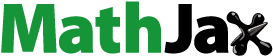
Abstract
The estimation of heat distribution during metal cutting is essential, as it contributes in workpiece deflection and quality of the machining. Inconel 625 is a high strength nickel-based superalloy that is broadly used in aerospace, automobile and nuclear applications. It is machining is very difficult due to their low thermal conductivity, high hardness even at high temperature. Therefore, a challenge in front of industries arises due to the properties of the material, high temperature of work-piece during cutting and hence might the cause of work-piece deformation & thermal stresses. However, by proper selection of cutting parameters and tool geometry, the work-piece temperature could be minimized. The present work emphasizes on the impact of machining parameters such as cutting speed, feed, depth of cut and step-over on the work-piece temperature during 2.5-D milling under dry condition. Box–Behnken design (BBD) model has been considered for three levels of input process parameters. The temperature of the work-piece has been estimated with the help of pyrometer type thermometer. The significance and adequacy of the proposed model and effects of process parameter on the temperature of work-piece has been carried out through ANOVA & regression methodology. The prognostic model in this study is generating values of the work-piece temperature which is close to those readings recorded experimentally. To optimize the machining parameters for minimization of work-piece temperature Genetic Algorithm has been applied on the prognostic model. Conformational experiments with a 5% error have also been performed to validate the results.
PUBLIC INTEREST STATEMENT
Inconel 625 is a high strength nickel-based superalloy with nickel and chromium as the major alloying elements. It is broadly used in aerospace, automobile, heat exchanger, combustors, seals, steam generators and nuclear applications. Because of low thermal conductivity, high temperature and high hardness, machining of this alloy is quite difficult. During the milling process, the tool work-piece interface generates extreme friction and intense cutting forces. The energy consumed during the cutting is converted into the heat which is further divided among the workpiece, tool & chip. Because of the lower thermal conductivity of the workpiece and the extreme heat generation, thermal expansion causes the deformation in the local area. Nickel-based superalloy workpiece commonly faces such type of challenges in the industries. The outcome of this investigation is likely to shed some light on various issues related to deformation in the material due to work-piece temperature.
1. Introduction
In the field of machining, 2.5D is a term often referred to as a part having multiple flat features with variable depth. 2.5D end milling is among the largely commercially trendy technologies used in machining of different products like aerospace, dies, marine and low rigidity thin-sectioned components (Kumar, Gupta, & Chandna, Citation2014). Inconel 625 is a high strength nickel-based superalloy that is broadly used in aerospace, automobile, heat exchanger, combustors, seals, steam generators and nuclear applications. Because of low thermal conductivity, high temperature and high hardness, machining of this alloy is quite difficult (Parida & Maity, Citation2018). During the milling process, the tool work-piece interface generates extreme friction and intense cutting forces. The energy consumed during the cutting is converted into the heat which is further divided among the work-piece, tool & chip (Vallavi, Gandhi, & Velmurugan, Citation2006). Because of the lower thermal conductivity of the work-piece and the extreme heat generation, thermal expansion causes the deformation in the local area. Nickel-based superalloy work-piece commonly faces such types of challenges in the industries (Cai, Qin, Li, An, & Chen, Citation2014).
Lot of researchers have been worked with aluminium, copper, etc. having high thermal conductivity-based alloy in machining for the prediction of temperature (Kaushik, Subramanian, & Sakthivel, Citation2018; Sivasakthivel & Sudhakaran, Citation2013; Sun, Sun, & Li, Citation2017; Vallavi et al., Citation2006). Sivasakthivel and Sudhakaran (Citation2013) optimized the machining parameters of end milling of AL 6063 for minimum temperature rise by using genetic algorithm and response surface methodology. The measurement of cutting temperatures is more difficult because the temperature is the scalar field which differs throughout the system. An infrared thermometer or pyrometer has also been preferred as the method of measuring temperatures by many researchers because this is a convenient sized, precise, non-contact method of measuring temperatures. The infrared pyrometer has been recognized in the machining processes where high temperatures can be gained easily as there is no direct contact with the heat source (Longbottom & Dailami, Citation2008). Lin and Lui (Citation2001) and Kaushik et al. (Citation2018) accomplished the performance of the pyrometer system that has been shown superior to those of thermocouples for the measurement of cutting temperature. But very few pay attention in low thermal conductive nickel-based alloy like Inconel 625. Due to low thermal conduction, the heat produced in work-piece has less probability for conduction and hence this residual heat in the work-piece responsible for enhancing in temperature ultimately deflection in the work-piece. The problem arises when machine low conductive materials. The heat flowing to the work-piece due to the material removing process remains in the fresh machine surface for a long time (Bhirud & Gawande, Citation2017).
Various statistical and artificial intelligence technique have been applied in the recent past for optimization of machining parameters for different responses such as MRR, SR, Tool life, cutting forces etc. The surface quality, i.e. surface roughness, MRR, etc., has been investigated by many researchers during the machining operation (Cai et al., Citation2014; Mall, Kumar, & Singh, Citation2014). Dabhade, Kathar, and Kadam (Citation2018) determined optimum machining parameters for a multi-response such as Material Removal Rate (MRR), and surface roughness (Ra) using RSM’S D-optimal method by using dry Inconel 625’s turning. Kosarajua et al., (Citation2018) employed Grey relational method by Taguchi for finding the better surface finish and lower cutting force under dry turning of Inconel 625. Cai et al. (Citation2014) have performed an experiment on Inconel 718’s high-speed end milling for its surface integrity. He provided the optimal cutting parameters like surface roughness Ra, surface topography and residual stresses. In the recent past by numerous of the researchers have applied many artificial intelligence techniques such as ant-colony optimization, Simulated Annealing (SA), Genetic algorithm (GA), Particle Swarm Optimization (PSO), etc. for optimization of machining parameters along with the various mathematical modeling techniques such as Fuzzy Logic Methodology (FOR), Artificial Neural Network (ANN), Spot Vector Machining (SVM), etc. in manufacturing (Kaushik et al., Citation2018);Kumar, Gupta, and Chandna (Citation2015); Sivasakthivel & Sudhakaran, Citation2013). Besides this, a few of researchers have studied the temperature prediction of the nickel-based alloy during the milling process. Feng et al., (Citation2018) also developed an analytical model for predicting the temperature variation during the milling of Inconel 718. He also considered microstructure evolution in his studies. At every rotation angle, the milling condition is shifted to the corresponding orthogonal cutting condition. Stoudt et al. (Citation2018) in his research considered the temperature effects on the microstructural stability and the consistency of mechanical properties of Inconel 625. Kitagawa, Kubo, and Maekawa (Citation1997) investigated Temperature and deterioration of cutting tools with the highly spend machine of lncooe1718 and Ti-6AI-6 V-2Sn alloys using numerical analysis and actual cutting experiments. Till date, many scientists have worked on optimizing process parameters of machining of Inconel 625 and Inconel 718 alloys using different methodology (Dabhade et al., Citation2018; Fang, Pai, & Edwards, Citation2013; Feng et al., Citation2018; Kitagawa et al., Citation1997; Raviteja & Sekhar, Citation2015). Mall et al. (Citation2014) optimized four parameters such as depth of cutting, nose radius, cutting speed of end milling and feeding rate of Inconel 718 with Taguchi method. Similarly, Kosarajua et al. (Citation2018) used Taguchi technique for optimizing Inconel 625’s (superalloy) parameters of high-speed turning.
The objective of this present work is to investigate the work-piece temperature during 2.5-D milling of Inconel 625 alloy considering the parameters such as cutting speed (CS), feed (F), depth of cut (DOC) and step-over (D). During machining, temperature distribution has been measured using pyrometer. Moreover, the statistical model has been developed to optimize the machining parameters to achieve a minimum temperature rise using the Genetic Algorithm. Box–Behnken fractional-factorial experimental design (BBD) model has been considered for three levels of input process parameters. The significance and adequacy of the proposed model and effects of process parameters on the temperature of work-piece have been carried out through ANOVA & regression methodology. The temperature rising due to the interaction effect 2.5 D end milling process parameter has also been analyzed, which assist to select process parameter to maintain a temperature rising minimum. The experiment results have been compared with the predictive regression model. The prognostic model in this study is supposed in generating values of the temperature rising which is close to those readings recorded experimentally. To optimize the machining parameters for minimization of the work-piece temperature Genetic Algorithm has been applied to the prognostic model. Conformational experiments with a 5% percentage error have also been performed to validate the results.
2. Design of experiments and analysis using RSM
2.1. Experimental setup
To access the relations among the input parameters and temperature of the work-piece, three levels have been considered for each input parameter based on the material Inconel 625 and the specification of the machine tool. The experimental runs have been conducted on Ace micrometric group AMS make model MVC-450 vertical, CNC milling machine having the highest spindle speed of 6000 rpm and drive motor of 15 kW as shown in Figure . As per the above discussion, the machining parameters along with their levels are presented in Table . A Maxtech IR1000 non-contact infrared pyrometer has been used in all the temperature-related observations as shown in Figure . The range of temperature measurement is from −13°C to 1050°C, with an accuracy of ±2% and a resolution of 0.1°C. The distance maintained between the pyrometer and work-piece of 30 mm and the response time is less than 250 ms.
Table 1. Input parameters with their levels
The composition of Inconal 625 includes Ni-58%, Cr-22%, Mo-9%, (Nb + Ta)-3.75%, Fe-5%, C-0.1%, Cu-0.5%, P-0.015%, Si-0.15% and Al-0.5% (Parida & Maity, Citation2018). A flat end tungsten carbide 10 mm diameter having two flutes has been used as a cutting tool. The property of Inconal 625 and cutting tool has been tabulated in Table .
Table 2. Mechanical and thermal properties of Inconel 625 and tungsten carbide
2.2. Design of experiment
In the present work Response surface methodology (RSM) has been considered for designing of Experiment. In modern ancient times, modelling and analysis of various manufacturing problems based on several influencing variables have been solved with the help of incorporated mathematical and statistical techniques (Aruna & Dhanalakshmi, Citation2012). It involves the correlation between the response & the variables and also optimizes afterward. In the present work Box–Behnken fractional-factorial design of experiment model (BBD) has been considered for finding the relationship among the input parameters cutting speed (CS), feed rate (F), depth of cut (DOC) and step-over (D) & the response of the work-piece temperature and this yield 29 runs as shown in Table . On comparing the Box–Behnken Design with the central composite design’s (CCDs) it has been observed that in the CCD, the centre point runs are required to detect the presence of any curvature of the response (indicating the start of the quadratic region) and the axial point runs are required for estimating the coefficients of the square terms. However, in Behnken designs the axial points are not needed, because the axial point may represent factor level combinations that are impossible to test due to physical process constraints or prohibitively expensive. Therefore, Behnken designs are more economical designs class than other combinations. (Gunst, Citation2012)
Table 3. Observation table with observed and predicted values of temperature and percentage error
The most effective method, i.e. response surface methodology has been used to analyze the results obtained from factorial experiments due to its effectiveness in modeling and analyzing the engineering problems. With less number of experimentation, RSM provides more information. In the present work, cutting speed, feed, depth of cut and step-over have been considered as the machining process parameters. The response work-piece temperature (T) can be expressed as a function of process parameters cutting speed (CS), feed (F), depth of cut (DOC) and step-over (D).
where ix represents the level of the ith factor in the xth observation.
2.3. Development of statistical regression models for work-piece temperature
A quadratic model with the response to the parameter for BBD is directed by the following relation (Kaushik et al., Citation2018).
where Y represents the response, xi (1, 2, ..., k) are the independent input parameters, is a constant and
,
and αij are the coefficients of linear, quadratic and interaction terms, respectively. where “ɛ” represents the error.
where CS is the cutting speed (rpm), F is feed (mm/min), DOC is depth of cut (mm) and D is step-over in the above table. The predicted values obtained from the regression model are shown in Table in predicted value Column.
2.4. Significance of input parameter with the response
Adequacy of proposed models has been tested by the ANOVA technique. F-ration is larger than the value at a 95% confidence level indicates the adequacy of the quadratic model. The estimated effects of each parameter obtained from ANOVA results based on the quadratic model (29 tests) are shown in Table . The parameters whose p-value is less than 0.05 represented by tick mark (✓) in Table and hence, these parameters are taken as statistically significant parameters.
Table 4. Model suggested for the response using fit summery sequential model sum of square
Table 5. ANOVA of RSM for work-piece temperature
It has been observed from Table that the feed rate is the most significant process parameter contributing 92.26%, whereas the cutting speed and depth of cut contributing 4.45% and 1.49%, respectively, with the work-piece temperature. The term % contribution has been calculated with the help of F-ratio and P values. This indicates that feed and cutting speed are more significant parameters for work-piece temperature. However, DOC, D, CS×F, CS2, F2 and DOC2 are also significant model terms to some extent. F-ratio value of lack of fit is observed as 5.434, this implies that it is not significant compared to the pure error. The Predicted R2of 0.9953 is an insensible agreement with the Adjusted R2 of 0.9983.
To understand the relationship among these most significant input parameters and work-piece temperature, 3D contour and response surface has been plotted as shown in Figures and . An interactive effect of feed rate, cutting speed and depth of cut has a significant effect on temperature. As the most significant parameter feed (F) increases, the work-piece temperature increases to a great extent, however, work-piece temperature increases comparatively with the slower pace with depth of cut and cutting speed. The process parameter step over is insignificant with work-piece temperature. As the feed rate increases, the heat generation and ultimately the cutting temperature increases. Similarly, the cutting speed increases the rate of energy dissipated through plastic deformation and friction. Therefore, an increased rate of heat generation produces more cutting temperature in the work-piece. The increase in depth of cut, remove large quantize of work-piece material, thus contribute to the temperature rise of the work-piece.
Figure 2. Contour plot for work-piece temperature. (a) Work-piece Temperature with F and CS. (b) Work-piece temperature with DOC and F
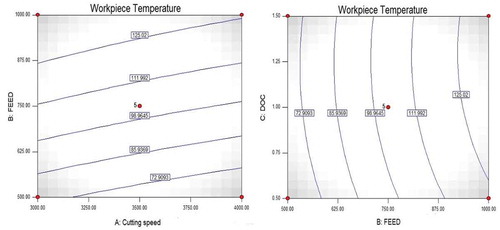
Figure 3. Surface plot for work-piece temperature. (a) Work-piece temperature with F and CS. (b) Work-piece temperature with DOC and F
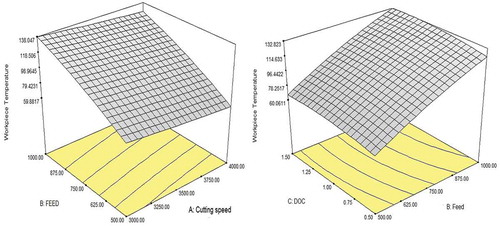
Figure shows the normal probability plot of residuals for work-piece temperature. It has been observed that residual collapse on a straight line which indicates that the errors are normally scattered and the proposed model is adequate. Figure shows the actual work-piece temperature vs. the predicted work-piece temperature. It has been observed that a random scatter fashion of data points divide equally with the straight line denotes the fitness of the model.
From above these results it clearly illustrated that the optimum set of parameters can be familiarized accordingly to minimize the work-piece temperature. Simultaneously, the normal plot of residuals data is normally distributed and all the experimental results are in the segment very close to the predicted results.
3. Optimization using genetic algorithm
The optimal selection ofprocessparametersfor minimizing the work-piece temperature during the milling, Genetic Algorithm has been applied. The Regression Quadratic Model for work-piece temperature obtained from RSM methodology is considered as the objective function in the algorithm. The minimization of the objective function is essential for the normal optimization problem. Using GA solver, present optimization problem has been solved for EquationEquation (1)(1)
(1) and (Equation2
(2)
(2) )
For this 100 population size, (PMX) Crossover function, (RX) Mutation function and 2 elite counts have been considered. The stopping criteria of 100 generations have been considered (Sivasakthivel & Sudhakaran, Citation2013).
The Genetic algorithm (GA) has been computed utilizing the steps given below (Kumar et al., Citation2014).
100 random initial combinations have been created initially by selecting 2.5-D milling process parameters and estimated the fitness value for every combination from the regression model as explained in Figure .
With the biological progression method of the Genetic Algorithm, each combination of the new population has been computedbythe steps given below.
The best fitness value has been obtained using the Rolette wheel selection algorithm for the proper combinations of the process parameter.
The combinations with the slightest fitness value are considered as elite.
Offspring generation has been generated with the combination of mutation and Crossover.
4. The stopping criteria for the algorithm are 100 iterations
The solution is continually improved by each generation and the work-piece temperature obtained for the average solution and the best solution is decreasing successively as shown in Figure . With the help of a diversity of combinations obtained using RX and PMX algorithm, the global minima have been achieved. This procedure continued until the best fitness one chromosome has not been achieved (Kumar et al., Citation2014) as illustrated in Figure .
Figure has been plotted between the number of generations and fitness values for the optimum process parameter of 2.5-D milling. The black dots represent the best fitness value and blue dots signify the average fitness value. Both the graph seems to meet at 52th generation and coincide with each other for trailing generations with the best fitness value (63.66) and no further decline in the fitness value has been predicted as shown and the optimum input parameters are of cutting speed 3945 m/min, feed rate 500 mm/tooth, depth of cut 0.51 mm and step-over 69.99% of tool diameter.
To verify the validity of the response of 2.5-D end milling process parameter five optimum trials of the genetic algorithm along with the confirmatory experiments have been performed in Table . Further, in the estimation of percentage error [10][12], the maximum percentage error obtained is 2.79% and the results of the respective combination from the conformational experiments obtained are also shown in Table .
Table 6. Confirmatory experiments
As illustrated in Figure , the predicted and experimental values are very close to each other. Therefore, it can be concluded that the proposed GA with the regression model has been predicted is much less percentage of errors with the prediction performance and hence is somewhat satisfactory. It has been observed that the minimum value obtained from optimization is more than the one of the experimental value. It seems that the optimization technique might not effective. However, this is due to the difference in the prediction and observed values. Therefore, it can be concluded that the workpiece temperature is also highly sensitive with environment conditions and hence the experiments should be performed under strictly controlled conditions to minimize the noise. Beside this, a better prediction model using artificial technique might also be effective in optimization of workpiece temperature problem.
5. Conclusion
In the present work, the effects of the process parameters of 2.5-D end milling of Inconel 625 nickel-based alloy such as cutting speed, feed, depth of cut and step-over on performance characteristics work-piece temperature has been studied. The following resulting conclusions from the calculation of work-piece temperature from the different process parameters:
The Feed rate (F) is the principally significant parameter and has a positive relationship with the work-piece temperature. As the feed rate increases, due to the low thermal conductivity of Inconel 625, the heat produced in the work-piece has less probability for conduction and hence this residual heat in the work-piece responsible for enhancing in temperature ultimately deflection in the work-piece.
The enhance in cutting speed (CS), feed rate (F) and depth of cut (DOC) increases work-piece cutting temperature to some extent but with a lesser rate as that of feed.
The Forth process parameter step-over (D) has observed a less significant effect with the work-piece temperature.
The process parameters interactions have been analyzed with work-piece temperature and a significant interaction has been observed between feed rate (F) and depth of cut (DOC).
The percentage error in the proposed model is found for less than 5%.
The genetic algorithm (GA) has been found as an effective tool for optimizing the machining parameters for the minimization of work-piece temperature.
The minimum temperature is of 63.66°C has been found with the optimal combination of machining parameters as3945 rpm, 500 mm/min, 0.5 mm and 69.9 mm for cutting speed, feed rate, depth of cut and step-over respectively. It has been observed that the minimum value obtained from optimization is more than the one of the experimental value. It seems that optimization technique might not effective. However, this is due to the difference in the prediction and observed values. Therefore, it can be concluded that the workpiece temperature is also highly sensitive with environment conditions and hence the experiments should be performed under strictly controlled conditions to minimize the noise. Beside this, a better prediction model using artificial technique might also be effective in the optimization of workpiece temperature problem.
Correction
This article has been republished with minor changes. These changes do not impact the academic content of the article.
Additional information
Funding
Notes on contributors
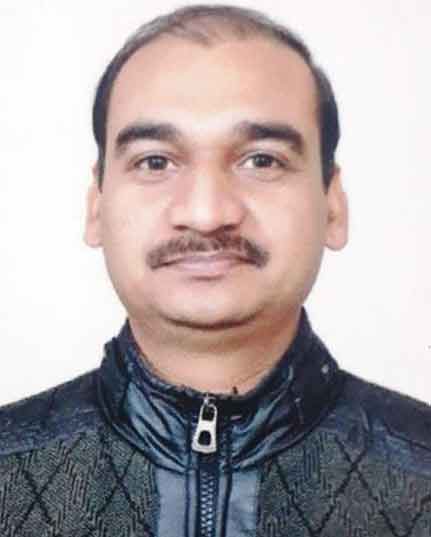
Satish Kumar
Satish Kumar is a research scholar in the Department of Mechanical Engineering at NIT Kurukshetra Haryana INDIA. He has obtained B.E. in Mechanical Engineering from Maharishi Dayanand University, Rohtak, Haryana INDIA and M.Tech in Mechanical Engineering from Guru Jambheshawar University of Science & Technology, Hisar, Haryana INDIA. He has guided many to undergraduate & post graduate students on projects thesis. His areas of research include: CAD/CAM, Finite element analysis, Manufacturing science and Processes, Statistical methods used in research, Artificial Intelligence Technique for modelling, Advance Optimization Technique etc.
References
- Aruna, M., & Dhanalakshmi, V. (2012). Optimization of turning parameters of Inconel 718 alloy using RSM. International Journal of Manufacturing Technology and Management, 25(1/2/3), 113–17.
- Bhirud, N. L., & Gawande, R. R. (2017). Optimization of process parameters during endsMilling and prediction of work-piece temperature rise. Archive of Mechanical Engineering, 64(3), 327–346. doi:10.1515/meceng-2017-0020
- Cai, X., Qin, S., Li, J., An, Q., & Chen, M. (2014). Experimental investigation on surface integrity of end milling Nickel-Based Alloy—Inconel 718. Machining Science And Technology, 18, 31–46. doi:10.1080/10910344.2014.863627
- Dabhade, D. S., Kathar, R. A., & Kadam, M. S. (2018). Optimization of process parameters during dry turning of Inconel 625 by using sialon ceramic insert. International Journal of Engineering Science Invention (IJESI), 7(11), 38–44.
- Fang, N., Pai, P. S., & Edwards, N. (2013). A comparative study of high-speed machining of Ti–6Al–4V and Inconel 718 - part I: Effect of dynamic tool edge wear on cutting forces. The International Journal of Advanced Manufacturing Technology, 68(5–8), 1839–1849. doi:10.1007/s00170-013-4981-2
- Feng, Y., Pan, Z., & Liang, Y. (2018). Temperature prediction in Inconel 718 milling with microstructure evolution. The International Journal of Advanced Manufacturing Technology, 95(9–12), 4607–4621. doi:10.1007/s00170-018-1581-1
- Gunst, R. F. (2012). Response surface methodology: Process and product optimization using designed experiments. Journal Techno Metrics, 38(3), 284–286. doi:10.1080/00401706.1996.10484509
- Kaushik, V. S., Subramanian, M., & Sakthivel, M. (2018). Optimization of processes parameters on temperature rise in CNC end milling of Al 7068 using hybrid techniques. Materials Today: Proceedings, 5, 7037–7046.
- Kitagawa, T., Kubo, A., & Maekawa, K. (1997). Temperature and wear of cutting tools in high-speed machining of Incone1718 and Ti-6A1-6V-2Sn. wear, 202, 142–148. doi:10.1016/S0043-1648(96)07255-9
- Kosarajua, S., Kumar, V., & Nc, S. (2018). Optimization of machining parameter in turning Inconel 625. Materials Today: Proceedings, 5, 5343–5348.
- Kumar, S., Gupta, A. K., & Chandna, P. (2014). Minimization of non-productive time during 2.5D milling. World Academy of Science, Engineering and Technology, 8(6), 1155–1160.
- Kumar, S., Gupta, A. K., & Chandna, P. (2015). Optimization of neural network parameters using Grey–Taguchi methodology for manufacturing process applications. Proceedings of the Institution of Mechanical Engineers, Part B, 229(14), 2651–2664.
- Lin, J., & Lui, C. Y. (2001). Measurement of cutting tool temperature by an infrared pyrometer. Measurement Science and Technology, 12, 1243–1249. doi:10.1088/0957-0233/12/8/335
- Longbottom, J. M., & Dailami, F. (2008). Work-piece temperature prediction by model and measurement by pyrometer. Aircraft Engineering and Aerospace Technology, 80(4), 378–385. doi:10.1108/00022660810882746
- Mall, V. K., Kumar, P., & Singh, B. (2014). A review of optimization of surface roughness of Inconel 718 in end milling using Taguchi method. International Journal of Engineering Research and Applications, 4(12), 103–109.
- Parida, A. K., & Maity, K. (2018). Comparison the machinability of Inconel 718, Inconel 625 and Monel 400 in hot turning operation. Engineering Science and Technology, an International Journal, 21(3), 364–370. doi:10.1016/j.jestch.2018.03.018
- Raviteja, P., & Sekhar, S. R. (2015). Optimization of process parameters for milling of Nickel Alloy Inconel 625 by using Taguchi method. SSRG International Journal of Mechanical Engineering, 2(2), 14–20.
- Sivasakthivel, P. S., & Sudhakaran, R. (2013). Optimization of machining parameters on temperature rise in end milling of Al 6063 using response surface methodology and genetic algorithm. International Journal of Advance Manufacturing Technology, 67, 2313–2323. doi:10.1007/s00170-012-4652-8
- Stoudt, M. R., Lass, E. A., Ng, D. S., Williams, M. S., Zhang, F., Campbell, C. E., … Levine, I. E. (2018). The influence of annealing temperature and time on the formation of d-phase in additively- manufactured Inconel 625. Metallurgical and Materials Transactions, 49(7), 3028–3037. doi:10.1007/s11661-018-4643-y
- Sun, Y., Sun, J., & Li, J. (2017). Modelling and experimental study of temperature distributions in end milling Ti6Al4V with solid carbide tool. Proceedings of the Institution of Mechanical Engineers, Part B: J Engineering Manufacture, 231(2), 217–227. doi:10.1177/0954405415577553
- Vallavi, A. M. S., Gandhi, M. D. N. &., & Velmurugan, C. (2006). Prediction of temperature rise in end milling of Al/SiCp metal matrix composite using response surface methodology. Asian Journal of Research in Social Sciences and Humanities, 6(7), 787–796. doi:10.5958/2249-7315.2016.00463.9