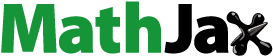
Abstract
In this paper, we report the optimization and development of predictive models for the corrosion inhibition of mild steel in sulphuric acid by Methyl-5-benzoyl-2-benzimidazole Carbamate (Mebendazole). Design expert was used to analyze the corrosion inhibition related process parameters, such as corrosion penetration rate, inhibition efficiency, inhibitor concentration, acid concentration, weight loss, and their relationships. An attempt is made to obtain the optimal settings for this corrosion inhibition related process parameters. Design methodology, weight loss measurement, open-circuit potential analysis, Tafel polarization, etc. were used for the evaluation of inhibition efficiency of mebendazole for mild steel in H2SO4. The corrosion inhibition process parameters were optimized and predictive mathematical models developed using the Response Surface Methodology (RSM) using the central composite design (CCD) tool of Design Expert software version 11. Experimental and theoretical corrosion inhibition parameters were used to develop a nonlinear regression model to predict the optimal inhibition efficiency. Also, a quadratic model was generated, with predicted optimum inhibition efficiency of 88.4095% obtained having very near unity desirability of 0.914. The developed model shows that inhibition efficiency is related to the inhibitor concentration, immersion time, and acid concentration. The regression models generated are successfully used to predict the corrosion inhibition behaviour of mebendazole for low carbon steel in sulphuric acid medium.
PUBLIC INTEREST STATEMENT
The economic impact and problems posed by metallic corrosion has drawn strong attention from researchers and engineers worldwide. Corrosion of mild steel is a major concern in chemical processing and metallurgical plants, oil and gas industry, manufacturing industries, marine operations, boiler plants, and power generation plants. High cost is associated with the replacement of metallic parts in these applications. The consequence often leads to plant shutdowns, breakdown of industrial equipment, reduced efficiency, industrial downtime, high maintenance cost due to replacement of damaged part, wastage of valuable resources, and expensive overdesign. Corrosion inhibition is of great practical importance, being extensively employed in curtailing wastage of engineering materials and minimizing costs of corrosion control.
1. Introduction
Mild steel finds wide application in various industries due to its low cost, availability, and its amenable to various fabrication techniques (Akintola et al., Citation2019; Odoni, Edoziuno, & Chukwurah, Citation2017; Ofuyekpone, Akaluzia, & Edibo, Citation2017; Olawale, Adediran, Talabi, Nwokocha, & Ameh, Citation2017). Mild steel corrosion in acidic environment occurs due to an electrochemical reaction with the service environment (Alasvand Zarasvand & Rai, Citation2014; Fiori-Bimbi, Alvarez, Vaca, & Gervasi, Citation2015; Loto, Popoola, Fayomi, & Loto, Citation2012; Uhlig & King, Citation1972). To prolong the design and service life span of mild steel, effective corrosion protection techniques, such as the use of corrosion inhibitors, are required (Arthur, Jonathan, Ameh, & Anya, Citation2013; Gece, Citation2011; Patni, Agarwal, & Shah, Citation2013; Rani & Basu, Citation2012).
Response surface methodology (RSM) is a statistical and experimental design tool in which a selected dependent variable responds to variations in one or more independent or factor variables (Adesina, Fatoba, Farotade, & Adediran, Citation2017; Kothari & Garg, Citation2014, Mohammed, Abakr, Yusup, & Kazi, Citation2016). It is a useful technique for predictive modelling and optimization of the corrosion inhibition properties (Kothari & Garg, Citation2014). The aim is to optimize the response of the independent variable (output) which is also affected by varying the design settings for one or more independent variables (inputs) (Kothari & Garg, Citation2014). Many researchers on corrosion inhibition have applied experimental design software packages and techniques like the RSM in optimizing the corrosion inhibition process parameters and predicting their responses (Anadebe, Onukwuli, Omotioma, & Okafor, Citation2018; Evelin, Rodriguez, Borbolla, Alvarado-Rodriguez, & Pandiyan, Citation2016; Okafor, Anadebe, & Onukwuli, Citation2019; Omotioma & Onukwuli, Citation2016; Onukwuli, Nwanekezie, Anadebe, & Omotioma, Citation2017; Onukwuli & Omotioma, Citation2016; Praveen, Manjunatha, Gaurav, & Mohammed, Citation2016). The results of gravimetric measurement for the corrosion inhibition study of Sapium ellipticum leaf extract was modelled and a regression model was generated for predicting its inhibition efficiency (IE) at optimal combination of corrosion inhibition factors (Okafor et al., Citation2019). Anadebe et al. (Citation2018) carried out an optimization study on the use of ethanol extract of bitter kola leaf as corrosion inhibitor for mild steel in HCl solution. RSM was utilized to predict the optimum range of parameters for controlling corrosion and hence reduced the number of experimental runs. Bitter kola leaf extract was also used to inhibit corrosion of mild steel in H2SO4 by Onukwuli et al. (Citation2017), and the results was optimized and modelled using the central composite design (CCD) and RSM of design expert software. A quadratic regression model was generated that adequately explained the relationship between IE and the considered factors of the corrosion inhibition process. Evelin et al. (Citation2016) optimized and developed predictive model for the corrosion inhibition of carbon steel in acidic service by imidazole and benzimidazole derivatives. Multiple linear regression models were obtained using the Moby Digs software. The model shows a quantitative structure–property relationship with descriptors that contribute to predict the corrosion IE of the imidazole and benzimidazole derivatives. The predictive model obtained indicates that IE of the inhibitors is related to the molecule volume charge, electronegativity, and aromaticity. Values of IE agree for both model proposed and experimental results, which indicates that theoretical model can be used to predict experimental results adequately. Omotioma and Onukwuli (Citation2016) modelled and optimized the IE of the extract of pawpaw leaves for mild steel in HCl using RSM. The analysis of the experimental results by RSM shows the simultaneous dependency of weight loss (WL), corrosion rate (CR), and IE on inhibitor concentration, temperature, and time. A quadratic model was generated with optimum IE of 80.29%. Onukwuli and Omotioma (Citation2016) also modelled and optimized the IE of mango extract using RSM and found that IE of the extract was concentration-, temperature-, and time dependent. Odejobi and Akinbulumo (Citation2019) carried out modelling and optimization of the IE of Euphorbia heterophylla extract for mild steel in HCl media using the CCD of RSM. 89.8% IE was obtained at optimum combination of the extract concentration, acid concentration, temperature, and immersion time.
This study is aimed at optimizing the IE of Methyl-5-benzoyl-2-benzimidazole Carbamate (Mebendazole; MBZ) as a suitable corrosion inhibitor for mild steel in H2SO4 and generating mathematical models for predicting the IE in the acid medium. It is important to examine the optimal inhibitive property of mebendazole and develop mathematical regression models for predicting the corrosion inhibition properties.
2. Experimental methods
2.1. Data collection
The materials used for this design optimization and modelling work were collected from experimentally generated data. The experimental corrosion data were obtained by both gravimetric (WL) and electrochemical techniques (potentiodynamic/Tafel polarization) (Edoziuno & Odoni, Citation2018). These techniques were used to evaluate the effectiveness of various concentrations of Methyl-5-benzoyl-2-benzimidazole Carbamate (0.5, 1.0, 1.5, 2.0, and 2.5 g) in inhibiting the corrosion of mild steel in 0.5, 1.0, and 1.5 M solutions of H2SO4 at room temperature (298 K) and elevated temperature (333 K), respectively.
For the weight-loss measurements, mild steel test coupons with average dimensions of 15 mm thickness, 10 mm length, and a 5 mm hole machined at the centre for suspension were weighed and completely submerged by suspension in each of the prepared test media contained in a 100-ml beaker with cover for different immersion period of 24, 48, 72, 96, and 120 h with and without MBZ inhibitor addition. They were taken out after the required exposure time had elapsed. The corrosion products were removed by washing with distilled water, rinsing in acetone, and drying in ambient air. The coupons were then re-weighed to determine their final weights. The WL are then computed and recorded.
The result data for the potentiodynamic polarization was obtained using an autolab potentiostat/galvanostat, VersaSTAT 4 Electrochemical System, controlled from a suitably equipped PC by a Universal Serial Bus (USB) interface using the Versa Studio electrochemistry software package. A conventional three-electrode Pyrex glass cell was used for each of the experiments. Test coupons encapsulated in a polyester resin with total area of 1 cm2 exposed to the corrosive media were used as working electrode and a platinum rod as counter electrode. Ag/AgCl rod was the reference electrode. These electrodes were connected by means of a luggin capillary to the electrolytic cell. All electrochemical tests were performed in stagnant aerated solutions at room temperature and 60°C. The test coupon was submersed in the test solution for one hour to attain an equilibrium open circuit potential. The potentiodynamic polarization test was maintained between a potential range of −250 mV and +250 mV with respect to the corrosion potential using linear sweep technique at a scan rate of 1 mV/s. To obtain the corrosion current density (icorr) values, the linear Tafel segments of the anodic and cathodic curves were extrapolated to equilibrium corrosion potential. The polarization experiment was carried out in the blank sulphuric acid solution and the solutions containing known amount of the inhibitor.
2.2. Optimization of inhibition efficiency and other corrosion properties using RSM
Employing RSM, the IE and other experimental corrosion properties were modelled and optimized based on CCD tool of Design Expert (DoE) software version 11. A quadratic design model was applied during the analysis. Inhibitor concentration (0.5–2.5 g/100 ml), acid concentration (0.5–1.5 M), and immersion time (24–120 h) were set as the independent variables (Factors 1, 2, and 3), while WL, CR, and IE were set as the response variables (Responses 1–3). A total of 90 runs of experiments were carried out to obtain the responses of the dependent variables shown in the design layout (Table ).
Table 1. Actual design layout
3. Results and discussion
3.1. Results of RSM analysis
Figures and are representative output showing the variations in the analysis of variance (ANOVA), predictive modelling, and optimization results. The results indicated that the WL, corrosion penetration rate, and IE are functions of inhibitor concentration, acid concentration, and immersion time. As observed in Figure , a linear trend was noticeable in the diagnostics plots of predicted IE against the actual IE. A similar trend was noticed in the same plots of predicted CR against the actual CR and predicted versus actual WL. These trends show that the design model is adequate to predict the IE of MBZ, the corrosion penetration rate and the WL of mild steel in the inhibited environment. The predicted versus actual values of the IE, corrosion penetration rate, and WL were highly correlated. Surface plots are very useful in understanding the behaviour of chosen output/response variable, when independent variables/factors are varied within their respective ranges or targets considered in the experimental design. Two factors are normally controlled concurrently while automatically keeping the remaining factors at their respective optimal values. The 3-D surface plot (Figure –c) showed the relationship between the independent factors (inhibitor concentration and acid concentration) and IE of mebendazole, corrosion penetration rate of the inhibited solution on mild steel and the WL of mild steel in the inhibited environment. The nature of the 3-D surface plots suggests significant interactions among the concerned factors and response variables during the corrosion protection process by the MBZ inhibitor. The summary of the design parameters are contained in Table .
Figure 1. (a) Diagnostic plot of predicted versus actual inhibition efficiency. (b) Diagnostic plot of predicted versus actual corrosion rate. (c) Diagnostic plot of predicted versus actual weight loss
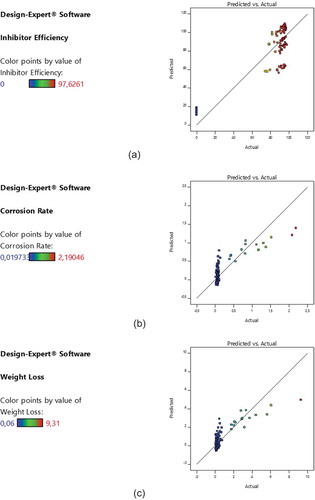
Figure 2. (a) 3-D surface plot of inhibition efficiency. (b) 3-D surface plot of corrosion rate. (c) 3-D surface plot of weight loss
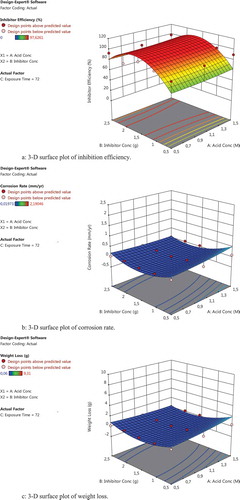
Table 2. (a & b) The summary of the design parameters for both the factors and response variables
3.2. Mathematical model
The experimental data collected with regard to CCD is utilized in developing nonlinear mathematical models that indicate the responses of the dependent variables at various levels of the factors. Final predictive model equations of IE of MBZ, CR, and WL as a function of the considered factors both in terms of coded and actual factors were generated. The quadratic model with combined significant and insignificant terms is presented in EquationEquations (1)1
1 –(Equation3
3
3 ), respectively. The regression models relates the IE, corrosion penetration rate, and WL with acid concentration (A), inhibitor concentration (B), and exposure time (C), respectively. A nonlinear, regression model sufficiently described the relationship between the dependent/response variables; IE, CR, WL, and the independent factors; acid concentration (A), inhibitor concentration (B), and exposure time (C), respectively. The predictive equations can be expressed both in terms of coded factors and actual factors. When the model equations are expressed in terms of coded factors they are useful in making predictions about the responses of the dependent variables for specified levels of each factor. By default, the high levels of the factors are given positive coding as +1, on the other hand the low levels of the factors are negatively coded as −1. The usefulness of the coded equation lies in the identification of the relative impact of the factors through comparison of the coefficients of the factor. Positive and negative factor coefficients respectively indicate maximum positive and negative impact of the factors to the response of the variable. Conversely, the mathematical models expressed in terms of actual factors can be used to make predictions about the response for given levels of each factor. Here, the levels should be specified in the original units for each factor. It is noteworthy that the model equation given in terms of the actual factors should not be used to ascertain the relative impact of each factor because the coefficients in this case are scaled to accommodate the units of each factor and the intercept is not at the centre of the design space. The generated regression models are significant and can be used to navigate the design space.
Final Mathematical Models for Inhibitor Efficiency Expressed Based on the Coded Factors (EquationEquation (1)1
1 ).
Final Mathematical Models for Corrosion Rate Expressed Based on the Coded Factors (Equation (2)).
Final Mathematical Models for Weight Loss Expressed Based on the Coded Factors (Equation (3)).
3.3. Statistical test and analysis of models
The CCD generated nonlinear mathematical models showing the levels of relationship between the response variables and various factors were tested to ascertain their statistical adequacy, practical usefulness and significance with the aid of ANOVA test. The statistical test (significance, ANOVA, coefficient of determination, and prediction accuracy) and model analysis (surface plots) were carried out in order to provide complete insight into the corrosion inhibition process (Chate, Manjunath, Deshpande, & Parappagoudar, Citation2017). The statistical analysis of the data is presented in Tables –. Factor coding used for the ANOVA test for all the response parameters is coded, while the Sum of squares is Type III—Partial.
Table 3. ANOVA results for the response parameter (inhibition efficiency)
Table 4. Fit statistics for the response parameter considered (inhibitor efficiency)
Table 5. ANOVA results for the response parameter (corrosion rate)
Table 6. Fit statistics for the response parameter considered (corrosion rate)
Table 7. ANOVA results for the response parameter (weight loss)
Table 8. Fit statistics for the response parameter considered (weight loss)
The Model F-value of 37.03 obtained as shown in Table is a significant model as there is only a 0.01% likelihood that an F-value that is this large could occur as a result of noise. The source of noise may be attributed to non-controllable factors of the inhibition process. On the other hand, P-values below 0.0500 is an indication of significant model terms. From the ANOVA results in Table , B, and B2 are significant model terms. P-values above 0.1000 is indicative of insignificant model terms. When there are many model terms that are not significant in a predictive model equation (excluding those model terms required to support hierarchy), model reduction in the analysis settings is recommended for the improving the model.
The F-value of this model presented in Table (20.02) is a significant model, because there is only a 0.01% possibility that an F-value of this magnitude could be obtained from the model owing to noise. Significant model terms are usually associated with P-values that are less than 0.0500. In the case of this response model, the significant model terms are B, AB, BC, and B2. Conversely, P-values higher than 0.1000 are used to indicate the insignificant model terms.
The F-value of the model in Table (19.06) is a significant model since there is only a 0.01% likelihood that such large F-value could be obtained due to noise. P-values below 0.0500 indicate significant model terms. Thus, B, C, AB, BC, and B2 are significant model terms in the WL mathematical model. P-values that are higher than 0.1000 show insignificant model terms. When there are many insignificant model terms in a response model (excluding those model terms required to support hierarchy), applying model reduction technique may enhance the developed model.
The R2 values predicted (Tables , and ) reasonably agreed with the Adjusted R2 values, since their differences are less than 0.2, respectively. The R2 are close to unity, thus the fitted models are considered to be good ones (Kothari & Garg, Citation2014).
Adeq Precision is a useful measure of the signal to noise ratio. A ratio that is above 4 is adequate and desirable. The ratio of 18.338, 18.311, and 18.469 obtained in Tables , and , respectively, indicates an adequate signal. Hence these models can be satisfactorily used to navigate the design space (Saha & Banerjee, Citation2013).
3.4. Optimization results
Twenty one optimized solutions (Table ) for IE (%), CR, and WL respectively were obtained for various predicted optimal levels of immersion time (h) acid concentration and inhibitor concentration (g/100 ml), respectively. One automatically selected optimized solution having a near unity desirability (0.914) (with optimum IE of 88.41% was achieved at optimal acid concentration of 0.5 M H2SO4, inhibitor concentration of 0.957 g/100 ml and immersion duration of 56.363 h) is depicted in the overlay plot (Figure ).
Table 9. Optimization solutions
4. Conclusions
A quadratic model was generated, with high IE of 88.4095%. The inhibitor was effective on the surface treatment of mild steel in acidic environment. It is therefore recommended that mebendazole be utilized as corrosion inhibitor in oil well acidizing. Additionally, it can serve in an industrial acid pickling and cleaning of other related surface treatment of mild steel in acidic environment. The quadratic predictive model equations adequately explains the relationship among; WL, CR, IE of MBZ and the considered independent factors of the inhibition process in the operating environment.
Acknowledgements
The authors acknowledge the financial support provided by the Tertiary Education Trust Fund (TETFund), Nigeria through the Institution Based Research (IBR) Interventions for the research. A.A.A acknowledge the support from Landmark University Centre for Research, Innovation and Development (LUCRID).
Additional information
Funding
Notes on contributors
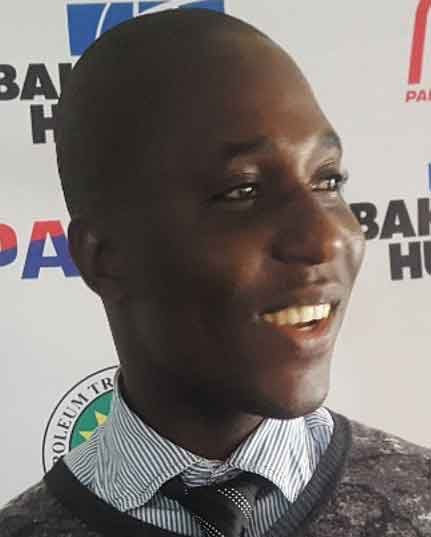
F. O. Edoziuno
Francis O. Edoziuno is presently a lecturer in the Department of Metallurgical Engineering, Delta State Polytechnic, Ogwashi-Uku, Nigeria. His research on corrosion phenomena aims to proffer solutions to the current depreciating effect of metallic degradation and failure in service in various engineering and industrial applications.
References
- Adesina, O. S., Fatoba, O. S., Farotade, G. A., & Adediran, A. A. (2017). Modelling and optimization of laser alloyed AISI 422 stainless steel using taguchi approach and response surface model (RSM). Current Journal of Applied Science and Technology, 23(3), 1–19. doi:10.9734/CJAST/2017/24512
- Akintola, S. A., Oki, M., Adediran, A. A., Akpor, O. B., Oluba, O. M., Ogunsemi, B. T., & Ikubanni, P. P. (2019). Valorized chicken feather as corrosion inhibior for mild steel in drilling mud. Results in Engineering, 4, 100026. doi:10.j.rineng.2019.100026
- Alasvand Zarasvand, K., & Rai, V. R. (2014). Microorganisms: Induction and inhibition of corrosion in metals. International Biodeterioration and Biodegradation, 87, 66–74. doi:10.1016/j.ibiod.2013.10.023
- Anadebe, V. C., Onukwuli, O. D., Omotioma, M., & Okafor, N. A. (2018). Optimization and electrochemical study on the control of mild steel corrosion in hydrochloric acid solution with bitter kola leaf extract as inhibitor. South African Journal of Chemistry, 71, 51–61. doi:10.17159/0379-4350/2018/v71a7
- Arthur, D. E., Jonathan, A., Ameh, P. O., & Anya, C. (2013). A review on the assessment of polymeric materials used as corrosion inhibitor of metals and alloys. International Journal of Industrial Chemistry, 4, 2. doi:10.1186/2228-5547-4-2
- Chate, G. R., Manjunath, P. G. C., Deshpande, A. S., & Parappagoudar, M. B. (2017). Modeling and optimization of furan molding sand system using design of experiments and particle swarm optimization. Journal of Process Mechanical Engineering, 1–20. doi:10.1177/0954408917728636
- Edoziuno, F. O., & Odoni, B. U. (2018). Corrosion inhibition studies of mebendazole (MBZ) for mild steel in sulphuric acid. 1. doi:10.17632/GBRFKZ2W2J.1
- Evelin, G., Rodriguez, J. A., Borbolla, J. C., Alvarado-Rodriguez, J. G., & Pandiyan, T. (2016). Development of a predictive model for corrosion inhibition of carbon steel by imidazole and benzimidazole derivatives. Corrosion Science, 108, 23–35. doi:10.1016/j.corsci.2016.02.036
- Fiori-Bimbi, M. V., Alvarez, P. E., Vaca, H., & Gervasi, C. A. (2015). Corrosion inhibition of mild steel in HCL solution by pectin. Corrosion Science, 92, 192–199. doi:10.1016/j.corsci.2014.12.002
- Gece, G. (2011). Drugs: A review of promising novel corrosion inhibitors. Corrosion Science, 53(12), 3873–3898. doi:10.1016/j.corsci.2011.08.006
- Kothari, C. R., & Garg, G. (2014). Research methodology: Methods and techniques (Third ed.). New Delhi: New Age International (P) Ltd., Publishers.
- Loto, C. A., Popoola, A. P. I., Fayomi, O. S., & Loto, R. T. (2012). Corrosion polarization behaviour of type 316 stainless steel in strong acids and acid chlorides. International Journal of Electrochemical Science, 7, 3787–3797.
- Mohammed, I. Y., Abakr, Y. A., Yusup, S, & Kazi, F. K. (2016). Valorization of Napier grass via intermediate pyrolysis: Optimization using response surface methodology and pyrolysis products characterization. Journal of Cleaner Production, 139, 717–728. doi:10.1016/J.Jclepro.2016.11.099
- Odejobi, O. J., & Akinbulumo, O. A. (2019). Modeling and optimization of the inhibition efficiency of euphorbia heterophylla extracts based corrosion inhibitor of mild steel corrosion in HCl media using а response surface methodology. Journal of Chemical Technology and Metallurgy, 54(1), 217–232.
- Odoni, B. U., Edoziuno, F. O., & Chukwurah, N. C. (2017). Corrosion inhibition potential of methyl-5-benzoyl-2-benzimidazole carbamate (mebendazole) for mild steel in 1.0m sulphuric acid. International Journal of Research in Engineering and Innovation, 1(6), 190–194. Retrieved from http://www.ijrei.com
- Ofuyekpone, O., Akaluzia, R. O., & Edibo, S. (2017). Investigating the influence of immersion time and inhibitor concentration on the inhibiting potential of imperrata cylindrica as Corrosion Inhibitor of mild steel. International Journal of Research in Engineering and Innovation (IJREI), 1(6), 147–152. Retrieved from http://www.ijrei.com
- Okafor, C. S., Anadebe, V. C., & Onukwuli, O. D. (2019). Experimental, statistical modelling and molecular dynamics simulation concept of sapium ellipticum leaf extract as corrosion inhibitor for carbon steel in acid environment. South African Journal of Chemistry, 72, 164–175. doi:10.17159/0379-4350/2019/v72a22
- Olawale, O., Adediran, A. A., Talabi, S. I., Nwokocha, G. C., & Ameh, A. O. (2017). Inhibitory action of vernonia amygdalina extract (VAE) on the corrosion of carbon steel in acidic medium. Journal of Electrochemical Science and Engineering, 7(3), 145–152. doi:10.5599/jese.353
- Omotioma, M., & Onukwuli, O. D. (2016). Modeling the corrosion inhibition of mild steel in HCl medium with the inhibitor of pawpaw leaves extract. Portugaliae Electrochimica Acta, 34(4), 287–294. doi:10.4152/pea.201604287
- Onukwuli, O. D., Nwanekezie, M. N., Anadebe, V. C., & Omotioma, M. (2017). Optimization of the inhibition efficiency of bitter kola leaf extract as corrosion inhibitor of mild steel in H2SO4. IJIETS, 1(2), 288–296.
- Onukwuli, O. D., & Omotioma, M. (2016). Optimization of the inhibition efficiency of mango extract as corrosion inhibitor of mild steel in 1.0 M H2SO4 using response surface methodology. Journal of Chemical Technology and Metallurgy, 51(3), 302–314. doi:10.1155/2015/410120
- Patni, N., Agarwal, S., & Shah, P. (2013). Greener approach towards corrosion inhibition. Chinese Journal of Engineering, 2013, 1–10. doi:10.1155/2013/784186
- Praveen, K. S. V., Manjunatha, B. K., Gaurav, M., & Mohammed, A. M. (2016). Optimization of crude oil and PAHs degradation by stenotrophomonas rhizophila KX082814 strain through response surface methodology using box-behnken design. Biotechnology Research International, 2016. doi:10.1155/2016/4769542
- Rani, B. E. A., & Basu, B. B. J. (2012). Green inhibitors for corrosion protection of metals and alloys: An overview. International Journal of Corrosion, 2012, 1–15. doi:10.1155/2012/380217
- Saha, P., & Banerjee, S. (2013). Optimization of process parameters for vinegar production using banana fermentation. International Journal of Research in Engineering and Technology, 2(9), 501–514. doi:10.15623/ijret.2013.0209076
- Uhlig, H. H., & King, C. V. (1972). Corrosion and corrosion control. Journal of the Electrochemical Society, 119, 327C. doi:10.1149/1.2404108