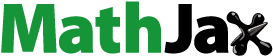
Abstract
The objective of this study was to develop a new method for freight movement in urban areas by combining a light rail transit system and a road transport system to distribute and deliver parcels. Utilisation of light rail transportation systems for parcel delivery can help reduce traffic congestion and air pollution in cities. This paper presents a location–allocation model to determine the optimum number and location of light rail stations suitable to establish rail distribution hubs, in a bid to minimise transportation costs. A case study is conducted to assess this approach in the city of Bangkok. The results show that the cost, time, and distance of transportation can be reduced by 10%, 16.67%, and 33.33%, respectively.
PUBLIC INTEREST STATEMENT
Distribution activities and freight transportation are vital for society, but are also the cause of negative impacts on the quality of living in urban areas in terms of traffic congestion, noise and pollution. A possible solution to these problems is to combine existing light rail transit and road transport for distributing and delivering parcel within urban areas. The main idea is to use the light rail to drive the parcel from the origin to destination station closed to distribution centers that spread throughout the city. This paper shows the method and solution for determining the optimal number and locations of light rail stations to be established as distribution hubs in order to minimize transportation cost in the city of Bangkok.
1. Introduction
Various modes of modern transportation have been developed, supporting the increasing population growth and density. With the increase in demand for shipment of goods, logistics businesses have grown rapidly. Therefore, various strategies to improve the effectiveness of transportation channels are crucial for improved cost efficiency. These strategies should support the future of transportation and logistics, including transportation support for online businesses. The increased freight movement has worsened traffic congestion and air pollution. According to marketing data reports from Thailand, online commerce has increased by 14.03% in 2015–2016 compared to the previous year, by 7.91% in 2016–2017, and by 14.04% in 2017–2018. In the online market, the growth of enterprises with an annual turnover of more than 50 million baht is 8.70%, while small businesses have grown by as much as 20.39% as a result of public and private sectors promoting a cashless society (Thailand e-government, Citation2017). The data show that online commerce has grown steadily, with parcels mainly transported via road. The higher the demand, the greater the freight movement, which has worsened traffic congestion in the city of Bangkok. The problem is further exacerbated by reduced road space because of the ongoing construction of roads, facilities, and mass transit projects. The use of a rail transit system to deliver parcels in the city might relieve traffic congestion and reduce air and noise pollution. Furthermore, it could improve the performance of transport operations in terms of delivery time and cost, which can lead to improved customer satisfaction. Therefore, this paper proposes a new method for parcel transportation via a mass rapid transit in the city of Bangkok. The method uses the location–allocation technique to determine the locations of transport stations along railway lines to minimise the transportation cost. A mathematical model is proposed to solve the problem of location selection, and it yields the proposed restrictions.
2. Relevant applications and literature review
Many countries have started using rail transportation systems for passenger and freight movement within urban areas for three main reasons. The first is in response to predicted growth in shipments. For example, product shipments in Belgium increased by more than 3% between 2013 and 2018. The second is in response to the increase in population and expansion of the city, which is anticipated to increase the transfer rate of passengers and parcels within and between urban areas. Merchandise transportation and movement of people are correlated (Trentini et al., Citation2013). These activities increase the transport rates between and to the outskirts of urban areas. The third is that rail transport helps mitigate environmental pollution. Behrends (Citation2011) stated that the EU should make more efforts in implementing policies for more efficient freight transportation within cities and surrounding towns. Jena et al. (Citation2016) introduced environmental-friendly modes of transportation by conducting research and studying the possibility of rail transport in urban areas. According to Ford et al. (Citation1995), railways can reduce energy consumption (tonnes/km) by up to 50% compared to road freight systems. They can also help increase the capacity for lightweight products. However, the main drawbacks of distributing goods within cities using rail systems are the poor door-to-door delivery service, lack of flexibility, need for railway infrastructure, and high cost of the systems involved (Robinson & Mortimer, Citation2004).
The CarGo Tram Project implemented in Dresden, Germany, is a successful example of a city rail transportation system. The project was funded by Volkswagen in collaboration with the Dresden Digital Video Broadcasting Project (DVB); the traffic organisation of Dresden was established in March 2001. Connecting Volkswagen’s factory and the logistics centre in Dresden, the CarGo Tram is practical and straightforward. It has only one departure point, one target point, and operators and customers in one location (Quak, Citation2008). The CarGo Tram uses a public rail network and creates freight schedules as a loop transport system. Freight can be transported by a locomotive trailer attachment, enabling the trams to carry the entire length of the locomotive. The CarGo Tram reduces carbon dioxide emissions and traffic at the centre of Dresden. Marufuzzaman et al. (Citation2016) studied the “Grand Paris” project, which aimed to shift toward a resilient smart city through innovation in services and infrastructure. The existing Parisian rail network is one of the largest in Europe and aims to add another 200 km of rail lines with 68 stations.
Behiri et al. (Citation2018) developed a model to investigate the feasibility and evaluate the potential benefits and other impacts of alternatives using passenger rail infrastructure in the Parisian metropolitan area. The model could provide better solutions to support decision makers to schedule customer demands. Hu et al. (Citation2020) proposed a simulation model to evaluate the operation performance of urban rail freight transportation in the city of Beijing. The results showed that the urban rail freight transportation system could reduce traffic congestion, air pollution, and accidents, along with improving logistics networks. Li et al. (Citation2021) developed an optimisation model for urban freight transportation to support the collaborative transportation of passengers and freight. The solution provided the most profitable train service and freight allocation plans. Motraghi and Marinov (Citation2012) presented a simulation model to analyse the existing metro rail system in Newcastle upon Tyne. The metro rail model was represented in the ARENA simulation computer software. The analyses of both these scenarios showed that rail transportation used for freight distribution in urban areas is beneficial to the existing system, through opportunities for new businesses, increased profits, and optimised utilisation of resources. Moreover, the rail system could accommodate 5% more goods.
Ishfaq and Sox (Citation2011) developed a mathematical model using multiple allocation to design a road–rail intermodal transportation network. Muñoz-Villamizar et al. (Citation2018) used a biased randomisation-based algorithm to solve problems with a multi-objective function and explore the relationship between delivery and environmental costs in the implementation of an electric fleet of vehicles in an urban goods distribution system for the city of Bogotá, Colombia. Hu et al. (Citation2020) established a bi-objective mixed-integer linear programming model for minimal costs and maximum system utilisation to formulate the location–allocation task for an underground logistics system in Beijing. Ghodratnam et al. (2020) developed a new hub location–allocation model to minimise the total costs and the sum of waiting times for processing goods in factories and warehouses. In this study, small and medium-sized problems were solved and effective solutions were obtained using the proposed model. Lin et al. (Citation2012) used an optimisation model to solve train connection services in China, which was a large-scale problem consisting of 5,544 stations and over 520,000 shipments. The problem was highly complex and hard to solve using mathematical models. A simulated annealing algorithm was, therefore, developed to find the solution.
3. Problem description and mathematical modelling
This study introduces a method for alternating the mode of transportation for goods and parcels. In cities with traffic congestion, vehicles are associated with greenhouse gas emissions and other types of pollution. Therefore, the transportation and distribution of goods should be realised by a combination of public transport systems using light rail and road carriages. The problem of effective transportation can be solved using both light rail trains and road vehicles, instead of the current forms of transportation, to deliver products from the origin to the destination distribution centre. Currently, parcels are delivered from distribution centres to other destination distribution centres by road. Each distribution centre acts as an origin and destination distribution centre for other distribution centres. In the proposed method of integrating a city’s existing light rail transit system with road transportation, parcels will be picked up by a pickup truck or motorcycle and transported by road from the origin distribution centres to the rail distribution hubs, which are located at rail stations. The parcels will then be distributed using a light rail system to their destination rail distribution hubs. Finally, road carriages will be used to carry the parcels from the rail distribution hub to the destination distribution centres. shows a conceptual image of the light rail transit system. Thus, the problem addressed in this study is the selection of light rail stations, which serve as rail distribution hubs or service points to deliver parcels through the light rail system. The objective of the model is to minimise the overall costs, including the cost of establishing a rail distribution hub, transportation cost, and delivery cost. The notations used in the proposed mathematical model are as follows:
Index
idistribution centres (i = 1, 2, 3 …, I)
jlight rail stations (j = 1, 2, 3 …, J)
tplanning periods (t = 1, 2, 3 …, T)
Parameters
Inumber of distribution centres
Jnumber of light rail stations
Tnumber of planning quarters
fixed cost of establishing a rail distribution hub at station j
transportation cost from distribution centre i to light rail station j (unit cost/km)
delivery cost from distribution centre i to light rail station j (unit cost/box)
demand quantity to be delivered from distribution centre i of period t (box)
capacity of light rail station j (box)
distance from distribution centre i to light rail station j (km)
maximum allowance distance between distribution centre i and rail distribution hub j (km)
Malarge value equal to 10,000,000,000
Decision variables
1 if a rail distribution hub is opened at a light rail station j at period t, 0 otherwise
1 if the parcels are transported from distribution centre i to rail distribution hub j at period t, 0 otherwise
transport quantity from distribution centre i to rail distribution hub j at period t
The problem is formulated as follows:
Subject to
The objective function (1) seeks to minimise the sum of the establishment cost, transportation cost, and delivery cost. The establishment cost is the fixed cost of opening a rail distribution hub at any light rail station. The transportation and delivery costs are the costs of transporting and carrying parcels from the distribution centre to the rail distribution hub, respectively. Constraint (2) ensures that the quantity of parcels delivered from the distribution centre i at period t does not exceed the capacity of the rail distribution hub j. Constraint (3) assigns each distribution centre to exactly one rail distribution hub. Constraint (4) deals with the quantity transported from the distribution centre, which must be equal to the demand quantity to be delivered. Constraint (5) ensures that the transport quantity must be less than or equal to the large value M defined above. Constraint (6) states that the transport distance between the distribution centre and the rail distribution hub should not be greater than the specified maximum allowance distance. Constraint (7) ensures that when a rail distribution hub is established, it is more than or equal to the amount of transport. Constraint (8) requires that the quantity to be transported must be at least zero. Constraints (9), (10), and (11) define variables.
4. Application to the city of Bangkok
In Bangkok, the capital city of Thailand, three separate mass transit systems are currently in operation to provide a long-term solution to traffic congestion on roads. The first one is the Bangkok Mass Transit System (BTS), operated by the Bangkok Mass Transit System Public Company (BTSC); the routes cover various stations in Bangkok and its surrounding areas. The second system is the Metropolitan Rapid Transit (MRT) System, which is operated by the Mass Rapid Transit Authority of Thailand (MRTA). The MRT is part of the rail transport network in Bangkok and its surrounding provinces, along with BTS Skytrain in Bangkok and the Suburban Railway System (Red Line) of the State Railway of Thailand (SRT). The third mass transit system is the Suvarnabhumi Airport Rail Link (ARL), which is a special mass transportation system project. Its service format is that of a heavy rail transit system with elevated runways at a height of 20 m throughout the project, except at the entrance to Suvarnabhumi Airport. This sky train is lowered to the ground level after crossing the Suvarnabhumi 2 Road and then to a subway station at a depth of 12 m underground. The rail size is 1.435 m (European standard gauge). shows the current routes and points of connection of the three mass transit systems.
Figure 2. Map of the existing transit system network in Bangkok (Bangkok Expressway & Metro Public Company Limited, Citation2018)
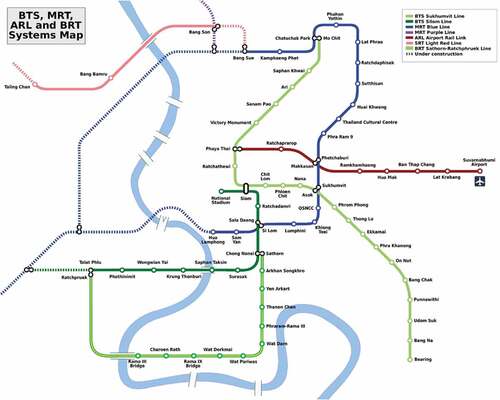
This section presents the results of the computational experiments conducted to find the optimum number and location of rail distribution hubs. A numerical study was conducted on freight transport using the light rail transit system in Bangkok. The company associated with our case study currently has 15 distribution centres for pick-up and drop-off, located in the Bangkok metropolitan area. Sixty vehicles in two sizes (20 pickup trucks and 40 motorcycles) were used to deliver parcels from the origin to the destination distribution centre. Each pickup truck can carry an average of 500 boxes, whereas the motorcycles can carry 50 boxes. The transportation cost of a four-wheel pickup truck was 7.45 baht per kilometre whereas it was 4.9 baht per kilometre for a two-wheel motorcycle. Currently, there are 97 light rail stations in total, with each supporting a maximum of 1,500 boxes of parcels. The establishment cost of one rail distribution hub was 100,000 baht. The maximum acceptable distance between a distribution centre and a rail distribution hub was 50 km. The actual shipping distances were obtained from Google Maps. lists the transport demand quantity of each distribution centre in 12 periods. The hub location–allocation problem to determine the locations of transport stations along railway lines in this case study can be categorised as a small and medium-sized problem. Therefore, the proposed mathematical model can be used to solve the problem. The computer software Lingo version 13.0 was used to solve the numerical model, which is a mixed-integer linear programming (MILP) problem.
Table 1. Transport demand quantity to be delivered from each distribution centre
The result showed that the optimum number of rail distribution hubs is 13 for the 97 light rail stations (station nos. 1–97). shows the selected light rail stations corresponding to the locations of the rail distribution hubs. As shown in the figure, the 13 selected stations cover the area for parcel transport and distribution based on customer demand. presents the selected station at which to establish the rail distribution hub and quantity to be transported for each distribution centre through a rail distribution hub during the planning horizon. shows the comparisons of the average transport cost, time, and distance between the current and the proposed freight transportation method. The cost of transportation decreased by 10%, the average transport time by 16.67%, and the transport distance by 33.33%. The decrease in the cost and time due to the decrease in transport distance was attributed to the positioning of the distribution hubs along the rail stations of the mass rapid transit system. Therefore, the efficiency of the light rail transit system is higher than that of the current system; this is because the light rail transit system has a shorter distance and less interruption.
Table 2. Result of selected station for rail distribution hub, and distribution centre and transport quantity
Table 3. Comparison of conventional and proposed transport methods
The additional cases for solving a large number of problems were established by increasing the number of distribution centres to 19, 23, 27, and 31. lists the results of the rail distribution hub for all the five cases. The optimum number of rail distribution hubs increases with the increase in the number of distribution centres. Different rail stations will be selected for a rail distribution hub because of the difference in the transport demand quantity and the distance from the distribution centre to the rail distribution hub. Some stations have been selected frequently; these are station nos. 97 and 5. In addition, station no. 97, which is Phaya Thai station, is the interchange station in the inner city. This implies that these stations are potential locations for fulfilling customer demand while minimising the total logistics cost.
Table 4. Computation results for the optimum number of rail distribution hubs with increasing number of distribution centres
5. Additional experiments
In this section, the sensitivity of the solutions based on variations of the transport demand were analysed. The problems with increasing the number of distribution centers (i.e., 15, 19, 23, 27, 31) were solved by proposed mathematical model. The sensitivities of the solutions in terms of the total cost and the selected stations for the rail distribution hubs when the transport demand level for each period varying by ±20%, ±15%, ±10%, and ±5% from its primal transportation demand were reported.
lists the results of all 45 example scenarios and shows the trends of the total cost. Transport demand 0% is the primal transport quantity. The sensitivity result suggests that when the transport demands are increased or decreased, the stations established as the rail distribution hubs are to be changed slightly, resulting in minor changes in the total costs. The model tries to respond to customer requirements while minimising the total costs of transportation and distribution services. The marginal change in the number of rail distribution hubs and their locations is due to constraint (6), which limits the transport distance between the distribution centre and the rail distribution hub, and the available capacity of the rail distribution hubs is sufficient to support the demand. represents the counted quantity of each mostly selected station to establish the rail distribution hub from all 45 scenarios. Station number 97, Phaya Thai station, is the most selected from all scenarios. The station is located in central Bangkok and is surrounded by several government buildings and office towers. The population along with condominiums is more concentrated in the area. Moreover, the station is the origin of the Airport Rail Link Line that connects Bangkok city centre to Suvarnabhumi International Airport. There is a direct interchange to the BTS Sukhumvit Line that connects the station to the rest of Bangkok.
Table 5. Computation results of each scenario
6. Conclusions
This study developed a location–allocation model to determine appropriate rail distribution hubs in a light rail transit system to deliver parcels in urban areas more efficiently. This method is applied to the light rail transit network in Bangkok, Thailand. The result illustrates that the proposed model is able to produce practical solutions. The stations to establish an optimal rail distribution hub cover the area for parcel transport and distribution based on customer demand. The light rail transit system can also improve the efficiency of the transportation process and reduce the transportation cost by 10%. Moreover, the transportation time can be reduced. In addition, the light rail transit system could alleviate the problems of traffic congestion and pollution, which are the main contributors to the greenhouse effect. Therefore, the proposed method can improve the performance of transport operations and reduce impact on the environment, making it a suitable plan for parcel distribution in urban areas. Furthermore, the proposed concept and model can be applied to a city like Bangkok which has high demand for freight movement and a problem of traffic congestion. A heuristics approach can be developed further for more complex problems, especially when the numbers of train stations and distribution centres increase. This study can also be extended by considering a light rail timetable for collaborative planning and scheduling of passenger and freight transportation.
Acknowledgements
This research article is supported by Research and Graduate Studies, Khon Kaen University.
Disclosure statement
The authors declare no conflict of interest regarding the publication of this paper.
Additional information
Funding
Notes on contributors
Arthit Apichottanakul
Arthit Apichottanakul has been working as a lecture in the Department of Production Technology, Faculty of Technology, Khon Kaen University, Thailand. His current research interest includes intelligent applications, optimization and data science in logistics and supply chain management. Nanpipat Thanawaritwatthana is currently a M.Eng. student in the Department of Industrial Engineering, Faculty of Engineering, Khon Kaen University, Thailand. He received his B.E. in industrial engineering from Khon Kaen University in 2017. His research of interest includes supply chain & logistics optimization. Sirawadee Arunyanart has been working as a lecturer in the Department of Industrial Engineering, Faculty of Engineering, Khon Kaen University, Thailand. Her research interest includes supply chain & logistics optimization and decision analysis.
References
- Bangkok Expressway & Metro Public Company Limited. (2018). Route map. Accessed October 3, 2019. from https://metro.bemplc.co.th/MRT-System-Map.
- Behiri, W., Belmokhtar-Berraf, S., & Chu, C. (2018). Urban freight transport using passenger rail network: Scientific issues and quantitative analysis. Transportation Research Part E: Logistics and Transportation Review, 115, 227–14. https://doi.org/10.1016/j.tre.2018.05.002
- Behrends, S. (2011). Urban freight transport sustainability. The interaction of urban freight and intermodal transport. PhD Thesis, Chalmers University of Technology.
- Ford, H., Smith, R., & Harris, N. (1995). Environmentally driven challenges, The railways - Challenges to science and technology. Proceedings of a Conference held at the Royal Society on 26 April 1995. Sheffield Academic Press, Sheffield.
- Ghodratnama, A., Arbabi, H. R., & Azaron, A. (2020). A bi˗objective hub location‐allocation model considering congestion. Operation Research, 20(4), 2427–2466. https://doi.org/10.1007/s12351-018-0404-3
- Hu, W., Dong, J., Hwang, B.-G., Ren, R., Chen, Y., & Chen, Z. (2020a). Using system dynamics to analyze the development of urban freight transportation system based on rail transit: A case study of Beijing. Sustainable Cities and Society, 53, 101923. https://doi.org/10.1016/j.scs.2019.101923
- Hu, W., Dong, J., Hwang, B.-G., Ren, R., & Chen, Z. (2020b). Hybrid optimization procedures applying for two-echelon urban underground logistics network planning: A case study of Beijing. Computers & Industrial Engineering, 144, 106452. https://doi.org/10.1016/j.cie.2020.106452
- Ishfaq, R., & Sox, C. R. (2011). Hub location–allocation in intermodal logistic networks. European Journal of Operational Research, 210(2), 213–230. https://doi.org/10.1016/j.ejor.2010.09.017
- Jena, S. D., Cordeau, J. F., & Gendron, B. (2016). Solving a dynamic facility location problem with partial closing and reopening. Computers & Operations Research, 67, 143–154. https://doi.org/10.1016/j.cor.2015.10.011
- Li, Z., Shalaby, A., Roorda, M. J., & Mao, B. (2021). Urban rail service design for collaborative passenger and freight transport. Transportation Research Part E: Logistics and Transportation Review, 147, 102205. https://doi.org/10.1016/j.tre.2020.102205
- Lin, B.-L., Wang, Z.-M., Ji, L.-J., Tian, Y.-M., & Zhou, G.-Q. (2012). Optimizing the freight train connection service network of a large-scale rail system. Transportation Research Part B: Methodological, 46(5), 649–667. https://doi.org/10.1016/j.trb.2011.12.003
- Marufuzzaman, M., Gedik, R., & Roni, M. S. (2016). A benders based rolling horizon algorithm for a dynamic facility location problem. Computers & Industrial Engineering, 98, 462–469. https://doi.org/10.1016/j.cie.2016.06.029
- Motraghi, A., & Marinov, M. V. (2012). Analysis of urban freight by rail using event based simulation. Simulation Modelling Practice and Theory, 25, 73–89. https://doi.org/10.1016/j.simpat.2012.02.009
- Muñoz-Villamizar, A., Quintero-Araújo, C. L., Montoya-Torres, J. R., & Faulin, J. (2018). Short- and mid-term evaluation of the use of electric vehicles in urban freight transport collaborative networks: A case study. International Journal of Logistics Research and Applications, 22(3), 229-252. https://doi.org/10.1080/13675567.2018.1513467.
- Quak, H. (2008). Sustainability of urban freight transport. Retail distribution and local regulations in cities. Ph.D. Thesis (Management), Erasmus Universiteit Rotterdam.
- Robinson, M., & Mortimer, P. (2004). Urban freight and rail: The state of the art. Logistics &Transport focus, 6(1), 46–51.
- Thailand e-government. (2017). Value of e-Commerce Survey in Thailand (1th ed.). Electronic Transactions Development Agency.
- Trentini, A., Masson, R., Lehuédé, F., Malhéné, N., Péton, O., & Tlahig, H. (2013). A shared “passengers & goods” city logistics system. 4th International Conference on Information Systems, Logistics and Supply Chain. Quebec, Canada.
- Truck data service center. (2020). Retrieved February 16, 2020 from https://www.thaitruckcenter.com/tdsc