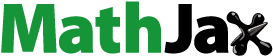
Abstract
Monitoring of effluent turbidity is essential to evaluate the coagulation process in wastewater treatment plants (WWTPs). A digital imaging system based on the texture analysis of flocs has been tested in a Norwegian municipal WWTP to predict changes in coagulation conditions and outlet turbidity. Principal component analysis (PCA) was applied to prove that the textural features of flocs’ images depend on the inlet wastewater parameters and coagulation conditions. Partial least squares regression (PLSR) was performed for the outlet turbidity prediction. The best model resulted in 86.6% prediction accuracy using two wastewater quality parameters (inlet flow and inlet turbidity) and 4 textural feature vectors retrieved from the images of flocs. Furthermore, the outlet turbidity predicted by this model resulted in a lower amount of underestimated values compare to the model, which contained only wastewater quality parameters. A new term—floc texture index (FTI) summarizes the textural features of flocs’ images resulting in a single variable (thought linear combination). This further simplifies the current multivariate dosage control system. Analysing the plant data indicates that an FTI value below 6 would result in the outlet turbidity values above 5. This can be used as an early warning system of coagulation failure. The results of these studies demonstrate the potential of the digital imaging system to improve an existing online coagulant dosing control strategy.
Public Interest Statement
Last decades researchers working with water and wastewater treatment often try to benefit from applying image analysis based systems to the different processes. Doing this we are able to monitor or even properly control these processes, for instance wastewater coagulation, which is often difficult otherwise. In this paper, we are testing how the digital imaging system based on texture analysis of particles (flocs) performs in a full-scale Norwegian wastewater treatment plant. Retrieving the textural parameters from the images of flocs obtained during different coagulation conditions in the plant, we were able to indicate the changes in wastewater inlet and outlet quality parameters. The prediction of outlet wastewater quality parameters (turbidity in this case) is necessary to provide efficient coagulant dosage control and/or develop the coagulation failure alarm system. The results of these studies are to be used to improve the existing online coagulant dosing control system.
1. Introduction
Coagulation is a well-known and widely used water and wastewater treatment method to remove suspended solids, phosphates and other water impurities. Particles which aggregate during the coagulation/flocculation process are called flocs. The amount of coagulant (chemical material, addition of which results in suspension’s destabilisation), its nature, concentration and mixing conditions are the main factors influencing flocs aggregation and breakage. The size and surface properties of flocs formed under different coagulation mechanisms strongly influence their behaviour during further solid-liquid separation process (Bache & Gregory, Citation2007). Many researchers have studied different floc features, such as size distribution and fractal dimension (Chakraborti, Atkinson, & Van Benschoten, Citation2000; Li, Zhu, Wang, Yao, & Tang, Citation2006; Vahedi & Gorczyca, Citation2011). Some attempts were done to create online systems for flocs characterisation and monitoring. A method of online floc size evaluation based on nephelometric turbidity measurements was presented (Cheng, Kao, & Yu, Citation2008) and further developed into nephelometric turbidimeter monitoring system (NTMS) (Cheng, Chang, Chen, Yu, & Huang, Citation2011; Yu, Chen, & Cheng, Citation2017). Photometric dispersion analyser (PDA) is an optical instrument often used to study the aggregates’ characteristics (Burgess, Curley, Wiseman, & Xiao, Citation2002; Chou, Lin, & Huang, Citation1998; Wu, Wang, Hu, & Ye, Citation2013). Nevertheless, many attempts were done so far to study, characterise and control particles aggregating during coagulation, there is still a gap in the application knowledge of how to use the floc features to optimize the coagulation process, provide cheap and robust dosage control system.
Nowadays the flow-proportional dosing concept is usually used for coagulation dosage control, while process optimisation often bases on results from the jar tests and the operator’s experience (Ratnaweera & Fettig, Citation2015). Streaming current detector (SCD) was evaluated (Dentel, Thomas, & Kingery, Citation1989a, Citation1989b) and tested in the drinking water treatment plants (DWTP) for automatic coagulation control (Critchley, Smith, & Pettit, Citation1990; Yavich & Van De Wege, Citation2013). The coagulant dosage strategy based on zeta potential measurements documented to be a promising control technique (Sharp et al., Citation2005, Citation2016). Advanced soft sensors and coagulation process control models employing artificial neural networks (ANN) have been tested in DWTPs (Baxter et al., Citation2002; Juntunen, Liukkonen, Lehtola, & Hiltunen, Citation2013; Valentin & Denœux, Citation2001). Advanced dosing control systems based on multiple water quality parameters that could be measured online have confirmed to be successful both in DWTPs (Liu, Citation2016; Liu, Ratnaweera, & Song, Citation2013) and wastewater treatment plants (WWTPs) (VA-Support, Citation2012). Application of such systems enables a reduction of coagulant consumption (i.e. minimise the operational costs), reduces the sludge volumes and maintains the desired removal of particles and phosphates (Manamperuma, Ratnaweera, & Rathnaweera, Citation2013; Manamperuma, Wei, & Ratnaweera, Citation2017). With the growing need of wastewater treatment processes optimisation, the need for further development of intelligent, accurate and reliable online dosing control systems arises (Ratnaweera & Fettig, Citation2015).
Last decades digital image analysis (DIA) techniques have often been employed to determine particles characteristics, such as floc size, fractal dimension, strength and breakage (Jarvis, Jefferson, Gregory, & Parsons, Citation2005). Fractal dimension and floc size appear to be the most known and conventional parameters used in numerous attempts to monitor and/or control the coagulation process by DIA (Bouyer, Coufort, Liné, & Do-Quang, Citation2005; Chang, Liu, & Zhang, Citation2005; He, Nan, Li, & Li, Citation2012; Vlieghe, Coufort-Saudejaud, Frances, & Liné, Citation2014; Xu, Chen, Cui, & Shi, Citation2016; Yu, Citation2014). Other floc parameters retrieved from the images (average equivalent diameter, eccentricity and colour index) are also essential, for instance, in combination with self-organizing maps to monitor changes during the coagulation process and estimate flocs quality (Juntunen, Liukkonen, Lehtola, & Hiltunen, Citation2014).
In most cases the applicability of particles detection methods in wastewater coagulation process has been limited to lab scale due to complicated and inaccurate measurements in the field, hardware and software limitations. We propose a new approach to image analysis of flocs, which was previously tested on the laboratory scale batch tests (jar tests) (Sivchenko, Kvaal, & Ratnaweera, Citation2014, Citation2016). It is a comparatively easy method of image characterization, which bases on analysis of the whole image texture instead of concerning the shape characteristics of each particle in the image. Such approach simplifies the image analysis stage, e.g. no need of particles extraction/segmentation from the image and its count which found to be problematic in wastewater applications (Sivchenko et al., Citation2016). Hence, it gives significant simplification of the software to be developed. Furthermore, out of focus flocs, which are often present in the images, are not a problem for this texture complexity recognition method. Thus, potentially the cheap cameras could be employed for the floc sensor development. This paper presents the applicability of the concept in continuous mode with real wastewater in the context to use it as a dosage control technique for optimizing the coagulation process.
2. Experimental setup and methods
2.1. Wastewater treatment plant
Full-scale tests were conducted in the Frogn wastewater treatment plant (Drøbak, Norway) in September and October 2015. Frogn WWTP receives municipal wastewater from Drøbak city and the neighbourhood area. Average inlet flow is 4,600 m3/day during the days without snowmelt and/or precipitations. The tests period include days when the long precipitation period took place. The maximum flow rate to the plant reached 18,845 m3/day on the 18 September 2015.
Frogn WWTP is a mechanical-chemical precipitation plant. The treatment process consists of the next stages: screens, two parallel pre-sedimentation basins, three sequenced coagulation chambers with the different velocity gradients, and two parallel sedimentation chambers. The plant also has the sludge dewatering and thickening system. The inlet and outlet water quality parameters are measured by online sensors and recorded (average values) with the 15 min interval. The data is available for observation in the plant’s SCADA system. The retrieved water parameters included inlet wastewater flow (QIN), inlet pH (PHI), inlet turbidity (TUI), inlet conductivity (CNI), coagulant dosage (Dose), pH after coagulant dosage (PHO) and outlet turbidity (TUO). The plant operators perform daily sampling of inlet and outlet total Phosphorous (total P). The summarised data of water quality parameters for the tests period is given in Table . Coagulant used in the Frogn WWTP is polyaluminium chloride (ECOFLOCK 90, Feralco), 9 ± 0.3% Al by weight, and density 1,356 ± 25 kg/m3.
Table 1. Inlet and outlet wastewater quality parameters of Frogn WWTP
2.2. Image acquisition and pre-processing
A special installation was designed to observe changes in flocs’ structure in situ. The installation was set above the second flocculation chamber and consisted of the tube, peristaltic pump, acrylic cell for image acquisition, digital camera and computer (Figure ). To minimise the potential danger of flocs breakage, the chosen tube was 3 cm in diameter, and the peristaltic pump was placed after the imaging cell. The water flow in the system was upstream and manually adjusted to approximately 40 l/h.
Figure 1. (a) Schematic representation of the installation and (b) a photograph from the Frogn WWTP.
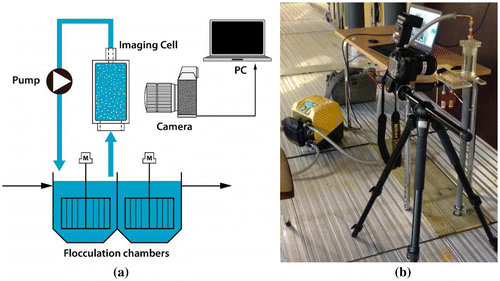
Images of flocs were constantly taken with a pre-set repeatability using free remote camera control software—DigiCamControl 1.2.0. Image capturing equipment used during the investigations was as follows: digital single lens reflex (DSLR) Nikon D600 camera, 105 mm Nikkor AF-S Micro 1:2.8 G ED lens (Nikkor, China), SpeedLite YN460 flash (Yongnuo, China). The size of the image-capturing zone in the cuvette was 3.3 × 10.3 cm. In order to obtain flocs with the proper depth of field, the black metal stripe was placed in the centre of the cuvette, which also became a background for the flocs. The choice of the background colour was based on the fact that the wastewater flocs are greyish coloured. Thus, using a contrasting background, it is easier to perform the further image analysis.
The obtained images have a resolution of 24.3 megapixels each. They were processed in the open source image analysis software ImageJ v.1.49 (Rasband, Citation1997/2016) that bases on plugins and macros. For each image 1380 × 3640 pixels (2.4 × 6.3 cm) area was cropped by manual investigation of the area. Because of slight changes in lighting conditions during image acquisition, all images were pre-processed in order to have the same brightness intensity. We wrote ImageJ plugin to mean centre the images’ grey-tone values.
2.3. Image analysis by Grey level co-occurrence matrix (GLCM)
GLCM is a common method for the image texture measurement. Previously it was successfully applied in the laboratory scale data (Sivchenko et al., Citation2016). The GLCM method was chosen among the other texture analysis methods because it is quite simply computable and does not require heavy programming for the sensor prototype to be developed.
ImageJ plugin “GLCM Texture Too” v. 0.009 was used to obtain the GLCM feature vectors. The resulting output was given as a vector of the next 9 parameters per each image: contrast, correlation, inverse difference moment (IDM), entropy, energy, homogeneity, prominence, variance, and shade. Hence, the data matrix was obtained with the size 342 × 9. The detailed description, explanation, and equations for above GLCM texture features are given elsewhere (Conners, Trivedi, & Harlow, Citation1984; Haralick, Shanmugam, & Dinstein, Citation1973; Zheng, Sun, & Zheng, Citation2006).
2.4. Conjugation of two data sets
Three images for each 15 min were chosen to be representative. The measured GLCM feature vectors were averaged for each 3 images and matched with the retrieved water quality parameters. According to the tracer tests conducted in Frogn WWTP, the outlet turbidity values were 45 min shifted to meet the response lag between the coagulant injection point and outlet from the sedimentation tank. After the removal of missing values and outliers, the resulting data-set contains 114 samples. The data include 81.6% of samples under the normal operation conditions, 15.8% of samples under rainy weather conditions and 2.6% of samples which had high inlet turbidity values due to the periodic discharge from the septic tanks to the inlet.
2.5. Multivariate statistical analysis and modelling
The resulted data matrix was processed in statistical software The Unscrambler® X 10.3 (CAMO Software AS, Norway) and in MATLAB® using PLS toolbox 8.2 (Eigenvector Research, Inc., USA). Principal component analysis (PCA) was performed to find the relationships between water quality parameters and GLCM feature vectors. PCA is a statistical data analysis technique to reduce the dimensionality of the data-set, overview and describe the interrelationships among variables and to find so-called hidden structures in the data. Partial least squares regression (PLSR) was performed to predict outlet turbidity based on different combinations of water quality parameters and GLCM texture features.
3. Results and discussion
3.1. Data overview
At the time when the tests were conducted, Frogn WWTP used the flow-proportional concept of coagulation dosage control with the ability of manual dose adjustment. However, now the plant has an advanced dosage control system—DOSCON® (DOSCON AS, Oslo, Norway), which uses the inlet wastewater quality parameters to calculate the optimal dose. A detailed description of the control strategy can be found elsewhere (Liu & Ratnaweera, Citation2017; Manamperuma et al., Citation2017). Although the coagulant consumption and the plant performance were significantly improved, the system still depends on the reliability of the online equipment functioning in a harsh environment.
Figure shows an example of the load changes during the 9 days observation period in September 2015. The first 3 days represent the typical wastewater flow variations during the day under the normal (dry) weather conditions. On the fourth day, the rainy week started, and the wastewater load had significantly increased reaching a peak of 900 m3/h at the beginning of the ninth day. Corresponding flow-proportional coagulant dosages in μl/l are also marked in the figure. The images of exemplary floc structures appeared under different coagulation conditions are shown for the days when image analysis observations took place. Visual investigation of flocs can be described as next: the flocs formed during normal operation conditions tend to be bigger compared to those formed during the rainy days. This can be explained by the change in inlet wastewater composition, when domestic wastewater was highly diluted by stormwater. During the rain events avarage inlet flow to the plant increased 3 times, avarage inlet turbidity decreased 2–2.5 times, the average inlet conductivity of wastewaer decreased 1.3 times and average inlet pH decreased from 7.2 to 6.9. These changes in wastewater inlet parameters could result in a change of aggregates properties and size (Bache & Gregory, Citation2007). Even though the coagulant dosing control in the plant bases on the flow-proportional concept, the dosage of coagulant was lowered manually during the rainy days period. Relatively high turbidity values of outlet water from the sedimentation tank during the last two rainy days point out on poor coagulation conditions with the non-optimal dosages. The multivariate statistical analysis was employed to test if the images of appearing during coagulation flocs reveal the information about coagulation conditions and have relations with the wastewater characteristics, doses and effluent water quality.
Figure 2. Graph showing the load changes in the Frogn WWTP during normal days and in rainy days. The images represent the typical floc structures for those particular days when the image analysis installation was functioning. Numbers within the each image mean the corresponding coagulant dosages in μl/l.
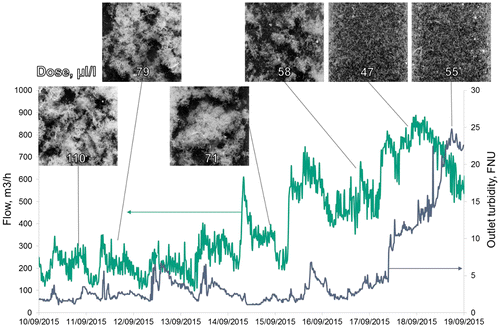
3.2. Principal component analysis
Previously obtained in the laboratory scale results, proved that the images of flocs are unique for the different water conditions and the texture analysis methods have a potential to be used for the further floc sensor development. Principal component analysis (PCA) applied to the full-scale data showed that the images, obtained from different inlet wastewater parameters and coagulation conditions, also contain unique information.
Figure (a) shows the results of PCA based only on 9 GLCM textural features of the images of flocs. With four principal components (PCs) the total explained variance equals 96.3% (PC1 = 57.4%, PC2 = 78.5%, PC3 = 91.5%) for calibration and 91.2% (PC1 = 49.4%, PC2 = 68.2%, PC3 = 85.2%) for cross-validation. The images of flocs by means of GLCM feature vectors separated samples on PC1 versus PC2 scores plot into three distinct classes. These classes match the wastewater coagulation conditions: normal, during the rain events and the high inlet turbidity. Thus, the images of flocs can be used as an early indication of the changes in the wastewater parameters to take further manual (or automated) troubleshooting action.
Figure 3. Scores plot of PCA, PC1 vs. PC2 for: (a) data with 9 GLCM textural features of the images of flocs; (b) data containing wastewater quality parameters, GLCM textural features and the coagulant dosage.
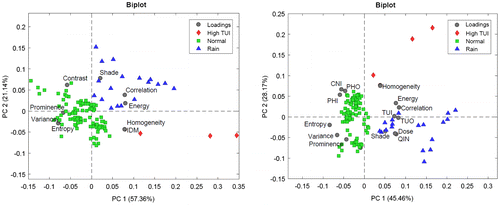
Figure (b) shows the results of PCA performed for all inlet and outlet wastewater quality parameters (QIN, TUI, PHI, CNI, PHO, TUO), GLCM textural features (9 variables) and the coagulant dosage in ml/s. It was done in order to compare how different would be the scores plot from the one described above. However, no huge differences can be observed in means of samples’ classes. Samples corresponding to the rain events are more stretched by PC2, which associates with high turbidity inlet and outlet, high wastewater flow and coagulant dosage. Total explained variance for calibration: PC1 = 45.5%, PC2 = 73.6%, PC3 = 83.4%, PC4 = 89.6%; for cross-validation: PC1 = 38.3%, PC2 = 66.9%, PC3 = 76.9%, PC4 = 83.3%.
3.3. Floc texture index (FTI)
Figure shows the potential for the sensor prototype to be developed. However, in order to have the instrument functioning as a sensor, preferably, there should be only one signal coming out from the sensor. Thus, 9 GLCM feature vectors should be reduced to one variable. We are introducing the entirely new term—floc texture index (FTI). FTI is a sum of four GLCM feature vectors:(1)
(1)
The other GLCM feature vectors were excluded from the equation, because they have very high (near 1) correlation coefficients to some of the included variables, what can be seen from the loadings in Figure . Since Variance has values in hundred scale, FTI was divided by 100 for simplification. The results of FTI calculation for 3 sample classes are presented in Table .
Table 2. FTI ranges for 3 classes in the data-set
The calculated FTIs and corresponding observed outlet turbidity values are presented in Figure . Frogn WWTP aims to keep the effluent turbidity (TUO) below 5 FNU. Hence, TUO values below 5 FNU are marked as orange triangles, while the values above 5 FNU are marked as orange open triangles. The lower limit for FTI was chosen to be 6. While FTI is above 6, the corresponding TUO in most cases is below 5 FNU, and vice versa. Three events are highlighted in the figure. The first red dashed box highlights the rain event. The images of flocs quantified as FTIs showed an early indication that the outlet turbidity would exceed the maximum desired level. Even though the TUO values were shifted in the data-set to correspond the inlet wastewater quality and dosed amount of coagulant, the flow through the treatment plant is a dynamic system and not an ideal plug flow, so sometimes the time lag between a flocculation chamber and an outlet from the sedimentation tank is higher than 45 min. In such cases, the early indication of the changes in coagulation conditions by the images of flocs (FTI) is desired and a significant advantage of the planned dosage control system. The 2a and 2b red dashed boxes highlight the events when coagulant dosages were not optimal, which resulted in the increase of effluent turbidity. The third event highlighted by the red dashed box corresponds to the high inlet turbidity. Overall, FTI was able to indicate all non-optimal conditions of the coagulation process in this particular data-set.
With a bigger training data-set, it is potentially possible that FTI will have lower and higher limits to indicate changes in coagulation conditions and/or take action in raising or lowering the coagulant dosage.
3.4. Effluent turbidity prediction
Different PLSR models were tested in order to get the best prediction of the effluent turbidity based on inlet wastewater quality parameters and images of flocs. Nowadays DOSCON® is using a multi-parameter based feed-forward control strategy (Manamperuma et al., Citation2017). To strengthen the robustness and wider applicability of the dosage control system, the soft sensor should be developed to predict the effluent turbidity. If the operator of the plant knows few hours in advance that there is a potential danger the outlet turbidity will exceed the maximum desired value, he has enough time to take action and justify the coagulant dosage. Ideally, such system is to be developed to the self-standing dosage control strategy.
The models with highest explained variances are presented in Table . The response (Y) for all models was the effluent turbidity (TUO), while the X-matrix consisted of different variables. The prediction by only wastewater inlet parameters (QIN, TUI, CNI, PHI, Dose) resulted in 80.5% calibration Y variance explained by two factors. The simplest model based on inlet parameters QIN and TUI resulted in 79.7% calibration Y variance explained by one factor. With the addition of image analysis results (FTI), the calibration R2 increased until 0.84. The best prediction model for TUO, about 87% calibration Y variance explained by two factors, included both inlet wastewater quality parameters (QIN and TUI), and some GLCM feature vectors (Variance, Prominence, Correlation and Contrast).
Table 3. Results of partial least squares regressions for the effluent turbidity prediction
Even though the addition of FTI or GLCM variables to the models resulted in 4 and 7% increase of explained Y variance respectively, the main advantage of the image analysis supported prediction is a better estimation of the over ranged outlet turbidity values (more than 5 FNU). Figure shows a comparison of two TUO estimation models. The first model (Figure (a)) is based only on wastewater inlet parameters and tend to underestimate TUO, which is acceptable for the TUO values below 5 FNU and close to it. However, there are two samples with considerably high TUO (over 10 FNU), which were underestimated by the model (predicted values lower than 5 FNU). The addition of the GLCM feature vectors (Variance, Prominence, Correlation and Contrast) as predictor variables increased the efficiency of estimation and resulted in the lower amount of underestimated effluent turbidity values.
3.5. Difficulties and further research needs
Some weaknesses of the described image analysis installation are that the employed camera is quite expensive and difficult to be properly controlled. Also, it has the battery charge limit, since cannot be charged directly from the electrical plug. The system was not able to work continuously, and this is the main reasons the test data-set resulted in a quite low number of samples. In addition, the resulting images have a high resolution but require quite much space for the storage. Currently, all the calculations of GLCM features and FTIs are done in an external computer. However, we see the potential for the sensor prototype to be further developed. In the next stage, it should be a self-standing computational system with a cheaper camera, which can constantly work with the pre-set settings.
The further research studies are necessary to develop the fully automated floc sensor prototype. The concept of the system will be based on automated flocs image acquisition and its texture image analysis with further matching of the resulted data to different mathematical models, defining the optimal coagulant dose. The improvement of existing on-line dosage control system is a key focus of this research.
4. Conclusions
The images of flocs give a sharp indication of the changes of inlet wastewater parameters and/or coagulation conditions.
The images of flocs are unique for different wastewater qualities and coagulation conditions. GLCM textural features (quantified images of flocs) can distinguish and separate different wastewater coagulation conditions: normal, during the precipitation events, wastewater with the high inlet turbidity.
Floc texture index was introduced and calculated by summarising four GLCM feature vectors—Contrast, Entropy, Homogeneity and Variance. FTI can be used as an early indication parameter of the changes in wastewater qualities and coagulation conditions, which lead to the increase of effluent turbidity. However, further research is needed with the bigger calibration and validation data sets.
Effluent turbidity values can be predicted by few inlet wastewater parameters—flow and inlet turbidity, with R2 = 0.79. The addition of processed flocs’ images data increases the outlet turbidity prediction up till R2 = 0.87.
The study shows a potential possibility of the floc sensor prototype to be developed. The images of flocs may be used online for troubleshooting and to improve the existing coagulant dosage control system.
List of abbreviations | ||
ANN | = | Artificial neural networks |
CNI | = | Inlet conductivity |
DIA | = | Digital image analysis |
DWTP | = | Drinking water treatment plant |
FNU | = | Formazin nephelometric unit |
FTI | = | Floc texture index |
GLCM | = | Grey level co-occurance matrix |
IDM | = | Inverse difference moment |
NTMS | = | Nephelometric turbidimeter monitoring system |
PC | = | Principal component |
PCA | = | Principal component analysis |
PDA | = | Photometric dispersion analyser |
PHI | = | Inlet pH |
PHO | = | pH after coagulant dosage |
PLSR | = | Partial least squares regression |
QIN | = | Inlet flow rate |
RMSEC | = | Root mean square error of calibration |
RMSECV | = | Root mean square error of cross-validation |
SCADA | = | Supervisory control and data acquisition |
SCD | = | Streaming current detector |
Total P | = | Total Phosphorous |
TUI | = | Inlet turbidity |
TUO | = | Outlet turbidity |
WWTP | = | Wastewater treatment plant |
Funding
Authors gratefully acknowledge the financial support from the IMPREC project funded by the Polish-Norwegian Research Fund and the Water Harmony project funded by SIU.no and Ministry of foreign affairs of Norway.
Acknowledgment
Authors would like to thank the head of the Frogn WWTP – Jan Erik Andersen for the support in conducting experiments in the plant and for the access to data.
Additional information
Notes on contributors
N. Sivchenko
The authors are members of Water, Environment, Sanitation and Health (WESH) research group at the Norwegian University of Life Sciences (NMBU), Faculty of Sciences and Technology (RealTek). WESH group focuses on water and wastewater related issues and is heavily involved in teaching and supervision of MSc and PhD programs in water engineering and technology. The group also has one of the largest externally funded research and educational project portfolios at RealTek, and collaborates with partners from EU, North America, Eurasia, Asia and Africa. It also has a number of Research, Development and Innovation projects with Norwegian partners. The main research and development areas of the WESH group are: process control and optimization of coagulation and biological treatment processes; membrane fouling and filtration processes; microbial water quality and risk assessment; decentralized wastewater systems; modelling of sewer systems.
References
- Bache, D., & Gregory, R. (2007). Flocs in water treatment. London: IWA Publishing.
- Baxter, C. W., Shariff, R., Stanley, S. J., Smith, D. W., Zhang, Q., & Saumer, E. D. (2002). Model-based advanced process control of coagulation. Water Science and Technology, 45, 9–17.
- Bouyer, D., Coufort, C., Liné, A., & Do-Quang, Z. (2005). Experimental analysis of floc size distributions in a 1-L jar under different hydrodynamics and physicochemical conditions. Journal of Colloid and Interface Science, 292, 413–428. doi:10.1016/j.jcis.2005.06.011
- Burgess, M., Curley, J., Wiseman, N., & Xiao, H. (2002). On-line optical determination of floc size. Part I: Principles and techniques. Journal of Pulp and Paper, 28, 63–65.
- Chakraborti, R. K., Atkinson, J. F., & Van Benschoten, J. E. (2000). Characterization of alum floc by image analysis. Environmental Science & Technology, 34, 3969–3976. doi:10.1021/es990818o
- Chang, Y., Liu, Q.-J., & Zhang, J.-S. (2005). Flocculation control study based on fractal theory. Journal of Zhejiang University Science B, 6B, 1038–1044. doi:10.1631/jzus.2005.B1038
- Cheng, W. P., Chang, J. N., Chen, P. H., Yu, R. F., & Huang, Y. W. (2011). Turbidity fluctuation as a measure of floc size in a coagulation pilot study. Desalination and Water Treatment, 30, 98–104. doi:10.5004/dwt.2011.1878
- Cheng, W. P., Kao, Y. P., & Yu, R. F. (2008). A novel method for on-line evaluation of floc size in coagulation process. Water Research, 42, 2691–2697. doi:10.1016/j.watres.2008.01.032
- Chou, S., Lin, S., & Huang, C. (1998). Application of optical monitor to evaluate the coagulation of pulp wastewater. Water Science and Technology. doi:10.1016/S0273-1223(98)00343-6
- Conners, R. W., Trivedi, M. M., & Harlow, C. A. (1984). Segmentation of a high-resolution urban scene using texture operators. Computer Vision, Graphics, and Image Processing, 25, 273–310. doi:10.1016/0734-189X(84)90197-X
- Critchley, R., Smith, E., & Pettit, P. (1990). Automatic coagulation control at water-treatment plants in the North-West region of England. Water and Environment Journal, 4, 535–543.10.1111/wej.1990.4.issue-6
- Dentel, S. K., Thomas, A. V., & Kingery, K. M. (1989a). Evaluation of the streaming current detector-I. Use in jar tests. Water Research, 23, 413–421. doi:10.1016/0043-1354(89)90132-2
- Dentel, S. K., Thomas, A. V., & Kingery, K. M. (1989b). Evaluation of the streaming current detector-II. Continuous flow tests. Water Research, 23, 423–430. doi:10.1016/0043-1354(89)90133-4
- Haralick, R. M., Shanmugam, K., & Dinstein, I. (1973). Textural features for image classification. IEEE Transactions on Systems, Man, and Cybernetics, 3, 610–621. doi:10.1109/TSMC.1973.4309314
- He, W., Nan, J., Li, H., & Li, S. (2012). Characteristic analysis on temporal evolution of floc size and structure in low-shear flow. Water Research, 46, 509–520. doi:10.1016/j.watres.2011.11.040
- Jarvis, P., Jefferson, B., Gregory, J., & Parsons, S. A. (2005). A review of floc strength and breakage. Water Research, 39, 3121–3137.10.1016/j.watres.2005.05.022
- Juntunen, P., Liukkonen, M., Lehtola, M., & Hiltunen, Y. (2013). Dynamic soft sensors for detecting factors affecting turbidity in drinking water. Journal of Hydroinformatics, 15, 416–426.10.2166/hydro.2012.052
- Juntunen, P., Liukkonen, M., Lehtola, M., & Hiltunen, Y. (2014). Characterization of alum floc by image analysis in water treatment processes. Cogent Engineering, 1, 944767.
- Li, T., Zhu, Z., Wang, D., Yao, C., & Tang, H. (2006). Characterization of floc size, strength and structure under various coagulation mechanisms. Powder Technology, 168, 104–110. doi:10.1016/j.powtec.2006.07.003
- Liu, W. (2016). Enhancement of coagulant dosing control in water and wastewater treatment process (Doctoral dissertation). Norwegian University of Life Sciences, Ås.
- Liu, W., & Ratnaweera, H. (2017). Feed-forward-based software sensor for outlet turbidity of coagulation process considering plug flow condition. International Journal of Environmental Science and Technology, 14, 1689–1696.
- Liu, W., Ratnaweera, H., & Song, H. (2013, September 18–20). Better treatment efficiencies and process economies with real-time coagulant dosing control. In 11th IWA Conference on Instrumentation Control and Automation. Narbonne.
- Manamperuma, L., Ratnaweera, H. C., & Rathnaweera, S. S. (2013, September 18–20). Retrofitting coagulant dosing control using real-time water quality measurements to reduce coagulant consumption. In 11th IWA Conference on Instrumentation Control and Automation. Narbonne.
- Manamperuma, L., Wei, L., & Ratnaweera, H. (2017). Multi-parameter based coagulant dosing control. Water Science and Technology, 75, 2157–2162 http://doi.org/org/10.2166/wst.2017.058
- Rasband, W. S. (1997/2016). ImageJ, US National Institutes of Health, Bethesda, MD. Retrieved from https://imagej.nih.gov/ij/
- Ratnaweera, H., & Fettig, J. (2015). State of the art of online monitoring and control of the coagulation process. Water, 7, 6574–6597. doi:10.3390/w7116574
- Sharp, E., Banks, J., Billica, J., Gertig, K., Henderson, R., Parsons, S. A., … Jefferson, B. (2005). Application of zeta potential measurements for coagulation control: Pilot-plant experiences from UK and US waters with elevated organics. Water Science and Technology: Water Supply, 5, 49–56.
- Sharp, E., Rigby, R., Hughes, R., Claronino, J., Maher, K., Patel, K., … Jefferson, B. (2016, June 22–24). Using online zeta potential measurements for full-scale coagulation control in drinking water treatment. In IWA Specialist Conference, Advances in particle science and separation: Meeting tomorrow’s challenges. Oslo.
- Sivchenko, N., Kvaal, K., & Ratnaweera, H. (2014, June 15–18). Image analysis of flocs and mathematical modelling applied to coagulation-flocculation process. In Poster, IWA Specialist Conference, Advances in particle science and separation: From mm to nm scale and beyond. Sapporo.
- Sivchenko, N., Kvaal, K., & Ratnaweera, H. (2016). Evaluation of image texture recognition techniques in application to wastewater coagulation. Cogent Engineering, 3(1). doi:10.1080/23311916.2016.1206679
- VA-Support. (2012). DOSCON ‐ Experience at NRA. (03.07.2012).
- Vahedi, A., & Gorczyca, B. (2011). Application of fractal dimensions to study the structure of flocs formed in lime softening process. Water Research, 45, 545–556. doi:10.1016/j.watres.2010.09.014
- Valentin, N., & Denœux, T. (2001). A neural network-based software sensor for coagulation control in a water treatment plant. Intelligent Data Analysis, 5, 23–39.
- Vlieghe, M., Coufort-Saudejaud, C., Frances, C., & Liné, A. (2014). In situ characterization of floc morphology by image analysis in a turbulent Taylor-Couette reactor. American Institute of Chemical Engineers, 60, 2389–2403. https://doi.org/DOI 10.1002/aic
- Wu, C., Wang, L., Hu, B., & Ye, J. (2013). Influential factors of formation kinetics of flocs produced by water treatment coagulants. Journal of Environmental Sciences, 25, 1015–1022. doi:10.1016/S1001-0742(12)60150-8
- Xu, Y., Chen, T., Cui, F., & Shi, W. (2016). Effect of reused alum-humic-flocs on coagulation performance and floc characteristics formed by aluminum salt coagulants in humic-acid water. Chemical Engineering Journal, 287, 225–232. doi:10.1016/j.cej.2015.11.017
- Yavich, A. A., & Van De Wege, J. (2013). Chemical feed control using coagulation computer models and a streaming current detector. Water Science & Technology, 67, 2814. doi:10.2166/wst.2013.198
- Yu, R., Chen, H., & Cheng, W. (2017). Applying online image analysis to simultaneously evaluate the removals of suspended solids and color from textile wastewater in chemical flocculated sedimentation. Journal of Environmental Informatics, 29(1).
- Yu, R. F. (2014). On-line evaluating the SS removals for chemical coagulation using digital image analysis and artificial neural networks. International Journal of Environmental Science and Technology, 11, 1817–1826. doi:10.1007/s13762-014-0657-1
- Zheng, C., Sun, D.-W., & Zheng, L. (2006). Recent applications of image texture for evaluation of food qualities—a review. Trends in Food Science & Technology, 17, 113–128. doi:10.1016/j.tifs.2005.11.006