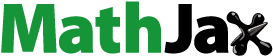
Abstract
In service and production area, problems such as severity of competition and increasing customers’ expectations, put the producers’ under a commitment to eliminate product defects and make up for any kind of shortage and deviations in its performance. In order to reach the mentioned aim, failure mode and effects analysis (FMEA) technique can be typically utilized for prevention, identification, control and elimination of possible errors. In this paper, FMEA approach is adopted to design and implement a system of quality control in the apparel production lines, to identify and rank the possible difficulties, and then finally, to issue the right commands to the quality control stations. For this purpose, the data are gathered from a medium-sized apparel factory and implemented by FMEA and then, improvability of production efficiency is analyzed using simulation-based optimization technique. The results of the simulation show significant decrease in product defects, rework, and total production cost.
Public Interest Statement
In order to meet customers’ expectations and to solve some of the most significant production and service problems, this study delivers practical solution by means of statistical optimization methods. The results show significant decrease in product defects, rework, total production cost, and finally caused better customer servicing.
Design and process failure mode and effects analysis (FMEA) is the selected methodology which has been applied to analyze the practical collected data. Design FMEA explores the possibility of product malfunctions, reduced product life, and safety and regulatory concerns. Process FMEA discovers failure that impacts product quality, reduced reliability of the process, customer dissatisfaction, and safety or environmental hazards.
1. Introduction
In the age of ever-growing global competition, production efficiency is one of the most important issues in factory management. Factories no longer can afford to incur delays, failures and cancellations. One of the most important problems in the production process is defect creation in labour stations and as a result, occurring delays in the production program. Hence, there has been an increase in using scientific approaches to recognize failure sources and prevent failures and defects from occurring in the production process. In the other words, the first step to control and eliminate such operational problems is to start up a production system and quality control system in a standard way based on scientific and experimental findings (Case, Nor, & Teoh, Citation2010; Sheng-Hsien & Shin-Yann, Citation1996). Those problems may occur due to inefficient management of production resources. Thus, management of resources, including human resources have become a fundamental issue in improving production control process; nonetheless, common tools and techniques of human resource management are not prevalent in apparel production lines (Dadashian, Monfared, & Nasrabadi, Citation2009; Liang-Hsuan & Wen-Chang, Citation2007; Stamatis, Citation2003). Therefore it is of great importance to design and utilize a system, capable of elaborate monitoring of operators work schedule and examining each station with regard to its properties each industry, dependent upon its own requirements is able to take advantage of these techniques. These techniques have their merits and demerits (Dadashian et al., Citation2009; Yuen, Fung, Wong, Hau, & Chan, Citation2008). Consequently, using these techniques will also play an important part in optimizing production processes. Failure modes and effects analysis (FMEA) is one of the most effective tools to systematically identify activities that can decrease or remove the chance of possible error occurrence managing implementation and documentation of these activities, and also is a technique to identify, define and, eliminate possible or definite failures, errors, and existing problems in the system, design, service and process, prior to end-use (Lipol & Haq, Citation2011). FMEA is one of the first steps of a system reliability study that involves reviewing as many components, assemblies, and subsystems as possible to identify failure modes, and their causes and effects. Also, all of factors such as equipment, environment, materials, and human are to be taken into account. To collect accurate and thorough data on the project, techniques such as interviews with informed and proficient people in workshops and organization were used (Bahrami, Bazzaz, & Sajjadi, Citation2012). After that, a list of errors, their causes and plausible mechanisms was supplied and the causes must be known. In order to assess risks more accurately, adequate attention should be paid to documents, requirements, operational standards, the workplace and the conditions of working. Commonly satisfactory level of possibility changes for each organization depends upon the financial and economic resources, technological constraints, human resource factors and management of making decisions (Ahire & Relkar, Citation2012). In this study the FMEA was implemented and accompanied by quality control of apparel production lines, to decrease the defective products and enhance the productivity. As a consequence, using Arena simulation software, enhancement programs and defensive actions can be taken prior to starting the project, through utilizing FMEA to avoid wasting resources and decreasing cost.
2. Theory
In1950s, procedures for conducting FMEA were described in US Armed Forces Military Procedures document, which was almost the elementary definition of that. In 1970s, NASA was the first organization which proposed FMEA as a means for their apparent reliability requirements.
Ever since, it has widely served as a powerful approach for systematic analysis of products and processes in different industries. In that decade the mentioned system was utilized in nuclear establishments and also in the automotive industry, including Ford Motor Company and Peugeot-Citroen Company (Bahrami et al., Citation2012).
In the subsequent decades use of this system was broadened to the point where, nowadays, this technique is applicable for more of the organizations. FMEA is straightforward to use and powerful engineering quality approach that assists to identify activities which can diminish or eliminate the chance of possible error during the design or process (Bahrami et al., Citation2012; Carbone & Tippett, Citation2004; McDermott, Mikulak, & Beauregard, Citation2009). Moreover, this method can decrease disastrous errors causing damage to the industries.
At first glance, it might seem that by this method, such problems will be solved temporarily. But the main philosophy is to change system behavior in same experiences when the company encounters operational problems. Entire accomplishment of this method strongly depends on its structural executing members. Institutionalization the executing of this method in a company, in long-term, will improve the whole system behavior by production optimization and controlling system improvement.
There have been some applications of FMEA on spinning and weaving industries; but this powerful technique has not been extensively used in apparel industries (De Toni & Meneghetti, Citation2000; Özyazgan & Engin, Citation2013; Walser, Citation2006). Therefore, this research aims at examining the applicability of FMEA on apparel production.
3. FMEA system explanation
In the mentioned system, each factor is examined to recognize possible failures. Therefore, three measures including the probability of failure occurrence (O), the severity or impact of the failure (S), and the capability of detection of failure occurrence (D) which will be clarified below, are taken into account. The multiplication of these measures leads to identification of the risk priority number (RPN) (Snooke & Price, Citation2012; Varzakas, Citation2011).
3.1. Probability of failure occurrence (O)
This factor determines the possibility of occurrence of a potential cause or mechanism of failure. In other words, the probability of occurrence specifies that a potential error happens with a specified frequency.
The probability of occurrence is evaluated on the basis of a range of 1–10. Now it is essential to examine the cause of a failure mode and its probability of occurrence. All the possible causes for a failure mode should be identified and documented. Examples of causes can be human faults, manufacturing errors, and so on. Therefore, only through the elimination or reduction of the causes or mechanisms of each failure, it would still be possible to reduce the number of different failures, possible values of which are shown in Table .
Table 1. Occurrence scale
3.2. Severity of failure (S)
Severity is the intensity of the effect of failure on the product or end-user’s experience thereof. Severity of failures is expressed on a scale from 1 to 10, ranging from “none” to “dangerously high” shown in Table .
Table 2. Severity scale
3.3. Detectability of failure (D)
This factor shows the probability of detection of a fault by the operator or end-user.
It should be clarified how the failure mode or cause can be detected by an operator under normal circumstances or if it can be detected by the maintenance team by some inspection or other types of actions, which is shown in Table .
Table 3. Detection certainty scale
Also, potential risk scores should be evaluated after ranking and making sure that the rating is still unchanged.
3.4. RPN evaluation
After achieving Severity (S), Occurrence (O) and Detect (D), these values should be multiplied, to calculate the RPN number. Indeed, Equation (1) shows the RPN number calculation:(1)
(1)
RPN value lies in a range of 1 and 1,000 and failures will be ranked with regard to their numbers. Failures of high risk priority are preferred to be examined more carefully, and operators should concentrate on errors owning a higher RPN value. RPN is a measure for separating tolerable and intolerable risks in the mentioned system.
In fact, the higher RPN value of a specific failure, the more unacceptable the failure (Almannai, Greenough, & Kay, Citation2008; Bahrami et al., Citation2012; Chiozza & Ponzetti, Citation2009; McDermott et al., Citation2009).
In general, regardless of the RPN value, special attention should be paid to suitability of present design control actions. For prevention of errors, precautionary or corrective actions should be taken by removal, decrease or control of their causes. After ranking errors and determining RPN, some solutions may be found to decrease or eliminate specified errors. It is worth mentioning that for the above RPN values the related team should take the appropriate action to decrease them. For example, for RPN values greater than 120, 30% per hour, RPN between 120 and 60, 12% per hour and for RPN less than 60, 7% in each work shift should be controlled.
It must be considered that the mentioned percentage of applied quality control may be varied by some independent parameters; such as production plan, type of product and etc.
Corrective actions including removal of the basic cause of risk, reduction of the error severity, heightening job satisfaction and increase in the likelihood of identification in the process should be taken (Geum, Shin, & Park, Citation2011; Ravi Sankar & Prabhu, Citation2001; Seung & Kosuke, Citation2003; Zhou & Stalhane, Citation2004).
These corrective actions should be followed by appropriate follow-up measures as mentioned above. Approaches like examination of the processes, plans and designs are used to improve the process and enhance efficiency.
It should be noted that if the administrative team has successfully completed all the last steps, the main job of this team will commence.
In other words, if the mentioned team cannot take appropriate actions to remove or decrease these cases, no good results will be obtained, and also lots of financial expenses will be imposed (Mariajayaprakash & Senthilvelan, Citation2013; McDermott et al., Citation2009).
With regard to the above statements, the precise mechanism of the FMEA method can be demonstrated as Figure .
For performing this approach, required data should be gathered by qualified engineers who are familiar with the production process and by experts who are the most knowledgeable about the process and product (McDermott et al., Citation2009; Sheng-Hsien & Shin-Yann, Citation1996).
The merit of this working manner is that all of the stations would be unaware about each action defined. There has been a significant lack of research regarding utilization of the FMEA system in apparel production lines.
The chief aim of this paper is to implement the FMEA system on the quality control of apparel production and evaluate the efficiency of the aimed system.
4. Objectives and contribution
This work has probed the effect of application of FMEA to the reduction of the flawed products, and thus, reduction of expenses and increase in the productivity of the apparel production line. In order to show the suggested problem, a method based on simulation optimization is applied for modeling the new quality control plan on the basis of FMEA system. A case study for T-shirt sewing section in an apparel production line will be implemented, and the consequences will be examined.
The suggested model will be explored by example using a genuine data representing quality control plan of apparel production.
5. Simulation modeling and optimization methodology
Computer simulation is a great technique for assessing complicated systems. These assessments are commonly used in forming the answers to questions of “what if” (Alibi, Fayala, Bhouri, Jemni, & Zeng, Citation2013; April, Glover, Kelly, & Laguna, Citation2003; Shokohyar & Mansour, Citation2013).
A simulation Experiment can be clarified as an examination or a series of examinations. Significant changes are made in the model of simulation input variables for observing and identifying the reasons for variations of the output variables.
When the experiment cost of the designed system is high and the simulation model is complicated, the simulation experiments become excessively costly (Blaga & Draghici, Citation2005; Carson & Maria, Citation1997; Kara, Rugrungruang, & Kaebernick, Citation2007; Saaty, Citation1980; Korytkowski, Wiśniewski, & Rymaszewski, Citation2013). In order to do this, a technique called simulation-based optimization has appeared for optimization of the simulation models. Simulation optimization can be clarified as finding process of the best input variables from all the possibilities with no evaluation of each one (Melouk, Freeman, Miller, & Dunning, Citation2013; Nguyen, Reiter, & Rigo, Citation2014; Shokohyar, Mansour, & Karimi, Citation2013).
In order to fulfil these purposes Arena simulation software was utilized in our work. Mentioned approach is employed to optimize the settled simulation model. Opt-Quest software is a widely- used applications for optimization of a simulation model.
This software combines meta-heuristics approach such as Tabu search, neural networks, and scatter search algorithm into simulation model and optimizes the blend of it. The optimization procedure in Opt-Quest software is demonstrated in Figure .
As demonstrated in this figure, simulation optimization is utilized to find the optimal settings of the input variables that optimize the objective function. Subsequent to each run of the simulation model, the objective function is calculated on the basis of simulation results, and new input parameters (on the basis of the above meta-heuristics approach) are set in the simulation model.
This process goes on until the predefined numbers of simulation runs are performed (Melouk et al., Citation2013; Shokohyar & Mansour, Citation2013; Shokohyar et al., Citation2013).
The developed simulation-based optimization model is applied to the FMEA system for determining the optimum quality control plan and eventually optimizing the number of controls, leads to the reduction in failure product rate, is built to determine the optimal solution to decrease the flawed products. The human resource restriction has been considered in the model of optimization process designed and the optimal quality control plan with higher productivity that finally leads to cost reduction are determined.
6. Case study
For validating the mentioned technique, a factory of apparel production was aimed. The main properties of the selected factory are demonstrated in Table . For implementation of the system, the sewing process of the one specific kind of apparel (T-shirt) was examined. The daily level of production is roughly 350 in unit number of apparel.
Table 4. Selected factory main properties
Seven operators in Seven stations are involved in the sewing of the product and one person is responsible for quality control of these stations.
In the current condition, this inspector would evenly control %12 of production of all stations, regardless of the flawed product level in each station. Using this quality control system, the percentage of final flawed product is 13%.
Subsequent to FMEA system implementation, quality inspector, will control each station, with regard to the RPN number.
Therefore, the more the number of defects, the more samples will be controlled in each station. The RPN number for each station is shown in Table .
Table 5. RPN value for each station
In order to compare the system efficiency before and after implementation of the FMEA system, sewing section was simulated using Arena simulation software.
The schematic picture of the sewing section that was simulated with the mentioned software is shown in Figure . This section consists of 7 stations. In first station the pocket part is joined to front part; in the second one, front and back parts is batch with each other and then finished sewing on the pocket, shoulder and collar sewing and the other finishing sewing is done in the subsequent stations. Finally, the last quality inspection is performed on the products and defected items are rejected.
In each station a number of products are possibly produced in a defective form and then an equal percentage of the each station’s product is randomly controlled and the defective products are reformed. In Figure one of the stations is demonstrated as a sample. As observed in this station, first, the front and back parts are joined to each other; then their quality is inspected and the defective items are repaired. At the end, the total products are transferred to the next station.
For each station, the details of RPN value are mentioned as below values;
7. Results and discussions
Considering the fact that most of defects in the process of apparel production happen in sewing stage, if it were possible to enhance productivity by any decrease in the number of flawed products, a remarkable cost reduction will occur.
In this in an attempt to optimize the inspection activities of the operator, in order to enhance the chance of finding the flawed product in each station, and eventually to decrease frequency of flaws in products. To fulfill this aim, the control percentage dedicated to each station and the total defective product percentage are defined as a variable and objective function in the optimization toolbox of the Arena simulation software (Opt-Quest). It should be noted that the lower and upper limits of variables are 5 and 30% and their summation should be lower than 84%. The procedure of the simulation optimization searches for an optimum solution with regard to specific objectives. This process is repeated for defined number of simulation runs upon which results are dependent.
In our method optimal solution for the developed model was run 600 times, with every run being composed of 2 replications, as shown in Figure . The results of the optimization process are shown in Figure .
According to Figure , the optimum controlled percentage for each station and the optimum total rejected products percentage are obtained. The comparison between these values and their primary values is illustrated in Figures and . As illustrated in the Figure , the control percentages that were equal in the primary condition are calculated with respect to RPN values in the optimized condition (mentioned in Table ). It is observed that for higher
Figure 8. Comparison between percent of accepted and rejected products, before and after optimization.
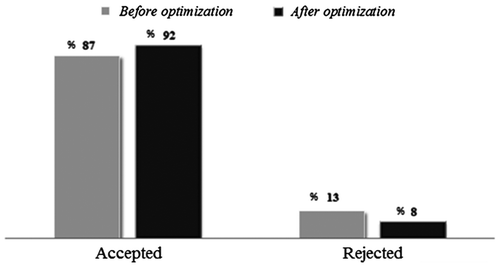
RPN Values, more control percentages were dedicated. As a result, the quality control operator would be able apportion his time more efficiently among the stations, and thus, improve resource allocation. In Figure , the percentage of the rejected product has diminished by almost 5%; consequently, this amount of reduction, cause the decreasing in total cost.
8. Conclusion
FMEA is simulated on an apparel production line, to examine improvability of efficiency. Simulation-based optimization technique is adopted and implemented using the Arena Simulation Software.
Final results reveal that FMEA can significantly improve allocation of inspection work to stations, based on the performance of each station, and thus result in a meaningful decrease in the number of defects and the amount of rework.
The other merits of this system can be quality improvement, enhancement of the certainty degree and security of the product, making the production process faster, decreasing the cost related to the defected products, and finally the improvement of customers’ image of organization and increase in its competitive advantage in the market.
It should be noted that this method is not “once for all” and should always be regarded as a dynamic process. It means that, RPN ranks must be revised and remeasured periodically to avoid increasing defects.
Acknowledgements
The researchers are grateful for diligent contributions and cooperation of the management and personnel of JPA Company on production data of which the research was mainly based.
Additional information
Funding
Notes on contributors
E. Pazireh
E. Pazireh educated in textile engineering, textile management at BSc and MSc levels from Polytechnic of Iran. He has published papers in scientific journals and conference proceedings. His research interests is research, development and production of new products, especially in textile and clothing industry.
A.H. Sadeghi
A.H. Sadeghi Educated in Textile engineering, Industrial management and engineering at BSc and MSc levels and obtained his PhD in textile engineering from Polytechnic of Iran. He’s assistant professor at Textile department of Amirkabir University of Technology. He has published several papers in scientific journals and conference proceedings. His research interests is quality and productivity management and control, especially in textile industry.
S. Shokohyar
S. Shokouhyar has been an Assistant Professor at the Department of Management and Accounting in Shahid Beheshti University in Iran. He received his PhD in Industrial Engineering from Polytechnic of Iran. He received his MSc and BSc in Industrial Engineering from Polytechnic of Iran. His research interests include data mining, soft computing, supply chain management and organizational issues.
References
- Ahire, C. P., & Relkar, A. S. (2012). Correlating failure mode effect analysis (FMEA) & overall equipment effectiveness (OEE). Procedia Engineering, 38, 3482–3486.10.1016/j.proeng.2012.06.402
- Alibi, H., Fayala, F., Bhouri, N., Jemni, A., & Zeng, X. (2013). An optimal artificial neural network system for designing knit stretch fabrics. Journal of the Textile Institute, 104, 766–783.10.1080/00405000.2012.756134
- Almannai, B., Greenough, R., & Kay, J. (2008). A decision support tool based on QFD and FMEA for the selection of manufacturing automation technologies. Robotics and Computer-Integrated Manufacturing, 24, 501–507.10.1016/j.rcim.2007.07.002
- April, J., Glover, F., Kelly, J., & Laguna, M. (2003). Practical introduction to simulation optimization. In Proceedings of the 2003 winter simulation conference (pp. 71–78). New Orleans, LA: IEEE.10.1109/WSC.2003.1261410
- Bahrami, M., Bazzaz, D. H., & Sajjadi, S. Mojtaba (2012). Innovation and improvements in project implementation and management; using FMEA technique. Procedia - Social and Behavioral Sciences, 41, 418–425.10.1016/j.sbspro.2012.04.050
- Blaga, M., & Draghici, M. (2005). Application of genetic algorithms in knitting technology. Journal of the Textile Institute, 96, 175–178.10.1533/joti.2004.0064
- Carbone, T. A., & Tippett, D. D. (2004). Project risk management using the project risk FMEA. Engineering Management Journal, 16, 28–35.10.1080/10429247.2004.11415263
- Carson, Y., & Maria, A. (1997). Simulation optimization: Methods and applications. In Proceedings of the 1997 winter simulation conference. Atlanta, GA.
- Case, K., Nor, A., & Teoh, P. C. (2010). A diagnostic service tool using FMEA. International Journal of Computer Integrated Manufacturing, 23, 640–654.10.1080/0951192X.2010.486007
- Chiozza, M. L., & Ponzetti, C. (2009). FMEA: A model for reducing medical errors. Clinica Chimica Acta, 404, 75–78.10.1016/j.cca.2009.03.015
- Dadashian, F., Monfared, M. A. S., & Nasrabadi, H. G. (2009). Design of a new quality assessment system for textile products. Journal of the Textile Institute, 100, 640–648.10.1080/00405000802131160
- De Toni, A., & Meneghetti, A. (2000). The production planning process for a network of firms in the textile-apparel industry. International Journal of Production Economics, 65, 17–32.10.1016/S0925-5273(99)00087-0
- Geum, Y., Shin, J., & Park, Y. (2011). FMEA-based portfolio approach to service productivity improvement. The Service Industries Journal, 31, 1825–1847.10.1080/02642069.2010.503876
- Kara, S., Rugrungruang, F., & Kaebernick, H. (2007). Simulation modelling of reverse logistics networks. International Journal of Production Economics, 106, 61–69.10.1016/j.ijpe.2006.04.009
- Korytkowski, P., Wiśniewski, T., & Rymaszewski, S. (2013). An evolutionary simulation-based optimization approach for dispatching scheduling. Simulation Modelling Practice and Theory, 35, 69–85.10.1016/j.simpat.2013.03.006
- Liang-Hsuan, C., & Wen-Chang, K. (2007). Fuzzy linear programming models for new product design using QFD with FMEA. Applied Mathematical Modelling, 33, 633–647.
- Lipol, L. S., & Haq, J. (2011). Risk analysis method: FMEA/FMECA in the organizations. International Journal of Basic & Applied Sciences, 11, 74–82.
- Mariajayaprakash, A., & Senthilvelan, T. (2013). Failure detection and optimization of sugar mill boiler using FMEA and Taguchi method. Engineering Failure Analysis, 30, 17–26.10.1016/j.engfailanal.2012.12.010
- McDermott, R., Mikulak, R., & Beauregard, M. (2009). The basics of FMEA. Boca Raton, FL: Taylor and Francis Group.
- Melouk, S. H., Freeman, N. K., Miller, D., & Dunning, M. (2013). Simulation optimization-based decision support tool for steel manufacturing. International Journal of Production Economics, 141, 269–276.10.1016/j.ijpe.2012.08.001
- Nguyen, A. T., Reiter, S., & Rigo, P. (2014). A review on simulation-based optimization methods applied to building performance analysis. Applied Energy, 113, 1043–1058.10.1016/j.apenergy.2013.08.061
- Özyazgan, V., & Engin, F. Z. (2013). FMEA analysis and applications in knitting industry. Tekstil ve Konfeksiyon, 23, 228–232.
- Saaty, T. L. (1980). The analytical hierarchy process, planning, priority setting, resource allocation. New York, NY: McGraw-Hill.
- Ravi Sankar, N., & Prabhu, B. S. (2001). Modified approach for prioritization of failures in a system failure mode and effects analysis. International Journal of Quality & Reliability Management, 18, 324–336.10.1108/02656710110383737
- Seung, R., & Kosuke, I. (2003). Using cost based FMEA to enhance reliability and serviceability. Advanced Engineering Informatics, 17, 179–188.
- Sheng-Hsien, T., & Shin-Yann, H. (1996). Failure mode and effects analysis: An integrated approach for product design and process control. International Journal of Quality & Reliability Management, 13, 8–26.
- Shokohyar, S., & Mansour, S. (2013). Simulation-based optimisation of a sustainable recovery network for waste from electrical and electronic equipment (WEEE). International Journal of Computer Integrated Manufacturing, 26, 487–503.10.1080/0951192X.2012.731613
- Shokohyar, S., Mansour, S., & Karimi, B. (2013). Simulation-based optimization of ecological leasing: A step toward extended producer responsibility (EPR). The International Journal of Advanced Manufacturing Technology, 66, 159–169.10.1007/s00170-012-4315-9
- Snooke, N., & Price, C. (2012). Automated FMEA based diagnostic symptom generation. Advanced Engineering Informatics, 26, 870–888.10.1016/j.aei.2012.07.001
- Stamatis, D. H. (2003). Failure mode and effect analysis: FMEA from theory to execution. Milwaukee, WI: ASQ Quality Press.
- Varzakas, T. H. (2011). Application of ISO22000, failure mode, and effect analysis (FMEA) cause and effect diagrams and pareto in conjunction with HACCP and risk assessment for processing of pastry products. Critical Reviews in Food Science and Nutrition, 51, 762–782.10.1080/10408391003799970
- Walser, I. (2006). Applying FMEA in the knitting process.
- Yuen, C. W. M., Fung, E. H. K., Wong, W. K., Hau, L. C., & Chan, L. K. (2008). Application of smart system to textile industry: Preliminary design of a smart hanger for garment inspection. Journal of the Textile Institute, 99, 569–580.10.1080/00405000701660160
- Zhou, J., & Stalhane, T. (2004). Using FMEA for early robustness analysis of Web-based systems. In Proceedings of the 28th Annual International Computer Software and Applications Conference (Vol. 2, pp. 28–29). Washington, DC: IEEE.