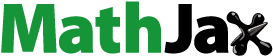
Abstract
The modelling and optimization of gear systems is a complex procedure with results dependent on the conceptual formulation of the problem, uncertainty, bias and imprecision in weight assignment. Furthermore, tacit information is often omitted in design processes. In this study, a gear concept selection procedure considering “voice of customer” VOC and tacit statements is discussed while extending a gear selection matrix introduced by N. Marjanovic In the current research, two case studies are investigated while considering tacit knowledge. To demonstrate this concept, fuzzy logic is applied to interpret linguistic evaluations by both customer and design teams in the (QFD) Quality Function Deployment and to select alternatives using the Fuzzy-AHP method. The obtained results reflect the impact of tacit knowledge on gear selection methods.
PUBLIC INTEREST STATEMENT
The main quest in any decision is to find the best compromise amongst numerous objectives. This research work also highlights this challenge in todays engineering problems and how computer tools can enhance problem solving. This research is focused on incorporating tacit knowledge in the gear concept selection. The voice of customer, tacit statements and a concept selection matrix are employed while ranking tools are applied to select alternatives. The method proposed allows uncodified knowledge to be introduced into the design process so as to add value.
1. Introduction
Gear systems are major contributors to the functionality of electric vehicles (Han et al., Citation2019), wind and thermal power generation units. However, gears contribute an average of 10–20% in energy losses depending on the type of gear applied (Radzevich, Citation2012). Early research in gear optimization points to the use of numerical procedures and computational tools. On the other hand, the combined application of computation, mathematical models and evolutionary techniques is common in the current literature (Arora et al., Citation2016, Citation2015). A multistage gear system for a rotary tiller was investigated by (Maputi & Arora, Citation2019) while using the bounded objective method. In other research work, (Stefanović-Marinović et al., Citation2017), focused on the application of a weighted sum method to formulate an aggregate objective function. Variable influence and relation are key to formulating an accurate mathematical description of an optimization problem. (Miler et al., Citation2017) investigated the impact of constraints on spur gear parameters and subsequently analyzed the effect of profile shift on spur gear optimization. Such research reflects the need to understand decision variables parameters and objective relations. Geometric and material specifications in a gear optimization problem were articulated using Decision-based design (DBD) framework inclusive of Analytical Hierarchy Process (AHP), Axiomatic Optimization (AD) axioms and Multiple Attribute Utility Theory (MAUT) (Das et al., Citation2016). Conceptual thinking influences decision making in engineering design (Ognjanovic, Citation1996). In other words, gear optimization is a decision-making process (Das et al., Citation2016), whose output is substantially dependent on primal decisions and orientation of the decision-maker (Wan & Krishnamurty, Citation2015).
Therefore, the role of practical experience in the optimization of gear systems cannot be overstated. In the conceptual phase, technical expertise, product specifications and voice of the customer (VOC) are the main sources of information. The former requires various methodology to interpret while the latter includes non- specific information herein defined as tacit knowledge (Marini et al., Citation2016). According to (Mcmahon et al., Citation2004), while discussing the management of knowledge in engineering optimization, the terms tacit and explicit references to personal and company knowledge respectively in the case of an engineering firm. Another researcher defines tacit knowledge as non-codified (Murphy et al., Citation2004), person-embodied, (Nightingale, Citation2009) knowledge. The input and effect of tacit knowledge in the engineering optimization process is a challenge that many decision-makers experience. Furthermore, tacit knowledge is not universally interpreted and will vary depending on experience and environmental circumstances (Murphy et al., Citation2004), peculiar to the area of impression.
However, surveyed literature indicates, concerning gears, that more work, focuses on the mathematical synthesis of gears (Hofstetter et al., Citation2018; Miler et al., Citation2018). (Malgorzata & Patalas-Maliszewski, Citation2015) argues that employee skills set in the form of codified knowledge have more value that company assets. To this end, researchers have sought to capture tacit knowledge. (Wahid et al., Citation2018) applied ontology to identify tacit knowledge from experts before they retire or die. According to (Puteh, Citation2018), optimization can be greatly enhanced by capturing tacit knowledge. In gear technology, methods such as Integrated Design System (IDS) (Karayel et al., Citation2013), have been applied to capture knowledge but fall short in terms of tacit knowledge. Computer-aided design (CAD) based methods have been applied to try and understand designer intent and thinking patterns (Sung et al., Citation2011). Tacit knowledge is fuzzy, while gear optimization problems are defined mathematically, implying that preferences must be evaluated to a numerical scale that translates to objective weights (Marler & Arora, Citation2004). Furthermore, due to imprecision, uncertainty and a vague description of some preferences (Li et al., Citation2011), biased numerical values may be appropriated for such preferences. To mitigate this challenge researchers have applied fuzzy-based techniques (Das et al., Citation2016; Fu et al., Citation2018; Luthra & Mangla, Citation2018; Mendi et al., Citation2010; Nallusamy et al., Citation2016). Very few researchers such as (Mahiddini et al., Citation2019) have considered customer preferences in gear design hence the following statements reflect the observations by the authors:
Tacit knowledge from experience is not captured in gear optimization processes
Lack of preference articulation of the VOC in practical gear optimization parameters
In this study, two gear concept selection case studies are investigated using a fuzzy influenced method integrating QFD, gear concept selection matrix, AHP and tacit statements. A concept selection matrix introduced by (Marjanovic et al., Citation2012) is extended and applied in this research. In section 2, the gear concepts, selection matrix and tacit statements are discussed.
Section 3 discusses QFD, AHP and Fuzzy logic. The proposed method is discussed in section 4 of the paper while section 5 discusses the method applied in the current research work. The results and discussions are also presented in section 6 while the conclusion is set in section 7. The concept selection matrix is shown in the Appendix 1.
2. Gear selection matrix
The following statements summarize the decisions to be made in a general gear concept selection process:
Selection of gear concept either by considering the type of gear system and shaft orientation.
Material selection.
Selection of geometric parameters for gears and shafts.
Selection of bearings.
In this section, a concept selection matrix introduced in literature (Marjanovic et al., Citation2012) is extended, as shown in Appendix 1, and discussed. General tacit statements are also introduced and discussed. This research work is focused on the gear concept selection only.
2.1. Concept selection matrix
The concept selection matrix applied in this research work was introduced by (Marjanovic et al., Citation2012), while considering spur gears and how to carry out optimization practically. In this study, the concept selection matrix is extended to include 32 concepts from the previous 28 concepts initially proposed by previous authors.
The current gear selection matrix does not consider planetary gears hence four new concepts based on planetary gear systems are introduced. To consider more than one gear type viz. bevel, worm and helical, the respective classifications have also been added to each concept. As such the selection of gear type is not predetermined as observed in previously published research. Furthermore, the mounting configuration of gearboxes is key in gear concept selection and this was not included in previous literature. The gear concepts considered in this research are housed units and the arrangement of internal gears on shafts for overhung or straddle gears was not considered. Decision making in gear systems is a complex process. Nevertheless, the numeracy of such methods and the variation in implementation testifies to the uniqueness of each problem.
Two types of gearbox mounting configurations were also included in the description of gear systems in the concept selection matrix. The concept coding system applied in this new selection matrix is similar to that applied by previous authors. Each gear concept is identified with a description, schematics, code, shaft configuration, gear ratio range, efficiency range, mounting configuration and possible gear types. For the coding system, the following was applied:
“S”—spur gear
“B”—bevel gear
“W”—worm gear
“H”- helical gear
Concerning shaft orientation:
“P”—parallel
“I”—intersecting
“A”—skew or
“C”—coincident
2.2. Tacit knowledge
Engineering optimization is a decision-making process (Renzi et al., Citation2017) in which a trade-off of various criteria/objectives is performed to obtain an optimal solution. Philosophically, an engineering designer utilises knowledge from various scientific (Pahl et al., Citation2007), social and cultural domains to produce a product. In a study carried out by (Salter & Gann, Citation2003) considering how innovation is inspired in engineering firms, conclusions signalled that peer to peer interaction accounted for substantially more results compared to the use of computer-aided tools. Furthermore, final product designers do not usually interface with the actual customer although the designer’s preferences may affect the outcome. Essentially, these conclusions indicate that there is personified knowledge, which aids innovation in optimization outputs. Such knowledge which is possessed by a particular person is known as uncodified knowledge [14]. Conterminously, other researchers concerning uncodified knowledge employ the term “tacit”. Although the term may refer to the intent of the action, another researcher (Li & Gao, Citation2003) applies the term to knowledge which is not easily expressed. In this research, this latter definition is adopted for preferences, which cannot be easily represented by numerical representation. Furthermore, these preferences are related to the designer’s perspective, rather than the customer’s perspective (Salter & Gann, Citation2003). The tacit statements applied in the current work are shown in Table have been obtained from literature and the experience of the authors in the maintenance of such gear systems.
Table 1. Tacit statements in gearbox optimization
3. Decision methodology
Decision methods may be classified into three categories viz. multi-criteria decision methods (MCDM), decision-making problem solving (DPS) and problem structuring methods (PSM) (Renzi et al., Citation2017). MCDM’s are further classified into multi-objective decision methods (MODM) and multi-attribute decision methods (MADM). AHP, VIKOR, (PROMETHEE) Preference Ranking Organisation Method for Enrichment Evaluations are examples of MADM methods. DPS methodology, on the other hand focuses on the conceptual stages of a decision problem. Quality Function Deployment (QFD), Theory of inventive solving (TRIZ), Axiomatic optimization (AD) and Pugh’s controlled Convergence (PuCC) are identified as decision techniques.
In this research the QFD method is applied to manage the translation of VOC statements while AHP is employed to manage the selection of alternatives/concepts. In this section, original QFD and AHP methods are first discussed while the fuzzified methods are dealt with in section 3.3.
3.1. QFD
QFD is a customer-oriented tool that is used to assure and improve customer satisfaction (Yan & Ma, Citation2014). Practically, it manages the translation of VOC statements voice into DR’s, production and manufacturing requirements (Lu et al., Citation2016), in unison (Hazelrigg, Citation2013). Visually it demonstrates this translation through a series of charts that reflect the correlation of VOC and DR statements. The “voice of customer” (VOC) concept (Renzi et al., Citation2017) refers to the expression of desires, expected values and performance of the product.
The QFD method captures product intent and perspectives from the consumer. A listing of customer needs is evaluated using an arithmetic scale with an incremental degree of importance (Pahl et al., Citation2007). According to (Madu, Citation2006) customer perceptions are subjective and require translation to remove uncertainty. In response to the VOC statements, DR’s, are interpreted by engineers to provide quantifiable and measurable specifications (Liu & Boyle, Citation2009). In some cases where multiple customers are interviewed VOC statements may overlap, there a hierarchical structure is used to address duplication and rationalize the VOC statements. As such, the correlation matrix forming the cone in Figure focuses on this aspect. The QFD, house of quality system, incorporates progress or target management modules such as competitive analysis, goals and degree of complexity. However, in this research work is focused on the relationship matrix in the QFD structure concerning VOC and DR statements. To remove bias and uncertainty, the VOC and DR linguistic statements have been translated using triangular fuzzy numbers. This concept is discussed in section 3.3.
3.2. AHP
AHP is classified as a Multi-Criterion Decision-Making method (MCDM). Generally, this technique ranks optimization alternatives to determine the optimal alternative that satisfies the criterion under consideration. Its popularity is credited due to its ease of application and a structurally simplistic procedure (Velasquez & Hester, Citation2015). The AHP technique is applied to manage criterion definition transition from uncertainty to increased certainty or clarity (Hermann et al., Citation2016). In AHP, multiple alternatives are compared pairwise to a criterion at a higher level. Figure shows the general steps that are carried out when executing AHP. The AHP technique is generally comprised of five steps as follows:
Pairwise comparison of optimization alternatives is done for each objective desired by the decision-maker. The alternatives are compared by rating their preferences using the scale suggested and presented by (Li et al., Citation2011), (Kilincci & Onal, Citation2011) as shown in Table .
Computation of preference vectors for each optimization/decision alternative is done by prioritizing each criterion.
For each criterion, the preference values are summed column-wise and a quotient of each value and this overall sum is obtained to normalize the preference values. These new values are then averaged row-wise to obtain preference vectors.
Determine the relative importance of each objective- The criterion is now compared pairwise to obtain preference values which are also normalized in the same manner as in (2) to obtain a set of preference vectors for criteria. Summation of Matrix multiplied values obtained in (2) and (3) computes the overall score for each alternative for ranking
Ranking- Alternatives are now ranked according to the score obtained in (3)
Consistency index—This index is evaluated by comparing it to the random index with a ratio of less than 0.1 reflecting acceptable consistency while otherwise reflects doubt in consistency. If the value is valid the preference vectors obtained are accepted or otherwise the process restarts.
Table 2. Preference schedule (Ayhan, Citation2013)
Fuzzy AHP (F-AHP) is an extension of the original AHP method and contributes fuzzy theory to the hybrid method. As shown in this section, the AHP method does not cater for vagueness in personal judgments, therefore fuzziness is introduced. The Fuzzy-AHP procedure is discussed in section 3.3.
3.3. Fuzzy logic
Since the introduction of fuzzy set theory (FST) by (Zadeh, Citation1996) numerous research articles focused on applications, and novel hybrid methods have been published. FST manages uncertainty due to human perception and linguistic limitations. A membership function as shown in EquationEq.1(1)
(1) denotes FST where the grade of association is rated on a scale of 0 to 1. The extrema values denote maxima and minimum association while intermediate grades reflect partial association.
X = {x} denotes all real values of the linguistic variable x, α, β and γ denote triangular fuzzy numbers. In this research fuzzy logic is applied to both QFD and AHP methods to remove uncertainty and vagueness in linguistic judgements or statements. In this section, computation procedures for F-QFD and F-AHP are presented.
3.3.1. Fuzzy-QFD
To remove imprecision and vagueness in the voice of customer linguistic statements, a triangular fuzzy method has been applied in this research. In the QFD method, the customers and the design team make importance ratings using linguistic terms. In the current research, seven terms denoting varying degrees have been adopted (Akman & Ozcan, Citation2011) and are presented in Table .
Table 3. Fuzzy-QFD triangular scale
Generally, in the QFD process, the voice of customer expressions are derived from more than one customer or stakeholder. Each customer provides a rating of each voice of customer statement and the triangular values are averaged against the number of customers as shown in EquationEq. 2(2)
(2) .
Where w-weight, d-number of customers, n-number of VOC statements. The fuzzy numbers obtained for each expert are then computed using EquationEq. 3(3)
(3) , thereby determining the total rating.
The weight obtained using EquationEq. 2(2)
(2) and the rating from EquationEq. 3
(3)
(3) are then computed using EquationEq. 4
(4)
(4) , thereby resulting in a weight factor ascribed to design requirements.
To rank DR’s, scores are generated using EquationEq. 5(5)
(5) .
3.3.2. Fuzzy-AHP
A fuzzy triangular scale described and shown in Table is applied for pairwise comparison of alternatives and criteria (Kilincci & Onal, Citation2011). In this research, Buckley’s method (Ayhan, Citation2013) of determining fuzzy priorities for comparison ratios is applied to determine importance weights.
The Triangular Fuzzy computational procedure is as follows:
Step 1: Criteria comparison
Elements or criteria are compared using linguistic expressions and triangular fuzzy numbers shown in Table above. Therefore, considering a pair of elements, if element 1 is considered as W.Imp to element 2, it is allocated FT scale of (2, 3, 4) while element 2 will adopt the FT scale of (1/4, 1/3, 1/2). As shown in EquationEq. 6(6)
(6) , matrix A shows a pairwise comparison. In the matrix
denotes ith criterion/element preference over jth criterion using fuzzy triangular scales.
Step 2: After evaluating various decision-makers, the individual preferences are then summed and averaged by the number of decision-makers, K, using EquationEq. 7(7)
(7) .
Step 3: A pairwise matrix is updated using the averaged preference values as shown in EquationEq. 8(8)
(8) .
Step 4: The geometric mean of fuzzy comparison values of each criterion is calculated as shown in Eq. 9. Here, denotes the triangular values.
Step 5: For each criterion considered, the fuzzy weights ( are computed as shown in EquationEq. 10
(10)
(10) and for each value of
, the vector summation is calculated and modified to increasing order obtain a reverse vector.
Step 6: Using the centre of area method shown in EquationEq. 11(11)
(11) , the fuzzy triangular numbers are de-fuzzified.
Step 7: The value obtained in step 6 is not a fuzzy number and is hence normalized using EquationEq. 12(12)
(12) .
In the triangular fuzzy method, the seven steps described above are done to determine the normalized weights of criteria and alternatives. Computation of alternative scores is the product of alternative weight and criteria. Ranking of the scores naturally identifies the highest score as the preferred alternative. To further expound on the methodology two case studies are presented in section 5 and 6 of this research.
4. Methodology
Broadly, the articulation of customer preferences may be categorized as either viz. “a priori”, posteriori or progressive articulation. This section focuses on “a priori” preference articulation method employed in this research work and shown diagrammatically in Figure .
The proposed method is composed of four stages viz. VOC evaluation, fuzzy-QFD, concept evaluation and concept ranking using Fuzzy-AHP. In the first stage, VOC statements are collected through interviews and decomposed into hierarchies. The rationalisation of VOC’s is also done to avoid duplication of statements. In most cases, more than one customer evaluation is recorded. In the second phase, relative importance weights are computed for each customer and aggregated to result in a single importance computation set.
A design team then translates the VOCs into DR’s. Since the designers are also prone to bias, fuzzy operators are also applied to compute the importance weights of the design requirements. It is important to note that the QFD process is intended to translate the voice of the customer to design requirements so that customer wishes are implemented. As such the use of DR’s is merely a translation of customer wish to scientific, mathematical or mechanical specifications for implementation. In this exercise fuzzy logic has been applied to avoid loss of intent and to improve the accuracy and repeatability of the process.
Phase 3 introduces the concept selection, in which tacit statements are used to evaluate the gear system options provided in the matrix. Tacit knowledge is somewhat dynamic as it may reveal new information as the conceptual exercise advances. Knowledge from experience is assumed to have been acquired from the behaviour of available concepts. The weighting for
each criterion is computed by summing the product of the customer requirement value and its respective scaling factor. The relative importance is then calculated as a percentage of each respective weight to total weighting for all criteria. Simultaneously the selection of alternatives is done from the available concepts. In this stage, the pith of tacit knowledge is reflected. Experience and knowledge are derived from interaction with various configurations based on mechanisms. These processes simultaneously converge into the concept selection stage. The selected concepts are handed over to phase 4, where the ranking of concepts is done using a fuzzy-AHP procedure. Basically, in this stage, evaluation of particular alternatives against given criteria, herein defined as the set of objectives, is performed. The AHP method is applied in the concept selection stage of the proposed model to determine concept ranking against objective preference.
5. Concept selection application
A concept selection procedure that incorporates tacit knowledge, QFD, AHP and Fuzzy logic is introduced in this research work. The procedure is demonstrated on two gearbox selection case problem for two conveyor applications in an industrial plant as shown in Table . In this section, the application to these case studies is demonstrated. According to Table , the input and final rpm indicate that the required gear ratio is 4 since the gear ratio is the ratio of the two variables. A gearbox mounted on an inclined conveyor is usually shaft-mounted whereas a horizontal conveyor can be base mounted.
Table 4. Application specifications
5.1. Case study 1
5.1.1. Phase 1 VOC evaluations
In this section, the gear concept selection problem for an industrial plant is discussed. Customer interviews were conducted at the company premises and three personnel from engineering, purchasing and production departments participated in the survey. The twelve VOC statements obtained are shown in Table .
Table 5. Voice of customer statements
According to the engineering personnel, a gear system should not be too complex because it will require special skill to repair, not too heavy so that workers are not injured in the process of installation or otherwise the use of extra equipment. Generally, machinery with fewer parts is easier to maintain. Furthermore, machinery with fewer vibrations are more durable and will result in fewer failure rates. The customer from the production department was concerned about the ability of the gear system to continue operating when the machinery is overloaded, and when production demands require a 24-hour working period with fewer failure rates. The ability to apply the gear unit to multiple operations was also key. The purchasing department was more concerned about acquiring a gear system at the least purchase and running costs.
The acquired VOC statements were composed into a hierarchical structure of four main classes’ viz. low cost, easy maintenance, reliable and more power output comprised of three VOC statements each as shown in Figure . Minimum cost option, long service life and local parts availability VOC statements were grouped under low-cost class as these are related to the purchase and ownership costs of the gear system. The easy maintenance class encompasses VOC statements viz. not complex, few parts and not heavy. The ability to withstand shocks, 24-h usage and less likelihood of breakdowns composed the reliability class. The class for more power output is described overload tolerance, energy-saving and multiple application probability of chosen concept.
5.1.2. Phase 2 Fuzzy-QFD
To apply the Fuzzy-QFD process, the triangular scale shown in Table is applied for evaluation of VOC statements as shown in Table . The weight values for each VOC and DR statement is computed by applying EquationEq. 2(2)
(2) and the results are shown in Tables and . The rating of VOC and DR correlation is obtained using EquationEq. 3
(3)
(3) and together with aggregated weight values, a weight factor is ascribed for DR’s. The final score is computed using EquationEq. 5
(5)
(5) thereby obtaining DR ranking as shown in Table . According to the results obtained in Table the most important DR is power output at 0.35 followed by volume, weight and centre distance at 0.24, 0.21 and 0.2, respectively.
Table 6. VOC evaluations
Table 7. DR evaluations
5.1.3. Phase 3: concept evaluation
The concept evaluation stage applies tacit knowledge to the concept selection matrix to eliminate concepts and remain with the selected number of possible concepts that will be evaluated using the Fuzzy-AHP method. In this research, tacit statements will be derived from two sources, as highlighted in Table and from application specifications in Table .
In the latter, the authors seek to demonstrate the generation of tacit knowledge during the conceptualization process. Furthermore, each concept will be evaluated independently. In this section, the concepts are evaluated using (1) tacit knowledge derived from application specifications and (2) using experience-based tacit knowledge as shown in Table .
5.1.4. Tacit knowledge (specification derived)
Concerning case study 1 and according to Table the chosen gearbox should have a ratio of 4, be shaft mounted. In the concept selection matrix, the gear ratio and mounting type parameters can be used to apply these requirements. Table shows the summary of the evaluation reflecting the concepts that meet the two requirements derived from specifications. Therefore the acceptable concepts are concept 1, 3, 4, 5, 6, and 29.
Table 8. Possible concepts (case study 1)
5.1.5. Tacit knowledge (experience derived)
The tacit statement applied in this section has been introduced in Table and are assumed to be derived from experience. Table shows an overall assessment using this type of tacit knowledge but considering the shortlisted concepts using specification derived tacit knowledge. Where the “+” symbol reflects a vote for the concept on a tacit statement while, ‘- ‘symbol reflects a vote against. A collation of the “+” votes is then given as the total preference vote.
Table 9. Application of tacit knowledge
Tacit statement no.1 refers to lower volume capability of planetary gearboxes based on low base plate area. This is an optimization inherent advantage for planetary gear. Therefore, considering the selected concepts, only concepts no. 29 is planetary based hence a preference vote in its favour is awarded. Tacit statement no. 2 is also on planetary gears but expresses their negative impact on cost and ease of maintenance. In this case, all other concepts are awarded votes in their favour. The intended application in case study 1 is for an inclined conveyor belt system.
Since the gear drive is installed at the elevated position, rigging equipment may be required for installation and maintenance operations. Therefore, the chosen concept must have low weight and volume properties and as such, concepts no. 3 and 29 are chosen. Based on the mounting configuration, volume and weight considerations resulted in favour for concept no. 4, 5 and 6. The overall gearbox efficiency, led to the selection of gear concepts 1, 4, 5 and 6 as more preferred to the rest. The generated preference vote suggests that concepts no. 1, 3, and 29 are the least preferred while concepts no. 4, 5 and 6 are the most equally preferred concepts.
5.1.6. Phase 4 concept raking using Fuzzy -AHP
The fuzzy-AHP method described in section 4 of this research work is implemented in this section whilst deriving preference vectors from phase 2 and alternatives from phase 3. In other words, the weights are assigned based on the ranking obtained in the Fuzzy-QFD process. The fuzzy-AHP method is comprised of seven steps, starting with pairwise comparison as shown in Table . DR’s viz. power output, volume, weight and centre distance have been ranked at 0.35, 0.24, 0.21 and 0.20 respectively as shown in Table . The DR weights applied in this section are derived from Table .
Table 10. Pairwise comparison of all criteria
In this study, only one decision-maker is employed hence step 2 is omitted. The geometric mean is calculated using EquationEq. 4(4)
(4) and tabulated as shown in Table . Each value of the geometric mean is now summed, modified to increasing order to obtain a reverse vector. Relative fuzzy weights Wi are calculated for each criterion as shown in Table using EquationEq. 5
(5)
(5) .
Table 11. The geometric mean and relative fuzzy weights
Using EquationEq.6(6)
(6) the average (Mi) and normalized (Ni) values are obtained as shown in Table . Table –1 show the pairwise comparison done for all concepts according to each criterion.
Table 12. Average and normalized weight
Table 13. Pairwise comparisons based on weight
Table 14. Pairwise comparison based on volume
Table 15. Pairwise comparison based on power output
Table 16. Pairwise comparison based on centre distance
For each alternative and criterion Table shows the normalized relative weights while the aggregated results are shown in Table . Ranking of these final concepts is done resulting in concept 6 as the chosen concept.
Table 17. Normalized non-fuzzy relative weights of each alternative for each criterion
Table 18. Aggregated results for each alternative according to each criterion for case study 1
5.2. Case study 2
The gearbox selection problem investigated as case 2 is to be applied to a horizontal conveyor as compared to the inclined conveyor application discussed in case 1. In this research, the customer preferences applied in case study 1 are similar to those applied in case study 2. Therefore the DR weights applied in case study 1 have been applied to case study 2. Therefore for the sake of brevity, results shown in Table are applied in this section where DR weights were recorded as 0.35, 0.24, 0.21, and 0.20 for power output, volume, weight and centre distance respectively. Furthermore, only Phase 3 is discussed in full while phase 4 results are only are reported.
5.2.1. Tacit knowledge (specification derived)
Two parameters gear ratio and mounting type were considered by the design team as shown in Table . As an initial screening procedure, these two parameters were derived from the input, output rpm and type of conveyor. Horizontal conveyors are closer to the ground or platform and hence there is no requirement for lifting applications. Furthermore, gear mounting will be preferably base mounted. Gear concepts that could attain a gear ratio of 4, with base mounting were selected as possible concepts. Seven concepts that satisfy the given technical criteria viz. concepts 1, 2, 3, 4, 5, 6 and 29 were selected.
Table 19. Possible concepts
5.2.2. Tacit knowledge (experience derived)
The tacit statements applied in this section are shown in Table and are derived from the literature and experience of the authors.
Table shows an overall assessment of the previously selected concepts using tacit knowledge and the preference votes for each concept. For case study 2, the intended application is for a horizontal conveyor system. In this case, the gearbox is base mounted with lesser considerations for weight as compared to volume.
Table 20. Tacit knowledge application
The generated preference vote suggests that concepts no. 3 and 29 are the least preferred while concepts no.1, 2, 4, 5 and 6 are the most, equally preferred concepts.
5.2.3. Phase 4 concept rating using fuzzy-AHP
The fuzzy-AHP method described in section 4 of this research work is implemented in this section whilst deriving preference vectors captured in Table for the Fuzzy-QFD process. Concepts selected in the previous step of case study 2 were analysed and the final ranking obtained is shown in Table . Accordingly, concept preference obtained for concepts 4, 2, 1, 5, 6 are 0.50644, 0.2560, 0.1250, 0.0663 and 0.0460, respectively. Therefore concept 4 is the selected concept.
Table 21. Aggregated results for each alternative according to each criterion for case study 2
6. Discussions
Two gear selection case study problems were discussed in section 5 while applying the proposed methodology described in section 4. Case study 1 focused on the design of an inclined belt conveyor while case study 2 focused on the design of a horizontal belt conveyor.
In both cases the input, output rpm and working conditions of the gear systems are similar. Voice of customer statements collected from three customers in different departments of the industrial setup was decomposed in a hierarchical structure to group similar statements. Using the Fuzzy QFD method preference weights were assigned using customer evaluations and designer requirements were interpreted from the customer requirements. Tacit statements were used to shortlist gear concepts in the selection matrix. These were evaluated in a Fuzzy-AHP process using importance ratings generated in the Fuzzy QFD.
Results obtained in section 5 indicate that in case 1, concept 6 was selected for the inclined conveyor while concept 4 was selected for the horizontal conveyor.
6.1. Evaluation of solutions
Concerning case study 1 where concept 6 was selected, the selection matrix shows that the gearbox is of double stage configuration and can achieve a wide spectrum of speed ratios. Concept 6 can be shaft base or flange mounted thereby making it suitable for multiple applications. Concept 6 can be applied for helical and spur gear tooth forms. Literature, (Gearboxes for incline conveyors, Citation2020) indicates that helical gearboxes are best suited for inclined conveyor as compared to worm gearboxes. Furthermore, helical gearboxes are more efficient and will result in lower running costs as compared to worm gearboxes. On the other hand, worm gearboxes require less purchase cost compared to helical gear systems. The voice of customer statements specified that the selected gearbox must be the least cost option and yet have a long service life. This is captured by concept 6 which emphasizes low running costs, which contribute more to the cost component of the gear system. Concept 6 is also has a coaxial gear arrangement which improves the attainment of low volume and low weight gear structures. (Rall & Staples, Citation2011) confirmed that there is an increased need for gearbox systems that should produce more power with less weight in conveyor systems.
While concept 4 can be a flange, base or shaft mounted. Concept 8 has the potential to have higher volume and weight that concept 6 due to gear arrangement. On the other hand technical requirements for case study 2 specify a base mounted gear system hence weight is a less important factor compared to case study 1 which requires a shaft mounted gearbox. Maintenance repairs on a shaft mounted gear system are greatly affected by weight. The selected concepts, are relevant and can be matched with customer requirements.
7. Conclusions
In this research work, a novel conceptual optimization method that applies decisions based on tacit knowledge has been introduced. The novel method is a hybrid method comprising the relationship matrix of the QFD method and AHP method implemented using fuzzy logic. Voice of customer statements collected from three customers in different departments of the industrial setup was decomposed in a hierarchical structure to group similar statements. Using the Fuzzy QFD method preference weights were assigned using customer evaluations and designer requirements were interpreted from the customer requirements. Tacit statements were used to shortlist gear concepts in the selection matrix. These were evaluated in a Fuzzy-AHP process using importance ratings generated in the Fuzzy QFD.
Two gear case studies are employed to demonstrate the effect of tacit statements on preference articulation results in an optimization process. A gear concept selection matrix adapted from (Marjanovic et al., Citation2012) and extended in the current work is applied. Similar customer requirements and tacit statements are applied to the two gear case studies to select a suitable gear concept.
Resultantly the following was observed from the current research:
There is a lack of formal gear optimization methods that employ tacit knowledge hence in the current work a method that focuses on the conceptual optimization phase has been introduced to incorporate tacit knowledge in preference articulation.
Previous research in gear technology focused on spur gears only while using a concept selection matrix with multiple concepts. In this research work, all concepts were considered and selected based on tacit statements which improved the concept selection.
A gear concept selection matrix was extended by the authors.
Method allows value addition to design knowledge by mechanism that assists discovery of tacit knowledge.
However the following limitations were observed:
The optimal concept is also dependent on the interpretation of tacit statements, which is person-embodied knowledge. In the current work, two gear case study examples with similar customer preferences have resulted in different gear concepts chosen regardless of similar technical requirements. This could be a positive or negative depending on selection.
The method lacks an efficient method for capturing tacit knowledge and assigning importance.
Additional information
Funding
Notes on contributors
Edmund S. Maputi
Edmund Shingirayi Maputi is a lecturer with the Department of Industrial and Manufacturing Engineering, Harare Institute of Technology, Zimbabwe. He is also a PhD Scholar at Amity University Haryana, India. He received his Masters in Engineering Design from the Jawaharlal Nehru Technological University Hyderabad. His research interests include engineering design, optimization, and soft computing. This research work is part of a study on multi-objective optimization focused on gear systems.
Rajesh Arora
Rajesh Arora is working as an Associate Professor in the Mechanical Engineering Department, Amity University Haryana, Gurgaon. He received his Doctorate from the YMCA University of Science & Technology, Faridabad and Masters from Indian Institute of Technology, Delhi. His areas of expertise are finite time thermodynamic analysis, energy and exergy analysis of thermal power plants, heat transfer, solar photovoltaics, computational fluid dynamics, multi-objective optimisation and decision making. He has made significant contributions in these fields as evident by his above 30 research publications in journals of repute at national and international levels.
References
- Akman, G., & Ozcan, B. (2011). A fuzzy quality function deployment (QFD) approach to determine customer needs for driving mirror. İstanbul Ticaret Üniversitesi Fen Bilimleri Dergisi Bahar, 2011(19), 1–21. http://acikersim.ticaret.edu.tr/xmlui/bitstream/handle/11467/605/M00431.pdf?sequence=1&isAllowed=y
- Arora, R., Kaushik, S. C., & Arora, R. (2015). Multi-objective and multi-parameter optimization of a two-stage thermoelectric generator in electrically series and parallel configurations through NSGA-II. Energy, 91(2015), 242–31. https://doi.org/10.1016/j.energy.2015.08.044
- Arora, R., Kaushik, S. C., & Arora, R. (2016). Thermodynamic modelling and multi-objective optimization of a two-stage thermoelectric generator in an electrically series and parallel configuration. Applied Thermal Engineering, 103(2016), 1312–1323. https://doi.org/10.1016/j.applthermaleng.2016.05.009
- Ayhan, M. B. (2013). A Fuzzy AHP approach for supplier selection problem : A case study in a gear motor company. The International Journal of Managing Value and Supply Chains, 4(3), 10–23. https://doi.org/10.5121/ijmvsc.2013.4302
- Das, D., Bhattacharya, S., & Sarkar, B. (2016). Decision-based design-driven material selection: A normative-prescriptive approach for simultaneous selection of material and geometric variables in gear design. Materials & Design, 92(2016), 787–793. https://doi.org/10.1016/j.matdes.2015.12.064
- Forsthoffer, W. (2011). Forsthoffer’s best practice handbook for rotating machinery. Butterworth-Hienemann. https://doi.org/10.1016/B978-0-08-096676-2.10004-9
- Fu, G.-Z., Li, Y.-F., Tao, Y., & Huang, H. Z. (2018). An interactive preference-based evolutionary algorithm for multi-criteria satisficing optimization. Journal of Intelligent & Fuzzy Systems, 34(4), 2503–2511. https://doi.org/10.3233/JIFS-17344
- Gearboxes for incline conveyors. Retrieved June 14, 2020, from https://www.stmuk.co.uk/blog/gearboxes-incline-conveyors .
- Han, J., Shin, J., Kim, J., & Oh, S. (2019). Design 2-speed transmission for a compact electric vehicle using dual brake system. Applied science, 9(9), 1973. https://doi.org/10.3390/app9091793
- Hazelrigg, G. A. (2013). A framework for decision-based engineering design. Journal of Mechanical Design, 120(4), 653–658. https://doi.org/10.1115/1.2829328
- Hermann, M., Pentek, T., & Otto, B. (2016). Design principles for Industrie 4.0 scenarios. Procceedings of Annual Hawaii International Conference on System Sciences, (2016), 3928–3937. https://doi.org/10.1109/HICSS.2016.488
- Hofstetter, M., Lechleitner, D., Hirz, M., Gintzel, M., & Schmidhofer, A. (2018). Multi-objective gearbox design optimization for x{EV}-axle drives under consideration of package restrictions. Forsch Ingenieurwes, 82(4), 361-370.. https://doi.org/10.1007/s10010-018-0278-9
- Karayel, D. G., Ozkan, S. S., & Vatansever, F. (2013). An integrated knowledge-based system for machine design. Advances in Mechanical Engineering, 5(2013), 702590. https://doi.org/10.1155/2013/702590
- Kilincci, O., & Onal, S. A. (2011). Fuzzy AHP approach for supplier selection in a washing machine company. Expert Systems with Applications, 38(8), 9656–9664. https://doi.org/10.1016/j.eswa.2011.01.159
- Li, M., & Gao, F. (2003). Why Nonaka highlights tacit knowledge: A critical review. Journal of Knowledge Management, 7(4), 6–14. https://doi.org/10.1108/13673270310492903
- Li, X. J., Bin, G. F., & Dhillon, B. S. (2011). Model to evaluate the state of mechanical equipment based on health value. Mechanism and Machine Theory, 46(3), 305–311. https://doi.org/10.1016/j.mechmachtheory.2010.11.008
- Liu, S., & Boyle, I. M. (2009). Engineering design: Perspectives, challenges, and recent advances. Journal of Engineering Design, 20(1), 7–19. https://doi.org/10.1080/09544820802670914
- Lu, R., Feng, Y., Zheng, H., & Tan, J. (2016). A product design based on interaction design and axiomatic design theory. Procedia CIRP, 53(2016), 125–129. https://doi.org/10.1016/j.procir.2016.07.061
- Luthra, S., & Mangla, S. K. (2018). Evaluating challenges to Industry 4.0 initiatives for supply chain sustainability in emerging economies. Process Safety and Environmental Protection, 117(2018), 168–179. https://doi.org/10.1016/j.psep.2018.04.018
- Madu, C. N. (2006). House of quality (QFD) in a minute (second ed.). Chi Publishers.
- Mahiddini, B., Chettibi, T., Benfriha, K., & Aoussat, A. (2019). Multidisciplinary design optimization of a gear train transmission. Concurrent Engineering, 27(3), 268–281. https://doi.org/10.1177/1063293X19862605
- Malgorzata, S., & Patalas-Maliszewski, J. (2015). Model of converting tacit knowledge into explicit knowledge on the example of the R & D department of the manufacturing company, including evaluation of knowledge workers’ usefulness. Journal of Theoretical and Computational Science, 9(3), 25–34. http://www.jtacs.org
- Maputi, E. S., & Arora, R. (2019). Design optimization of a three-stage transmission using advanced optimization techniques. International Journal for Simulation and Multidisciplinary Design Optimization, 10(2019), 1-11, A8. https://doi.org/10.1051/smdo/2019009
- Marini, C. D., Fatchurrohman, N., Azhari, A., & Suraya, S. (2016). Product development using QFD, MCDM and the combination of these two methods. IOP Conference on Science and Innovated Engineering Paper, 114(2016), 1–9. https://doi.org/10.1088/1757-899X/114/1/012089
- Marjanovic, N., Isailovic, B., Marjanovic, V., Milojevic, Z., Blagojevic, M., & Bojic, M. (2012). A practical approach to the optimization of gear trains with spur gears. Mechanism and Machine Theory, 53(2012), 1–16. https://doi.org/10.1016/j.mechmachtheory.2012.02.004
- Marler, R. T., & Arora, J. S. (2004). Survey of multi-objective optimization methods for engineering. Structural and Multidisciplinary Optimization, 26(6), 369–395. https://doi.org/10.1007/s00158-003-0368-6
- Mcmahon, C., Lowe, A., & Culley, S. (2004). Knowledge management in engineering design: Personalization and codification. Journal of Engineering Design, 15(4), 307–325. https://doi.org/10.1080/09544820410001697154
- Mendi, F., Başkal, T., Boran, K., & Boran, F. E. (2010). Optimization of module, shaft diameter and rolling bearing for spur gear through genetic algorithm. Expert Systems with Applications, 37(12), 8058–8064. https://doi.org/10.1016/j.eswa.2010.05.082
- Miler, D., Lončar, A., Žeželj, D., & Domitran, Z. (2017). Influence of profile shift on the spur gear pair optimization. Mechanism and Machine Theory, 117(2017), 189–197. https://doi.org/10.1016/j.mechmachtheory.2017.07.001
- Miler, D., Žeželj, D., Lončar, A., & Vučković, K. (2018). Multi-objective spur gear pair optimization focused on volume and efficiency. Mechanism and Machine Theory, 125(2018), 185–195. https://doi.org/10.1016/j.mechmachtheory.2018.03.012
- Murphy, F., Stapleton, L., & Smith, D. (2004). Tacit knowledge and human-centred systems: The key to managing the social impact of technology. International Multitrack Conference of Advances in Control Systems, 1–7. Retrieved from http://eprints.wit.ie/1115/
- Nallusamy, S., Sri Lakshmana, D., Kumar, K., Balakannan, P. S., & Chakraborty. (2016). MCDM tools application for selection of suppliers in manufacturing industries using AHP, fuzzy logic and ANN. International Journal of Engineering Research in Africa, 19(2016), 130–137. https://doi.org/10.4028/www.scientific.net/JERA.19.130
- Nightingale, P. (2009). Tacit Knowledge and Engineering Design. In Philosophy of Technology and Engineering Sciences (Vol. 9, pp. 351–374). https://doi.org/10.1016/B978-0-444-51667-1.50017-3
- Ognjanovic, M. (1996). Decisions in gear train transmission design. Research in Engineering Design -Theory, Applications, and Concurrent Engineering, 8(1996), 178–187. https://doi.org/10.1007/BF01608352
- Pahl, G., Beitz, W., Feldhusen, J., & Grote., K. H. (2007). Engineering design: A systematic approach (Third ed.). Springer, Berlin Heidelberg.
- Puteh, F. (2018). Measuring tacit knowledge : A deliberate construct validation using structural equation modelling. J. Inf. Knowl. Manag., 17(3), 1–18. https://doi.org/10.1142/S0219649218500259
- Radzevich, S. P. (2012). Dudley’s handbook of practical gear design and manufacture (Second ed.). CRC Press, Taylor and Francis Group. https://doi.org/10.1201/b11842
- Rall, J. H., & Staples, P. (2011) Belt conveyor drives – A consideration of some design aspects. Retrieved June 14, 2020, from http://www.saimh.co.za/beltcon/beltcon1/paper16.html .
- Renzi, C., Leali, F., & Di Angelo, L. (2017). A review on decision-making methods in engineering design for the automotive industry. Journal of Engineering Design, 28(2), 118–143. https://doi.org/10.1080/09544828.2016.1274720
- Salter, A., & Gann, D. (2003). Sources of ideas for innovation in engineering design. Research Policy, 32(8), 1309–1324. https://doi.org/10.1016/S0048-7333(02)00119-1
- Stefanović-Marinović, J., Troha, S., & Milovančević, M. (2017). An Application of multicriteria optimization to the two-carrier two-speed planetary gear trains. Facta Universitatis, series: Mechanical Engineering, 15(1), 85. https://doi.org/10.22190/FUME160307002S
- Sung, R., Ritchie, J. M., Rea, H. J., & Corney, J. (2011). Automated design knowledge capture and representation in single-user CAD environments. Journal of Engineering Design, 22(7), 1–17. https://doi.org/10.1080/09544820903527187
- Velasquez, M., & Hester, P. T. (2015). An analysis of multi-criteria decision-making methods. International Journal of Operations Research, 10(2), 56–66. https://doi.org/10.3917/spi.066.0056
- Wahid, C., Zidat, S., & Marir, F. (2018). An approach to the acquisition of tacit knowledge based on an ontological model. Journal of King Saud University-Computer and Information Sciences. https://doi.org/10.1016/j.jksuci.2018.09.012
- Wan, J., & Krishnamurty, S. (2015). Learning-based preference modeling in engineering design. Journal of Mechanical Design, 123(2), 191–198. https://doi.org/10.1115/1.1361061
- Yan, H. B., & Ma, T. (2014). A group decision-making approach to uncertain quality function deployment based on fuzzy preference relation and fuzzy majority. European Journal of Operational Research, 241(3), 1–15. https://doi.org/10.1016/j.ejor.2014.09.017
- Zadeh, L.A (1996). “Fuzzy sets, fuzzy logic, and fuzzy systems: Selected papers of Lofti”. In Zadeh, A., Klir, G. J., & Yuan, B (Eds.), Advances in Fuzzy Systems River Edge:NJ World Scientific. Applications and Theory, 6. https://doi.org/10.1142/2895
Appendix 1. Gear Concept Selection Matrix