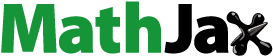
Abstract
The selection of the welding process is a matter of decision-making involving a variety of information according to the type of product.Accordingly, the vast information will significantly enhance the decision-making process. A combined p-robust technique, fuzzy credibility constrained programming and data envelopment analysis (DEA) are proposed to select the welding process for repairing the shredder hammer. The p-robust technique has regret value to control relative regret of data, and fuzzy credibility constraint programming has confidence level to satisfy chance constraints. In addition, the proposed approach is able to handle fuzzy input data and fuzzy output data to uncertainty data. The proposed method is used to investigate which welding process is best based on two cases. Three welding processes are analyzed for the first case that focuses on manual welding process, and four welding processes are investigated for the second case that focuses on automatic welding process. According to the results, increasing the credibility level would also increase the discrimination power of the proposed approach, so the ranking of DMUs could be seen easily.
PUBLIC INTEREST STATEMENT
The important point in the decision-making process in the criteria selection problem by using DEA is the output data obtained from the experts. The ability to maintain the robustness of some expert opinions is a crucial issue. P-robust fuzzy DEA credibility constraint developed in this article considers the input data aspects of the experts that would make the robustness of the decision made more valid. This happens because the basic principle of this approach is that is there is one item of data from an expert that does not synchronize with other data, the results of the decisons obtained will be invalid.
1. Introduction
In the sugar industry, the shredder hammer is used to shred sugarcane billets and pulverize sugarcane billet into a fibrous mat (Yin, Authurs, Wright, & Santarossa, Citation2014a). Generally, the shredder machine has 90–250 of shredder hammers that rotate on a rotor at around 1,000 RPM, and it can process sugarcane at 7,000–15,000 tons/day (Yin, Authurs, Wright, & Santarossa, Citation2014b). The shredder machine is essential for manufacturing industries, but the cost that involved in the operation of shredder machine is expensive because the service life of shredder hammer is relatively short. The shredder hammer needs to be checked for balance to prevent something that can damage the machine. The vibration monitoring system is the system for checking the balance of shredder hammer if the shredder hammer broken during operation, this machine has to stop. The shredder hammer consists of carbon steel as the base metal, and hard-facing is stacked o/n the base metal using the welding process. The hard-facing is a form surfacing that is applied to reduce wear, abrasion, impact, erosion, galling, and cavitation (Bęczkowski, Gucwa, Wróbel, & Kulawik, Citation2016; Mehta, Mittal, & Gupta, Citation2017). Shredder hammer consists of base metal made of carbon steel and Hardfacing is stacked on base metal using welding process. Usually, the shredder hammer is produced and repaired by using hardfacing, which consist of hard metal overlays deposited on the new or worn surfaces by using different welding processes, techniques, and alloy. The problem is to choose the best welding process using hardfacing to fix the shredder hammer. Little research has been done for selection of the welding process by the different method, however, none of that research care with welding process selection for repairing shredder hammer.
Typically, the selection of welding process depends on experiences or follows the general application in the similar companies. However, this strategy often ignores many criteria or parameters that can be affecting the suitable welding process selection. Therefore, knowledge management is a critical element for a better decision. Knowledge from an expert will benefit greatly from using sophisticated methods to analyze it.
Some research has been done on the selection of the welding process, but none of them deal with choosing the welding process using hard-facing to repair the shredder hammer. Choudhury, Bandyopadhyay, and Rudrapati (Citation2014) studied about welding process selection used confidence level for each qualifier. Ravisankar, Balasubramanian, and Muralidharan (Citation2006) used analytic hierarchic process (AHP) as a method for welding process selection of high strength aluminum alloys to fabricate butt joint. Jafarian and Vahdat (Citation2012) studied various welding processes using AHP in high-pressure shipbuilding. Balasubramanian, Varahamoorthy, Ramachandran, and Muralidharan (Citation2009) and Jayant and Dhillon (Citation2015) used AHP for welding process selection of hard-facing on carbon steel. As is seen, the AHP method is a popular method for welding process selection, but the issue of AHP is the weight of criteria by a judgment of decision-maker. It means if the view is changed, the final ranking will change as well. So the limitation of AHP depends on the decision-maker’s judgment. Thus, this article develops a novel group decision-making method by combining p-robust technique, fuzzy credibility constrained programming, and data envelopment analysis (DEA) to select the welding process for repairing the shredder hammer.
2. Literature review
2.1. Multi-criteria decision-making (MCDM) techniques
A multi-criteria decision-making (MCDM) is a discipline which appears from problems of ranking or selecting of alternative (choice). MCDM tool allows conflicting parameters to choose several alternatives to help a decision-maker. A multi-criteria decision-making (MCDM) framework has four elements (Mousavi-Nasab & Sotoudeh-Anvari, Citation2017) as follows:
Criteria (parameter factor for making decision).
Alternatives (as choice of candidate).
Weight of attribute.
Score for making decision.
The welding process selection problem is one of the multi-criteria decision-making problem. Nowadays, more than 70 MCDM methods have been introduced (Sun, Gollnick, Li, & Stumpf, Citation2014). Furthermore, it is very hard to identify which MCDM is the best because each technique has advantages and disadvantages (Sarkis, Citation2000).
Based on previous research, the Analytic Hierarchy Process (AHP) is one of the favorite of MCDM techniques (Balasubramanian et al., Citation2009; Jafarian & Vahdat, Citation2012); however, several reasons conclude that AHP is a MCDM technique which is not appropriate for the selectionf the welding process. Chatterjee, Athawale, and Chakraborty (Citation2011) used AHP technique for material selection problem, but the time of calculation is very high. Hatami-Marbini, Tavana, Moradi, and Kangi (Citation2013) defined that AHP is a controversial frame and also the deficiency of transparency in the procedure. Jahan, Mustapha, Ismail, Sapuan, and Bahraminasab (Citation2011) mentioned that AHP has a limited amount of options, which is often not over 15. But in welding process selection problem the number of options and criteria are often large. For example, Darwish, Tamimi, and Al-Habdan (Citation1997) studied a welding process selection problem with 30 alternatives along with various decision factors.
Furthermore, when the number of alternatives is increased, causing the computation of MCDM methods will be difficult. For example, the GTMA method, which was proposed by Rao (Citation2006) as an MCDM tool, has a limited number of criteria of 5 or 6 criteria due to difficult calculation procedure (Karande & Chakraborty, Citation2012). Another method like Ashby when the amount of attribute is increased will be difficult for computation procedure (Yazdani & Payam, Citation2015). Another method of MCDM is ELECTRE, ELECTRE method, as the number of choices increased, the volume of calculations increased fast (Mousavi-Nasab & Sotoudeh-Anvari, Citation2017). Furthermore, ELECTRE method only provides the rank of each alternative and ELECTRE does not determine or show the numerical score of each alternative for more understanding of differences between each option (Jahan, Ismail, Sapuan, & Mustapha, Citation2010). On the other hand, PROMETHEE method aims to minimize the limitations with ELECTRE by giving a more clear approach. PROMETHEE has smaller computational efforts than ELECTRE method (Peng & Xiao, Citation2013). However, PROMETHEE has various weaknesses that is designing the problem and identifying the weights (Hatami-Marbini et al., Citation2013).
Sun et al. (Citation2014) claimed that selection of the most appropriate procedures of MCDM is difficult. Mela, Tiainen, and Heinisuo (Citation2012) studied several MCDM techniques in the building design field. However, they show that the best MCDM technique can hardly be found. The most significant factor in MCDM context is to find an MCDM technique that can evaluate what it is expected to measure (Løken, Citation2007). The various studies in MCDM context have emphasized the use of easy and understandable techniques to resolve the MCDM problems, Chatterjee et al. (Citation2011) explained that the difficulty of an MCDM problem will be determined by the number of choice criteria and data form (deterministic, stochastic, etc.). Therefore, the MCDM method in the welding process selection, the calculation should be simple, easy, and save time.
2.2. Data envelopment analysis (DEA)
Charnes, Cooper, and Rhodes (Citation1978) introduced Data Envelopment Analysis (DEA). DEA is a non-parametric performance measurement method to measure the relative efficiencies of decision-making units (DMUs). The aim of DEA is to obtain the efficiency score of a DMU by comparing how creatively this DMU transforms inputs into outputs (Ramanathan, Citation2006). The activities related to DEA grow at faster rates because of some advantages. On the other hand, the DEA can be considered to be providing an approximate of the production function (Wang, Zhao, Zhou, & Zhou, Citation2013). Also, the DEA does not need a decision-maker to set weights to inputs and outputs. Moreover, DEA does not require any transformation of the variables into one unit (Shabani, Saen, & Torabipour, Citation2012). Lohtia, Donthu, and Yaveroglu (Citation2007) reported that the DEA is more precise than “Regression” technique in determining efficient and inefficient. Furthermore, DEA is a very potent method for benchmarking. Lee and Kim (Citation2014) argue that DEA is capable to give suggestion on how to improve efficiency score. Furthermore, DEA also can handle quantitative and qualitative variables.
Nowadays, DEA still grows very fast, Liu, Lu, Lu, and Lin (Citation2013) reported around 4500 papers have been published about DEA prior to 2010. Furthermore, during 2010 to 2014, around 2000 papers related to DEA were published (Liu, Lu, & Lu, Citation2016). They argue that the DEA has five research fronts: “bootstrapping and two-stage analysis”, “undesirable factors”, “cross-efficiency and ranking”, “network DEA, dynamic DEA, and SBM”, and “Other coherent subareas”.
Bootstrapping constructs a base for statistical inference in DEA and a two-stage analysis establishes procedures for contextual factor analysis. Undesirable factors method uses reference technology and efficiency measure under the traditional DEA framework (Zhou, Ang, & Poh, Citation2008). The cross-efficiency concept was first proposed by Sexton, Silkman, and Hogan (Citation1986) and Du, Cook, Liang, and Zhu (Citation2014) re-examine the cross-efficiency in detail. Some research applies DEA as an MCDM for the problem of ranking, to make DEA into MCDM requires “methodological connection” (SARKIS, Citation2000). The Network DEA was first used by Färe, Grosskopf, and Whittaker (Citation2007) and Tone and Tsutsui (Citation2009) were the first researchers to introduce SBM. The objective of this approach is inserting a time factor in the network structure, which is clearly the core research activity in this research. Furthermore for other coherent subareas, 3 small kinds of research also use DEA for the methodology such as emphasizing on fuzzy and imprecise DEA, variable selection, and models that handle stochastic data (Kao & Liu, Citation2009).
2.3. Applying DEA as a multiple criteria decision-making (MCDM) tool
Mousavi-Nasab and Sotoudeh-Anvari (Citation2017) studied about comparison traditional objective of DEA and MCDM (see Figure ). The objective of DEA is to determine the efficiency score of DMUs by comparing how well these units convert inputs into outputs, while MCDM comes up from problems of ranking and selecting from a set of the alternative that has conflicting criteria (Masudin & Saputro, Citation2016; Saputro, Masudin, & Daneshvar Rouyendegh, Citation2015). He defines a “methodological connection” between MCDM and DEA is to specify maximizing criteria as output and minimizing criterion as inputs. It should be noted that in MCDM context, the attribute whose bigger value is better called maximizing, and the attribute whose lower value is better called minimizing (Mirhedayatian, Vahdat, Jelodar, & Saen, Citation2013). They adopted this methodological connection for welding process selection for repairing nodular cast iron engine block by the DEA and TOPSIS approaches, in this research; they mention the positive effect as maximizing and negative effect as minimizing. Srdjevic, Medeiros, and Porto (Citation2005) and Opricovic and Tzeng (Citation2003) also made this methodological connection for applying DEA as an MCDM. Furthermore, Opricovic (Citation2016) in his research in the case of hydropower system planning believed that DEA could be used as a supplement for screening alternatives within MCDM.
Although MCDM methods have been frequently used for welding process selection, applying DEA as MCDM for welding process is very small. Based on previous research, only Mirhedayatian et al. (Citation2013) which used the fuzzy DEA and TOPSIS approaches as MCDM for the welding process selection. Although DEA can handle quantitative and qualitative variables, however, conventional DEA still has weakness about group decision-making. In addition, judgments of experts are often imprecise or vague owing to lack of their knowledge of the input and output parameters. Furthermore, ambiguity often occurs because of several overlapping opinions of an expert in a group decision-making process. Moreover, they used fuzzy DEA model for solving the vague issue, but their literature did not discuss the ambiguity issue.
3. Input and output selection
Research is going on in the area of welding process selection using various methods and different attributes. Some research on welding process selection is given below.
In order to make ranking and selecting from a set of alternatives (DMUs), selecting appropriate criteria is a critical phase in applying data envelopment analysis (DEA) as a multiple criteria decision-making tool. The criteria could be considered an input or output in the DEA model.
4. Method
This section will discuss the conceptual framework for welding process selection for repairing shredder hammer. In accordance with the existing literature, Charnes et al. (Citation1978) introduced Data Envelopment Analysis (DEA) and Fasanghari, Amalnick, Anvari, and Razmi (Citation2015) develop data envelopment analysis (DEA) to be able to resolve ambiguity and vagueness from group decision-making by integrated data envelopment analysis (DEA) and p-robust fuzzy credibility constrained programming technique approaches. Fasanghari et al. (Citation2015) used that method as a multi-criteria decision-making (MCDM) for enterprise architecture (EA) scenario analysis.
This research uses integrated data envelopment analysis (DEA) and p-robust fuzzy credibility constrained programming technique approaches for welding process selection for repairing shredder hammer. According to welding process selection for repairing shredder hammer has been discussed in the literature review, there are six welding processes regarded as DMU and the description of DMU as given in Table .
Table 1. Summary of attributes (parameters)
Table 2. Decision-making units (DMUs) for this study
Based on input and output selection that has been discussed in the literature review, the input variable and output variable used in welding process selection for repairing shredder hammer is given in the Table .
Due to data collection for this research comes from the experts, so it is essential for this research to identify the experts. The expert meaning has been discussed in the literature review and expert who becomes our data source should have enough knowledge about welding, repairing shredder hammer and all of the attributes of this research. A questionnaire is a tool for collecting data from an expert. The proposed approach is illustrated in Figure .
5. Model development
5.1. Data envelopment analysis (DEA)
Charnes et al. (Citation1978) introduced data envelopment analysis (DEA) and CCR (Charnes-Cooper-Rhodes) model as the most common DEA models. Suppose there are oth DMUs (DMU1, DMU2, …, and DMUs), each of DMUs have m inputs (i = 1, …, n) and s output
(r = 1, …, m). The efficiency of DMU is the division of the weighted sum of outputs (virtual output) by the weighted sum inputs (virtual input). To solve CCR model, the fractional program (FPo) can be used as follows:
Subject to
The model (1) is an input-oriented model and (FPo) is a measure of the efficiency score of DMUs. The vi is valued for input “weight”, and the ur is the output “weight”. Suppose the DMU has an efficiency score equal to 1 (FPo = 1), that means the DMU is efficient; otherwise, it is inefficient. The model (1) is equivalent to the following model:
Model (1) and (2) are the basic of DEA, let ath is the opinion of the expert added to basic model of DEA. Using ath expert’ opinions (see Table -), the model (1) will be changed as follows:
is the efficiency score of oth DMU with inputs and outputs obtained according to the ath expert’s opinion. However, the main issue of conventional DEA cannot cope with uncertainty data. In the group decision-making has two main uncertainty data, such as ambiguity and vagueness (Fasanghari et al., Citation2015). The ambiguity refers to multiple interpretations of the expert, and vagueness is due to the lack of expert opinion precision. In order to solve these problems, the p-robustness technique is applied to solve ambiguity, and fuzzy credibility constraint technique is applied to solve vagueness.
5.2. P-robustness technique
To introduce the robustness technique, let ath expert’s opinion be a set of scenarios, and is the highest efficiency score of oth DMU in ath expert’s opinion. This technique has regret value (P) for control final efficiency score and the best efficiency score of each scenario, which is called “relative regret”. In the following, we recall p-robustness technique theory for modifying conventional DEA to be p-robustness DEA technique (Snyder & Daskin, Citation2006).
Definition 1. Let regret value (P) ≥ 0 be a constant. Let (u, v) be a feasible solution to (Pa) for all a A, and let Eo(u, v) is the final efficiency score for oth DMU. Moreover, (u, v) is the weight of input and output of data envelopment analysis (DEA). Furthermore, (u, v) is called p-robust if for all a
A.
The model (4) is equivalent to the following model:
Base on the model (2), the model (5) can be formulated as follow:
Furthermore, weighted summation () of ath expert’ opinions also need to add to model (3).
Using constraint model (6) and objective function model (7), now the model (3) will be changed to the p-robustness DEA technique as follows:
The model (8) is the integrated data envelopment analysis (DEA) and p-robustness technique which the objective function is maximizing the oth DMU. Furthermore, the constraint of force the score of final efficiency (
) more than 100(P)% of the score of ideal efficiency (
). The regret value (P) is flexible value. In case the regret value (P) is small, the model (8) potential infeasible. And if P =
, the constraint (6) will be inactive. Furthermore, increasing the relative regret (P) will affect to improve the final efficiency score (Meng & Liu, Citation2007). However, the higher regret value (P) will generate the final efficiency score, and the best efficiency score of each scenario increase too. Hence, this research will use 0.6 as regret value (P) to keep the final efficiency (
) not more than 60% of score of ideal efficiency (
).
5.3. Fuzzy credibility constraint technique
Fuzzy credibility constraint technique adopts the concept of fuzzy chance-constrained programming. The fuzzy chance-constrained programming applies on the objective function and all of constrained with fuzzy inputs and fuzzy output in the model (8). In the previous research (Fasanghari et al., Citation2015), they studied about p-robust fuzzy credibility constrained group DEA model in the analysis and selection of enterprise and architecture (EA) scenario. However, their technique only capable of handling outputs parameters, but the inputs parameters are considered as dummy input or the input equal to one. This research develops a new model that is capable of handling both the inputs and outputs parameters. Subsequently, we take the basic concept of credibility theory (Li & Liu, Citation2006; Meng & Liu, Citation2007) used to apply in the model (8), and it becomes new integrated p-robustness fuzzy credibility constraint DEA technique.
Definition 2. Let θ be a nonempty set, P(θ) the power set of θ, and Pos a possibility measure on P(θ). If A is an element in P(θ), The credibility measure Cr is defined by
For any A P(θ).
Definition 3. An n-dimensional fuzzy vector is defined as a function from a credibility space {θ, P(θ), Pos} to the set of n-dimensional real vector. If n = 1, then it is called a fuzzy variable. Let is fuzzy variable, and for any
, we have
, then it is a positive fuzzy variable.
Definition 4. The fuzzy variable are said to be independent if
For any subsets
Definition 5. Let be a fuzzy variable, and
. Then
Is called the -optimistic value to
.
Furthermore, p-robustness DEA technique or model (8) needs to transform to be model with fuzzy inputs and fuzzy outputs. The fuzzy DEA can build as follows:
Where is the ith fuzzy input of DMUo based on the ath opinion of the expert, and
is the rth fuzzy output of DMUo based on the ath opinion of the expert. Moreover, based on fuzzy chance-constrained programming (FCCP) (Liu & Liu, Citation2009), model (12) can be formulated as follows:
are the predetermined credibility level.
is credibility level for satisfying the chance constraints of objective function,
is credibility level for satisfying of constraints of oth DMU and ath expert, and
is credibility level for satisfying p-robustness constraints of ath expert. Normally, the credibility level should be satisfied for a confidence level greater than 0.5
(Fasanghari et al., Citation2015; Meng & Liu, Citation2007). Furthermore, the model (13) can be formed using Theorems 6 and 7 (Lin, Citation2004).
Theorem 6. Consider a triangular fuzzy variable =
and
. When
, we have:
Theorem 7. Let =
and
=
are triangular fuzzy variable. Moreover, let
, and let
. When
, we have:
According Theorems 6 and 7, model (13) becomes the fractional linear programming as follow:
The model (18) is equivalent to the following model:
According to the formula above, the proposed approach can be summarized as follow:
Step 1: Determine the efficiency score () of oth DMU with the likely number inputs and the likely number outputs according to ath expert’s opinion through solving model (3).
Step 2: Determine the ideal efficiency () from each expert’s point of view.
Step 3: Determine the final efficiency score with triangular fuzzy inputs and triangular fuzzy outputs through solving model (19). Furthermore, rank the candidates based on the final efficiency score.
5.4. Selection of welding process
The application that was investigated is the shredder hammer. Furthermore, medium carbon steel (standard: JIS G4051, and grade: S45S) is the standard base metal that was used, and the specifications are presented in Table . The shredders hammer is used in the sugar industry to shred sugarcane billets and pulverize sugarcane billet into a fibrous mat. The shredder hammer needs to be checked for balance to prevent something unexpected which can damage the shredder machine. The shredder hammer can be repaired when it is not too badly worn. However, the shredder hammer cannot be fixed if the shredder hammer lost half of its dimension. The procedure of repairing the shredder hammer is the hammer must be removed from the shredder machine for rebuilding, replacing the mild steel lost and reapplying a hard-facing.
Furthermore, this research proposes two welding process types for investigating such as manual welding process and automatic welding process. For manual welding process, this research only focuses on shielded metal arc welding (SMAW), oxyacetylene gas welding (OAW), and gas tungsten arc welding (GTAW). Furthermore, flux cored arc welding (FCAW), submerged arc welding (SAW), gas tungsten arc welding (GTAW), and gas metal arc welding (GMAW) become the candidate of the automatic welding process. Moreover, thirteen parameters will be used to determine the best welding process either manual welding process or automatic welding process. The thirteen parameters are taken from Ferjutz and Davis (Citation1993) and the description of parameters shown in Table .
The primary goal of DEA is to determine the efficiency of decision-making units (DMUs), while multiple criteria decision-making (MCDM) is used for ranking or selecting from a set of the alternative that has different criteria. The “methodological connection” is necessary used to apply DEA as MCDM. The methodological connection between MCDM and DEA is to specify maximizing criteria as outputs of DEA and minimizing criteria as inputs of DEA (Sarkis, Citation2000). The criteria that bigger value is better called maximizing and the criteria whose lower is better called minimizing. Based on the methodology connection, the inputs of DEA are initial preparation required, welding procedures, post-weld cleaning, new equipment cost, consumable cost, electricity cost, welder’s skill need, welder fatigue, and welding hazard and risk level. Moreover, the outputs of DEA are the availability of consumable, flexibility of welding position, deposition rate, and weld-ability on base metal (medium carbon steel).
6. Results and discussion
Manual welding process and automatic welding process are the common welding process types for repairing the shredder hammer, so this research has two cases to investigating (Table ). The summary of two cases, parameters (Table ), and candidate of the welding process is shown in Table . Furthermore, five experts opinion will be analyzed by using a triangular fuzzy number such as =
and
=
. Moreover, the experts’ opinion is presented in A1–A5. Based on the proposed approach, the step of applying the approach for case 1 and case 2 as follows:
Table 3. Input-output variables (parameters) for this study
Step 1: After solving model (3), the efficiency score () of case 1 and case 2 is shown in Table .
Table 4. Input-output variables (parameters) for this study (cont.)
Step 2: Determine the ideal efficiency () from each expert’s point of view (Table ).
Case 1: SMAW = 1, OAW = 1, GTAW = 1.
Case 2: FCAW = 1, SAW = 1, GTAW = 1, GMAW = 1.
Step 3: We solve model (19) with p = 0.60 as the regret value and as weight opinion of experts. The final efficiency score of case 1 and case 2 is shown in Table .
Table 5. Specifications of base metal (shredder hammer)
Table 6. Specifications of base metal (shredder hammer)
Table 7. Specifications of base metal (shredder hammer)
Table 8. Specifications of base metal (shredder hammer)
Table 9. The final efficiency score obtained by proposed approach
According to Table , case 1 shows SMAW is the most appropriate welding process for the manual welding process. By using , SMAW is the first one with the final efficiency score of 0.451, GTAW is the second one with the final efficiency score of 0.445, OAW is the third one with the final efficiency score of 0.434. By using
, SMAW is the first one with the final efficiency score of 0.406, GTAW is the second one with the final efficiency score of 0.396, OAW is the third one with the final efficiency score of 0.383. By using
, SMAW is the first one with the final efficiency score of 0.366, GTAW is the second one with the final efficiency score of 0.353, OAW is the third one with the final efficiency score of 0.337.
In case 2, FCAW is the most appropriate welding process for the automatic welding process. By using , FCAW and GTAW are the first ones with the final efficiency score of 0.44, GMAW is the third one with the final efficiency score of 0.438, etc. By using
, FCAW is the first one with the final efficiency score of 0.387, GTAW and GMAW are the second ones with the final efficiency score of 0.386, etc. By using
, FCAW is the first one with the final efficiency score of 0.341, GTAW is the second one with the final efficiency score of 0.34, GMAW is the third one with the final efficiency score of 0.339, etc.
As we can see in case 2 (Table ), the final efficiency score of FCAW and GTAW with credibility level
is equal to 0.44.
This condition means the discrimination power is not satisfactory because it is difficult to decide which welding is the best. In order to solve this problem, we need to increase the credibility level to increase the discrimination power of the proposed approach. By increasing credibility level to be , we can decide FCAW is the best welding process for the automatic welding process. However, now the final efficiency score of GTAW and GMAW is the same of 0.386. Due to GTAW and GMAW have the same score, so it is impossible to decide which welding process is the second best, now we increase the credibility level to be
Furthermore, now we can see the exact ranking of automatic welding process by using
. Moreover, the characteristic of increasing credibility level (
) is the final efficiency score decrease as we can see in Table .
7. Conclusion
In this paper, a newly developed approach was used for welding process selection for repairing shredder hammer based on five experts’ opinion. P-robust fuzzy DEA credibility constraint that used in this article considers not only the output data from expert perception as commonly used in DEA but also the input data from the expert. The most advantage of the capability in handling both fuzzy inputs data and fuzzy outputs data is to cope with uncertainty data. An important point that can be gained by using robust fuzzy DEA credibility constraint is the ability to maintain the robustness of some expert opinions. If there is only one item that is not the same opinion or contradiction with another item, then the results will be invalid.
P-robust fuzzy DEA credibility constraint method in this article has applied to analyze the best welding processes of two cases. The result shows SMAW and FCAW are, respectively, the appropriate welding process for the manual welding process and the appropriate welding process for the automatic welding process. Furthermore, in the future research prospective, this model would be applied better in the situation of a higher uncertainty decision-making which considers a higher-better and smaller-better decision-making.
Additional information
Funding
Notes on contributors
Rahmad Wisnu Wardana
Rahmad Wisnu Wardana is a lecturer in Industrial Engineering department, University of Muhammadiyah Malang, Indonesia. His research interests are industrial system optimization, supply chain management and operations management (E-mail: [email protected], tel: +62-341 464 318 ext. 166).
Ilyas Masudin
Ilyas Masudin is an associate professor in Industrial Engineering department, University of Muhammadiyah Malang, Indonesia. He holds a PhD in Logistics from RMIT University, Australia. His research interests include logistics optimization, supply chain management, multi-criteria decision-making and operations management (E-mail: [email protected], tel: +62-341 464 318, ext.166).
Dian Palupi Restuputri
Dian Palupi Restuputri is a lecturer and researcher in Industrial Engineering department at the University of Muhammadiyah Malang. Her research interests are in the area of ergonomics and human factor engineering. She received his bachelor’s degree in Industrial Engineering from the Diponegoro University, Indonesia (2007). She holds a master’s degree in Industrial Engineering from Institute of Technology Bandung, Indonesia (2012) (Email: [email protected], tel: +62-341 464 318 ext. 166).
References
- Balasubramanian, V., VArahamoorthy, R., Ramachandran, C., & Muralidharan, C. (2009). Selection of welding process for hardfacing on carbon steels based on quantitative and qualitative factors. The International Journal of Advanced Manufacturing Technology, 40, 887–30. doi:10.1007/s00170-008-1406-8
- Bęczkowski, R., Gucwa, M., Wróbel, J., & Kulawik, A. (2016). The impact of the bead width on the properties of the anti-abrasion surfacing weld. 13th International conference of numerical analysis and applied mathematics Rhodes, Greece, 23–29 September. AIP Conference Proceedings (pp. 480095). AIP Publishing LLC.
- Capraz, O., Meran, C., Wörner, W., & Gungor, A. (2015). Using AHP and TOPSIS to evaluate welding processes for manufacturing plain carbon stainless steel storage tank. Archives of Materials Science, 158, 158.
- Charnes, A., Cooper, W. W., & Rhodes, E. (1978). Measuring the efficiency of decision making units. European Journal of Operational Research, 2, 429–444. doi:10.1016/0377-2217(78)90138-8
- Chatterjee, P., Athawale, V. M., & Chakraborty, S. (2011). Materials selection using complex proportional assessment and evaluation of mixed data methods. Materials & Design, 32, 851–860. doi:10.1016/j.matdes.2010.07.010
- Choudhury, N., Bandyopadhyay, A., & Rudrapati, R. (2014). Design optimization of process parameters for TIG welding based on Taguchi method. International Journal of Current Engineering and Technology, 2, 12–16.
- Darwish, S., Tamimi, A. L., & Al-Habdan, S. (1997). A knowledge base for metal welding process selection. International Journal of Machine Tools and Manufacture, 37, 1007–1023. doi:10.1016/S0890-6955(96)00073-9
- Davis, J. R. (1993). Hardfacing, weld cladding, and dissimilar metal joining. ASM International, ASM Handbook., 6, 789–829.
- Du, J., Cook, W. D., Liang, L., & Zhu, J. (2014). Fixed cost and resource allocation based on DEA cross-efficiency. European Journal of Operational Research, 235, 206–214. doi:10.1016/j.ejor.2013.10.002
- Färe, R., Grosskopf, S., & Whittaker, G. (2007). Network DEA. In Modeling Data Irregularities and Structural Complexities in Data Envelopment Analysis (pp. 209–240). Springer, Boston, MA.
- Fasanghari, M., Amalnick, M. S., Anvari, R. T., & Razmi, J. (2015). A novel credibility-based group decision making method for Enterprise Architecture scenario analysis using data envelopment analysis. Applied Soft Computing, 32, 347–368. doi:10.1016/j.asoc.2015.03.052
- Hatami-Marbini, A., Tavana, M., Moradi, M., & Kangi, F. (2013). A fuzzy group electre method for safety and health assessment in hazardous waste recycling facilities. Safety Science, 51, 414–426. doi:10.1016/j.ssci.2012.08.015
- Jafarian, M., & Vahdat, S. E. (2012). A fuzzy multi-attribute approach to select the welding process at high pressure vessel manufacturing. Journal of Manufacturing Processes, 14, 250–256. doi:10.1016/j.jmapro.2011.10.006
- Jahan, A., Ismail, M., Sapuan, S., & Mustapha, F. (2010). Material screening and choosing methods–a review. Materials & Design, 31, 696–705. doi:10.1016/j.matdes.2009.08.013
- Jahan, A., Mustapha, F., Ismail, M. Y., Sapuan, S., & Bahraminasab, M. (2011). A comprehensive VIKOR method for material selection. Materials & Design, 32, 1215–1221. doi:10.1016/j.matdes.2010.10.015
- Jayant, A., & Dhillon, M. (2015). Use of analytic hierarchy process (AHP) to select welding process in high pressure vessel manufacturing environment. International Journal of Applied Engineering Research, 10, 586–595.
- Kao, C., & Liu, S.-T. (2009). Stochastic data envelopment analysis in measuring the efficiency of Taiwan commercial banks. European Journal of Operational Research, 196, 312–322. doi:10.1016/j.ejor.2008.02.023
- Karande, P., & CHAKRABORTY, S. (2012). Application of multi-objective optimization on the basis of ratio analysis (MOORA) method for materials selection. Materials & Design, 37, 317–324. doi:10.1016/j.matdes.2012.01.013
- Lee, H., & Kim, C. (2014). Benchmarking of service quality with data envelopment analysis. Expert Systems with Applications, 41, 3761–3768. doi:10.1016/j.eswa.2013.12.008
- Li, X., & Liu, B. (2006). A sufficient and necessary condition for credibility measures. International Journal of Uncertainty, Fuzziness and Knowledge-Based Systems, 14, 527–535. doi:10.1142/S0218488506004175
- Lin, B. (2004). Uncertainty theory: An introduction to its axiomatic foundations. Berlin: Springer-Verlag.
- Liu, B., & Liu, B. (2009). Theory and practice of uncertain programming,239. Berlin: Springer.
- Liu, J. S., Lu, L. Y., & Lu, W.-M. (2016). Research fronts in data envelopment analysis. Omega, 58, 33–45. doi:10.1016/j.omega.2015.04.004
- Liu, J. S., Lu, L. Y., Lu, W.-M., & Lin, B. J. (2013). Data envelopment analysis 1978–2010: A citation-based literature survey. Omega, 41, 3–15. doi:10.1016/j.omega.2010.12.006
- Lohtia, R., Donthu, N., & Yaveroglu, I. (2007). Evaluating the efficiency of Internet banner advertisements. Journal of Business Research, 60, 365–370. doi:10.1016/j.jbusres.2006.10.023
- Løken, E. (2007). Use of multicriteria decision analysis methods for energy planning problems. Renewable and Sustainable Energy Reviews, 11, 1584–1595. doi:10.1016/j.rser.2005.11.005
- Masudin, I., & Saputro, T. E. (2016) Evaluation of B2C website based on the usability factors by using fuzzy AHP & hierarchical fuzzy TOPSIS. 2nd International Manufacturing Engineering Conference and The 5th Asia Pacific Conference on Manufacturing Systems. University of Malaysia. Pahang, Malaysia, 14 November. IOP Conference Series: Materials Science and Engineering (pp. 012091). IOP Publishing.
- Mehta, J., Mittal, V. K., & Gupta, P. (2017). Role of thermal spray coatings on wear, erosion and corrosion behavior: A review. Journal of Applied Science and Engineering, 20, 445г452.
- Mela, K., Tiainen, T., & Heinisuo, M. (2012). Comparative study of multiple criteria decision making methods for building design. Advanced Engineering Informatics, 26, 716–726. doi:10.1016/j.aei.2012.03.001
- Meng, M., & Liu, Y. (2007) Fuzzy data envelopment analysis with credibility constraints. Fourth International Conference on Fuzzy Systems and Knowledge Discovery. Haikui, China, 24–27 August (FSKD 2007) (pp. 149–153). IEEE. doi:10.1016/S0065-2776(07)95005-1
- Mirhedayatian, S. M., Vahdat, S. E., Jelodar, M. J., & Saen, R. F. (2013). Welding process selection for repairing nodular cast iron engine block by integrated fuzzy data envelopment analysis and TOPSIS approaches. Materials & Design, 43, 272–282. doi:10.1016/j.matdes.2012.07.010
- Mousavi-Nasab, S. H., & Sotoudeh-Anvari, A. (2017). A comprehensive MCDM-based approach using TOPSIS, COPRAS and DEA as an auxiliary tool for material selection problems. Materials & Design, 121, 237–253. doi:10.1016/j.matdes.2017.02.041
- Opricovic, S. (2016). A comparative analysis of the DEA-CCR model and the VIKOR method. Yugoslav Journal of Operations Research, 18 (2).
- Opricovic, S., & Tzeng, G.-H. (2003). Comparing DEA and MCDM method. In Multi-Objective Programming and Goal Programming (227–232). Springer, Berlin, Heidelberg.
- Peng, A.-H., & Xiao, X.-M. (2013). Material selection using PROMETHEE combined with analytic network process under hybrid environment. Materials & Design, 47, 643–652. doi:10.1016/j.matdes.2012.12.058
- Ramanathan, R. (2006). Data envelopment analysis for weight derivation and aggregation in the analytic hierarchy process. Computers & Operations Research, 33, 1289–1307. doi:10.1016/j.cor.2004.09.020
- Rao, R. V. (2006). A material selection model using graph theory and matrix approach. Materials Science and Engineering: A, 431, 248–255. doi:10.1016/j.msea.2006.06.006
- Ravisankar, V., Balasubramanian, V., & Muralidharan, C. (2006). Selection of welding process to fabricate butt joints of high strength aluminium alloys using analytic hierarchic process. Materials & Design, 27, 373–380. doi:10.1016/j.matdes.2004.11.008
- Saputro, T. E., Masudin, I., & Daneshvar Rouyendegh, B. (2015). A literature review on MHE selection problem: Levels, contexts, and approaches. International Journal of Production Research, 53, 5139–5152. doi:10.1080/00207543.2015.1005254
- Sarkis, J. (2000). A comparative analysis of DEA as a discrete alternative multiple criteria decision tool. European Journal of Operational Research, 123, 543–557. doi:10.1016/S0377-2217(99)00099-5
- Sexton, T. R., Silkman, R. H., & Hogan, A. J. (1986). Data envelopment analysis: Critique and extensions. New Directions for Program Evaluation, 1986, 73–105. doi:10.1002/(ISSN)1551-2371
- Shabani, A., Saen, R. F., & Torabipour, S. M. R. (2012). A new benchmarking approach in cold chain. Applied Mathematical Modelling, 36, 212–224. doi:10.1016/j.apm.2011.05.051
- Snyder, L. V., & Daskin, M. S. (2006). Stochastic p-robust location problems. IIE Transactions, 38, 971–985. doi:10.1080/07408170500469113
- Srdjevic, B., Medeiros, Y. D. P., & Porto, R. L. L. (2005). Data envelopment analysis of reservoir system performance. Computers & Operations Research, 32, 3209–3226. doi:10.1016/j.cor.2004.05.008
- Sun, X., Gollnick, V., Li, Y., & Stumpf, E. (2014). Intelligent multicriteria decision support system for systems design. Journal of Aircraft, 51, 216–225. doi:10.2514/1.C032296
- Tone, K., & Tsutsui, M. (2009). Network DEA: A slacks-based measure approach. European Journal of Operational Research, 197, 243–252. doi:10.1016/j.ejor.2008.05.027
- Wang, Q., Zhao, Z., Zhou, P., & Zhou, D. (2013). Energy efficiency and production technology heterogeneity in China: A meta-frontier DEA approach. Economic Modelling, 35, 283–289. doi:10.1016/j.econmod.2013.07.017
- Yazdani, M., & Payam, A. F. (2015). A comparative study on material selection of microelectromechanical systems electrostatic actuators using Ashby, VIKOR and TOPSIS. Materials & Design (1980–2015), 65, 328–334. doi:10.1016/j.matdes.2014.09.004
- Yin, L., Authurs, D., Wright, S., & Santarossa, L. (2014a). Fracture and wear in shredder hammer tungsten carbide tips in the sugar cane shredding process. In Recent advances in structural integrity analysis (pp. 57–61). UK: Woodhead Publishing.
- Yin, L., Authurs, D., Wright, S., & Santarossa, L. (2014b). Fracture and wear in shredder hammer tungsten carbide tips in the sugar cane shredding process. UK: Woodhead Publishing.
- Zhou, P., Ang, B. W., & Poh, K.-L. (2008). A survey of data envelopment analysis in energy and environmental studies. European Journal of Operational Research, 189, 1–18. doi:10.1016/j.ejor.2007.04.042
Appendix
Table A1. Data from expert 1
Table A2. Data from expert 2
Table A3. Data from expert 3
Table A4. Data from expert 4
Table A5. Data from expert 5