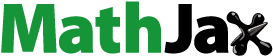
Abstract
The need for improved performance, operational efficiency as well as cost and environmental sustainability necessitate the selection of appropriate materials and assembly methods during railcar manufacturing and maintenance. The selection of the most appropriate materials and assembly methods amidst different materials is a Multi-Criteria Decision (MCD) which requires a scientific decision support framework. In this study, a model based on the Analytical Hierarchy Process (AHP) was used for the selection of the most suitable material and method of assembly for the development and maintenance of the body shell of a railcar. The performance of stainless steel, aluminum and carbon steel as well as welding methods such as Laser Arc Welding (LAW), Friction Stir Welding (FSW) and Metal Inert Gas (MIG) and the Resistance Spot Welding (RSW) currently used in railcar development were examined. The strength to weight ratio, crash worthiness, mechanical properties, degree of formability as well as cost effectiveness, end of life, and functional requirements were the key decision variables that form the criteria, which were broken down into a number of sub-criteria. The criteria and sub-criteria were pairwise compared to determine their priorities to the design and service requirements (goal). The AHP gave a detailed analysis of the materials as well as the methods of assembly that meet the service requirements of the railcar in a hierarchical order. Hence, this work provides the application of decision support framework for meeting the increasing design and service requirements in the railcar manufacturing industries.
PUBLIC INTEREST STATEMENT
This work was conceived in an effort to develop a sustainable and innovative approach geared towards the development and maintenance of a railcar. The need for improved performance, operational efficiency as well as cost and environmental sustainability necessitate the selection of the appropriate materials and assembly methods during the railcar manufacturing and maintenance. The selection of the materials and assembly methods amidst different materials are Multi-Criteria Decision (MCD) problems which require a scientific decision support framework. Over the years, there have been over reliance on the manufacturers’ experience in the selection of materials and welding methods without any scientific justification. The practice of relying on the manufacturer's experience is fast becoming unreliable amidst the emerging materials and dynamic production factors. It is in view of this, that a model based on the Analytical Hierarchy Process (AHP) was developed for the selection of suitable material and method of assembly during the development a railcar.
1. Introduction
The materials used for the manufacturing and maintenance of the rolling stock as well as the assembly methods are important factors which need a decision supports framework for its selection. The requirements to be satisfied by the material may include: design, assembly, functional, energy, environmental, service, and end of life requirements. In addition to these requirements, the use of some emerging materials will take into consideration the maintainability, crash worthiness and fire retardant ability amongst others. This is because the failure of structural members due to wrong selection of materials pose a great risk to safety and can have catastrophic consequences on the supplier, manufacturer and operator. On the part of the supplier and operator, there may be loss of integrity and good will with an increase in the cost of compensations while the manufacturers may incur the cost of rectifying the structural defects. While steel is mostly preferred for the roof and the underframe due to its high strength, aluminium finds application in the body shell development due to its low weight to strength ratio (The Railway Technical Website, Citation2019). Researches have however proven that the performance of steel and aluminium alloys as well as other polymer-based composites currently used in railcar in terms of strength and flame retardation are less than satisfactory (Kotsikos, Citation2016; Toldi et al., Citation2017; Xiaosong, Citation2009), hence, the quest for the development and selection of materials that will provide satisfactory performance in service. However, the performance of some materials are sometimes influenced by the methods of assembly employed. Furthermore, the End of Life (EoL) options applicable to some materials can also be a function of the assembly method. For instance, both steel and aluminium can be recycled and reused after its end of life while retaining their properties but the nature of alloy used with aluminium, method of assembly as well as the intricate shapes formed often make the recycling of aluminium a challenge. Some recent advances in the material development for railcar applications have brought about the development of carbon-reinforced polymers because of the following characteristics: high strength to weight ratio, good rigidity, resistance to corrosion and fatigue, good tensile strength, low coefficient of thermal expansion, high stiffness, and strength, low density, non-poisonous nature, excellent shielding against electromagnetic interference, biological inertness and permeability to X-rays etc. (Campus & Roure, Citation2005; Perret et al., Citation2011; Quintiere et al., Citation2007; Stoliarov et al., Citation2007). The challenge, however, lies in the low rate of recovery of polymer-based composite materials in violation of the concept of circular economy and principles of environmental protection. Therefore, besides the structural integrity of materials employed for the manufacture of rolling stock, there is a need to also consider the environmental requirements. Going by the quest for a cleaner environment through significant reduction in emissions by transport vehicles, regulatory agencies are coming up with tough measures to reduce environmental pollutions through emissions and waste generation at the EoL of materials used for manufacturing (Marques et al., Citation2012; United Nations Economic Commission for Europe [UNECE], Citation2016). Hence, there is need for stakeholders in the development and maintenance of rolling stock to embrace recent innovations and adjust their manufacturing models to reduce emission and waste generations in order to gain a competitive advantage and emerge as industry leaders. The use of emerging cost-effective composites and light metallic alloys, which reduce the energy consumption of the system with attendant reductions in the emissions has occupied the font burners in recent times but the limitations lie in the fact most of the composites are non-recyclable materials, hence, considerable amount of materials and profit are lost by the end of their life. In addition, the wastes generated will have considerable environmental impact due to improper disposal. For instance, research has proven that the wastes generated from the end of life of a single railcar cargo is equivalent to the ones generated by dismantling 20 automobile vehicles (Silva & Kaewunruen, Citation2017). If means are not sought promote a circular economy via the reuse, recycling or recovering of the generated wastes, it will increase the environmental and production concerns relating to greenhouse emissions, energy efficiency challenges, high cost of raw materials, increased cost of production, and disposal problems amongst others.
Also, during the development or maintenance of the railcar body shell and underframe, the weight of materials to be employed is a critical requirement in order to ensure that the railcar runs at a higher speed on curved track sections. Significant reduction in weight of a railcar without sacrificing the structural integrity will also reduce stresses on the track and the curved rail profiles with reduction in the energy requirement for the operation, with increased cost and environmental sustainability. In order to improve the performance of the railcar in terms of high energy absorption due to impact, acoustic damping, better stability, structure toughness, high bi axial compression load-bearing ability, improved fatigue life, improved speed and manoeuvring abilities, high load carrying ability, improved energy efficiency and environmental sustainability, high rigidity and strength to low weight ratio, there is a need to develop an analytical approach for the material and assembly selection processes.
Table shows the various materials often employed for the various component parts of the railcar.
Table 1. Components parts and the material usually employed
The selection of the right materials and welding method for the railcar assembly can help address the aforementioned challenges is a critical task, which represents a Multi-Criteria Decision (MCD) based on the different characteristics of different type materials and welding methods as well as their conflicting features and selection criteria. Over the years, there have been over reliance on the manufacturers’ experience in the selection of appropriate materials and welding methods without any scientific basis or justification based on the pairwise comparison between the selected solution and its alternatives. The practice of relying on the manufacturers’ experience is fast becoming unreliable amidst emerging materials, dynamic production factors and the quest for increased productivity, efficiency and reliability during the development of rolling stocks.
The combination of welding process parameters as well as the characteristics of the different welding techniques often influences the structural integrity of the welded components as well as the overall welding time and cost. Commonly used welding methods during the railcar development, maintenance or assembly operations include; Laser Arc Welding (LAW), Resistance Spot Welding (RSW), Metal Inert Gas (MIG) and Friction Stir Welding (FSW) amongst others. The aforementioned welding methods have their merits and demerits, which makes each of them suitable for some specific area of application during the assembly operation and maintenance of the railcar. The use of the knowledge-based decision support system such as the Analytical Hierarchy Process (AHP) will be suitable for the evaluation of the characteristics of each welding method with a view of determining the most appropriate material and assembly technique, which will strike the right balance among the competing criteria. For instance, the Laser Arc Welding (LAW) method, combines the merits of the laser method with the gas metal arc using low energy input to bring about welding operation that penetrates deeply between the materials, hence, it is a fast, cavity-free welding method that produces welded structure of high integrity and durability. It is also suitable for joining several materials with different thicknesses and complex geometries. The limitations being the cost of installation and consumables and the fact that the welding operation often requires expensive and time-consuming post-welding operations (Satish & Khod, Citation2015; Ugur & Baykan, Citation2017). The Friction Stir Welding (FSW) is considered a suitable method for joining lightweight materials particularly for structures with low weight and high strength requirements. The process is cost-effective without the need for expensive and time-consuming post-welding operations producing welded structures with good appearance and mechanical properties. The limitations however lie in the fact that it is slow, with the tendency to create cavity-welds and it is often difficult to use for non-linear welds and in joining materials of varying thicknesses (Chauhan & Pradhan, Citation2014; Das & Chakraborty, Citation2011). On the other hand, the Metal Inert Gas (MIG) welding is a versatile, fast, clean and efficient welding method but limited to thin materials and indoor operations because it is cumbersome coupled with the fact that it requires a shielding gas to protect the purity of the weld (Jayant, Citation2011; Sadhu & Chakraborty, Citation2011). The Resistance Spot Welding (RSW) is a safe, fast, efficient and cost-effective technique which can be used to join different materials of varying thicknesses but if the process is not adequately controlled it can bring about the production of welded structure with poor strength and integrity (Capraz et al., Citation2014; Jayant, Citation2012).
The main objective of selecting the most suitable material and assembly method, however, is to produce quality and reliable structural welds or joints, in a time and cost-effective manner, which will satisfy the service requirements during the operation of a railcar (Daniyan et al., Citation2019a; Citation2019b; Daniyan et al., Citation2020, Citation2020). It is in view of this, that a model based on AHP was developed for the selection of the most suitable material and method of assembly during the development or maintenance of railcar.
The Multi-Criteria Decision (MCD) problems are problems with a finite number of alternatives and conflicting criteria (Musingwini, Citation2010), hence, the MCD techniques can be used to make strategic decisions in such scenarios (Petit & Fraser, Citation2013). Musingwini (Citation2010) and Mahase et al. (Citation2016) highlighted the general procedural steps in solving a MCD problem as follows: identification of objectives and the criteria, assignment of weights in the form of numerical values to the identified criteria, ranking of the alternatives and decision-making based on the outcome. Some widely used MCD techniques include; Simple Multi-Attribute Rating Technique, Multi-Attribute Utility Theory (MAUT), case-based reasoning, goal programming, AHP, Technique for Order of Preference by Similarity to Ideal Solution (TOPSIS), VIKOR, ELECTRE, PROMETHEE, etc. (Odu, Citation2019; Velasquez & Hester, Citation2013).
One of the peculiarities of the AHP is the fact that it can be used to establish a correlating relationship among the performance goal, criteria and alternatives which will enhance the ranking of alternatives and decision-making (Abdelhamid et al., Citation2011; Felice & Petrillo, Citation2010; Sharma et al., Citation2015; Subramanian & Ramanathan, Citation2012). Furthermore, the established relationship can be developed into a predictive model for keeping the dynamic goals, the proposed solution and its alternatives as well as the multi or sub criteria within a realistic forecast.
The TOPSIS can be used to increase the confidence level of the solutions obtained from the AHP in that it can estimate the closeness of the most feasible alternative to the positive-ideal solution and the distance from the negative ideal solution (Odu, Citation2019; Velasquez & Hester, Citation2013). TOPIS is easy to use and program and the number of steps remains the same regardless of the number of attributes, thus, simplifying the decision-making process though it does not take into account the correlation of the attributes of the competing criteria or factors. With the use of TOPSIS, the best alternative will be close to the positive ideal solution and farther from the negative ideal solution (Odu, Citation2019; Velasquez & Hester, Citation2013). Both the AHP and TOPSIS are suitable for estimating the weights of the criteria and sub criteria, and in the ranking of the alternatives (Behzadian et al., Citation2012; Capraz et al., Citation2015; Mirhedayatian et al., Citation2013). It has been reported that the combination of the AHP and TOPSIS offers a knowledge-based multi-criteria decision support systems for determining the optimum manufacturing method amidst complex and conflicting multiple manufacturing methods, emerging technologies and materials (Jayant & Singh, Citation2015; Mostafa & Vahdat, Citation2012). The Fuzzy concept has also been introduced into the AHP or TOPSIS structure (Fuzzy-AHP or Fuzzy-TOPSIS) to provide some modifications aimed at increasing the robustness of the MCD technique. The significance of integrating a Fuzzy concept into AHP or TOPSIS is to address the complexity of the ranking problem, by accounting for uncertainties or imprecise data that could affect the consistency or robustness of the MCD approach. However, the fuzzy concept may require numerous simulations for effective performance (Velasquez & Hester, Citation2013). The case-based reasoning is not data-intensive but may require many cases while the goal programming can produce infinite alternatives for large-scale problems but requires the use of other MCD techniques for weighing coefficients. The VIKOR approach can be deployed to solve decision problems with conflicting and non-commensurable criteria, assuming the compromise is acceptable for conflict resolution (Arvind & Janpriy, Citation2018). The VIKOR approach ranks and selects the most feasible alternative from a set of alternatives by determining the compromise solution closest to the ideal solution (Odu, Citation2019). The MAUT, ELECTRE and PROMETHEE take into account the uncertainty, vagueness and imprecision that characterize some other MCD techniques but the MAUT may require a lot of input variables, while the process of the ELECTRE may become complex and the process of weight allocation in the PROMETHEE can also be a challenge (Velasquez & Hester, Citation2013). However, the integration of some these MCD approaches for solving MCD in order to complement the weaknesses in one another and improve the overall robustness of the solution have been reported (Odu, Citation2019; Velasquez & Hester, Citation2013).
Handfield et al. (Citation2002) applied the AHP for the assessment of the suppliers using the environmental criteria while Gnanasekaran et al. (Citation2010) developed an integrated fuzzy-AHP model for the selection of suppliers in a steel plant. In addition, Jayant and Singh (Citation2015) employed the AHP for the selection of the most feasible welding process in high-pressure vessel manufacturing environment while Ugur and Baykan (Citation2017) proposed a model for making decision that relates to the selection of wall material using the AHP. The overview of some existing literature employed for solving some MCD problems is presented in Table . The research findings from these literature demonstrated the feasibility of the AHP and other MCD techniques as well the combination of the MCD techniques in resolving MCD problems. The main contribution of this study is mostly on the practical and industrial aspects. The selection of the most appropriate material and welding method for the railcar assembly amidst existing and emerging materials and welding methods are multi-criteria decision, which has not been sufficiently reported by the existing literature. Hence, with the aid of the AHP, this work provides the application of decision support framework in the quest to meet the increasing design and service requirements in the railcar industries.
Table 2. Overview of MCD problems and techniques employed
2. Methodology
The AHP was considered suitable for the selection of the most suitable material and the method of assembly during the development or maintenance of the body shell of a railcar. This is because as compared to other MCD approaches, AHP can interactively combine qualitative and quantitative factors to simplify multi-criteria problems and make consistent judgement via pairwise comparison. AHP is scalable with a hierarchical structure which makes it easy to adjust to accommodate MCD problems (Velasquez & Hester, Citation2013). It is also suitable for the allocation of weights and ranking of the priorities among the competing criteria and factors (Kardi, Citation2006; Saaty, Citation2008). The use of pairwise comparisons can permit decision makers to weigh coefficients and compare alternatives with relative ease. However, the judgment regarding the allocation of weights to competing criteria or factors are usually done subjectively thus calling to question the robustness and consistency of the analytical process. In order to ensure the robustness and consistency of the process, this study ensures the computation of the consistency ratios for each pairwise comparison matrix as well as the overall consistency ratio and the value was compared with the benchmark ( as given by Saaty (Citation2008). In addition, the sensitivity analysis was carried out to determine the degree of changes of the process to random changes in the pairwise weights allocated to the competing criteria and factors. This provides an insight into the stability, consistency and robustness of the process in relation to the pairwise comparison judgments.
The procedural steps for the application of the AHP methodology are as follows:
The identification of the MCD problem involving an overlapping and conflicting criteria as well as the identification of the overall goal. For the purpose of this study, the MCD is the selection of the appropriate materials and assembly method for the development of the body shell of a railcar which will promote the concept of circular economy and enhance structural integrity, aesthetics, ease of assembly, good finish in addition to sustainability in terms cost, time and End of Life (goals).
The identification of the alternatives (competing factors). In this case, the materials commonly used for assembly operations are aluminium, carbon and stainless steel while the common method of assembly includes the friction stir welding, metal inert gas welding, laser arc welding as well as the resistance spot welding.
Allocation of weights to the alternatives as well as pairwise comparison among the set of criteria. The comparison is made between the paired factors according to their relative preference in the rank of 1–9 based on the Saaty’s scale (Saaty, Citation2008). This is presented in Table .
Computation of the priority vector and the overall weights of each criterion and the alternatives with the ranking done in the order of hierarchy.
The computation of the consistency index and ratio. This is to determine the level of consistency in the responses obtained as well as in the pairwise comparison earlier made. Once the consistency ratio is less than 10%, the level of consistency is said to be high (Kardi, Citation2006; Saaty, Citation2008).
Table 3. The pairwise comparison scale for the AHP preferences
The following assumptions underlie the use of AHP:
When two factors are pairwise compared, the value of the preference should satisfy the reciprocal of the weights allocated to the paired factors.
The weights of the factors at the lower levels of the matrix is a function of the weights of the factors at the upper levels (Song & Kang, Citation2016).
2.1. Material selection
The selection of aluminium, carbon steel and stainless steel were based on the mechanical properties of the materials and their suitability for the development of a railcar. The design requirements specify properties such as high strength to weight ratio, durability, hardness, cost, structural integrity and end of life requirements, as the failure of a structural component pose significant consequences for the operator and the manufacturer. This is because the recalling of already delivered railcar through reverse logistics in order to rectify structural defects pose some cost implications. In addition, in order to promote the concept of circular economy with emphasis on zero tolerance for waste generation during product’s life cycle as well as sustainability in terms of materials, energy and environment, there is a need to consider the use of materials that will be easy to reclaim into service at the end of their lives (Wu et al., Citation2014). Hence, the nature of material and welding methods considered as well as the selection criteria were established from the literature (Campus & Roure, Citation2005; Merkisz-Guranowska et al., Citation2014; Silva & Kaewunruen, Citation2017; The Railway Technical Website, Citation2019).
The framework for the development of the MCD model for the selection of the material and the assembly method is presented in Figure .
The challenges of deciding the most suitable material and assembly process for the development of the railcar body represent a multi-criteria problem and the framework for such decision is shown in Figure . The criteria are represented by for the selection of the materials and welding method. For the material selection part of this work, Figure simplifies the framework (Figure ) into two levels AHP. This leads to the generation of matrix and the pairwise comparisons among the criteria and suitable materials (alternatives) based on criteria and sub criteria. The computation of the values of priority vectors, Consistency Index (CI), and Consistency Ratio (CR) assist in the ranking of the alternatives as well as the determination of the level of consistency among the criteria and the alternatives. This was followed by the computation of the average and overall weights, which form the basis for the selection of the most appropriate material and welding method.
From Figure , the goal, criteria A-D and the alternatives are represented by levels 0–2, respectively. While the goal is represented by level 0, the criteria are represented by level 1 and the different materials suitable for the development of the railcar body considered in this study (aluminium alloy, stainless steel alloy (austenite) and carbon steel) are the alternatives represented by level 2. Level 1 has a comparison matrix, having 4 factors and 6 comparisons while level 2 is a
matrix which is made up of three choices (3 comparisons).
The four main criteria in the order of priority considered for the material selection process are as follows:
Strength to weight ratio denoted as criterion A
Structural integrity denoted by criterion B
Formability as denoted by criterion C
Corrosion resistance and end of life as denoted by criterion D
Other sub criteria include: cost, surface finish and aesthetics, assembly cycle time and operation cost, energy and consumable requirements amongst others.
2.2. Method of assembly
The welding operation is one of the methods for the assembly of the pre-assembled parts, which makes up the body shell of the railcar in the manufacturer’s shop. The materials for the assembly of the parts as well as the design and finish requirements of the final assembly determine the type of welding method to be employed. The pre-assembled parts of the railcar include the underframe, body shell, under side, end and roof amongst others. This study however is limited to the railcar body shell. Notable among materials used are stainless steel, aluminum, and carbon steel. Irrespective of the material used for the fabrication, the quest for a cost-effective assembly process that will meet the design, finish and service requirements requires a scientific basis for selection rather than the welder’s experience alone. The criteria for selecting the most suitable welding method include: the ability to meet the robust design and service requirements (safety, structural strength and integrity as well as crashworthiness), surface finish and aesthetics, the time and cost effectiveness of the assembly process, end of life requirements (dismantling, recycling, reuse to maximize the value of scrap). The end of the life requirement is also linked to the quest for a circular economy with zero tolerance for waste generation during the product’s life cycle. The selection of a robust assembly technique for the component parts that would enhance ease of dismantling at their end of lives without sacrificing the strength of the assembled joint is necessary. This will promote significant energy, material, cost and time savings with improved environmental friendliness during the product’s life cycle. For the selection of the appropriate welding method, some commonly used welding methods during the railcar development, maintenance or assembly operations with the potential to meet the aforementioned criteria were considered based on the findings from the literature. These include: Laser Arc Welding (LAW), Resistance Spot Welding (RSW), Metal Inert Gas (MIG) and Friction Stir Welding (FSW) (Capraz et al., Citation2014; Jayant, Citation2011; Satish & Khod, Citation2015; Sharma et al., Citation2015; Ugur & Baykan, Citation2017). Figure simplifies the framework (Figure ) into two levels of the AHP, which indicates the relationship among the goal, criteria and alternatives.
From Figure , the goal represents level 0 while criteria A-D represent level 1. The different welding methods (LAW, FSW/MIG/RSW) which are the alternatives represent level 2. Level 1 has a comparison matrix having 4 factors with 6 comparisons while level 2 is a
comparison matrices consisting of 3 choices (3 comparisons).
The sub-criteria are hidden under the criteria in order to simplify the structure of the hierarchy. The four main criteria for the welding methods considered are as follows:
Crash worthiness and structural integrity denoted as criterion A
Surface finish and aesthetics denoted as criterion B
Welding cycle time and operation cost denoted as criterion C; and
End of Life (EoL) requirements denoted as criterion D.
The Laser Arc Welding (LAW) method is denoted as welding method 1 while the combination of the Friction Stir Welding (FSW) method and the Metal Inert Gas (MIG) is denoted as welding method 2. The Resistance Spot Welding (RSW) is denoted as welding method 3.
The expression for the determination of the eigen values and priority vectors is given by EquationEquation 1(1)
(1) .
Where: A is the m-by-n matrices, which represents the products of the pairwise comparison of the criteria and factors, is a column vector having length n, and
is the scalar quantity. The values of λ which satisfy the equation are the eigenvalues while the values of
are the right eigenvectors. The manual computation of the priority vectors may be vague and prone to error, hence, the computations of the priority vectors were done in the MATLAB 2018 b environment.
EquationEquations 2(2)
(2) and Equation3
(3)
(3) express the Consistency Index (CI) and the Consistency Ratio (CR) respectively.
Where:
is the largest Eigen value,
is the order of the matrix and
is the Random Consistency Index. The Random Index (
is the ratio of the consistency index to the consistency ratio and is a function of the order of the matrix
. The random index (RI) for a
and
matrices (applicable to this study) are obtained as 0.58 and 0.90, respectively, as given by Saaty (Citation2008) and Kardi (Citation2006).
The average Consistency Ratio ( which is the ratio of the sum of the weighted consistency index (
and Random Consistency Index (
for all the levels is expressed as EquationEquation 4
(4)
(4) . The equation is necessary in the determination of the overall consistency of the analytical process.
Where: is the computed weight (priority vectors) of criteria or the competing factors.
2.3. Sensitivity analysis
The univariate (one-at-a time) local sensitivity analysis method involving only one input change per model evaluation was employed in this study. This was to understudy the percentage change in the output with changes in the input variables. One characteristic of the one-way sensitivity analysis is its simplicity and its suitability for exploring small design space as applicable in this study (Qian & Mahdi, Citation2020). The weight of one of the criteria of the MCD model was changed at only one time and the results obtained for the entire matrix were compared with the values of the initial output in order to determine the percentage change. The essence of the sensitivity analysis is to reduce the chances of the model failing due to numerical error or improper allocation of weights which is subjective in nature. The analysis will also reveal some critical factors which may be responsible for variations in the final output, and as such, such factors can be tracked within the design space to ensure high degree of precision and accuracy in the MCD analysis. The percentage change is the percent ratio of the difference between the initial and the final outputs to the initial outputs. To perform the sensitivity analysis, the model parameter for evaluation (in this case the weights of the paired criteria for the material selection and welding methods (AB, AC, AD, BC BD and CD) were changed (changes taken to be of the initial weight allocated per model evaluation at a time). This serves as the representatives of the set of values used to explore the design space. Holding other factors constant, the new priority vectors were computed for the material selection and welding methods and the corresponding percentage change in the outputs were also computed.
3. Results and discussion
3.1. Materials selection
Table shows the paired comparison of the criteria for the material selection. The competing criteria are paired based on their relative importance to the overall goal and the pairing produces six comparisons namely: AB, AC, AD, BC, BD and CD. The more important pair takes higher value of integer and vice versa. The pairwise comparison was done using Table for preferences (Equal, moderate, strong, very strong and extreme preferences on a scale of 1–9).
Table 4. The paired comparison of the criteria with respect to the goal for material selection
The maximum eigen value is calculated thus;
The ,
. Since
is less than 10%, the level of consistency is said to be high (Kardi, Citation2006; Saaty, Citation2008).
Table presents the pairwise comparison for the material selection process in respect of criterion A.
Table 5. The paired comparison with respect to the material selection (criterion A)
The maximum eigen value is calculated thus;
The
Since
is less than 10%, the level of consistency is said to be high (Kardi, Citation2006; Saaty, Citation2008).
Table presents the pairwise comparison for the material selection process in respect of criterion B.
Table 6. The paired comparison with respect to the material selection (criterion B)
The maximum eigen value is calculated thus;
The ,
. Since
is less than 10%, the level of consistency is said to be high (Kardi, Citation2006; Saaty, Citation2008).
Similarly, Tables and , present the results obtained for the paired comparison for the material selection process with respect to criteria C and D are as follows;
Table 7. The paired comparison with respect to the selection of the material (Criterion C)
Table 8. The paired comparison with respect to the selection of the materials (criterion D)
For factor C, the maximum eigen value is calculated thus;
The ,
. Since
is less than 10%, the level of consistency is said to be high (Kardi, Citation2006; Saaty, Citation2008).
The maximum eigen value is calculated thus;
The ,
. Since
is less than 10%, the level of consistency is said to be high (Kardi, Citation2006; Saaty, Citation2008).
The computation of the overall weights for the material selection process are as follows;
From EquationEquation 3(3)
(3) , the overall consistency ratio
is calculated thus;
Since the overall is less than 10%, the level of consistency during the analytical process is said to be high (Kardi, Citation2006; Saaty, Citation2008).
Table shows the average and overall weights computed for the material selection process. The average weight is the mean weight for the four comparison matrices considered.
Table 9. The average overall weights for the material selection
From Figure , aluminium and its alloy is ranked first and considered the most suitable material for the fabrication of the railcar body shell followed by stainless steel alloy. Carbon steel and its alloy is ranked least in terms of the average and overall weights. The suitability of the aluminum alloy can be linked to its properties of high strength to weigh ratio, good strength which will ensure structural integrity and good formability in a bid to achieve energy, cost and environmental sustainability during the product’s life cycle thus meeting criteria A, B and C. Aluminum material is also corrosion resistant and boast of high recovery rate (ranging between 80 and 95%) at the product’s end of life (Silva & Kaewunruen, Citation2017) thereby meeting criterion D. Stainless steel on the other hand boast of excellent strength, good formability and good corrosion resistance ability thus meeting criterion B but the weight of stainless steel material is major constraint in a bid to achieve cost, energy and environmental sustainability during the product’s life cycle thus failing to meet criterion A. Stainless steel also boast of good formability thereby meeting criterion B and it is highly recoverable (with recovery rate ranging between 90 and 98%) thus meeting criteria D. The non-suitability of the carbon steel for the fabrication of the railcar body shell is connected with its characteristics of producing low strength to high weight ratio when compared to aluminum and stainless steel thus failing to meet the criterion of crash worthiness and structural integrity denoted as criteria A and B which were highly prioritized. Although, it has high rate of material recovery at the products’ end of life thus partially meeting criteria D, however, its corrosion resistance ability and ease of formability are also lower compared to that aluminium and stainless steel alloys. Figure shows the Pareto analysis of the materials in the order of their ranking based on the overall weight of the materials considered.
3.2. Selection of the assembly process
Table shows the paired comparison of the criteria with respect to the goal for the method of assembly. The competing criteria are paired based on their relative importance to the overall goal and the pairing produces six comparisons namely: AB, AC, AD, BC, BD and CD. The more important pair takes higher value of integer and vice versa.
Table 10. The paired comparison of the criteria for the welding methods
The maximum eigen value is calculated thus;
,
Since
is less than 10%, the level of consistency is said to be high (Kardi, Citation2006; Saaty, Citation2008).
Table presents the pairwise comparison of the alternatives (assembly methods).
Table 11. The paired comparison matrix 2 with respect to the welding methods (criterion A)
The maximum Eigen value is calculated thus;
,
. Since
is less than 10%, the level of consistency is said to be high (Kardi, Citation2006; Saaty, Citation2008).
Table presents the pairwise comparison for the welding methods considered in respect of criterion B.
Table 12. The paired comparison with respect to the welding methods (criterion B)
The maximum Eigen value is calculated thus;
,
Since
is less than 10%, the level of consistency is said to be high (Kardi, Citation2006; Saaty, Citation2008).
Similarly, the results obtained for the paired comparison for the selection of the welding method with respect to criteria C and D, respectively, are presented in Tables and .
Table 13. The paired comparison with respect to the welding methods (criterion C)
Table 14. The paired comparison with respect to the welding methods (criterion D)
For factor C, the maximum Eigen value is calculated thus;
,
. Since
is less than 10%, the level of consistency is said to be high (Kardi, Citation2006; Saaty, Citation2008).
The maximum Eigen value is calculated thus;
The ,
. Since
is less than 10%, the level of consistency is said to be high (Kardi, Citation2006; Saaty, Citation2008).
The computation of the overall weights for the selection of the appropriate welding method are as follow;
From EquationEquation 3(3)
(3) , the overall consistency ratio
is calculated thus;
Since the overall is less than 10%, the level of consistency is said to be high (Kardi, Citation2006; Saaty, Citation2008).
Table shows the average and overall weights for the welding methods considered.
Table 15. The average and overall weights for the welding methods
From Figure , the welding method 2, which is the combination of the Friction Stir Welding (FSW) and Metal Inert Gas (MIG) is considered most suitable process for the assembly operation of the railcar body with the highest magnitude of the overall weight followed by the welding method 1; the Laser Arc Welding (LAW) while the Resistance Spot Welding (RSW) (welding method 3) has the lowest values of the average and overall weights.
Crash worthiness and structural integrity denoted as criterion A
Surface finish and aesthetics denoted as criterion B
Welding cycle time and operation cost denoted as criterion C; and
End of Life (EoL) requirements denoted as criterion D.
The Laser Arc Welding (LAW) is considered a suitable welding method for welding the materials considered (aluminium, stainless steel and carbon steel alloys). It is also considered a fast welding method for producing cavity-free-welded structure of high integrity and durability thereby meeting criteria A and C. However, the process often requires expensive and time-consuming post-welding operations (Satish & Khod, Citation2015; Ugur & Baykan, Citation2017), thus, failing to meet criterion B. The recovery rate of materials welded by LAW however is a function of the welding conditions, joint design and configurations, laser source, materials to be joined amongst others.
The use of aluminium for the body side panel boast of high strength to weight ratio with significant energy conservation but requires the process of Metal Inert Gas (MIG) and Friction Stir Welding (FSW) for the production of joint with high structural integrity. The FSW of the aluminium is suitable for the pre-fabrication of the longitudinal side of the railcar body because the welding method operates below the melting point of aluminium, hence, it can produce welded parts with excellent mechanical properties and permissible distortion. The Friction Stir Welding (FSW) meets the criterion of crash worthiness and structural integrity (criterion A), with its suitability for joining lightweight materials particularly for structures with low weight and high strength requirements. It also meets the criterion of aesthetics and good surface finish denoted as criterion B, producing welded structures with good appearance and mechanical properties without the need for expensive and time-consuming post-welding operations. Characteristically, the process is slow compared to other assembly methods, thus, failing to meet the criterion of fast welding cycle time denoted as criterion C but with high affinity for material recovery at the products End of Life (EoL) thereby meeting criterion D., In addition, the process is also considered to be cost effective (Chauhan & Pradhan, Citation2014). On the other hand, the Metal Inert Gas (MIG) welding is a versatile, fast, clean and efficient welding method but limited to thin materials and indoor operations because it is cumbersome coupled with the fact that it requires a shielding gas to protect the purity of the weld (Jayant, Citation2011; Sadhu & Chakraborty, Citation2011). Hence, it can complement the FSW welding process in order to achieve cost, energy, material and environmental sustainability in addition to high structural strength and good finish during the welding process. RSW is prone to hot cracking, hence, the non-suitability of the RSW is connected with its characteristics of producing low strength thus failing to meet the crash worthiness and structural integrity denoted as criterion A which is highly prioritized though meeting criterion D. Furthermore, the process is considered safe, fast, efficient and cost effective (Capraz et al., Citation2014; Jayant, Citation2012), thereby meeting criterion C but may require some post-processing operations most especially under-uncontrolled conditions thus failing to meet criterion B. In addition, aluminium is often difficult to resistance weld to a high degree of strength compared to steel because of its high electrical conductivity which requires high welding current and electrode inputs thus reducing the overall process sustainability (Mathers, Citation2002). Figure shows the Pareto analysis of the welding methods in the order of their ranking based on their overall weight.
3.3. Sensitivity analysis
Tables – show the results obtained for the sensitivity analysis and the percent change in the outputs for both the material selection and the welding methods. After the sensitivity analysis, the consistency ratio of the final outputs of the model for the paired criteria for both the material selection and the welding methods were all found to be less than 10%. This agrees significantly with the consistency ratio of the initial output of the model (Tables and ). Furthermore, the rank of each of the criteria for the material selection and welding methods also remain the same for both the initial and final outputs (before and after the change) thus, indicating that the pairwise judgment is feasible and consistent. Also, the similarity in the pattern of the plots for the initial and final outputs of the model for the material selection and welding methods show that there is a high degree of agreement (consistency) between the initial and final outputs of the AHP models (Figures and ).
Table 16. Sensitive analysis for the criteria for material selection
Table 17. Percent change in output for the criteria for material selection
Table 18. Sensitive analysis for the criteria for welding methods
Table 19. Sensitive analysis for the criteria for welding methods
In addition, the percent change which is a measure of variation from the initial weight output of the criteria due to change in the input values was found to be within the permissible limit for both the material selection and welding methods (Tables and ).
On the overall, as evidenced by all the consistency ratios ( as well as the overall consistency ratio (
found to be less than 10% for all the matrices, the solution proposed for the selection of material and welding method was found to be consistent. This further lends credence to the study that the MCD analysis performed was very consistent. This implies that the solution is robust and the outputs of the AHP models are unlikely to be significantly affected by a change in one input per model.
Figure 8. The comparison of the initial and final model output for material selection (Criteria A-D are represented by 1–4 on the horizontal axis)
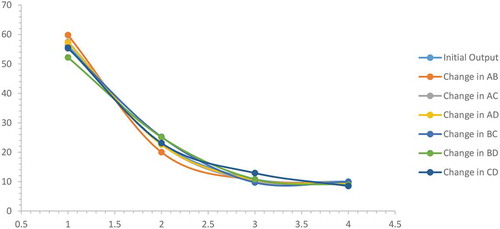
(Criteria A-D are represented by 1–4 on the horizontal axis).
4. Conclusion
This study presents a scientific-based methodology for evaluating and choosing the most suitable material and welding method for the railcar body development or maintenance. Based on the design, service and process requirements, the AHP was employed for structuring the multi-criteria decision problem into hierarchy and ranking of the criteria with respect to the goals and alternatives. Aluminium and its alloy as well as the combination of the Friction Stir Welding (FSW) and Metal Inert Gas (MIG) welding method were ranked first and considered most suitable material and the method of fabrication for the railcar body shell. This is because the material and the method present good characteristics such as the excellent mechanical properties and good surface finish etc. which are in line with the selection criteria. The work also provides a decision support framework for solving a Multi-Criteria Decision (MCD) problem and sorting out alternatives according to their relative importance to the overall goal. The findings of this work will serve as a scientific decision-making tool which will assist the railcar manufacturer in the development or maintenance of railcar parts that will meet the required customer, design, service and functional requirements. Future work can consider the integration of the AHP with FUZZY or TOPSIS for such decision making as well as the comparative analysis of the results obtained from the AHP with other MCD approaches such as VIKOR, ELECTRE, PROMETHEE, etc.
Additional information
Funding
Notes on contributors
Ilesanmi Daniyan
Ilesanmi Afolabi Daniyan is a Postdoctoral Research Fellow in the Department of Industrial Engineering, Tshwane University of Technology, Pretoria, South Africa. He played an active role toward the completion of this research. His research interests include: Production Engineering, Automation and Robotics as well as Renewable Energy.
References
- Abdelhamid, M., Shen, Q., Abdelhamid, M., Shan, D., Qattawi, A., & Omar, M. (2011). Using quality function deployment and analytical hierarchy process for material selection of body-in-white. Materials & Design, 32(5), 2771–24. https://doi.org/10.1016/j.matdes.2011.01.001
- Al-Subhi Al-Harbi, K. M. (2001). Application of the AHP in project management,”. International Journal of Project Management, 19(1), 19–27. https://doi.org/10.1016/S0263-7863(99)00038-1
- Arsovski, S., Todorovic, G., Lazić, Z., Arsovski, Z., Ljepava, N., & Aleksic, A. (2017). Model for Selection of the Best Location Based on Fuzzy AHP and Hurwitz Methods. Mathematical Problems in Engineering, 1–12. https://doi.org/10.1155/2017/2803461
- Arvind, J., & Janpriy, S. (2018). A Comprehensive literature review of MCDM techniques ELECTRE, PROMETHEE, VIKOR, and TOPSIS applications in business competitive environment. International Journal of Current Research, 10(2), 65461–65477. http://www.journalcra.com/sites/default/files/issue-pdf/28831.pdf
- Behzadian, M., Otaghsara, S. K., Yazdani, M., & Ignatius, J. (2012). A state-of the-art survey of TOPSIS applications. Expert Systems with Applications, 39(17), 13051–13069. https://doi.org/10.1016/j.eswa.2012.05.056
- Campus, B., & Roure, B. (2005). Use of composite materials in railway applications. Centre d’Ingénierie du Matériel (CIM).
- Capraz, O., Meran, C., & Gungor, A. (2014). Welding process selection for stainless steel milk storage tank using multi-criteria decision-making tools. Proceedings of 15th International Materials Symposium (IMSP’2014) (pp. 784–793), Denizli - Turkey.
- Capraz, O., Meran, C., Wörner, W., & Gungor, A. (2015). Using AHP and TOPSIS to evaluate welding processes for manufacturing plain carbon stainless steel storage tank. International Scientific Journal, 76(2), 157–162. http://www.amse.acmsse.h2.pl/vol76_2/7628.pdf
- Chauhan, A., & Pradhan, M. K. (2014, December 12th–14th). Selection of non-conventional manufacturing process: A combine TOPSIS-AHP approach. 5th international & 26th All India Manufacturing Technology, Design and Research Conference (AIMTDR 2014) (pp. 991–996). Assam, India: IIT Guwahati.
- Daniyan, I. A., Mpofu, K., & Adeodu, A. O. (2019a). Optimization of welding parameters using taguchi and response surface methodology for railcar bracket assembly. The International Journal of Advanced Manufacturing Technology, 100(9–12), 2221–2228. https://doi.org/10.1007/s00170-018-2878-9
- Daniyan, I. A., Mpofu, K., & Adeodu, A. O. (2019b). Investigating the effect of carbon steel stkm13a thermal properties during welding assembly of railcar suspension system. Procedia CIRP, 81, 886–891. https://doi.org/10.1016/j.procir.2019.03.219
- Daniyan, I. A., Mpofu, K., Fameso, F. O., & Adeodu, A. O. (2020). Numerical simulation and experimental validation of the welding operation of the railcar bogie frame to prevent distortion. The International Journal of Advanced Manufacturing Technology, 106(11–12), 5213–5224. https://doi.org/10.1007/s00170-020-04988-6
- Das, S., & Chakraborty, S. (2011). Selection of non-traditional machining processes using analytic network process. Journal of Manufacturing Systems, 30(1), 41–53. https://doi.org/10.1016/j.jmsy.2011.03.003
- Felice, F. D., & Petrillo, A. (2010). A multiple choice decision analysis: An integrated QFD – AHP model for the assessment of customer needs. International Journal of Engineering, Science and Technology, 2(9), 25–28. https://www.ajol.info/index.php/ijest/article/view/63849
- Ghadge, A., Er Kara, M., Mogale, D. G., Choudhary, S., & Dani, S. (2020). Sustainability implementation challenges in food supply chains: A case of UK artisan cheese producers. Production Planning & Control, 1–16. https://doi.org/10.1080/09537287.2020.1796140
- Gnanasekaran, S., Velappan, S., & Manimaran, P. (2010). An integrated model for supplier selection using fuzzy analytical hierarchy process: A steel plant case study. International Journal of Procurement Management, 3(3), 92–315. https://doi.org/10.1504/IJPM.2010.033447
- Handfield, R., Walton, S. V., & Sroufe, R. (2002). Applying environmental criteria to supplier assessment: A study in the application of the analytical hierarchy process. European Journal of Operational Research, 141(1), 70–87. https://doi.org/10.1016/S0377-2217(01)00261-2
- Jayant, A. (2011). An application of analytic network process to evaluate supply chain logistics strategies. International Journal of Analytic Hierarchy Process (USA), 4(1), 149–163. Doi:10.1051/matecconf/20165703003
- Jayant, A. (2012). Evaluation of 3PL service provider in supply chain management: An analytic network process approach. International Journal of Business Insights and Transformation, 6(2), 78–82.
- Jayant, A., & Singh, M. (2015). Use of Analytic Hierarchy Process (AHP) to select welding process in high pressure vessel manufacturing environment. International Journal of Applied Engineering Research, 10(8), 5869–5884.
- Kardi, T. (2006). Analytical Hierarchy Process (AHP) tutorial. Retrieved June 01, 2020, from http://people.revoledu.com/kardi/tutorial
- Kotsikos. (2016). Fire resistant composite polymer. EURailmag Bus Technology, 27, 1–3.
- Mahase, M. J., Musingwini, C., & Nhleko, A. S. (2016). A survey of applications of multi-criteria decision analysis methods in mine planning and related case studies. The Journal of the Southern African Institute of Mining and Metallurgy, 116(11), 1051–1056. https://doi.org/10.17159/2411-9717/2016/v116n11a7
- Marques, A., Fuinhas, J., & Gonçalves, B. (2012). Dieselization and road transport CO2 emissions: Evidence from Europe. Low Carbon Economy, 3(3), 54–62. DOI:10.4236/lce.2012.33008
- Mathers, G. (2002). The welding of aluminium and its alloys. Woodhead Publishing Series in Welding and Other Joining Technologies.
- Merkisz-Guranowska, A., Merkisz, J., Jacyna, M., Stawecka, H., & Wasiak, M. (2014). Recycling guidelines of the rolling stock. In J. POMBO (Ed.), Railway technology: Research, development and maintenance (pp. 1-11). Civil-Comp Press.
- Mirhedayatian, S. M., Vahdat, S. E., Jelodar, M. J., & Saen, R. F. (2013). Welding process selection for repairing nodular cast iron engine block by integrated fuzzy data envelopment analysis and TOPSIS approaches. Materials & Design, 43, 272–282. https://doi.org/10.1016/j.matdes.2012.07.010
- Mostafa, J., & Vahdat, S. E. (2012). A fuzzy multi-attribute approach to select the welding process at high pressure vessel manufacturing. Journal of Manufacturing Processes, 14(3), 250–256. https://doi.org/10.1016/j.jmapro.2011.10.006
- Musingwini, CA2010. A review of the theory and application of multi-criteria decision analysis techniques in mine planning. Proceedings of Mine Planning and Equipment Selection (MPES) 2010 (pp. 129–140). Melbourne: Australasian Institute of Mining and Metallurgy.
- Nagy, L., Ruppert, T., & Abonyi, J. (2020). Analytic hierarchy process and multilayer network-based method for assembly line balancing. Applied Sciences, 10(11), 1–16. https://doi.org/10.3390/app10113932
- Odu, G. O. (2019). Weighting methods for multi-criteria decision making technique. Journal of Applied Sciences and Environmental Management, 23(8), 1449–1457. DOI:10.4314/jasem.v23i8.7
- Özcan, T., Çelebi, N., & Esnaf, Ş. (2011). Comparative analysis of multi-criteria decision making methodologies and implementation of a warehouse location selection problem. Expert Systems with Applications, 38(8), 9773–9779. https://doi.org/10.1016/j.eswa.2011.02.022
- Perret, B., Schartel, B., Stob, K., Ciesielski, M., Diederichs, J., Döring, M., Krämer, J., & Altstädt, V. (2011). Novel DOPO-based flame-retardants in high-performance carbon-fibre epoxy composites for aviation. European Polymers Journal, 47(5), 1081–1089. https://doi.org/10.1016/j.eurpolymj.2011.02.008
- Petit, P., & Fraser, P. (2013). What is the best energy-delivery system for hand-held stope drilling and associated equipment in narrow-reef hard rock mines? Journal of the Southern African Institute of Mining and Metallurgy, 113(3), 243–249. https://doi.org/10.1016/j.eurpolymj.2011.02.008
- Qian, G., & Mahdi, A. (2020). Sensitivity analysis methods in the biomedical sciences. Institute of Biomedical Engineering University of Oxford.
- Quintiere, J. G., Walters, R. N., & Crowley, S. (2007). Flammability properties of aircraft carbon-fiber structural composite. Technical Report Documentation (pp. 1–43). Springfield, Virginia. https://www.fire.tc.faa.gov/pdf/07-57.pdf
- Saaty, T. L. (2008). Decision making with the analytic hierarchy process”. International Journal of Services Sciences, 1(1), 83–98. https://doi.org/10.1504/IJSSCI.2008.017590
- Sadhu, A., & Chakraborty, S. (2011). Non-traditional machining processes selection using Data Envelopment Analysis (DEA). Expert Systems with Applications, 38(7), 8770–8781. https://doi.org/10.1016/j.eswa.2011.01.088
- Saivaew, N., & Butdee, S. (2020). Decision making for effective assembly machined parts selection using Fuzzy AHP and Fuzzy logic. Materials Today: Proceedings, 26, 2265–2271. https://doi.org/10.1016/j.matpr.2020.02.491
- Salleh, N. H. M, Aman, A. A. and Saharuddin, A. H. (2017). Selection of Artificial Reef Deployment Sites by Using Evidential Reasoning. American Fisheries Society Symposium, 86, 251–264.
- Satish, S. K., & Khod, U. (2015). Implementation TOPSIS-AHP based approach for the selection of third-party reverse logistics providers; a case-study. International Journal of Technical Research, 4(1), 31–38.
- Sharma, A., Gupta, P., & Srivastava, R. K. (2015). Application of AHP and ANP methods for selection of best material for an axle. International Journal of Innovative Research in Science, Engineering and Technology, 4(5), 2894–2901. https://doi.org/10.15680/IJIRSET.2015.0405032
- Silva, R. F., & Kaewunruen, S. (2017). Recycling of rolling stocks: Review. Environments, 4(39), 1–18. https://doi.org/10.3390/environments4020039
- Song, B., & Kang, S. (2016). A method of assigning weights using a ranking and nonhierarchy comparison. Advances in Decision Sciences, Article ID8963214, 1–9. https://doi.org/10.1155/2016/8963214
- Stoliarov, S. I., Walters, R. N., & Lyon, R. E. (2007, June 4-6). Determination of heats of gasification of polymers using differential scanning calorimetry. Proc. 52nd intl. SAMPE symposium & exhibition, Baltimore, MD.
- Subramanian, N., & Ramanathan, R. (2012). A review of applications of analytic hierarchy process in operations management. International Journal of Production Economics, 138(2), 215–241. https://doi.org/10.1016/j.ijpe.2012.03.036
- The Railway Technical Website (2019). Rolling stock manufacture. Retrieved October 20, 2019, from http://www.railway-technical.com/trains/rolling-stock-manufacture.html.
- Toldi, A., Niedermann, P., Pomázi, A., Marosi, G., & Szolnoki, B. (2017). Flame retardancy of carbon fibre reinforced sorbitol based bioepoxy composites with phosphorus-containing additives. Materials, 10(467), 1–12. doi: 10.3390/ma10050467
- Ugur, L. O., & Baykan, U. (2017). A model proposal for wall material selection decisions by using Analytic Hierarchy Process (AHP). Acta Physica Polonica A, 132(3), 577–579. https://doi.org/10.12693/APhysPolA.132.577
- UNECE, United Nations Economic Commission for Europe (2016). Sustainable development brief: Emissions testing for cars and environmental regulations (pp. 1–5). Retrieved October 20, 2019, from http://www.unece.org/fileadmin/DAM/Brief_4__Emissions_testing_for_cars_and_environmental_regulations.pdf
- Velasquez, M., & Hester, P. T. (2013). An analysis of multi-criteria decision making methods. International Journal of Operations Research, 10(2), 56–66.
- Wu, H., Shi, Y., Xia, Q., & Zhu, W. (2014). Effectiveness of the policy of circular economy in China: A DEA-based analysis for the period of Eleventh five-year-plan. Resource Conservation Recycle, 83(1), 63–75. https://doi.org/10.1016/j.resconrec.2013.10.003
- Xiaosong, H. (2009). Carbon fibre. Materials, 2(4), 2369.
- Zeferino, E. F. S., Mpofu, K. Makinde, O. A. Ramatsetse, B. I. and Daniyan, I. A. (2020).FR/CoG Multi-attribute-based Comparison Methods for Selection of the Location of a Research Institute. Journal of Facility Management, 18(1), 20–35. doi:10.1108/JFM-09-2019-0050