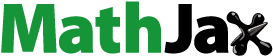
Abstract
Logistics distribution in the event of natural disasters, such as volcanic eruptions, earthquakes and floods, is an important matter that needs attention of both government and society. The distribution of logistics in many disaster-prone countries, including Indonesia, is poorly planned and managed. Therefore, various problems arise, such as low efficiency and effectiveness of delivery and poor cost-effective flow. This study developed location-allocation models for distribution and evacuation of refugees and logistics. The investigation was conducted by determining the characteristics of the disaster management system of volcanic eruption in Mount Merapi, Indonesia. The mathematical model was built and a set of solution procedure was proposed to solve the problem. The objective of the model is to minimize total costs of the system and determine the optimal solutions at the three alert-levels, which are alert phase, danger phase and post-disaster phase. The results were discussed and models were analyzed to give several managerial insights regarding the model’s optimal solution. Based on the results, the proposed models are proven to give more effective solutions at lower costs. The usage of each evacuation shelter can be utilized to its maximum level. Therefore, it allows minimum waste of capacity as well as costs related to evacuation and distribution.
Public Interest Statement
Merapi volcanic eruption belongs to be the third largest disaster in Indonesia. Several problems occurred for the result of poor disaster management planning. Location-allocation of logistics and refugees were several issues that gave major impact to the related activity. This research suggested new models to get the optimal decisions of the location-allocation problem. Within this research, the optimal route of logistics distribution and refugees evacuation were obtained. Moreover, this research output also included the optimal number of logistics and refugees that should be allocated for each chosen route. The proposed models helped a local government as well as The Indonesian National Board for Disaster Management to conduct a better disaster management, particularly in the case of volcanic eruption. By implementing the proposed models, the needs of refugee can be fulfilled at minimum cost.
1. Introduction
Indonesia is located at the confluence of three active earth slabs, namely the Indo-Australian plate, the Eurasian continental plate and the Pacific plate. Those plates periodically move and collide with each other, causing earthquake and series of active volcanoes. For instance, the Indian Plate is subducting beneath the Eurasian continental plate, which has formed the volcanic arc in western Indonesia, home to 129 active volcanoes in Indonesia that are a part of the Pacific Ring of Fire. This causes Indonesia to be vulnerable to natural disasters. Volcanic eruption was recorded as the third largest disaster in Indonesia after earthquake and tsunami (Leitmann, Citation2007). Mount Merapi is one of the most active volcanoes in Indonesia with a period of eruptions ranging between 2 and 7 years (Susilo & Iwan, Citation2014). Mount Merapi is located on the border of Central Java and Yogyakarta District and has a great potential danger when it erupts. The Indonesian National Mitigation Agency (BNPB) stated that Merapi eruption in 2010 caused 374 deaths and 279,702 inhabitants. The disaster also paralyzed local’s activities and caused enormous material losses.
According to Van Wassenhove (Citation2006), “Logistic is the most important element in any disaster relief effort, and it is the one that makes the difference between a successful and a failed operation. Yet, the logistic operation becomes the most expensive part of any disaster relief. Logistics accounts for about 80% of the total costs in disaster relief.” Effective mitigation planning is needed to minimize losses and costs of distribution and evacuation during the eruption. Indonesian constitution No. 24 year 2007 about disaster management defines mitigation as a series of effort aims to reduce disaster risks, through both physical development and awareness as well as increasing capacity to deal with natural disaster. In addition, it also aims to prevent and minimize the risk of loss and protection of property.
So far, investigations related to facility location of disaster management activities in the context of natural disasters have been attracted many academics and practitioners. Sherali, Carter, and Hobeika (Citation1991) developed a location-allocation model and studied the evacuation plan for hurricane/flood using nonlinear mixed integer programming method. A set of shelters was intended to be selected and an evacuation plan was prescribed in order to minimize the total evacuation time. ElDessouki (Citation1998) investigated both pre and post disaster phase for the natural disaster and aimed to evacuate the displaced population to the assigned shelters to obtain the minimum total evacuation time. Balcik and Beamon (Citation2008) developed a model that aims to maximize the demand and location coverage that can be provided to the displaced populations by determining the number and location of facilities. In the study, the budget constraint was applied with a decision regarding inventory level at distribution centers. Azlia (Citation2010) proposed a model with the objective to determine the location of a preparedness warehouse facility for the preparation of natural disasters. In the study, the vulnerability of a region to disaster, distribution center, and the number of inventories of each established distribution center were considered. Another works of location-allocation model in the case of natural disaster management have been done by Feng and Wen (Citation2003), Tuydes and Ziliaskopoulos (Citation2004), Murray and Mahmassani (Citation2003), Kongsomsaksakul, Yang, and Chen (Citation2005), Bozorgi-Amiri, Jabalameli, Alinaghian, and Heydari (Citation2012), Hong, Xie, and Jeong (Citation2012), Chang, Tseng, and Chen (Citation2007), McCall (Citation2006), Rawls and Turnquist (Citation2012), Zhang, Li, and Liu (Citation2012), Abounacer, Rekik, and Renaud (Citation2014) and Lin, Batta, Rogerson, Blatt, and Flanigan (Citation2012).
Despite those growing location-allocation models for natural disasters, few models specifically address location-allocation optimization for the case of Mount Merapi volcanic eruption. One of the studies that deal with the related case is Nugraha and Halim (Citation2012) in which a model was developed to choose the location of evacuation barracks, warehouse as well as service coverage to minimize total cost. In dealing with the real existing problems, it is necessary to develop a location-allocation model of the evacuation and the distribution with the criteria to minimize the relevant costs in accordance with the alert level of the disaster. Another work was done by Handayani, Rinawati, and Wiguna (Citation2015). The criteria was identified for determining the location of logistics warehouse in Sleman, Yogyakarta, Indonesia and the best alternative location of logistics warehouse was determined. Analytical Hierarchy Process (AHP) was applied to identify the criteria and select the best alternative location. The study concluded that the most important criteria is the national stability, access to logistics, and safety level.
According to Nugraha and Halim (Citation2012), the mitigation planning and management of Mount Merapi volcanic eruption in 2010 did not work well particularly in the area of Disaster Prone Areas III (DPA III). DPA III was located in Klaten district that includes Balerante Village, Panggang Village, Sidorejo Village, Tlogowatu Village and Tegalmulyo Village. There were many unsatisfied demand since the distributions of logistics did not fully fulfill the demands of the refugees. This could be noticed by the uneven distribution of aid, especially in Bawukan shelters and its surrounding areas. Those problem may end up to many devastating issues such as food scarcity, disease, or even worse, loss of life. These portrayal may be ample to make a point that is critical for the government to develop and plan a mitigation strategy of volcanic eruption. In order to satisfy all demands of aid, it is also necessary to make a planning allocation and distribution of the logistics. Those are done to ensure that all of the logistics are not piled up in certain barracks but rather be evenly distributed at a lowest cost.
This study presents a location-allocation optimization model of evacuation and distribution in volcanic eruption disaster. The objective of the model is to minimize the costs related to evacuation and distribution by choosing the best route and allocating the optimal number of evacuated refugees as well as the amount of aid distributed for each route. Different alert levels of disaster are considered which will end up in a different strategy of allocation, evacuation and distribution. Three mathematical models are developed to represent three different alert levels, which are alert, danger and post-disaster phases. A numerical example is presented using empirical data of the Mount Merapi volcanic eruption in 2010.
2. System description
The emergency plan and disaster management of volcanic eruptions are carried out based on the alert levels of the disaster. The Indonesian National Board for Disaster Management (BNBP) also implement the policy in managing natural disasters (Citation2015). By this policy, the distribution and evacuation of each alert level should be managed differently from one to another. In the case of Mount Merapi volcanic eruption, the alert level is classified into four categories ascending from its severity:
(1) | Normal level. The normal level is the basic level where there is no symptoms of magma pressure and the locals are safe to do some activities around the disaster-prone areas. | ||||
(2) | Attention phase. At this phase, there is an occurrence of significant changes in both physical and chemical parameters of the volcano in the form of increased seismic activity and other volcanic events. BNPB will provide a socialization to the locals regarding the current condition of the volcano. | ||||
(3) | Alert phase. The alert phase indicates that the volcano starts to enter the early warning where the volcano is moving towards eruption or causing disaster. Under these conditions, the Indonesian Regional Board for Disaster Management (BPBD) required to prepare emergency facilities. At this phase, the locals who want to leave can do safely, without fear of leaving their homes unattended. | ||||
(4) | Danger phase. At this phase, a certain eruption almost comes. All of the residents around the disaster-prone areas must be already evacuated to the evacuation shelters. The eruption began with the opening of ash and smoke which is likely to occur within 24 h. BPBD needs to clear the disaster-prone areas, especially some critical zones which is potential to receive more lava and hot clouds. BPBD also needs to provide a coordination to the populations to evacuate them into permanent barracks that located outside the disaster-prone areas. | ||||
(5) | Post-disaster phase. At this phase, eruption had arrived and the volcano experiences a decrease in seismic and volcanic activities. Refugees will be moved back from permanent barracks to temporary barracks. Even though the disaster was over, however the logistics distribution is still needed. This is due to prevent the occurrence of hunger and to treat health problems that may come at the end of the disaster. |
This study focuses on the alert phase, danger phase as well as the post-disaster phase. Logistics of aid were collected from government, non-governmental organization, individual communities and corporation, which subsequently be held and managed by BPBD. BPBD office as the warehouse, will distribute those supports to permanent barracks that located outside the disaster-prone areas. Permanent barracks will keep a portion of the logistical support as stocks and partially transmit to temporary barracks. Permanent barracks could send logistical support to various locations of temporary barracks, considering the distance between the permanent barracks to temporary barracks and capacity of each barrack.
At the alert phase, evacuation is started from disaster locations that located around Disaster Prone Areas III (DPA III). At this time, BPBD will provide assistance as well as socialization to the locals and expects them to immediately evacuate to temporary barracks as the eruption is getting closer. At the danger phase, refugees at the temporary barracks needs to evacuated to the safer shelter which is permanent barrack, located outside the disaster prone areas. The logistics of aid are directly distributed from the warehouse to permanent barracks.
After the danger phase, Mount Merapi experiences a decrease in both seismic and volcanic activities. The eruption causes enormous losses to the displaced population, including loss of livestocks and settlements or even loss of life. The refugees will return to temporary barracks and stay there for awhile during the recovering process. Meanwhile, logistical supports are still distributed to the refugees. Logistic assistances from permanent barracks will be channeled back to each temporary barrack. The comparison between evacuation and distribution of each alert-level are depicted in Figure .
3. Model development
This study develops humanitarian logistics based optimization models of aids distribution and refugees evacuation. As defined by Humanitarian Logistics Conference (2004), “humanitarian logistic refers to all processes of disaster management including planning, implementing and controlling the efficiency, cost-effectiveness and storage of goods and related information, from the point of origin (warehouses) to the point of consumption (refugees) to meet the end beneficiary’s requirements and alleviate the suffering of vulnerable people” (Ortuño et al., Citation2013). Humanitarian logistic models intend to optimize the management of the disasters in order to determine the best decision hence cost and loss can be minimized.
In the development of the model, new stages of distribution and evacuation are included, which are permanent barracks and temporary barracks. Here, three models are developed based on the classification of the emergency situations, i.e. the alert phase (Model I), the danger phase (Model II) and the post-disaster phase (Model III). The optimization problems of the proposed models are to minimize costs related to logistics distribution and refugees evacuation by determining the optimal values of decision variables, which are:
(1) | Ylk = decision to evacuate from disaster area l to temporary barrack k (Model I), | ||||
(2) | Xjk = decision to supply from permanent barrack j to temporary barrack k (Model I and III), | ||||
(3) | Zij = decision to supply from warehouse i to permanent barrack j (Model I, II and III), | ||||
(4) | Wkj = decision to evacuate from temporary barrack k to permanent barrack j (Model II), | ||||
(5) | Tjk = decision to evacuate from permanent barrack j to temporary barrack j (Model III). |
Considers,(1)
(1)
(2)
(2)
(3)
(3)
(4)
(4)
(5)
(5)
The notations used to develop the proposed model are listed as follows:
l | = | index of disaster area, l ∊ L |
k | = | index of temporary barrack, k ∊ K |
j | = | index of permanent barrack, j ∊ J |
i | = | index of warehouse, i ∊ I |
Vl | = | number of populations of disaster area l (person) |
Uk | = | capacity of temporary barrack k to resettle refugees (person) |
Uj | = | capacity of permanent barrack j to resettle refugees (person) |
Pj | = | capacity of permanent barrack j to resettle logistics (unit) |
Pi | = | capacity of warehouse i to resettle logistics (unit) |
B | = | demand (unit/person) |
Raij | = | distance between warehouse i and permanent barrack j (km) |
Rbjk | = | distance between permanent barrack j and temporary barrack k (km) |
Rclk | = | distance between disaster area l and temporary barrack k (km) |
Haij | = | cost to distribute one unit of aid from warehouse i to permanent barrack j ($/unit/km) |
Hbjk | = | cost to distribute one unit of aid from permanent barrack j to temporary barrack k ($/unit/km) |
Hclk | = | cost to evacuate one person from disaster area l to temporary barrack k ($/person/km) |
Hdjk | = | cost to evacuate one person from temporary barrack k to permanent barrack j ($/person/km) |
Hejk | = | cost to evacuate one person from permanent barrack j to temporary barrack k ($/person/km) |
Here, the assumption that evacuations and distributions are done in one time is used to develop the model. There is sufficient number of trucks to carry all units, which each truck has a capacity higher than or at least equal to the number of units. In this study, the meeting point of the refugees in each disaster area is determined using center of graphity method. The meeting point is then used to calculate the distance from each disaster area to the barracks. Models in this study also consider that evacuation shelters, including permanent barracks and temporary barracks, are assumed to have sufficient capacity to resettle the entire populations of refugee.
An evacuation cost and distribution cost that consists of fuel cost and evacuation trucks renting cost are considered in the model. The capacity of each truck is also taken into consideration. If one truck is not sufficient enough to accommodate all units, BPBD will rent an additional truck so that all units can be carried. The developed models will result in the optimal allocation of evacuation and distribution at every alert level of the disaster. Refugees can choose a desired evacuation barrack if there is an indifferent solutions within a predetermined allocation.
3.1. Model I: The alert phase
The first model is applied for a condition when situation is under alert phase. As explained in the previous section, the alert phase is the third level which indicates that the volcano starts to enter the early warning. The costs related to distribution and evacuation at the alert phase includes evacuation cost (EClk), second site distribution costs (DCjk) and first site distribution costs (DCij).
3.1.1. Evacuation costs (EClk)
Evacuation cost (EClk) for Model I refers to all costs related to evacuate refugees from the disaster location l to the temporary barrack k. Given a disaster location l and a temporary barrack k, decision to choose barrack k as temporary shelter for refugees from disaster location l is determined by the following capacity constraint:
(6)
(6)
Subsequently, the total evacuation cost from disaster location l to temporary barrack k is given by the following equation
(7)
(7)
3.1.2. Second stage distribution costs (DCjk)
Second stage distribution cost (DCjk) is incurred for every single item of logistics (including food, aid etc.) delivered from permanent barrack j to temporary barrack k. The decision to choose permanent barrack j as primary supplier for temporary barrack k, Xjk, is determined by the condition that the amount of items supplied to temporary barrack k should be equal to the number of refugees evacuated to the temporary barrack k and should be less than or equal to the capacity of permanent barrack j. Mathematically, the condition is formulated by Equation (8).
(8)
(8)
Subsequently, the total distribution cost from permanent barrack j to temporary barrack k is formulated by.
(9)
(9)
3.1.3. First stage distribution costs (DCij)
First stage distribution cost (DCij) is incurred for every single item of logistics that delivered from warehouse i to permanent barrack j. The decision to supply aid to permanent barrack j from warehouse i, Zij, is determined by the condition that the amount of items supplied to permanent barrack j should be equal to the number of refugees evacuated to the permanent barrack j and should be less than or equal to the capacity of warehouse i. This condition can be presented mathematically by Equation (10).
(10)
(10)
With denotes the total number of refugees evacuated to permanent barracks from temporary barracks. Hence, the calculation of the total distribution cost from warehouse i to permanent barrack j is as follows.
(11)
(11)
The total cost function (TCijlk) of Model I is obtained by summing up all costs related to evacuation and distribution including EClk, DCjk and DCij. Therefore, the following function of TCijlk is obtained
(12)
(12)
3.2. Model II: The danger phase
The second model is developed for the danger phase of volcanic disaster. Model II aims to find the optimal decisions of distribution and evacuation for a condition when there are indications that the volcano starts to move towards eruption. The costs related to distribution and evacuation at the danger phase include evacuation cost (ECkj) and distribution costs (DCij).
3.2.1. Evacuation costs (ECkj)
Evacuation cost (ECkj) for Model II refers to all costs related to transfer refugees from temporary barrack k to permanent barrack j. The decision to choose barrack j as permanent shelter for refugees from temporary barrack k is determined by the following constraint of capacity:
(13)
(13)
Subsequently, the total evacuation cost from temporary barrack k to permanent barrack j is obtained as follows.
(14)
(14)
3.2.2. Distribution costs (DCij)
In Model II, distribution cost (DCij) is incurred for any kind of logistics that delivered from warehouse i to permanent barrack j. The decision to choose warehouse i for supplying logistics to permanent barrack j, Zij, is determined by the condition that the amount of items supplied to permanent barrack j should be equal to the number of refugees evacuated to the barrack and should be less than or equal to the capacity of warehouse i. These constraint capacity is formulated by Equation (15).
(15)
(15)
Subsequently, the total distribution cost from warehouse i to permanent barrack j is expressed as follows.
(16)
(16)
The total cost function (TCijlk) of Model II is obtained by summing up all costs related to evacuation and distribution including ECkj and DCij. Therefore, the function of TCijlk is formulated as follows
(17)
(17)
3.3. Model III: The post-disaster phase
The third model is developed for the post-disaster phase and aims to find the optimal decisions of distribution and evacuation for a condition when the eruption has ended and the seismic and volcanic activities have decreased. The optimization of Model III is done after Model II has already been optimized. The costs related to distribution and evacuation at the post-disaster phase include evacuation cost (ECjk), costs of second stage of distribution (DCjk) and costs of first stage of distribution (DCij).
3.3.1. Evacuation costs (ECjk)
Evacuation cost (ECjk) for Model III refers to all costs related to move refugees from permanent barrack j back to temporary barrack k. The decision to move refugees from barrack j to barrack k is determined by the following constraint of capacity:
(18)
(18)
The total evacuation cost from permanent barrack j to temporary barrack k is given by.
(19)
(19)
3.3.2. Second stage distribution costs (DCjk)
Distribution cost for Model III is similar to distribution cost in Model I. The distribution also divided into two stages. The first stage for distribution from the warehouse to permanent barrack and the second stage refers to distribution from permanent barrack to temporary barracks. Therefore, the constraints and function of DCjk for Model III is calculated from Equations (8) and (9), respectively.
3.3.3. First stage distribution costs (DCij)
Costs to distribute logistics from the warehouse to permanent barrack at the post-disaster phase can be calculated using Equation (11), subject to Equation (10). The total cost function (TCijlk) for Model III is the total of all costs related to evacuation and distribution, including ECjk, DCjk and DCij, which is.(20)
(20)
3.4. Transforming the models into linear functions
Models I, II and III that have been developed in the previous section, still contain some multiplications of two binary variables that cause non-linear functions. In this case, a global optimal solution is hard to be generated. Therefore, the function must be linearized by replacing two binary variables with one new binary variable (Smith & Taşkın, Citation2008). According to Vialar (Citation2015), “linear models are easier to understand than nonlinear models and are necessary for most control system design methods”. In this section, the linear transformation for both Model I and II is provided.
3.4.1. Model I: the alert phase
After performing a linear transformation to the current Model I, a new function of TCijkl for Model I is obtained as:
(21)
(21)
Subject to:
(22)
(22)
(23)
(23)
(24)
(24)
(25)
(25)
(26)
(26)
(27)
(27)
(28)
(28)
(29)
(29)
(30)
(30)
(31)
(31)
(32)
(32)
(33)
(33)
(34)
(34)
(35)
(35)
(36)
(36)
(37)
(37)
3.4.2. Model II: the danger phase
By using the same procedure, the function of TCijkl in Equation (17) can be rewritted as follows:
(39)
(39)
Subject to:
(39)
(39)
(40)
(40)
(41)
(41)
(42)
(42)
(43)
(43)
(44)
(44)
(45)
(45)
(46)
(46)
(47)
(47)
(48)
(48)
(49)
(49)
3.4.3. Model III: the post-disaster phase
By using the same procedure, the function of TCijkl in Equation (Equation2(2)
(2) 0) can be transformed into:
(50)
(50)
Subject to:
(51)
(51)
(52)
(52)
(53)
(53)
(54)
(54)
(55)
(55)
(56)
(56)
(57)
(57)
(58)
(58)
(59)
(59)
(60)
(60)
(61)
(61)
(62)
(62)
(63)
(63)
(64)
(64)
(65)
(65)
(66)
(66)
(67)
(67)
(68)
(68)
(69)
(69)
(70)
(70)
4. Case study: Mount Merapi volcanic eruption in 2010
In this section, an example of the model’s application is given based on the case of volcanic eruption in Mount Merapi, Indonesia. Mount Merapi is one of the most active volcanoes in Indonesia which located in the border of Central Java, approximately 28 km north of Yogyakarta City. Ever since 1500s, Mount Merapi has been erupting regularly and still has potential to erupt after its last eruption in 2014. One largest disaster caused by Mount Merapi volcanic eruption happened in 2010.
In 2010, planning and execution of Mount Merapi disaster mitigation did not run effectively, particularly for Disaster Prone Area III in Klaten District, including Balerante, Panggang, Sidorejo, Tlogowatu and Tegalmulyo. One major problem was bad distribution of logistics, i.e. foods and aid, to the refugees. As the distribution did not run efficiently, the cost increased yet the needs of refugees could not be fully fulfilled. By applying the proposed optimization model to the system, the optimal solution, including location of the permanent barrack and temporary barrack as well as warehouses allocation to supply the needs of each barrack, is determined. Disaster Prone Area III is then used as an object of the study. The population of Disaster Prone Area III are presented in Table .
Table 1. Number of population in each region of Disaster Prone Area III
In the early stage of eruption, residents around the Disaster Prone Area III will be evacuated to temporary barracks. Some temporary barracks have been provided in Kepurun, Bawukan, Dompol and Keputran Villages. Each temporary barrack has a capacity of 3,200 persons and 3,200 units of aid. The distance between disaster areas in Disaster Prone Area III and temporary barracks (in km) can be found throughout Table .
Table 2. Distances between disaster areas in DPA III and temporary barracks
In the alert phase, aid are distributed from the warehouse to the temporary barracks through the permanent barracks. Warehouse is located in BPBD office of Klaten District. Whereas, permanent barracks are located in Demak Ijo village, Menden village and Kebondalem Lor village. Each permanent barrack has a capacity of 4,200 persons and 4,200 units of aid. Distances from the warehouse to each permanent barrack and distances from permanent barracks to temporary barracks are given in Tables and , respectively.
Table 3. Distances between warehouse and permanent barracks
Table 4. Distances between temporary barracks and permanent barracks
As for the danger phase, aid are distributed directly from the warehouse to the permanent barracks. Since the situation is more dangerous, refugees are already moved from the temporary barracks to the permanent barracks. With the assumption that all of the aid received in the previous delivery were already ran out. Given a consumption rate of aid (B) is 1 unit/person, cost of evacuation (Hclk) is $0.115/km/person and cost of distribution (Hbjk and Haij) is $0.115/km/unit, a strategic planning of disaster mitigation can be generated using the proposed model.
4.1. Optimization of Model I: Alert phase
From the above case, the optimal solution that minimizes the cost of evacuation and distribution can be determined using linear programming method. Empirical data used as input values of each parameter as presented in Table .
Table 5. Empirical data as input parameters of the model
Using the proposed solution procedure, the optimal allocations and the total cost for Model I are obtained. The optimal solutions of evacuation and distribution are presented in Figure .
The optimal solutions in Figure give a minimum total cost (TCijlk) for Model I as calculated as:
4.2. Optimization of Model II: Danger phase
Applying the same solution procedure, the optimal allocations of evacuation and the distribution for Model II are obtained as presented by Figure .
The optimal solutions in Figure give a minimum total cost (TCijlk) for Model II as:
4.3. Optimization of Model III: Post-disaster Phase
Using the same solution procedure, the optimal allocations of evacuation and the distribution for Model III are obtained as presented by Figure .
The optimal solutions in Figure give a minimum total cost (TCijlk) of Model III as calculated as:
5. Result discussion and analysis
In this section, the mathematical model along with the numerical example results that have been developed in the previous section are discussed further.
5.1. The optimal route of evacuation and distribution
The percentage of evacuated populations and the optimal distribution and evacuation routes are shown in Tables and .
Table 6. Percentage of evacuated populations on each temporary barrack
Table 7. Percentage of evacuated populations on each temporary barrack
By optimizing the model, the utility of each barrack can be maximized up to 100%, except for Kepurun barrack which has 3% of remaining capacity. From those results, it can be said that the model is effective to utilize the usage of each evacuation shelter. Waste in term of capacity is minimized, hence the related costs can be minimized either. However, the proposed model only consider cost related to transportation, including fuel cost and leasing cost of trucks. Whereas another costs such as holding cost, stocks deterioration cost as well as intangible losses, including time losses and emotional losses, were not considered in the model. Nevertheless, since the focus of the study is on the allocation model of the distribution and the evacuation, the generated solution is the optimal solution which gives the lowest cost.
5.2. Comparison of Models I, II and III
The comparison presented in Table shows that Model III, the post-disaster phase, gives highest cost of evacuation and distribution. Whereas, Model II, the danger phase, delivers lowest cost of evacuation and distribution compared to the other models. The reason is that on the danger phase, distribution was done directly from the warehouse to permanent barracks. Meanwhile, for Model I and III, distributions were done twice, which are distribution from warehouse to permanent barrack and distribution from permanent barrack to temporary barrack.
Table 8. Total cost comparison of Model I, II and III
The cost related to distribution in the phase of alert and post-disaster can be reduced by applying a one-time distribution from warehouse directly to temporary barracks. Another alternative is to relocate the warehouse to be closer to the temporary barrack, yet not too far from the permanent barrack. The location of the warehouse is currently considered far enough that it does not allow direct delivery from the warehouse to the temporary barrack. By relocating the warehouse to be closer to the temporary barrack, costs related to distribution can be reduced.
5.3. Effects of undesired situations on the Model’s optimal solutions
The proposed model is developed by ignoring any undesired intrusions. However, in fact, any particular situation that is not in accordance with the expected conditions may occur. Here, some possible actions that can be done in order to maintain the optimal solutions are suggested. Undesired situations that may be experienced by the system include damage in either evacuation or distribution pathways, number of population that exceeds the capacity of the barrack and lack of the available evacuation and distribution trucks.
5.3.1. Damage in either evacuation or distribution pathways
Damaged pathways might happened before or after a disaster occurred. In a case when the pathways were damaged before the disaster, some possible causes were the lanes that often being passed by material carrier such as rocks and sand. Whereas, the damaged path after disaster might be a result of the cold lava material that could destroy the evacuation and distribution path instantly. If there exists a damaged pathway, BPBD will immediately change the evacuation or distribution path using other shortest alternative path.
From the above case study, a scenario is given to represents the condition during the post-disaster phase which is, the main road from Keputran Village to Kepurun Village was destroyed by cold lava flood. The other shortest alternative route that can connect the two places should be determined. The new distances between locations of permanent barrack and temporary barrack are given in Table . As a response to the situation, a new possible solution is suggested to determine the minimum cost of evacuation and distribution as presented by Table .
Table 9. Distances between temporary barracks and permanent barracks
Table 10. New possible solution of distribution during the post-disaster phase (as a result of damaged pathways between Kepurun and Keputran Villages)
From the brand-new solutions presented in Table , the new total cost for post-disaster phase is obtained which is, TCijkl = $47,131.13. Within the new solution, it is concluded that the total cost is increased as a result of any undesired situations. Moreover, the travel time will be much longer and may allow changes in the evacuation and distribution allocation of each barracks.
5.3.2. Number of population exceeds the capacity of the barrack
Capacity of each barrack is primarily designed to accommodate all displaced residents from any disaster sites. If the population exceeds the barrack capacity due to population growth, BPBD will increase the number of tents for the temporary barracks. As for the permanent barracks, BPBD will use the buildings owned by the government of Klaten District that are available and close to the location of permanent barracks. This situation may increase the cost of evacuation. The optimal solution should be revisited if the system experiences this kind of undesired situation.
5.3.3. Lack of the available evacuation and distribution trucks
Truck becomes a very important vehicle in this system as it plays a major role in accommodating a large quantity of refugees and logistics. If the available trucks are not sufficient enough to carry all units in one time, there will be repeated transports from the origin point to its destination. The repetition will result in longer evacuation and distribution time. Under these circumstances, BPBD will decide to add more trucks in order to perform the evacuation and distribution in one time. By adding more number of trucks, the evacuation as well as distribution costs will be much higher. Therefore, the optimal solution should be revisited again in accordance with the situation experienced by the system.
6. Conclusions
This study developed optimization models to solve the allocation problem of volcanic eruption disaster. The objective of the proposed model was to determine the optimal route and allocation of refugees and logistics that minimize costs related to evacuations and distributions. Different policies for different alert levels of disaster were presented. Three models were developed for three alert levels, which are alert phase, danger phase and post-disaster phase. Each model has a different solution considering the different evacuation and distribution paths required for each level alert. Two additions of evacuation shelter were included, which are temporary barrack and permanent barrack. Constraint-based optimizations were done and optimal allocations of each model were obtained.
The models developed in this study can be further extended by including other costs such as costs for predisaster setup, holding cost, shortage cost, stocks deterioration cost as well as intangible losses, including time losses and emotional losses. In addition, other issues concerning the humanitarian supply chain in the context of disaster management also need to be considered. According to Ortuño et al. (Citation2013), the main issues of disaster management include unpredictable demand and timing, short lead time, suddenness demand of huge amounts of products or services and lack of initial resources. The models in this study can also be further developed by picturing the problem of disaster management into a broader view that includes not only mitigation phase but also other phases such as preparedness, response as well as recovery as these phases are considered to form a non-stop cycle of disaster management (Tomasini & Wassenhove, Citation2009).
Funding
The authors received no direct funding for this research.
Acknowledgements
The authors thank the anonymous referees for their constructive and helpful comments on an earlier version of the manuscript.
Additional information
Notes on contributors
Aditya Respati Prabowo
Aditya Respati Prabowo is a junior researcher in Production System Laboratory, Sebelas Maret University. His research interests are operations research, production system and logistics management.
Anindya Rachma Dwicahyani
Anindya Rachma Dwicahyani is a master student in Industrial Engineering Department, Sebelas Maret University. Her research interests are inventory management, supply chain management and operations research.
Wakhid Ahmad Jauhari
Wakhid Ahmad Jauhari is currently a senior researcher in Center for Research in Manufacturing Systems (CRIMS), Sebelas Maret University. His research interests include modelling inventory, supply chain management and manufacturing design.
Azizah Aisyati
Azizah Aisyati is a senior researcher in Center for Research in Manufacturing Systems (CRIMS), Sebelas Maret University and a PhD student in Industrial Engineering Department, Institut Teknologi Bandung. Her research interests are operations research, production management and supply chain management.
Pringgo Widyo Laksono
Pringgo Widyo Laksono is a senior researcher in Center for Research in Manufacturing Systems (CRIMS), Sebelas Maret University. His research interests are production and manufacturing systems, design working system, optimisation, and operation management.
References
- Abounacer, R., Rekik, M., & Renaud, J. (2014). An exact solution approach for multi-objective location-transportation problem for disaster response. Computers & Operations Research, 41, 83–93.10.1016/j.cor.2013.08.001
- Azlia, W. (2010). Model determination of preparedness warehouse facilities for natural disaster by considering region vulnerability factors (Master Thesis). Industrial Technology Faculty of Institut Sepuluh November, Surabaya (in Indonesian).
- Badan Nasional Penanggulangan Bencana. (2015). Strategic planning of Indonesian national board for disaster management 2015–2019. Jakarta: Author.
- Balcik, B., & Beamon, B. M. (2008). Facility location in humanitarian relief. International Journal of Logistics Research and Applications, 11, 101–121.10.1080/13675560701561789
- Bozorgi-Amiri, A., Jabalameli, M. S., Alinaghian, M., & Heydari, M. (2012). A modified particle swarm optimization for disaster relief logistics under uncertain environment. The International Journal of Advanced Manufacturing Technology, 60, 357–371.10.1007/s00170-011-3596-8
- Chang, M.-S., Tseng, Y.-L., & Chen, J.-W. (2007). A scenario planning approach for the flood emergency logistics preparation problem under uncertainty. Transportation Research Part E: Logistics and Transportation Review, 43, 737–754.10.1016/j.tre.2006.10.013
- ElDessouki, W. M. (1998). Some developments in transportation network analysis and design with application to emergency management problems (PhD Dissertation). North Carolina State University, Raleigh, NC.
- Feng, C. M., & Wen, C. C. (2003). Traffic control management for earthquake-raided area. Journal of the Eastern Asia Society for Transportation Studies, 5, 3261–3275.
- Handayani, N. U., Rinawati, D. I., & Wiguna, Y. K. (2015). Model of Pre-Positioning Warehouse Logistics for Disaster Eruption of Mount Merapi in Sleman, Yogyakarta. In Proceeding of the Joint International Conference on Electric Vehicular Technology and Industrial, Mechanical, Electrical and Chemical Engineering (pp. 401–405). Yogyakarta.
- Hong, J.-D., Xie, Y., & Jeong, K.-Y. (2012). Development and evaluation of an integrated emergency response facility location model. Journal of Industrial Engineering and Management, 5, 4–21.
- Kongsomsaksakul, S., Yang, C., & Chen, A. (2005). Shelter location-allocation model for flood evacuation planning. Journal of the Eastern Asia Society for Transportation Studies, 6, 4237–4252.
- Leitmann, J. (2007). Cities and calamities: Learning from post-disaster response in Indonesia. Journal of Urban Health, 84, 144–153.10.1007/s11524-007-9182-6
- Lin, Y.-H., Batta, R., Rogerson, P. A., Blatt, A., & Flanigan, M. (2012). Location of temporary depots to facilitate relief operations after an earthquake. Socio-Economic Planning Sciences, 46, 112–123.10.1016/j.seps.2012.01.001
- McCall, V. M. (2006). Designing and pre-positioning humanitarian assistance pack-up kits (HA PUKs) to support pacific fleet emergency relief operations (Master Thesis). Naval Postgraduate School, Monterey, CA.
- Murray, P. M., & Mahmassani, H. S. (2003). Model of household trip chain sequencing in an emergency evacuation. Presented at 82nd Annual Meeting of the Transportation Research Board, Washington, DC.
- Nugraha, I. S., & Halim, A. H. (2012). Model determination of location of evacuation barracks and supplier warehouse in natural disaster management. Prosiding Seminar Sistem Produksi, Industrial Technology Faculty, Institut Teknologi Bandung, Indonesia (in Indonesian).
- Ortuño, M. T., Cristóbal, P., Ferrer, J. M., Martín-Campo, F. J., Muñoz, S., Tirado, G., & Vitoriano, B. (2013). Decision aid models and systems for humanitarian logistics. A survey. In Decision aid models for disaster management and emergencies, Atlantis computational intelligence system 7 (pp. 17–44). Paris: Atlantis Press. doi:10.2991/978-94-91216-74-9_2
- Rawls, C. G., & Turnquist, M. A. (2012). Pre-positioning and dynamic delivery planning for short-term response following a natural disaster. Socio-Economic Planning Sciences, 46, 46–54.10.1016/j.seps.2011.10.002
- Sherali, H. D., Carter, T. B., & Hobeika, A. G. (1991). A location-allocation model and algorithm for evacuation planning under hurricane/flood conditions. Transportation Research Part B: Methodological, 25, 439–452.10.1016/0191-2615(91)90037-J
- Smith, J. C., & Taşkın, Z. C. (2008). A tutorial guide to mixed-integer programming models and solution techniques. Engineering and Management Innovation, 10, 1–23.
- Susilo, A. N., & Iwan, R. (2014). Analysis of the risk level of mount merapi eruption on the settlements in Kemalang District. Klaten Regency. Jurnal Teknik PWK, 3, 34–39.
- Tomasini, R., & Wassenhove, L. (2009). Humanitarian logistics. NewYork, NY: Palgrave Macmillan.10.1057/9780230233485
- Tuydes, H., & Ziliaskopoulos, A. K. (2004). Network re-design to optimize evacuation contraflow. Presented at 83rd Annual Meeting of the Transportation Research Board, Washington, DC.
- Van Wassenhove, L. (2006). Humanitarian aid logistics: Supply chain management in high gear. Journal of the Operational Research Society, 57, 476–489.
- Vialar, T. (2015). Handbook of mathematics. Paris: Books on Demand.
- Zhang, J.-H., Li, J., & Liu, Z.-P. (2012). Multiple-resource and multiple-depot emergency response problem considering secondary disasters. Expert Systems with Applications, 39, 11066–11071.10.1016/j.eswa.2012.03.016