsibility of the authors and does not necessarily represent the official views of the National Institutes of Health.
Supplemental_Material.docx
Download MS Word (595 KB)Additional information
Notes on contributors
Audra Davidson
Audra Davidson is a researcher and Dr Emily S. Gardinier is a postdoctoral fellow in The Rehabilitation Biomechanics Laboratory, run by Dr Deanna H. Gates. In the Rehabilitation Biomechanics Laboratory, we focus on the study of repetitive human movements such as walking and reaching. Throughout these studies, we aim to determine which aspects of movement a person actively controls and how this function can be modeled most effectively. Along with governing control strategies, these models can be used to design both passive and active devices, which can mimic biological function and restore or improve function in individuals with disability. Another focus of our research is determining appropriate outcomes to measure performance with new technology. Without such guidelines, it is difficult to assess the efficacy of assistive devices. The following work aids in this endeavor, providing additional tools for the evaluation of assistive devices.
References
- Balaban, B. R. , Yasar, E. , Dal, U. , Yazi˙ci˙oglu, K. L. , Mohur, H. , & Kalyon, T. A. (2007). The effect of hinged ankle-foot orthosis on gait and energy expenditure in spastic hemiplegic cerebral palsy. Disability and Rehabilitation , 29 , 139–144.10.1080/17483100600876740
- Bland, J. M. , & Altman, D. G. (1999). Measuring agreement in method comparison studies. Statistical Methods in Medical Research , 8 , 135–160.10.1191/096228099673819272
- Blessinger, J. , Sawyer, B. , Davis, C. , Irving, B. , Weltman, A. , & Gaesser, G. (2009). Reliability of the VmaxST portable metabolic measurement system. International Journal of Sports Medicine , 30 , 22–26.10.1055/s-2008-1038744
- Brockway, J. (1987). Derivation of formulae used to calculate energy expenditure in man. Human Nutrition. Clinical Nutrition , 41 , 463–471.
- Darter, B. J. , Rodriguez, K. M. , & Wilken, J. M. (2013). Test–retest reliability and minimum detectable change using the K4b 2: Oxygen consumption, gait efficiency, and heart rate for healthy adults during submaximal walking. Research Quarterly for Exercise and Sport , 84 , 223–231.10.1080/02701367.2013.784720
- Donahoo, W. T. , Levine, J. A. , & Melanson, E. L. (2004). Variability in energy expenditure and its components. Current Opinion in Clinical Nutrition & Metabolic Care , 7 , 599–605.10.1097/00075197-200411000-00003
- Gates, D. H. , Wilken, J. M. , Scott, S. J. , Sinitski, E. H. , & Dingwell, J. B. (2012). Kinematic strategies for walking across a destabilizing rock surface. Gait Posture , 35 , 36–42. doi:10.1016/j.gaitpost.2011.08.001
- Herr, H. M. , & Grabowski, A. M. (2012). Bionic ankle–foot prosthesis normalizes walking gait for persons with leg amputation. Proceedings of the Royal Society B: Biological Sciences , 279 , 457–464.10.1098/rspb.2011.1194
- Houdijk, H. , Pollmann, E. , Groenewold, M. , Wiggerts, H. , & Polomski, W. (2009). The energy cost for the step-to-step transition in amputee walking. Gait & Posture , 30 , 35–40.10.1016/j.gaitpost.2009.02.009
- Lassere, M. , van der Heijde, D. , & Johnson, K. R. (2001). Foundations of the minimal clinically important difference for imaging. The Journal of Rheumatology , 28 , 890–891.
- Littlewood, R. , White, M. , Bell, K. , Davies, P. , Cleghorn, G. , & Grote, R. (2002). Comparison of the cosmed K4 b2 and the deltatrac IITM metabolic cart in measuring resting energy expenditure in adults. Clinical Nutrition , 21 , 491–497.10.1054/clnu.2002.0580
- Major, M. J. , Twiste, M. , Kenney, L. P. , & Howard, D. (2014). The effects of prosthetic ankle stiffness on ankle and knee kinematics, prosthetic limb loading, and net metabolic cost of trans-tibial amputee gait. Clinical Biomechanics , 29 , 98–104.10.1016/j.clinbiomech.2013.10.012
- Malcolm, P. , Derave, W. , Galle, S. , & De Clercq, D. (2013). A simple exoskeleton that assists plantarflexion can reduce the metabolic cost of human walking. PLoS ONE , 8 , e56137.10.1371/journal.pone.0056137
- McClave, S. A. , Spain, D. A. , Skolnick, J. L. , Lowen, C. C. , Kleber, M. J. , Wickerham, P. S. , ... Looney, S. W. (2003). Achievement of steady state optimizes results when performing indirect calorimetry. Journal of Parenteral and Enteral Nutrition , 27 , 16–20.10.1177/014860710302700116
- Menotti, F. , Laudani, L. , Damiani, A. , Mignogna, T. , & Macaluso, A. (2014). An anterior ankle-foot orthosis improves walking economy in Charcot–Marie–Tooth type 1A patients. Prosthetics and Orthotics International , 38 , 387–392.10.1177/0309364613506250
- Menotti, F. , Laudani, L. , Damiani, A. , Orlando, P. , & Macaluso, A. (2014). Comparison of walking energy cost between an anterior and a posterior ankle-foot orthosis in people with foot drop. Journal of Rehabilitation Medicine , 46 , 768–772.10.2340/16501977-1837
- Mian, O. S. , Thom, J. M. , Ardigo, L. P. , Narici, M. V. , & Minetti, A. E. (2006). Metabolic cost, mechanical work, and efficiency during walking in young and older men. Acta Physiologica , 186 , 127–139.10.1111/aps.2006.186.issue-2
- Portney, L. G. , & Watkins, M. P. (2009). Foundations of clinical research: Applications to clinical practice (3rd ed.). Philadelphia, PA: Prentice Hall.
- Reeves, M. M. , Davies, P. S. , Bauer, J. , & Battistutta, D. (2004). Reducing the time period of steady state does not affect the accuracy of energy expenditure measurements by indirect calorimetry. Journal of Applied Physiology , 97 , 130–134.10.1152/japplphysiol.01212.2003
- Sawicki, G. S. , & Ferris, D. P. (2008). Mechanics and energetics of level walking with powered ankle exoskeletons. Journal of Experimental Biology , 211 , 1402–1413.10.1242/jeb.009241
- Sawicki, G. S. , & Ferris, D. P. (2009). Powered ankle exoskeletons reveal the metabolic cost of plantar flexor mechanical work during walking with longer steps at constant step frequency. Journal of Experimental Biology , 212 , 21–31.10.1242/jeb.017269
- Segal, A. D. , Zelik, K. E. , Klute, G. K. , Morgenroth, D. C. , Hahn, M. E. , Orendurff, M. S. , ... Czerniecki, J. M. (2012). The effects of a controlled energy storage and return prototype prosthetic foot on transtibial amputee ambulation. Human Movement Science , 31 , 918–931. doi:10.1016/j.humov.2011.08.005
- Stookey, A. D. , McCusker, M. G. , Sorkin, J. D. , Katzel, L. I. , Shaughnessy, M. , Macko, R. F. , & Ivey, F. M. (2013). Test-retest reliability of portable metabolic monitoring after disabling stroke. Neurorehabilitation and Neural Repair , 27 , 872–877. doi:10.1177/1545968313497103
- Thomas, S. S. , Buckon, C. E. , Schwartz, M. H. , Sussman, M. D. , & Aiona, M. D. (2009). Walking energy expenditure in able-bodied individuals: A comparison of common measures of energy efficiency. Gait & Posture , 29 (4), 592–596.10.1016/j.gaitpost.2009.01.002
- Weir, J. P. (2005). Quantifying test-retest reliability using the intraclass correlation coefficient and the SEM. The Journal of Strength & Conditioning Research , 19 , 231–240.
- Welch, W. , Strath, S. , & Swartz, A. (2015). Congruent validity and reliability of two metabolic systems to measure resting metabolic rate. International Journal of Sports Medicine , 36 , 414–418.
- Zelik, K. E. , Collins, S. H. , Adamczyk, P. G. , Segal, A. D. , Klute, G. K. , Morgenroth, D. C. , & Kuo, A. D. (2011). Systematic variation of prosthetic foot spring affects center-of-mass mechanics and metabolic cost during walking. IEEE Transactions on Neural Systems and Rehabilitation Engineering , 19 , 411–419.10.1109/TNSRE.2011.2159018
- Zissimopoulos, A. , Fatone, S. , & Gard, S. A. (2007). Biomechanical and energetic effects of a stance-control orthotic knee joint. The Journal of Rehabilitation Research and Development , 44 , 503.10.1682/JRRD.2006.09.0124