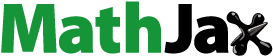
Abstract
This research is an application of machine learning in medical sciences. The purpose of this research was to use machine learning through the simulated data to study the association of age, body mass index, cigarettes smoked per day, alcohol consumed per week, diastolic blood pressure, and systolic blood pressure on hypertension and coronary heart disease. The Structural Equation Modelling using Partial Least Square Method was used for the analysis of data. The results have revealed that except for age, body mass index and systolic blood pressure all the rest of the factors had a significant positive association with hypertension and coronary heart disease. The results can be of use for medical practitioners as well as researchers in machine learning, as it adds to the repository of earlier studies, which have attempted to seek relationships between these variables.
PUBLIC INTEREST STATEMENT
Research on the identification of antecedents of hypertension and coronary heart disease has been active since the past several years and newer methods are being designed to identify the most significant factors, which may be responsible for the risk of hypertension and coronary heart disease. In this research machine learning technique has been used to study the association of age, body mass index, cigarettes smoked per day, alcohol consumed per week, diastolic blood pressure, and systolic blood pressure on hypertension and coronary heart disease. The Structural Equation Modelling was used for the analysis of data. The results have revealed that except for age, body mass index and systolic blood pressure all the rest of the factors had a significant positive association with hypertension and coronary heart disease. The results can be of use to the medical practitioners, as it adds to the repository of earlier studies in this area.
1. Introduction
Heart disease is one of the major killer diseases today (Aljanabi, Qutqut, & Hijjawi, Citation2018). One of the most challenging task is to identify the causes of this disease and prevent it to the extent possible. Thus, one of the most anticipated application of Machine Learning (ML) has been disease detection and prevention. Medical diagnostic reasoning is becoming popular application of ML in which expert systems and model-based schemes provide mechanisms used for the development of hypotheses, which will be then tested using the modelling and simulation techniques or statistical analysis (Magoulas & Prentza, Citation1999). Deep neural networks is another promising application of machine learning which is used to relate the input and output of complex models through layers so that the relationships may be studied in detail (Bakator & Radosav, Citation2018). Convolutional neural networks (CNN) have proved to be very promising and successfully used in anatomical localization (de Vos, Wolterink, de Jong, Viergever, & Išgum, Citation2016), automated segmentation (Dou et al., Citation2017), brain tumour grading (Pan et al., Citation2015), glaucoma detection (Chen, Xu, Wong, Wong, & Liu, Citation2015), Alzheimer’s disease prediction (Payan & Montana, Citation2015), Automatic breast tissue classification (Dubrovina, Kisilev, Ginsburg, Hashoul, & Kimmel, Citation2018), Automatic detection of myocardial infarction (Acharya et al., Citation2017). Deep belief network path (DBN-NN) based on back-propagation algorithm of Artificial Neural Network (ANN) has been very promising in breast cancer classification (Abdel-Zaher & Eldeib, Citation2016). While these are few of the evidences of successful applications of ML in medical field the endeavour to use it in the prediction of the causes of disease well in advance to prevent it is a continuous and never-ending endeavour. Among various approaches, structural equation modelling approach has been very promising in recent past and this paper is an attempt in that direction.
2. Research methodology
2.1. Study population
The research is based on the real-life parameters obtained from Offspring Cohort data of the Framingham Heart Study (Mi, Eskridge, George, & Wang, Citation2011). The average values of body mass index, cigarettes smoked per day, alcohol consumed per week, diastolic blood pressure, and systolic blood pressure in the given range of age were randomized through the program. Each of the latent variable has been divided into three mutually exclusive groups, as the SEM approach demands at least two indicators for a given latent variable. The range of the population in terms of age was from 45 to 60 in steps of 5, the BMI ranged from 23 to 28.5 in steps of 2.9, CPD ranged from 5 to 15, APW ranged from 1.5 to 4.9 Oz, DBP ranged from 65.4 to 78.5, SBP ranged from 107.4 to 128.6, percentage chance of HPT ranged from 10 to 30, percentage chance of CHD ranged from 5 to 15 as indicated in the Framingham Heart Study (Mi et al., Citation2011).
2.2. Structural equation modelling
The method adopted in this research to identify the interaction of the antecedents with hypertension and coronary heart disease is Structural Equation Modelling (SEM). SEM has been specified as the tool for analysis as it can perform factor analysis and regression analysis simultaneously and as it is based on Partial Least Square Method (PLSM) it addresses the issue of multi-collinearity very effectively. SEM has two distinct components: the measurement model, which provides the reliability and validity of the research data, and the structural model, which tests the hypotheses.
2.3. Mathematical background
The hypothetical model adopted in this research is fundamentally derived through the Threshold Model proposed by Muthén (Citation1984), according to which the dichotomous response for the CHD or presence or absence of hypertension (ϕijk (k = 1,2 … p)) is a function of unobserved continuous response ϕ*ijk via the threshold model, mathematically.
where τk = is the threshold parameter.
The structural model is developed based on the above-defined threshold model with Level-1 model which is within the Family Model and Level-2 which is Family Random-intercept Model.
At Level-1, the equations are in the form of linear form of the structural model given by,
where
vi = a vector (p × 1)
B = p x p matrix of structural parameters
Γ = coefficient matrix describing the relationship between latent response and predictor variables
δij = is a vector of residuals (p × 1)
At Level-2,
where
vi = a vector (p × 1) in the ith family,
γ = constant, and
= a vector of residuals (p × 1).
The above Level 1 (jth individual) and the Level 1(ith family) equations provide the generalized equations for the second level SEM.
2.4. Hypothetical model
While HPT and CHD have been causally linked to each other by quite a good number of researchers (e.g. Wu et al., Citation2015) both have been associated with several other factors. Age is one of the factors, which has been associated with HPT and CHD (e.g. Franklin & Wong, Citation2013; Jousilahti, Vartiainen, Tuomilehto, & Puska, Citation1999; Kjeldsen, Citation2018; Sekhri et al., Citation2014; Wu at al., Citation2015). Kjeldsen (Citation2018) has specifically found the need to develop age-adjusted models, models based on relative risk based on age, within and between countries to generalize the association of age with HPT and CHD. Increasing blood pressure with age has been considered as a major factor, which can induce HYP or CHD. Age-related stiffening of the aorta, which leads to a reduction in the capacity of elastic reservoir and a greater chance of CHD particularly during SBP (Franklin & Wong, Citation2013). Franklin and Wong (Citation2013) found that both SBB and DBP have a significant association with CHD. Mi et al., (Citation2011) have undertaken separate studies for male (n = 496) and female (n = 470) to study the influence of various factors on HPT and CHD and found that in case of male age had an influence on HPT, whereas factors such as BMI, CPD, APW, DBP and SBP had no influence on HPT, whereas in case of female age, DBP and SBP had significant influence on HPT, whereas CPD and APW had no significant influence on HPT. Wu at al., (Citation2015) through a study with a large sample of 77,389 found that the influence of HPT on CVD was much higher in the case of women in comparison to that of men. The study also revealed that HYP was significantly influenced by age, BMI, CPD and APW. Franklin and Wong (Citation2013) have found that the association of SBP with CHD was higher than that of DBP.
The above studies indicate that while there are several parameters which are associated with the HPT and CHD it is evident that there is a need to investigate more on the influence of age, body mass index, cigarettes smoked per day, alcohol consumed per week, diastolic blood pressure, and systolic blood pressure, thus the following hypotheses:
H1: Age has a significant association with hypertension.
H2: Body mass index has a significant association with hypertension.
H3: Cigarettes smoked per day has a significant association with hypertension.
H4: Alcohol consumed per week has a significant association with hypertension.
H5: Diastolic blood pressure has a significant association with hypertension.
H6: Systolic blood pressure has a significant association with hypertension.
H7: Age has a significant association with Coronary heart disease.
H8: Body mass index has a significant association with Coronary heart disease.
H9: Cigarettes smoked per day has a significant association with Coronary heart disease.
H10: Alcohol consumed per week has a significant association with Coronary heart disease.
H11: Diastolic blood pressure has a significant association with Coronary heart disease.
H12: Systolic blood pressure has a significant association with Coronary heart disease.
H13: Hypertension has a significant association with Coronary heart disease.
The hypothetical SEM is depicted in Figure
3. Results and discussions
3.1. Measurement model
The Cronbach’s Alpha which is a measure of internal consistency of the data varied from 0.7 to 0.9, indicating a moderate-to-high level of acceptance (Taber, Citation2018) (Table ). The composite reliability of the model varied from 0.7 to 0.9 confirming moderate to high reliability (Ahmad, Zulkurnain, & Khairushalimi, Citation2016). Rho-A is another measure of composite reliability and values of above 0.6 are considered acceptable (Rigdon, Ringle, & Sarstedt, Citation2010), and in the present case it ranges from 0.7 to 0.8 which indicate moderately high composite reliability. The convergent validity measured as standardized factor loading (FL) after factor reduction (Table ) ranged from 0.5 to 0.9 indicating a good correlation between the factor and the observed variable. While the aforementioned reliability values are referring to the reliability of measurement in terms of the items in the given factor and the factors in a research construct Discriminant Reliability is a measure of how these factors are mutually exclusive of each other. As square roots of AVE of all the dimensions are greater than their respective correlation between the remaining constructs, acceptable discriminant validity is confirmed (Table ).
Table 1. Reliability and validity
Table 2. Factor loading
Table 3. Inter-item correlation
3.2. Structural model
The path coefficient values ranged from 0.01 to 0.7 (Figure ), and the R-square values obtained for percentage chance of hypertension and coronary heart disease are 0.7 and 0.6, respectively, which is quite adequate in comparison to the other research studies in this field (R-square cut off 0.1). The t-values (Table and Figure ) indicate that the following hypotheses are supported:
Table 4. Significance test table
Results indicate that the following hypotheses are supported:
H3: Cigarettes smoked per day has a significant association with hypertension.
H4: Alcohol consumed per week has a significant association with hypertension.
H5: Diastolic blood pressure has a significant association with hypertension.
H9: Cigarettes smoked per day has a significant association with Coronary heart disease.
H10: Alcohol consumed per week has a significant association with Coronary heart disease.
H11: Diastolic blood pressure has a significant association with Coronary heart disease.
H13: Hypertension has a significant association with Coronary heart disease.
Following hypotheses are not supported:
H1: Age has a significant association with hypertension.
H2: Body mass index has a significant association with hypertension.
H6: Systolic blood pressure has a significant association with hypertension.
H7: Age has a significant association with Coronary heart disease.
H8: Body mass index has a significant association with Coronary heart disease.
H12: Systolic blood pressure has a significant association with Coronary heart disease.
4. Discussions
The study results indicate that hypertension (HPT) and coronary heart disease (CHD) have a significant association. Hypertension is considered to have a significant causation with CHD by quite a good number of researchers (e.g. Biswas, Singh, & Singh, Citation2017; Cubrilo-Turek, Citation2003; Pan et al., Citation2015; Milane et al., Citation2014). Several pathophysiologic mechanisms link these two variables and research shows that mortality due to CHD is 2.3 times greater when HPT is present (Escobar, Citation2002; Olafiranye et al., Citation2011). Milane et al. (Citation2014) have found that the association is significant only at a later age. In another study with a large sample (n = 147,201), it was found that hypertension when associated with age, education, and place of stay had a relationship with CHD than individually. Hansson and Lundin (Citation1984) have established a cause/consequence relationship between hypertension and coronary heart disease and found that the relation could be bidirectional too. So, by and large, the outcome of this study is in agreement with the findings of earlier researchers. In contrary to these findings, there are also studies which have revealed that despite the high cases of hypertension, coronary heart disease rate has been low, and hence, coronary heart disease has been considered to be clearly multifactorial (Poulter, Citation1999). Thus, the revelation of this study can add to the existing literature to support the fact that HPT and CHD are associated with each other.
The results of the study indicate that cigarettes smoked per day (CSPD) has a significant association with HPT as well as CHD. In the medical field, it has been established that cigarette smoke can cause a functional and initially transient damage primarily of the endothelium (Leone, Citation2015). Medical explanation to the association of cigarette smoking to CHD is that smoking activates the platelet function which leads to thrombosis formation and myocardial infarction thus leading to the CHD (Inoue, Citation2004). Further, the ill-effects of nicotine and carbon monoxide are reduced tolerance to exercises, and hence, it increases the chance of HPT. So, this outcome is in alignment with the earlier findings (e.g. Ain & Regmi, Citation2015; Bowman, Gaziano, Buring, & Sesso, Citation2007; Gao, Shi, & Wang, Citation2017; Pardell & Rodicio, Citation2005; Robertson et al., Citation2014; Stallones, Citation2015; Yathish, Manjula, Srinivas, & Gayathree, Citation2011). On the contrary to these findings, there are also studies which have reported lower BP levels among smokers and lesser chance of HPT (Bowman et al., Citation2007; Gao et al., Citation2017; Pankova et al., Citation2015). Primatesta, Falaschetti, Gupta, Marmot, and Poulter (Citation2001) found that the influence of cigarette on HPT was only for the age group of above 45 years. In another study, it was observed that direct causal relationship between cigarette smoking and HPT cannot be established (Virdis, Giannarelli, Fritsch Neves, Taddei, & Ghiadoni, Citation2010). Keto et al. (Citation2016) have found through a sample size of over 5000 respondents that cigarette smoking has no clinically significant influence on CHD. These contradictions call for further research with additional parameters such as family history of smoking, duration of smoking, environment of smoking (indoor or outdoor), type of smoking habit (chain or intermittent) etc., to study the influence smoking on HPT and CHD.
The analysis indicates that alcohol consumed per week (ACPW) has a significant association with HPT and CHD. The findings of this research are in alignment with the findings of earlier research (e.g. Chevli, Ahmad, Rasool, & Herrington, Citation2019; Criqui & Thomas, Citation2017; Fuchs, Chambless, Whelton, Nieto, & Heiss, Citation2001; Roerecke et al., Citation2017; Santana et al., Citation2018; Xin et al., Citation2001). Several researchers have made attempts to study the influence of alcohol consumption in light, moderate, and heavy dosages leading to HPT and CHD. The classification of alcohol consumption has been mainly in terms of quantity of intake and frequency/week. Briasoulis, Agarwal and Messerli, (Citation2012) claim that alcohol intake increases the risk of HPT, but the relationship between light to moderate alcohol consumption and hypertension is controversial. Santana et al. (Citation2018) claim that the association between ACPW and HPT itself is controversial. While the contradictions regarding the association of ACPW and HPT/CHD exist, there are studies with concrete findings. For instance, Miller, Anton, Egan, Basile, and Nguyen (Citation2005) found that three or more standard drinks per day were associated with predictive HPT. Reduction in ACPW had no significant influence on BP in people who drank two or fewer drinks per day, but BP increased with the reduction in alcohol intake if the consumption was more than three drinks a day (Roerecke et al., Citation2017). There are studies, which found that light-to-moderate alcohol consumption can reduce hypertension (e.g. Gillman, Cook, Evans, Rosner, & Hennekens, Citation1995; Son, Citation2011; Thadhani et al., Citation2002). Thus, the inference was that a dose-dependent reduction in BP can be achieved with a reduction in alcohol consumption. Santana et al. (Citation2018) have found that alcohol consumption increases BP. In a large sample study with 14,727,591 respondents, it was established that alcohol abuse leads to CHD (Whitman et al., Citation2017). There are many other similar studies in which the association between heavy alcohol consumption and CHD has been established (Klatsky, Citation2015). On the contrary to these findings, it has also been established that while heavy alcoholism (more than 3 standard consumptions per day) is associated with CHD some research show that moderate drinking can bring down the chance of CHD (e.g. Klatsky, Citation1999). Researchers have found that moderate alcohol consumption, irrespective to the type of alcohol, reduces the risk of CHD (Criqui & Thomas, Citation2017; Hines & Rimm, Citation2001). The medical explanation is that alcohol can cause changes in lipids and haemostatic factors, which in turn has a protective effect on the cardiovascular system (Yuan, Ross, Gao, Henderson, & Mimi, Citation1997). Thus, further research may be required to draw a conclusive evidence about the association between ACPW and CHD. It is also necessary to conduct research with standardized parameters such as demographic background, health condition, type of work, etc. and the amount of alcohol intake to draw a conclusive evidence about the association between ACPW and HPT/CHD.
It was revealed in hypothesis testing that Diastolic Blood Pressure (DBP) has a significant association with HPT and CHD. A group of researchers have associated DBP with HPT (Behradmanesh & Nasri, Citation2012; Tringali & Huang, Citation2017; Wang et al., Citation2015) and DBP with to CHD (e.g. Drozdz & Kawecka-Jaszcz, Citation2014; Franklin et al., Citation2001; Lichtenstein, Shipley, & Rose, Citation1985; Shu et al., Citation2013; Tsujimoto & Kajio, Citation2018). Bavishi, Goel, and Messerli (Citation2016) consider the association between DBP and HPT to be controversial. There are findings on both the sides of the argument. For instance, in a study, it is found that DBP elevations of as little as 2 mm Hg can lead to HPD (Sander, Citation2011). Franklin et al. (Citation2001) have found that with age there is a gradual shift from DBP to SBP as the predictors of CHD. But there are also study results which have shown that systolic or diastolic blood pressure has no association with HYP or CHD. For instance, D’Agostino, Belanger, Kannel, and Cruickshank (Citation1991) based on a sample size of 5209 concluded that DBP had no significant influence on CHD. Further, in another large sample study of 1.25 million sample size with respondents of 30 years of age and above, it was concluded that both systolic and diastolic blood pressures have no influence on HPT and CHD (Rapsomaniki et al., Citation2014). Thus, while it is difficult to conclude that DBP has an influence on the risk of HPT and CHD more studies in this direction can be recommended strongly to draw a conclusive evidence.
The generally accepted concept that age has a significant association with HPT, which has been revealed through earlier researches (e.g. Borzecki, Glickman, Kader, & Berlowitz, Citation2006; Hosseini et al., Citation2015; Rigaud & Forette, Citation2001), is not supported in this research. The result is in agreement with the findings of Soudarssanane, Karthigeyan, Stephen, and Sahai (Citation2006). Increase in BP is considered an inevitable consequence of ageing particularly in the industrialized environment, which eventually leads to HPT (Pinto, Citation2007). There are also studies, which disagree with this conclusion and claim that in hypertensives of different age, if comparable blood pressure levels are considered the reference, then the variability of blood pressure is not consistently related to age (Brennan, O’Brien, & O’Malley, Citation1986). So, there is a need to further investigate on the association of age with HPT as it is a multifactorial construct.
This research has revealed that body mass index (BMI) had no association with HPT or CHD. Many researchers have found that BMI is a predictor of HPT (e.g. Dua, Bhuker, Sharma, Dhall, & Kapoor, Citation2014; Linderman et al., Citation2018; Soudarssanane et al., Citation2006). In another study, with sample drawn from three populations from Africa and Asia, it was found that BMI has a significant positive correlation with HPT. Gadhavi, Solanki, Rami, Bhagora, and Thakor (Citation2015) have shown that there is a significant relationship between BMI and HPT through a sample of 775 respondents of 40 to 50 years of age. Tuan, Adair, Suchindran, He, and Popkin (Citation2009) found that physical exercise, food habits, cultures, religions, and demographic characteristics may interact with BMI in predicting HPT. It is imperative that more research is required to arrive at a conclusion on whether BMI has an association with HPT or CHD.
Systolic Blood Pressure (SBP) is found to have no significant influence on HPT or CHD as revealed in this study. Research has shown that there is a linear increase in SPR up to the fifth or sixth decades of life after which later slows down gradually (Bavishi et al., Citation2016). The finding of this study is in contradiction to that by a group of researchers who claim that variability in SBP has a significant association with HPT and CHD (e.g. Franklin, Citation2004; Mehlum et al., Citation2018; Stevens et al., Citation2016). Zhou et al. (Citation2017) in their study of 1479 articles across nations found that the variability of BP was a regional phenomenon and it cannot be generalized in its association with HPT or CHD. Thus, further research may be required to arrive at a conclusion of the association of SBP with HYP and CHD.
5. Conclusion
Using Machine Learning in medical sciences has always been challenging due to the complexity as well as the dynamic nature of the variables involved. Nevertheless, machine learning can be very useful in disease detection and prevention. In this research, it is specifically found that cigarette smoking and alcohol consumption has a significant positive association with the risk of hypertension and coronary heart disease. This research indicated that alcohol intake has also been significantly and positively associated with coronary heart disease. Further, this research has indicated that diastolic blood pressure had a positive significant influence on both hypertension and coronary heart disease; however, systolic blood pressure has no significant influence on hypertension and coronary heart disease. It was revealed that hypertension and coronary heart disease have a significant positive relationship. These revelations could be of help to the further researchers and it adds to the knowledge on the antecedents of hypertension and coronary heart disease.
In conclusion, this research has shown that machine learning could be very effective in the determination of relationships between the factors such as age, body mass index, cigarette smoking, alcohol consumption, and diastolic/systolic blood pressure. Among the factors considered in this research age, body mass index and systolic blood pressure have not shown an association with either hypertension or coronary heart disease. Deeper research may be required to verify this outcome, as there are earlier studies, which have associated these variables with hypertension and coronary heart disease.
Abbreviations
AGE: | = | Age |
APW: | = | Alcohol Per Week |
BMI: | = | Body Mass Index |
CHD: | = | Coronary Heart Disease |
CPD: | = | Cigarettes Per Day |
DBP: | = | Diastolic Blood Pressure |
HPT: | = | Hypertension |
SBP: | = | Systolic Blood Pressure |
Article highlights
Machine learning through the simulated data to study the association of age, body mass index, cigarettes smoked per day, alcohol consumed per week, diastolic blood pressure, and systolic blood pressure on hypertension and coronary heart disease.
Machine learning, a relatively new technology, is fast emerging as a better prediction model in healthcare applications.
This research has indicated that diastolic blood pressure had a positive significant influence on both hypertension and coronary heart disease. However, systolic blood pressure has no significant influence on hypertension and coronary heart disease.
The factors like age, body mass index and systolic blood pressure have not shown an association with either hypertension or coronary heart disease.
Additional information
Funding
Notes on contributors
Lewlyn L. R. Rodrigues
Lewlyn L. R. Rodrigues is Professor in Humanities & Management at MIT, Manipal, India and guided 11 PhDs in Engineering, Management and Education.
Dasharathraj K Shetty
Dasharathraj K Shetty is Assistant Professor in Humanities & Management at MIT, Manipal, India. He has about 15 years of experience in teaching.
Nithesh Naik
Nithesh Naik is Asst. Professor in Mechanical & Manufacturing Engineering, at MIT Manipal, India and has about 10 years of experience.
Chetana Balakrishna Maddodi
Chetana Balakrishna Maddodi is Asst. Professor at SOM, Manipal, India and has over 8 years of experience in teaching.
Anuradha Rao
Anuradha Rao is Assistant Professor in Information and Communication Technology, at MIT, Manipal, India has over 15 years of experience in teaching.
Ajith Kumar Shetty
Ajith Kumar Shetty at Sahyadri Narayana, Hospital, India and has an experience of about 10 years.
Rama Bhat
Rama Bhat is Professor in Kasturba Medical College, Manipal India with over 3 decades of teaching experience.
B. M. Zeeshan Hameed
Zeeshan Hameed is Associate Professor in Kasturba Medical College, Manipal, India with over 5 years of teaching.
References
- Abdel-Zaher, A. M., & Eldeib, A. M. (2016). Breast cancer classification using deep belief networks. Expert Systems with Applications, 46, 139–15. doi:10.1016/j.eswa.2015.10.015
- Acharya, U. R., Fujita, H., Oh, S. L., Hagiwara, Y., Tan, J. H., & Adam, M. (2017). Application of deep convolutional neural network for automated detection of myocardial infarction using ECG signals. Information Sciences, 415, 190–198. doi:10.1016/j.ins.2017.06.027
- Ahmad, S., Zulkurnain, N. N. A., & Khairushalimi, F. I. (2016). Assessing the validity and reliability of a measurement model in Structural Equation Modeling (SEM). Journal of Advances in Mathematics and Computer Science, 1(1), 1–8.
- Ain, Q. U., & Regmi, K. (2015). The effects of smoking in developing hypertension in Pakistan: A systematic review. South East Asia Journal of Public Health, 5(1), 4–11. doi:10.3329/seajph.v5i1.24845
- Aljanabi, M., Qutqut, H. M., & Hijjawi, M. (2018). Machine learning classification techniques for heart disease prediction: A review. International Journal of Engineering & Technology, 7(4), 5373–5379.
- Bakator, M., & Radosav, D. (2018). Deep learning and medical diagnosis: A review of literature. Multimodal Technologies and Interaction, 2(3), 47. doi:10.3390/mti2030047
- Bavishi, C., Goel, S., & Messerli, F. H. (2016). Isolated systolic hypertension: An update after SPRINT. The American Journal of Medicine, 129(12), 1251–1258. doi:10.1016/j.amjmed.2016.08.032
- Behradmanesh, S., & Nasri, P. (2012). Serum cholesterol and LDL-C in association with level of diastolic blood pressure in type 2 diabetic patients. Journal of Renal Injury Prevention, 1(1), 23.
- Biswas, A., Singh, S. K., & Singh, R. K. (2017). Linkages between hypertension and coronary heart disease in India: Evidence from India human development survey-2 (2011–2012). Indian Journal of Community Medicine: Official Publication of Indian Association of Preventive & Social Medicine, 42(4), 200. doi:10.4103/ijcm.IJCM_168_16
- Borzecki, A. M., Glickman, M. E., Kader, B., & Berlowitz, D. R. (2006). The effect of age on hypertension control and management. American Journal of Hypertension, 19(5), 520–527. doi:10.1016/j.amjhyper.2005.10.022
- Bowman, T. S., Gaziano, J. M., Buring, J. E., & Sesso, H. D. (2007). A prospective study of cigarette smoking and risk of incident hypertension in women. Journal of the American College of Cardiology, 50(21), 2085–2092. doi:10.1016/j.jacc.2007.08.017
- Brennan, M., O’Brien, E., & O’Malley, K. (1986). The effect of age on blood pressure and heart rate variability in hypertension. Journal of Hypertension. Supplement : Official Journal of the International Society of Hypertension, 4, S269–S272.
- Briasoulis, A., Agarwal, V., & Messerli, F. H. (2012). Alcohol consumption and the risk of hypertension in men and women: a systematic review and meta‐analysis. The Journal of Clinical Hypertension, 14(11), 792–798.
- Chen, X., Xu, Y., Wong, D. W. K., Wong, T. Y., & Liu, J. (2015, August). Glaucoma detection based on deep convolutional neural network. In 2015 37th annual international conference of the IEEE engineering in medicine and biology society, Milano, Itally, (pp. 715–718). IEEE.
- Chevli, A. P., Ahmad, M., Rasool, S., & Herrington, D. (2019). Alcohol consumption and risk of hypertension. Journal of the American College of Cardiology, 73(9), 12. doi:10.1016/s0735-1097(19)33774-x
- Criqui, M. H., & Thomas, I. C. (2017). Alcohol consumption and cardiac disease. Journal of the American College of Cardiology, 69(1), 25–27. doi:10.1016/j.jacc.2016.10.049
- Cubrilo-Turek, M. (2003). Hypertension and coronary heart disease. Electronic Journal of International Federation of Clinical Chemistry and Laboratory Medicine, 14(2), 67–73.
- D’Agostino, R. B., Belanger, A. J., Kannel, W. B., & Cruickshank, J. M. (1991). Relation of low diastolic blood pressure to coronary heart disease death in presence of myocardial infarction: The Framingham Study. Bmj, 303(6799), 385–389. doi:10.1136/bmj.303.6799.385
- de Vos, B. D., Wolterink, J. M., de Jong, P. A., Viergever, M. A., & Išgum, I. (2016, March). 2D image classification for 3D anatomy localization: Employing deep convolutional neural networks. In Medical Imaging 2016: Image Processing (Vol. 9784, p. 97841Y). International Society for Optics and Photonics, Gaziabad, India.
- Dou, Q., Yu, L., Chen, H., Jin, Y., Yang, X., Qin, J., & Heng, P. A. (2017). 3D deeply supervised network for automated segmentation of volumetric medical images. Medical Image Analysis, 41, 40–54. doi:10.1016/j.media.2017.05.001
- Drozdz, D., & Kawecka-Jaszcz, K. (2014). Cardiovascular changes during chronic hypertensive states. Pediatric Nephrology, 29(9), 1507–1516. doi:10.1007/s00467-013-2614-5
- Dua, S., Bhuker, M., Sharma, P., Dhall, M., & Kapoor, S. (2014). Body mass index relates to blood pressure among adults. North American Journal of Medical Sciences, 6(2), 89. doi:10.4103/1947-2714.127751
- Dubrovina, A., Kisilev, P., Ginsburg, B., Hashoul, S., & Kimmel, R. (2018). Computational mammography using deep neural networks. Computer Methods in Biomechanics and Biomedical Engineering: Imaging & Visualization, 6(3), 243–247.
- Escobar, E. (2002). Hypertension and coronary heart disease. Journal of Human Hypertension, 16(S1), S61. doi:10.1038/sj.jhh.1001345
- Franklin, S. S. (2004). Systolic blood pressure: It’s time to take control. American Journal of Hypertension, 17(S3), 49S–54S. doi:10.1016/j.amjhyper.2004.08.020
- Franklin, S. S., Larson, M. G., Khan, S. A., Wong, N. D., Leip, E. P., Kannel, W. B., & Levy, D. (2001). Does the relation of blood pressure to coronary heart disease risk change with aging? The Framingham heart study. Circulation, 103(9), 1245–1249. doi:10.1161/01.CIR.103.9.1245
- Franklin, S. S., & Wong, N. D. (2013). Hypertension and cardiovascular disease: Contributions of the Framingham heart study. Global Heart, 8(1), 49–57. doi:10.1016/j.gheart.2012.12.004
- Fuchs, F. D., Chambless, L. E., Whelton, P. K., Nieto, F. J., & Heiss, G. (2001). Alcohol consumption and the incidence of hypertension: The atherosclerosis risk in communities study. Hypertension, 37(5), 1242–1250. doi:10.1161/01.HYP.37.5.1242
- Gadhavi, R., Solanki, D., Rami, K., Bhagora, S., & Thakor, N. (2015). Relationship between blood pressure and BMI: A cross sectional study among government employees of Gujarat state, India. International Journal of Research in Medical Sciences, 3(7), 1637–1640. doi:10.18203/2320-6012.
- Gao, K., Shi, X., & Wang, W. (2017). The life-course impact of smoking on hypertension, myocardial infarction and respiratory diseases. Scientific Reports, 7(1), 4330. doi:10.1038/s41598-017-04552-5
- Gillman, M. W., Cook, N. R., Evans, D. A., Rosner, B., & Hennekens, C. H. (1995). Relationship of alcohol intake with blood pressure in young adults. Hypertension, 25(5), 1106–1110. doi:10.1161/01.HYP.25.5.1106
- Hansson, L., & Lundin, S. (1984). Hypertension and coronary heart disease: Cause and consequence or associated diseases? The American Journal of Medicine, 76(2), 41–44. doi:10.1016/0002-9343(84)90955-0
- Hines, L. M., & Rimm, E. B. (2001). Moderate alcohol consumption and coronary heart disease: A review. Postgraduate Medical Journal, 77(914), 747–752. doi:10.1136/pgmj.77.914.747
- Hosseini, M., Baikpour, M., Yousefifard, M., Fayaz, M., Koohpayehzadeh, J., Ghelichkhani, P., & Gouya, M. M. (2015). Blood pressure percentiles by age and body mass index for adults. EXCLI Journal, 14, 465. doi:10.17179/excli2015-565
- Inoue, T. (2004). Cigarette smoking as a risk factor of coronary artery disease and its effects on platelet function. Tobacco Induced Diseases, 2(1), 27. doi:10.1186/1617-9625-2-1-27
- Jousilahti, P., Vartiainen, E., Tuomilehto, J., & Puska, P. (1999). Sex, age, cardiovascular risk factors, and coronary heart disease: A prospective follow-up study of 14 786 middle-aged men and women in Finland. Circulation, 99(9), 1165–1172. doi:10.1161/01.CIR.99.9.1165
- Keto, J., Ventola, H., Jokelainen, J., Linden, K., Keinänen-Kiukaanniemi, S., Timonen, M., & Auvinen, J. (2016). Cardiovascular disease risk factors in relation to smoking behaviour and history: A population-based cohort study. Open Heart, 3(2), e000358. doi:10.1136/openhrt-2015-000358
- Kjeldsen, S. E. (2018). Hypertension and cardiovascular risk: General aspects. Pharmacological Research, 129, 95–99. doi:10.1016/j.phrs.2017.11.003
- Klatsky, A. L. (1999). Moderate drinking and reduced risk of heart disease. Alcohol Research and Health, 23(1), 15–24.
- Klatsky, A. L. (2015). Alcohol and cardiovascular diseases: Where do we stand today? Journal of Internal Medicine, 278(3), 238–250. doi:10.1111/joim.2015.278.issue-3
- Leone, A. (2015). Smoking and hypertension. Journal of Cardiology and Current Research, 2(2), 00057.
- Lichtenstein, M. J., Shipley, M. J., & Rose, G. (1985). Systolic and diastolic blood pressures as predictors of coronary heart disease mortality in the Whitehall study. British Medical Journal (Clinical Research Ed.), 291(6490), 243–245. doi:10.1136/bmj.291.6490.243
- Linderman, G. C., Lu, J., Lu, Y., Sun, X., Xu, W., Nasir, K., & Krumholz, H. M. (2018). Association of body mass index with blood pressure among 1.7 million Chinese adults. JAMA Network Open, 1(4), e181271–e181271. doi:10.1001/jamanetworkopen.2018.1271
- Magoulas, G. D., & Prentza, A. (1999, July). Machine learning in medical applications. Advanced course on artificial intelligence (pp. 300–307). Berlin, Heidelberg: Springer.
- Mehlum, M. H., Liestøl, K., Kjeldsen, S. E., Julius, S., Hua, T. A., Rothwell, P. M., & Berge, E. (2018). Blood pressure variability and risk of cardiovascular events and death in patients with hypertension and different baseline risks. European Heart Journal, 39(24), 2243–2251. doi:10.1093/eurheartj/ehx760
- Mi, X., Eskridge, K. M., George, V., & Wang, D. (2011). Structural equation modeling of gene–environment interactions in coronary heart disease. Annals of Human Genetics, 75(2), 255–265. doi:10.1111/j.1469-1809.2010.00634.x
- Milane, A., Abdallah, J., Kanbar, R., Khazen, G., Ghassibe-Sabbagh, M., Salloum, A. K., & Platt, D. E. (2014). Association of hypertension with coronary artery disease onset in the Lebanese population. Springer Plus, 3(1), 533. doi:10.1186/2193-1801-3-533
- Miller, P. M., Anton, R. F., Egan, B. M., Basile, J., & Nguyen, S. A. (2005). Excessive alcohol consumption and hypertension: Clinical implications of current research. The Journal of Clinical Hypertension, 7(6), 346–351. doi:10.1111/jch.2005.7.issue-6
- Muthén, B. (1984). A general structural equation model with dichotomous, ordered categorical and continuous latent variable indicators. Psychometrika, 49(1), 115–132. doi:10.1007/BF02294210
- Olafiranye, O., Zizi, F., Brimah, P., Jean-Louis, G., Makaryus, A. N., McFarlane, S., & Ogedegbe, G. (2011). Management of hypertension among patients with coronary heart disease. International Journal of Hypertension, 2(1), 34–41.
- Pan, Y., Huang, W., Lin, Z., Zhu, W., Zhou, J., Wong, J., & Ding, Z. (2015, August). Brain tumor grading based on neural networks and convolutional neural networks. In 2015 37th Annual International Conference of the IEEE Engineering in Medicine and Biology Society (EMBC) (pp. 699–702), Milano, Italy.
- Pankova, A., Kralikova, E., Fraser, K., Lajka, J., Svacina, S., & Matoulek, M. (2015). No difference in hypertension prevalence in smokers, former smokers and non-smokers after adjusting for body mass index and age: A cross-sectional study from the Czech Republic, 2010. Tobacco Induced Diseases, 13(1), 24. doi:10.1186/s12971-015-0049-4
- Pardell, H., & Rodicio, J. L. (2005). High blood pressure, smoking and cardiovascular risk. Journal of Hypertension, 23(1), 219–221. doi:10.1097/00004872-200501000-00037
- Payan, A., & Montana, G. (2015). Predicting Alzheimer’s disease: A neuroimaging study with 3D convolutional neural networks (arXiv preprint arXiv:1502.02506).
- Pinto, E. (2007). Blood pressure and ageing. Postgraduate Medical Journal, 83(976), 109–114. doi:10.1136/pgmj.2006.048371
- Poulter, N. (1999). Coronary heart disease is a multifactorial disease. American Journal of Hypertension, 12(S6), 92S–95S. doi:10.1016/S0895-7061(99)00163-6
- Primatesta, P., Falaschetti, E., Gupta, S., Marmot, M. G., & Poulter, N. R. (2001). Association between smoking and blood pressure: Evidence from the health survey for England. Hypertension, 37(2), 187–193. doi:10.1161/01.HYP.37.2.187
- Rapsomaniki, E., Timmis, A., George, J., Pujades-Rodriguez, M., Shah, A. D., Denaxas, S., & Williams, B. (2014). Blood pressure and incidence of twelve cardiovascular diseases: Lifetime risks, healthy life-years lost, and age-specific associations in 1· 25 million people. The Lancet, 383(9932), 1899–1911.
- Rigaud, A. S., & Forette, B. (2001). Hypertension in older adults. The Journals of Gerontology Series A: Biological Sciences and Medical Sciences, 56(4), M217–M225. doi:10.1093/gerona/56.4.M217
- Rigdon, E. E., Ringle, C. M., & Sarstedt, M. (2010). Structural modeling of heterogeneous data with partial least squares. Review of Marketing Research, 7(7), 255–296.
- Robertson, J. O., Ebrahimi, R., Lansky, A. J., Mehran, R., Stone, G. W., & Lincoff, A. M. (2014). Impact of cigarette smoking on extent of coronary artery disease and prognosis of patients with non–ST-segment elevation acute coronary syndromes: An analysis from the ACUITY trial (Acute Catheterization and Urgent Intervention Triage Strategy). JACC: Cardiovascular Interventions, 7(4), 372–379. doi:10.1016/j.jcin.2013.11.017
- Roerecke, M., Kaczorowski, J., Tobe, S. W., Gmel, G., Hasan, O. S., & Rehm, J. (2017). The effect of a reduction in alcohol consumption on blood pressure: A systematic review and meta-analysis. The Lancet Public Health, 2(2), e108–e120. doi:10.1016/S2468-2667(17)30003-8
- Sander, G. E. (2011). Drugs that increase blood pressure. Clinical Practice, 8(3), 275.
- Santana, N. M. T., Mill, J. G., Velasquez-Melendez, G., Moreira, A. D., Barreto, S. M., Viana, M. C., & Molina, M. D. C. B. (2018). Consumption of alcohol and blood pressure: Results of the ELSA-Brasil study. PloS One, 13(1), e0190239. doi:10.1371/journal.pone.0190239
- Sekhri, T., Kanwar, R. S., Wilfred, R., Chugh, P., Chhillar, M., Aggarwal, R., … Singh, S. (2014). Prevalence of risk factors for coronary artery disease in an urban Indian population. BMJ Open, 4(12), e005346. doi:10.1136/bmjopen-2014-005346
- Shu, W., Jing, J., Fu, L. C., Min, J. T., Bo, Y. X., Ying, Z., & Dai, C. Y. (2013). The relationship between diastolic pressure and coronary collateral circulation in patients with stable angina pectoris and chronic total occlusion. American Journal of Hypertension, 26(5), 630–635. doi:10.1093/ajh/hps096
- Son, M. K. (2011). Association between alcohol consumption and hypertension. Journal of the Korean Society of Hypertension, 17(2), 65–73. doi:10.5646/jksh.2011.17.2.65
- Soudarssanane, M. B., Karthigeyan, M., Stephen, S., & Sahai, A. (2006). Key predictors of high blood pressure and hypertension among adolescents: A simple prescription for prevention. Indian Journal of Community Medicine, 31(3), 164.
- Stallones, R. A. (2015). The association between tobacco smoking and coronary heart disease. International Journal of Epidemiology, 44(3), 735–743. doi:10.1093/ije/dyv124
- Stevens, S. L., Wood, S., Koshiaris, C., Law, K., Glasziou, P., Stevens, R. J., & McManus, R. J. (2016). Blood pressure variability and cardiovascular disease: Systematic review and meta-analysis. bmj, 354, i4098. doi:10.1136/bmj.i4098
- Taber, K. S. (2018). The use of Cronbach’s alpha when developing and reporting research instruments in science education. Research in Science Education, 48(6), 1273–1296. doi:10.1007/s11165-016-9602-2
- Thadhani, R., Camargo, C. A., Stampfer, M. J., Curhan, G. C., Willett, W. C., & Rimm, E. B. (2002). Prospective study of moderate alcohol consumption and risk of hypertension in young women. Archives of Internal Medicine, 162(5), 569–574. doi:10.1001/archinte.162.5.569
- Tringali, S., & Huang, J. (2017). Reduction of diastolic blood pressure: Should hypertension guidelines include a lower threshold target? World Journal of Hypertension, 7(1), 1–9. doi:10.5494/wjh.v7.i1.1
- Tsujimoto, T., & Kajio, H. (2018). Low diastolic blood pressure and adverse outcomes in heart failure with preserved ejection fraction. International Journal of Cardiology, 263, 69–74. doi:10.1016/j.ijcard.2018.04.031
- Tuan, N. T., Adair, L. S., Suchindran, C. M., He, K., & Popkin, B. M. (2009). The association between body mass index and hypertension is different between East and Southeast Asians. The American Journal of Clinical Nutrition, 89(6), 1905–1912. doi:10.3945/ajcn.2008.26809
- Virdis, A., Giannarelli, C., Fritsch Neves, M., Taddei, S., & Ghiadoni, L. (2010). Cigarette smoking and hypertension. Current Pharmaceutical Design, 16(23), 2518–2525. doi:10.2174/138161210792062920
- Wang, Y., Xing, F., Liu, R., Liu, L., Zhu, Y., Wen, Y., & Song, Z. (2015). Isolated diastolic hypertension associated risk factors among Chinese in Anhui Province, China. International Journal of Environmental Research and Public Health, 12(4), 4395–4405. doi:10.3390/ijerph120404395
- Whitman, I. R., Agarwal, V., Nah, G., Dukes, J. W., Vittinghoff, E., Dewland, T. A., & Marcus, G. M. (2017). Alcohol abuse and cardiac disease. Journal of the American College of Cardiology, 69(1), 13–24. doi:10.1016/j.jacc.2016.10.048
- Wu, C. Y., Hu, H. Y., Chou, Y. J., Huang, N., Chou, Y. C., & Li, C. P. (2015). High blood pressure and all-cause and cardiovascular disease mortalities in community-dwelling older adults. Medicine, 94, 47. doi:10.1097/MD.0000000000002160
- Xin, X., He, J., Frontini, M. G., Ogden, L. G., Motsamai, O. I., & Whelton, P. K. (2001). Effects of alcohol reduction on blood pressure: A meta-analysis of randomized controlled trials. Hypertension, 38(5), 1112–1117. doi:10.1161/hy1101.093424
- Yathish, T. R., Manjula, C. G., Srinivas, R. D., & Gayathree, L. (2011). A study on the association of coronary artery disease and smoking by a questionnaire method. Journal of Clinical and Diagnostic Research : JCDR, 5(2), 264–268.
- Yuan, J. M., Ross, R. K., Gao, Y. T., Henderson, B. E., & Mimi, C. Y. (1997). Follow up study of moderate alcohol intake and mortality among middle-aged men in Shanghai, China. Bmj, 314(7073), 18. doi:10.1136/bmj.314.7073.18
- Zhou, B., Bentham, J., Di Cesare, M., Bixby, H., Danaei, G., Cowan, M. J., & Taddei, C. (2017). Worldwide trends in blood pressure from 1975 to 2015: A pooled analysis of 1479 population-based measurement studies with 19· 1 million participants. The Lancet, 389(10064), 37–55. doi:10.1016/S0140-6736(16)31919-5