Abstract
The blink reflex presents a promising, objective, neurophysiologic measure for use in sports-related concussion diagnosis and monitoring. The purpose of this study was to describe blink reflex metrics obtained using the EyeStat across various demographic factors in a group of healthy high school and collegiate athletes. Participants were 975 healthy high school and collegiate athletes ages 13–23 who underwent pre-season baseline blink reflex testing with EyeStat from 2018 to 2020. Separate two- and three-way analyses of variance tested the effects of age, sex and race on each blink reflex parameter including latency (ms), differential latency (ms), time under threshold (ln(ms)), oscillations (qty), and excursions (px). Bivariate correlations demonstrated uniformly negligible (|r|<0.2) inter-measure correlations, except between latency and oscillations (r = −0.37). Latency was significantly slower in males versus females (p = 0.001), while latency in 19–21 year olds (college) was faster as compared to 13–15 year olds (high school) (p = 0.008). Differential latency was faster in females versus males (p = 0.033), and Caucasians had faster differential latency values as compared with African Americans (p < 0.0005). The number of oscillations were significantly higher in females versus males (p < 0.0005), and African Americans demonstrating significantly more oscillations as compared to Caucasians (p = 0.006). Excursions were greater in females compared to males (p = 0.003) and Caucasians demonstrated greater excursions as compared to African Americans (p = 0.008). Natural log of time under threshold resulted in significant differences in race, with Caucasians having lower values compared to African Americans (p = 0.026). The current results provide baseline values when applying this new technology to the assessment and monitoring of concussion.
PUBLIC INTEREST STATEMENT
The statistics of concussion and mild TBI indicate 5–6% occurrence in collegiate football and 8–20% in a military-related deployment. Repeated concussive events in military and athletes can result in second impact syndrome or chronic traumatic encephalopathy, both with the potential for long-term disabilities or death. Thus, identifying and properly treating concussive events is a clinical imperative. However, current methods to diagnosis concussion are primarily focused on subjective assessments, which can be modified by the individual. Research suggests that the blink reflex provides an objective, involuntary response of brain function. This objective tool described in our research utilizes the blink reflex and has proven to provide data within baseline, concussion diagnosis and treatment. Emerging data indicates a correlation between mTBI and increased risk for neurological disease later in life. Thus, future research should understand how this technology may play a role in the diagnosis of neurological diagnosis and treatment.
1. Introduction
Athletes participating in collision or contact sports are at heightened risk for a concussive event, (Brett et al., Citation2018; Kerr et al., Citation2019; Zuckerman et al., Citation2015) with roughly 250,000 individuals age 19 and younger visiting a US emergency department for a sport or recreation-related concussion (SRC) annually (DePadilla et al., Citation2018). Repeat SRC are prevalent, with 9% of collegiate SRCs and 6% of high-school SRCs presenting as recurrent injuries. Furthermore, playing through a SRC while symptomatic is associated with prolonged recovery and increased risk of recurrent injury (Asken et al., Citation2016; McCrea et al., Citation2020). The need to accurately diagnose SRC and track recovery is critical to an athlete’s health. However, validated, objective measures are lacking to diagnose a SRC or assess the recovery process, forcing providers to rely on clinical intuition, diagnostic adjuncts, and subjective symptom assessment (Garcia et al., Citation2019; Okonkwo et al., Citation2014; Patricios et al., Citation2017). Therefore, the field needs SRC-diagnostic and monitoring technology that is user-agnostic, adaptable and precise.
Current SRC adjunct assessments first establish the athlete’s baseline, which is measured against a post-concussion assessment in the areas of cognition, balance, and/or visuomotor abilities (Broglio et al., Citation2019; Garcia et al., Citation2020; Legarreta et al., Citation2019; Mummareddy et al., Citation2018; Yengo-Kahn et al., Citation2016). SRC assessment tools include the Acute Concussion Evaluation, which queries for the presence of concussion symptoms and the SCAT-5 (Sports Concussion Assessment Test), which provides information about the injury, symptoms, cognition, and balance (Echemendia et al., Citation2017; McCrea et al., Citation2014; Yengo-Kahn et al., Citation2016). However, common concussion assessment tools also assess higher order neurologic function (i.e. cerebral cortex), such as SCAT-5, King-Devick, ImPACT, which may be manipulated by the individual, especially during baseline assessment (Chrisman et al., Citation2013; Schatz et al., Citation2017; Torres et al., Citation2013). Instruments that can identify SRC through objective physiology that cannot be readily manipulated are necessary.
To address these issues, recent technological advances, predominantly involving concussion research, have focused on eye tracking. Oculomotor dysfunction provide a marker of concussion due to widely distributed volitional and reflexive visual and oculomotor pathways within the brain (Onge et al., Citation2019). Eye tracking assessments include detailed physical examinations such as the Vestibular Ocular Motor Screening Exam (VOMS) to advanced automated visual tracking combined with neurocognitive assessments (Mucha et al., Citation2014). Several oculomotor changes have been reported in concussion including increased shew, (Howell et al., Citation2018) increased saccades, (Rizzo et al., Citation2016) and deficits in coordinated eye movement (Broglio et al., Citation2018; Samadani et al., Citation2015). While sophisticated oculomotor assessments have demonstrated some success, reliability data is inconsistent which may be attributed to their reliance on higher brain center function (Broglio et al., Citation2018) that requires cognitive effort and can be more readily manipulated by the individual (Chrisman et al., Citation2013; Torres et al., Citation2013). Additionally, computerized cognitive assessments have the potential to exacerbate symptoms during the acquisition of the data due to the cognitive load exerted on the subject during assessments (Covassin et al., Citation2013), with research citing greater symptoms to include headache, nausea, dizziness, depression, mental fogginess and visual problems (Covassin et al., Citation2013).
In contrast to oculomotor assessments, one novel eye-tracking technology (EyeStat) uses non-invasive high-speed videography and algorithms to assess the blink reflex. The mechanically induced blink reflex cannot be manipulated nor relies on the individual to utilize higher brain centers in its response. The EyeStat is a Food and Drug Administration cleared blink reflexometer that incorporates high-speed videography with air puffs to initiate and measure a blink reflex response (Tsai et al., Citation2017). Initial investigations have shown differential performances between athletes at baseline, after active play, and post-concussion (Garner et al., Citation2018). Importantly, early studies have demonstrated dissimilar blink reflex parameters for those engaged in active play compared to those diagnosed with a concussion (Garner et al., Citation2018). Thus, the blink reflex shows promise for use in SRC and could represent a much-needed objective tool to diagnose SRC. However, psychometric data about the blink reflex in normative populations are lacking but are needed to understand changes in the blink reflex following injury.
The purpose of this study was to describe blink reflex metrics obtained using the EyeStat across various demographic factors in a group of healthy high school and collegiate athletes. We hypothesized that EyeStat parameters would vary by demographic subgroups known to affect SRC assessments requiring stratified normative values (Asken et al., Citation2020; Brett et al., Citation2020; Covassin et al., Citation2006).
2. Materials and methodS
2.1. Participants
This study utilizes a subset of data obtained from a larger prospective, non-randomized study of the EyeStat device conducted between 2018 and 2020 at 20 sites within the Southeastern United States. Institutional Review Board approval was obtained and those participants that consented/assented and underwent first-time baseline scans from 2018 to 2020 were eligible for inclusion in this study (n = 1075). Athletes ages >23 or <13 were excluded, as were those participants missing key demographic information. After assessing the overall population for test validity (described below), athletes with invalid tests were excluded (n = 23). Those in racial/ethnic groups with very small frequency (n < 20) were excluded ().
2.2. Testing procedure
Each athlete underwent a baseline EyeStat scan performed by the sites’ certified athletic trainer or physician. Investigational testing utilized the EyeStat device (Generation 3, BlinkTBI, Charleston, SC) pictured in . As described previously, (Garner, Citation2020, Citation2019; Garner et al., Citation2018) once the subject was situated, the test sequence was initiated, in which the computer recorded 20 s of high-speed video (280 frames/sec.) of the subject’s eyes while initiating blink stimuli. Air puffs initiated from a carbon dioxide (CO₂) cartridge delivered filtered air at prescribed and random intervals during the testing period and randomly to the right and left eyes. The device computer utilizes an eyelid tracking algorithm to track the subject’s right and left eyelids. The algorithm differentiated stimulated blinks and spontaneous blinks, and the data from the location of the eyelids was outputted along with calculations of each blink’s characteristics. Typical testing sessions, from start to finish, occurred over a period of approximately 2 minutes.
2.3. Measures
To establish reference positions for blink parameter measurements, a rest position was defined as the “Tonic Lid Position” or the moving average of the pixel location of the top eyelid when not in a blink. The “Threshold” was defined as 20 pixels below the tonic lid position. From the displacement profile for each eyelid, five primary measures were calculated and considered for normative data. A graphical representation is provided in and the full definitions are provided in .
Table 1. Definitions of EyeStat parameters
Figure 3. A-E: Diagrammatic representation of parameters paired with eye photographs of a stimulated blink. A-D: Sequential stages of the stimulated blink reflex with paired eyelid location over time charting. E: Complete charting of a stimulated blink reflex with parameters labeled. The “threshold” line is considered the horizontal mid-pupil position. Time under threshold (not shown) measures the total time the eyelid remains below the mid-pupillary line (i.e. threshold).
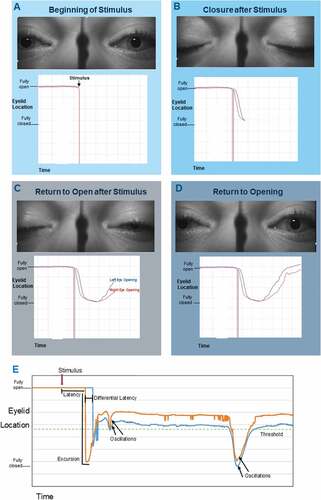
2.4. Statistical analyses
Outlier thresholds for each outcome measure were determined on the full cohort with complete data (n = 1022) by calculating the interquartile range [IQR] using Tukey’s hinges. Standard outliers were defined as values 1.5 times the IQR outside the 25th or 75th percentile. Extreme outliers were 3 times the IQR outside the 25th or 75th percentile. If any 1 metric fell outside of the extreme outlier threshold, or if ≥2 metrics were outside of the standard outlier threshold, the entire test was excluded from normative analyses. Bivariate correlations were performed to assess inter-measure relationships as well as the effect of age on each measure. Age was included in further multivariable analyses if a statistically significant correlation was detected after correcting for multiple comparisons (adjusted p < 0.01).
Given the influence of sex and race on other baseline neurocognitive metrics used in the evaluation of head trauma, (Asken et al., Citation2020; Brett et al., Citation2020) these variables were selected as potential modifiers to be tested for all measures. Two or three-way analysis of variance (ANOVA) was performed using age (if a significant bivariate correlation existed), sex and race to examine their effects on each of the five EyeStat measures. If no statistically significant interaction was detected, normative data (i.e., descriptive and percentiles) were stratified and calculated by modifying factors with significant main effects on ANOVA testing. Race and sex were binary variables and post-hoc testing was performed using independent samples 2-tailed t-tests. Given small sample sizes at the extremes of age, age was grouped into four, intuitive, sizeable groups for testing: 1) early high school (ages 13–15), 2) late high school (16–18), 3) early college (19–21), 4) late college (22–23) for testing. Post-hoc (Tukey’s Honestly Significant Difference) tests were used to identify specific differences between age groups. Statistical significance was set a priori at p < 0.05. Statistical analyses were performed with IBM SPSS Statistics 27 (IBM Corp, Armonk, NY).
3. Results
Data from the EyeStat were processed and analyzed based on each of the blink reflex parameters, which included latency, differential latency, oscillations, excursions, and natural log of time under threshold. Standard and extreme outliers were calculated () and 14 tests (1.4%) were invalidated for having at least 1 measure being an extreme outlier and 9 additional tests were invalidated (0.9%) for having 2 or more measures being standard outliers. After the application of all inclusion criteria, 975 athletes with valid baseline tests were included who were mostly male (71%) and Caucasian (76%). Athletes were mainly between 16 and 21 years of age (81%) with the majority being college-aged (64%). The cohort’s basic demographics are provided in .
Table 2. Invalid test thresholdsa
Table 3. Study cohort demographics
Bivariate correlations showed that inter-measure correlations were uniformly very weak or negligible (|r|<0.2) except for the relationship between latency and oscillations, which were weakly correlated (r = −0.37). Given these weak or negligible correlations, each measure was tested as a separate outcome. Age was only very weakly correlated with latency (r = −0.17, p < 0.0005) and there was no relationship between age and the other measures. Due to this, age was only considered in the further analysis of latency.
3.1. Latency
A three-way ANOVA was performed to consider the effects of age, sex, and race on latency. There were no statistically significant interactions detected between the three independent variables (F(3,959) = 0.924, p = 0.428), age and race (F(3,959) = 0.547, p = 0.650), age and sex (F(3,959) = 0.057, p = 0.982), or sex and race (F(1,959) = 0.152, p = 0.697). Main effects were detected for sex (F(1,959) = 10.19, p = 0.001, n2 = 0.011) and age (F(3,959) = 3.97, p = 0.008, n2 = 0.012), but not race (F(1,959) = 0.877, p = 0.349, n2 = 0.001).
Post-hoc testing demonstrated slower mean latency in males versus females (49.5 ± 7.6 vs. 46.2 ± 7.1, p < 0.0005, Cohen’s d = 0.43). Individuals aged 19–21 (early college) demonstrated significantly faster mean latency (47.1 ± 7.3) than high-school athletes ages 13–15 (51.3 ± 7.3, p < 0.0005) and ages 16–18 (50.1 ± 7.4, p < 0.0005), however, there was no significant difference within the pairs of high school or college age groups (). Given the differences observed, normative values were stratified by sex and age with the age groups condensed to two groups: high school (ages 13–18) and college (age >18; ).
Table 4. Normative data for latency by gender and age
3.2. Differential latency
A two-way ANOVA was performed to test the effects of sex and race on differential latency. There was no statistically significant interaction between the two demographic variables (F(1,971) = 0.403, p = 0.526). There was a significant main effect for sex (F(1,971) = 4.57, p = 0.033,n2 = 0.005) and for race (F(1,971) = 24.98, p < 0.005,n2 = 0.025). Females demonstrated faster differential latency than males (4.6 ± 2.4 vs 5.6 ± 2.6, p < 0.0005, Cohen’s d = 0.37) and Caucasian athletes demonstrated faster differential latency than African American athletes (5.0 ± 2.3 vs 6.3 ± 3.1, p < 0.0005, Cohen’s d = 0.55). Normative values were therefore stratified by sex and race ().
Table 5. Normative values of EyeStat parameters differing by gender and race
3.3. Oscillations
A two-way ANOVA was performed to test the effects of sex and race on oscillations. There was no statistically significant interaction between the two demographic variables (F(1,971) = 0.646, p = 0.422). There was a significant main effect for sex (F(1,971) = 18.58, p < 0.0005,n2 = 0.019) and for race (F(1,971) = 7.46, p = 0.006,n2 = 0.008). Females demonstrated more oscillations than males (17.1 ± 8.0 vs 14.6 ± 6.9, p < 0.0005, Cohen’s d = 0.35) and Caucasian athletes demonstrated slightly fewer oscillations than African American athletes (15.1 ± 7.2 vs 16.1 ± 7.7,p = 0.068, Cohen’s d = 0.14). Although, post-hoc testing did not reach statistical significance for an effect of race, normative values were stratified by sex and race given the significant main effect of race observed when the variables were initially tested together ().
3.4. Excursions
A two-way ANOVA was performed to test the effects of sex and race on excursions. There was no statistically significant interaction between race and sex (F(1,971) = 0.819, p = 0.366). There was a significant main effect for sex (F(1,971) = 8.93, p = 0.003,n2 = 0.009) and for race (F(1,971) = 7.04, p = 0.008,n2 = 0.007). Females demonstrated larger mean excursions than males (140.4 ± 24.7 vs 131.8 ± 28.1, p < 0.0005, Cohen’s d = 0.32) and Caucasian athletes showed larger mean excursions than African American athletes (136.9 ± 26.9 vs 125.9 ± 27.6, p < 0.0005, Cohen’s d = 0.41). Normative values were therefore stratified by sex and race ().
3.5. Natural log of time under threshold
A final two-way ANOVA was performed to test the effects of sex and race on the natural log of time under threshold. There was no statistically significant interaction between the two independent variables (F(1,971) = 0.951, p = 0.330). There was a significant main effect for race (F(1,971) = 4.95, p = 0.026, n2 = 0.005), but not for sex (F(1,971) = 0.059, p = 0.808). Caucasian athletes demonstrated slightly lower time under threshold than African American athletes (4.6 ± 0.6 vs 4.7 ± 0.7, p < 0.0005, Cohen’s d = 0.30). Normative values were therefore stratified by race only ().
Table 6. Normative values of natural log of time under threshold by race
4. Discussion
4.1. Main findings
The current cross-sectional, normative study of high school and collegiate athletes provides an initial investigation of the blink reflex, evaluated through five metrics, obtained with the EyeStat device. Demographic variables, such as age, sex, and race, influenced certain aspects of performance and should be considered when interpreting blink reflex data. Latency was slower in males versus females and in high school (13–15) as compared to college (19–21); differential latency was faster in females versus males and in Caucasians versus African Americans; number of oscillations was higher in females versus males and in African Americans versus Caucasians; excursions were greater in females as compared to males and in Caucasians versus African Americans; and natural log of time under threshold was lower in Caucasians as compared to African Americans. Additionally, statistics revealed a very weak correlation between each blink reflex metric, thereby suggesting that the EyeStat device provides multidimensional, objective data that has potential for monitoring athletes after injury. Cut-off values for each metric are also provided for identifying invalid performances. Taken together, the current normative study provides baseline, objective blink reflex data that can be used to monitor athletes after SRC.
4.2. Comparison and interpretation
Our findings align with existing work finding that age, sex, and race influence performance on standard SRC assessment tools, such as ImPACT and the King-Devick (Asken et al., Citation2020; Covassin et al., Citation2006; Legarreta et al., Citation2019; Walton et al., Citation2020). Age has been a significant factor in other concussion assessments, such as neurocognitive testing, (Covassin et al., Citation2012; ImPACT Applications Inc, Citation2012; Walton et al., Citation2020) balance assessment, (Covassin et al., Citation2012; Yengo-Kahn et al., Citation2016) and memory tests (Yengo-Kahn et al., Citation2016). Surprisingly, age only weakly correlated with one of five blink reflex measures. High-school-aged athletes took longer to initiate the blink reflex (increased latency) following the stimulus compared to college-aged athletes, likely reflecting differences in brainstem excitability that change with age (Valls-Sole, Citation2012). A potential explanation for this is that the blink reflex is involuntary and not a learned skill. Unlike neurocognitive tests that improve with maturity, executive function, and overall knowledge, the blink reflex represents a primitive brain function, (Esteban, Citation1999; Helmchen & Rambold, Citation2007) which appears to be essentially stable regardless of age, at least across the age range in this study (13–23 years).
Unlike age, sex-influenced performance on most eye blink metrics, with women showing faster latency and differential latency as well as more oscillations and larger excursions. This pattern is as expected, given previous research suggesting women have quicker blink reflex metrics (Kofler et al., Citation2013). The mechanism by which sex influences blink reflexes is somewhat unknown but may reflect different underlying sensory thresholds between men and women (Valls-Sole, Citation2012). Additionally, another explanation may be linked to increased neural conduction in central pathways, specifically visual evoked potentials, in women as compared to men (Chiappa, Citation1997). However, understanding how SRC influences blink reflex metrics in men and women is an important area of future research, as sex differences are seen in other disease states (Kumari et al., Citation2004).
Racial differences in blink reflex performances were also noted, which aligns with previous research highlighting differing performance on other SRC assessment tools by race, such as ImPACT and King-Devick (Houck et al., Citation2018; Wallace et al., Citation2020). While this racial difference in eye blink reflex is not seen consistently, our findings align with others showing a difference in startle reflex between Caucasians and African Americans (Hasenkamp et al., Citation2008). In these studies, African Americans showed a reduced or slowed startle reflex, which partially aligns with our findings showing that African American males and females tended to show a longer differential latency in the blink reflex. However, there was no difference in overall latency between races. The mechanism behind these differences is unclear, though genetics may play a role given that reflex responsiveness differs by different genetic strains in rodents (Logue et al., Citation1997; Palmer et al., Citation2000). A variation on the serotonin transporter gene allele has been linked to increased hyperactivity of the amygdala, subsequently affecting the startle response (Brocke et al., Citation2006; Lesch et al., Citation1996).
4.3. Implications
As researchers and providers search for complete objectivity in SRC diagnosis, assessment of the blink reflex is a promising area. Assessing the blink reflex with EyeStat is non-invasive and automated. Other assessments such as serum biomarkers (Asken et al., Citation2018) are invasive and potentially painful or troublesome for younger patients. Moreover, both serum and saliva, (Hicks et al., Citation2020) while promising, (Di Pietro et al., Citation2021) require advanced processing, special transport considerations (i.e., refrigeration) and medical expertise in either obtaining and/or processing samples. Other testing focused on the visual and vestibular systems (e.g., King-Devick or Vestibular/Ocular Screening tests) evaluate some objective visuomotor properties, but results may be influenced by patient motivation (Legarreta et al., Citation2019) or subjective responses (Kontos et al., Citation2016). The blink reflex does not rely on athlete effort, nor does it require an expert to interpret the results. Therefore, providing baseline data of athletes as it relates to the blink reflex is the critical first step in providing clarity in the role the blink reflex may play in concussion diagnosing and monitoring. This study provides data to support research in the area of clinical utility of this device.
4.4. Strengths, limitations, future directions
The strengths of the current study include providing an initial assessment of the psychometric properties of the EyeStat using a diverse population of high school and collegiate athletes. Normative data are provided to account for common demographic confounders. Furthermore, we provide an objective definition of invalid tests based on a large cohort. There are several limitations to this study. First, athletes were recruited from a single region within the Southeastern United States. Presumably, the blink reflex would not be expected to demonstrate regional differences, however, nationwide, or international studies are required to support this assumption. Secondly, while most subgroup sample sizes were large enough to calculate normative data, there were a limited number of African American, female athletes, and normative values for this specific subgroup require cautious use until recapitulated with larger samples. Similarly, we did not have medical histories or concussion histories for athletes in this study. The blink reflex is potentially susceptible to medications and neurologic or psychiatric illnesses such as attention deficit hyperactivity disorder (Fine et al., Citation1992). Future studies should assess whether separate normative values accounting for these factors are needed, which has been done with other neurocognitive SRC assessments (Asken et al., Citation2020; Gardner et al., Citation2017). Additionally, while participants were asked about tobacco/cigarette use prior to testing, this information was not verified. This is important, as prior studies have demonstrated a significant effect on the blink reflex with the use of cigarette and tobacco (Evinger et al., Citation1988). Tobacco/cigarette use in the population, while likely minimal due to the population type, should be monitored more closely in future studies. Finally, this study relied on the analysis of single baseline tests; therefore, we are unable to provide measures of test reliability. Further study of the reliability of EyeStat and the potential for blink reflex habituation with multiple testing is required to understand post-injury test results.
5. Conclusion
The purpose of this study was to establish baseline values of the blink reflex in high school and college athletes. The significant findings in latency, differential latency, number of oscillations, excursions, and natural log of time under threshold within gender and/or race suggest this technology can provide meaningful individualized data related to a brainstem response. Additionally, results demonstrated that very weak correlations existed between variables, indicating the blink reflex variables provide unique data, not contingent on one another. This study provides a robust sampling of baseline data to support further data collection as it relates to concussion diagnosis and monitoring within high school and college aged athletes. The EyeStat device provides a simple, fast, and objective method for quantifying different aspects of the blink reflex. While continued research is needed to understand the clinical utility and meaningfulness of each aspect, the current results highlight the potential of the EyeStat in providing a robust, objective, user-agnostic assessment of the blink reflex in athletes.
Nomenclature
Acknowledgements
The authors thank blinktbi for donating EyeStat devices to participating centers.
Additional information
Funding
Notes on contributors
Dena P. Garner
Our research focuses on understanding concussion using an objective tool that assesses changes in the involuntary response of the blink reflex. However, in order to establish the validity of this tool, baseline measures must articulate. Therefore, this research provides a foundation for baseline data of the blink reflex in a group of athletes. We continue to collect baseline data on various populations as well as collecting data on athletes affected by concussion and their response during the concussion recovery trajectory. As we define these changes, across this population and additional populations, it is our goal to determine the efficacy of this assessment tool in the context of concussion and its recovery. More broadly, previous data establishes a link between concussion and later onset of neurological disease(s). Thus, describing blink reflex data within various ages and populations may provide insight on correlations between the blink reflex and neurological diseases.
References
- Asken, B. M., Bauer, R. M., DeKosky, S. T., Svingos, A. M., Hromas, G., Boone, J. K., DuBose, D. N., Hayes, R. L., & Clugston, J. R. (2018). Concussion BASICS III: Serum biomarker changes following sport-related concussion. Neurology, 91(23), e2133–18. https://doi.org/10.1212/wnl.0000000000006617
- Asken, B. M., Houck, Z. M., Schmidt, J. D., Bauer, R. M., Broglio, S. P., McCrea, M. A., McAllister, T. W., & Clugston, J. R. (2020). A normative reference vs. baseline testing compromise for impact: The CARE consortium multiple variable prediction (CARE-MVP) norms. Sports Medicine, 50(8), 1533–1547. https://doi.org/10.1007/s40279-020-01263-2
- Asken, B. M., McCrea, M. A., Clugston, J. R., Snyder, A. R., Houck, Z. M., & Bauer, R. M. (2016). ‘Playing Through It’: Delayed reporting and removal from athletic activity after concussion predicts prolonged recovery. Journal of Athletic Training, 51(4), 329–335. https://doi.org/10.4085/1062-6050-51.5.02
- Brett, B. L., Kuhn, A. W., Yengo-Kahn, A. M., Solomon, G. S., & Zuckerman, S. L. (2018). Risk factors associated with sustaining a sport-related concussion: An initial synthesis study of 12,320 student-athletes. Archives of Clinical Neuropsychology, 33(8), 984–992. https://doi.org/10.1093/arclin/acy006
- Brett, B. L., Zuckerman, S. L., Terry, D. P., Solomon, G. S., & Iverson, G. L. (2020). Normative data for the sway balance system. Clinical Journal of Sport medicine: Official Journal of the Canadian Academy of Sport Medicine, 30(5), 458–464. https://doi.org/10.1097/jsm.0000000000000632
- Brocke, B., Armbruster, D., Muller, J., Hensch, T., Jacob, C. P., Lesch, K.-P., Kirschbaum, C., & Strobel, A. (2006). Serotonin transporter gene variation impacts innate fear processing: Acoustic startle response and emotional startle. Molecular Psychiatry, 11(12), 1106–1112. https://doi.org/10.1038/sj.mp.4001908
- Broglio, S. P., Harezlak, J., Katz, B., Zhao, S., McAllister, T., & McCrea, M. (2019). Acute sport concussion assessment optimization: A prospective assessment from the CARE consortium. Sports Medicine, 49(12), 1977–1987. https://doi.org/10.1007/s40279-019-01155-0
- Broglio, S. P., Katz, B. P., Zhao, S., McCrea, M., & McAllister, T. (2018). Test-retest reliability and interpretation of common concussion assessment tools: Findings from the NCAA-DoD CARE consortium. Sports Medicine, 48(5), 1255–1268. https://doi.org/10.1007/s40279-017-0813-0
- Chiappa, K. H. (1997). Evoked potentials in clinical medicine. Lippincott Williams & Wilkins. https://play.google.com/store/books/details?id=h5mOjnTimT8C
- Chrisman, S. P., Quitiquit, C., & Rivara, F. P. (2013). Qualitative study of barriers to concussive symptom reporting in high school athletics. Journal of Adolescent Health, 52(3), 330–335.e3. https://doi.org/10.1016/j.jadohealth.2012.10.271
- Covassin, T., Crutcher, B., & Wallace, J. (2013). Does a 20 minute cognitive task increase concussion symptoms in concussed athletes? Brain Injury, 27(13–14), 1589–1594. https://doi.org/10.3109/02699052.2013.823656
- Covassin, T., Elbin, R. J., Harris, W., Parker, T., & Kontos, A. (2012). The role of age and sex in symptoms, neurocognitive performance, and postural stability in athletes after concussion. The American Journal of Sports Medicine, 40(6), 1303–1312. https://doi.org/10.1177/0363546512444554
- Covassin, T., Swanik, C. B., Sachs, M., Kendrick, Z., Schatz, P., Zillmer, E., Kaminaris, C., Iverson, G., & Stearne, D. J. (2006). Sex differences in baseline neuropsychological function and concussion symptoms of collegiate athletes. British Journal of Sports Medicine, 40(11), 923–927. https://doi.org/10.1136/bjsm.2006.029496
- DePadilla, L., Miller, G. F., Jones, S. E., Peterson, A. B., & Breiding, M. J. (2018). Self-reported concussions from playing a sport or being physically active among high school students - United States, 2017. MMWR. Morbidity and Mortality Weekly Report, 67(24), 682–685. https://doi.org/10.15585/mmwr.mm6724a3
- Di Pietro, V., O’Halloran, P., Watson, C. N., Begum, G., Acharjee, A., Yakoub, K. M., Bentley, C., Davies, D. J., Iliceto, P., Candilera, G., Menon, D. K., Cross, M. J., Stokes, K. A., Kemp, S. P., & Belli, A. Unique diagnostic signatures of concussion in the saliva of male athletes: The study of concussion in rugby union through MicroRNAs (SCRUM). British Journal of Sports Medicine, 55 (24). Epub ahead of print 23 March 2021. 1395–1404. https://doi.org/10.1136/bjsports-2020-103274.
- Echemendia, R. J., Meeuwisse, W., McCrory, P., Davis, G. A., Putukian, M., Leddy, J., Makdissi, M., Sullivan, S. J., Broglio, S. P., Raftery, M., Schneider, K., Kissick, J., McCrea, M., Dvořák, J., Sills, A. K., Aubry, M., Engebretsen, L., Loosemore, M., Fuller, G., … Herring, S. (2017). The sport concussion assessment tool 5th edition (SCAT5): Background and rationale. British Journal of Sports medicine, 51(11), 848–850. https://doi.org/10.1136/bjsports-2017-097506scat5
- Esteban, A. (1999). A neurophysiological approach to brainstem reflexes. Blink reflex. Neurophysiologie Clinique/Clinical Neurophysiology, 29(1), 7–38. https://doi.org/10.1016/S0987-7053(99)80039-2
- Evinger, C., Sibony, P. A., Manning, K. A., & Fiero, R. A., & Evinger C, Sibony PA, Manning KA, et al. (1988). A pharmacological distinction between the long and short latency pathways of the human blink reflex revealed with tobacco. Experimental Brain Research, 73(3), 477–480. https://doi.org/10.1007/bf00406604
- Fine, E. J., Sentz, L., & Soria, E. (1992). The history of the blink reflex. Neurology, 42(2), 450–450. https://doi.org/10.1212/wnl.42.2.450
- Garcia, -G.-G. P., Lavieri, M. S., Jiang, R., McAllister, T. W., McCrea, M. A., & Broglio, S. P. (2019). A data-driven approach to unlikely, possible, probable, and definite acute concussion assessment. Journal of Neurotrauma, 36(10), 1571–1583. https://doi.org/10.1089/neu.2018.6098
- Garcia, -G.-G. P., Yang, J., Lavieri, M. S., McAllister, T. W., McCrea, M. A., & Broglio, S. P. (2020). Optimizing components of the sport concussion assessment tool for acute concussion assessment. Neurosurgery, 87(5), 971–981. https://doi.org/10.1093/neuros/nyaa150
- Gardner, R. M., Yengo-Kahn, A., Bonfield, C. M., & Solomon, G. S. (2017). Comparison of baseline and post-concussion ImPACT test scores in young athletes with stimulant-treated and untreated ADHD. The Physician and Sportsmedicine, 45(1), 1–10. https://doi.org/10.1080/00913847.2017.1248221
- Garner, D. (2019). Effects of football practice on blink reflex parameters in division I football athletes. Neurology, 93(14 Suppl. 1), S21–S21. https://doi.org/10.1212/01.wnl.0000581036.59302.a6
- Garner, D. (2020). A follow-up study on the effects of football scrimmages on blink reflex parameters in Division I athletes. Neurology, 95(20 Suppl. 1), S3–S3. https://doi.org/10.1212/01.wnl.0000719888.52369.bc
- Garner, D. P., Goodwin, J. S., Tsai, N. T., Kothera, R. T., Semler, M. E., & Wolf, B. J. (2018). Blink reflex parameters in baseline, active, and head-impact Division I athletes. Cogent Engineering, 5(1), 1429110. https://doi.org/10.1080/23311916.2018.1429110
- Hasenkamp, W., Norrholm, S. D., Green, A., Lewison, B., Boshoven, W., Keyes, M., & Duncan, E. (2008). Differences in startle reflex and prepulse inhibition in European-Americans and African-Americans. Psychophysiology, 45(5), 876–882. https://doi.org/10.1111/j.1469-8986.2008.00680.x
- Helmchen, C., & Rambold, H. (2007). The eyelid and its contribution to eye movements. Developments in Ophthalmology, 40, 110–131. https://doi.org/10.1159/000100352
- Hicks, S. D., Onks, C., Kim, R. Y., Zhen, K. J., Loeffert, J., Loeffert, A. C., Olympia, R. P., Fedorchak, G., DeVita, S., Rangnekar, A., Leddy, J., Haider, M. N., Gagnon, Z., McLoughlin, C. D., Badia, M., Randall, J., Madeira, M., Yengo-Kahn, A. M., Wenzel, J.,Middleton, F. (2020). Diagnosing mild traumatic brain injury using saliva RNA compared to cognitive and balance testing. Clinical and Translational Medicine, 10(6), e197. https://doi.org/10.1002/ctm2.197
- Houck, Z., Asken, B., Clugston, J., Perlstein, W., & Bauer, R. (2018). Socioeconomic status and race outperform concussion history and sport participation in predicting collegiate athlete baseline neurocognitive scores. Journal of the International Neuropsychological Society, 24(1), 1–10. https://doi.org/10.1017/s1355617717000716
- Howell, D. R., Brilliant, A. N., Storey, E. P., Podolak, O. E., Meehan, W. P., & Master, C. L. (2018). Objective eye tracking deficits following concussion for youth seen in a sports medicine setting. Journal of Child Neurology, 33(12), 794–800. https://doi.org/10.1177/0883073818789320
- ImPACT Applications Inc. Immediate post-concussion assessment test (ImPACT): Technical Manual, https://www.impacttest.com/pdf/ImPACTTechnicalManual.pdf (2012, accessed 6 April 2013)
- Kerr, Z. Y., Chandran, A., Nedimyer, A. K., Arakkal, A., Pierpoint, L. A., & Zuckerman, S. L. Concussion incidence and trends in 20 high school sports. Pediatrics, 144 (5). Epub ahead of print November 2019. https://doi.org/10.1542/peds.2019-2180.
- Kofler, M., Kumru, H., Schaller, J., & Saltuari, L. (2013). Blink reflex prepulse inhibition and excitability recovery: Influence of age and sex. Clinical Neurophysiology, 124(1), 126–135. https://doi.org/10.1016/j.clinph.2012.07.001
- Kontos, A. P., Sufrinko, A., Elbin, R. J., Puskar, A., & Collins, M. W. (2016). Reliability and associated risk factors for performance on the vestibular/ocular motor screening (VOMS) tool in healthy collegiate athletes. The American Journal of Sports Medicine, 44(6), 1400–1406. https://doi.org/10.1177/0363546516632754
- Kumari, V., Aasen, I., & Sharma, T. (2004). Sex differences in prepulse inhibition deficits in chronic schizophrenia. Schizophrenia Research, 69(2–3), 219–235. https://doi.org/10.1016/j.schres.2003.09.010
- Legarreta, A. D., Mummareddy, N., Yengo-Kahn, A. M., & Zuckerman, S. L. (2019). On-field assessment of concussion: Clinical utility of the King-Devick test. Open Access Journal of Sports Medicine, 10, 115–121. https://doi.org/10.2147/oajsm.s171815
- Lesch, K. P., Bengel, D., Heils, A., Sabol, S. Z., Greenberg, B. D., Petri, S., Benjamin, J., Müller, C. R., Hamer, D. H., & Murphy, D. L. (1996). Association of anxiety-related traits with a polymorphism in the serotonin transporter gene regulatory region. Science, 274(5292), 1527–1531. https://doi.org/10.1126/science.274.5292.1527
- Logue, S. F., Owen, E. H., Rasmussen, D. L., & Wehner, J. M. (1997). Assessment of locomotor activity, acoustic and tactile startle, and prepulse inhibition of startle in inbred mouse strains and F1 hybrids: Implications of genetic background for single gene and quantitative trait loci analyses. Neuroscience, 80(4), 1075–1086. https://doi.org/10.1016/s0306-4522(97)00164-4
- McCrea, M., Broglio, S., McAllister, T., Zhou, W., Zhao, S., Katz, B., Kudela, M., Harezlak, J., Nelson, L., Meier, T., Marshall, S. W., & Guskiewicz, K. M. (2020). Return to play and risk of repeat concussion in collegiate football players: Comparative analysis from the NCAA Concussion Study (1999–2001) and CARE Consortium (2014–2017). British Journal of Sports Medicine, 54(2), 102–109. https://doi.org/10.1136/bjsports-2019-100579
- McCrea, M., Guskiewicz, K., Doncevic, S., Helmick, K., Kennedy, J., Boyd, C., Asmussen, S., Ahn, K. W., Wang, Y., Hoelzle, J., & Jaffee, M. (2014). Day of injury cognitive performance on the military acute concussion evaluation (MACE) by US military service members in OEF/OIF. Military Medicine, 179(9), 990–997. https://doi.org/10.7205/milmed-d-13-00349
- Mucha, A., Collins, M. W., Elbin, R. J., Furman, J. M., Troutman-Enseki, C., DeWolf, R. M., Marchetti, G., & Kontos, A. P. (2014). A brief vestibular/ocular motor screening (VOMS) assessment to evaluate concussions. The American Journal of Sports Medicine, 42(10), 2479–2486. https://doi.org/10.1177/0363546514543775
- Mummareddy, N., Brett, B. L., Yengo-Kahn, A. M., Solomon, G. S., & Zuckerman, S. L. Sway balance mobile application: reliability, acclimation, and baseline administration. Clinical Journal of Sport Medicine, Publish Ahead of Print. Epub ahead of print 25 June 2018. https://doi.org/10.1097/jsm.0000000000000626.
- Okonkwo, D. O., Tempel, Z. J., & Maroon, J. (2014). Sideline assessment tools for the evaluation of concussion in athletes: A review. Neurosurgery, 75(Suppl 4), S82–95. https://doi.org/10.1227/NEU.0000000000000493
- Onge, P. S., St Onge, P., Temme, L. A., O’Brien, K. J., & Byrd, B. K. (2019). Evaluation of the commercial, off-the-shelf (COTS) King-Devick eye tracking system. Military Medicine, 184(Suppl._1), 571–578. https://doi.org/10.1093/milmed/usy380
- Palmer, A. A., Dulawa, S. C., Mottiwala, A. A., Conti, L. H., Geyer, M. A., & Printz, M. P. (2000). Prepulse startle deficit in the Brown Norway rat: A potential genetic model. Behavioral Neuroscience, 114(2), 374–388. https://doi.org/10.1037/0735-7044.114.2.374
- Patricios, J., Fuller, G. W., Ellenbogen, R., Herring, S., Kutcher, J. S., Loosemore, M., Makdissi, M., McCrea, M., Putukian, M., & Schneider, K. J. (2017). What are the critical elements of sideline screening that can be used to establish the diagnosis of concussion? A systematic review. British Journal of Sports medicine, 51(11), 888–894. https://doi.org/10.1136/bjsports-2016-097441
- Rizzo, J.-R., Hudson, T. E., Dai, W., Birkemeier, J., Pasculli, R. M., Selesnick, I., Balcer, L. J., Galetta, S. L., & Rucker, J. C. (2016). Rapid number naming in chronic concussion: Eye movements in the King–Devick test. Annals of Clinical and Translational Neurology, 3(10), 801–811. https://doi.org/10.1002/acn3.345
- Samadani, U., Ritlop, R., Reyes, M., Nehrbass, E., Li, M., Lamm, E., Schneider, J., Shimunov, D., Sava, M., Kolecki, R., Burris, P., Altomare, L., Mehmood, T., Smith, T., Huang, J. H., McStay, C., Todd, S. R., Qian, M., Kondziolka, D., … Huang, P. (2015). Eye tracking detects disconjugate eye movements associated with structural traumatic brain injury and concussion. Journal of Neurotrauma, 32(8), 548–556. https://doi.org/10.1089/neu.2014.3687
- Schatz, P., Elbin, R. J., Anderson, M. N., Savage, J., & Covassin, T. (2017). Exploring sandbagging behaviors, effort, and perceived utility of the ImPACT baseline assessment in college athletes. Sport Exerc Perform Psychol, 6(3), 243–251. https://doi.org/10.1037/spy0000100
- Torres, D. M., Galetta, K. M., Phillips, H. W., Dziemianowicz, E. M. S., Wilson, J. A., Dorman, E. S., Laudano, E., Galetta, S. L., & Balcer, L. J. (2013). Sports-related concussion: Anonymous survey of a collegiate cohort. Neurology: Clinical Practice, 3(4), 279–287. https://doi.org/10.1212/cpj.0b013e3182a1ba22
- Tsai, N. T., Goodwin, J. S., Semler, M. E., Kothera, R. T., Van Horn, M., Wolf, B. J., & Garner, D. P. (2017). Development of a non-invasive blink reflexometer. IEEE Journal of Translational Engineering in Health and Medicine, 5, 3800204. https://doi.org/10.1109/jtehm.2017.2782669
- Valls-Sole, J. (2012). Assessment of excitability in brainstem circuits mediating the blink reflex and the startle reaction. Clinical Neurophysiology, 123(1), 13–20. https://doi.org/10.1016/j.clinph.2011.04.029
- Wallace, J., Worts, P., Moran, R., Mason, J., Wiese, K. K., Swanson, M., & Murray, N. (2020). Socioeconomic status and race as social determinants of health to be considered in clinical use of pre-season vestibular and oculomotor tests for concussion. Translational Research: The Journal of Laboratory and Clinical Medicine, 6, 168–178. https://doi.org/10.18053/jctres.06.202005.002
- Walton, S. R., Broshek, D. K., Freeman, J. R., Hertel, J., Meyer, J. P., Erdman, N. K., & Resch, J. E. (2020). Institutionally based ImPACT Test® normative values may differ from manufacturer-provided normative values. Archives of Clinical Neuropsychology, 35(3), 275–282. https://doi.org/10.1093/arclin/acz068
- Yengo-Kahn, A. M., Hale, A. T., Zalneraitis, B. H., Zuckerman, S. L., Sills, A. K., & Solomon, G. S. (2016). The sport concussion assessment tool: A systematic review. Neurosurgical Focus, 40(4), E6. https://doi.org/10.3171/2016.1.focus15611
- Zuckerman, S. L., Kerr, Z. Y., Yengo-Kahn, A., Wasserman, E., Covassin, T., & Solomon, G. S. (2015). Epidemiology of sports-related concussion in NCAA athletes from 2009-2010 to 2013-2014: Incidence, recurrence, and mechanisms. The American Journal of Sports Medicine, 43(11), 2654–2662. https://doi.org/10.1177/0363546515599634