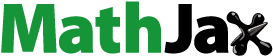
Abstract
The COVID-19 pandemic has caused more than 200 million infected cases and 4 million deaths across the world. The pandemic has triggered a massive epidemic, with a significant effect on the health and lives of many people worldwide. Early detection of this disease is very important for maintaining social well-being. Generally, the RT-PCR test is a diagnosis method used for the detection of the COVID-19, yet it is not the only reliable diagnostic tool. In this study, we discuss the image-based modalities for the detection of coronavirus utilizing Deep Learning methodology, which is one of the most innovative technologies today and has proven to be an efficient solution for a number of medical conditions. Coronavirus affects the respiratory tract of individuals. One of the best ways is to identify this disease from chest radiography images. Early research demonstrated unique anomalies in chest radiographs of covid-positive patients. By using Deep Learning Multi-layered networks, we classified the chest images as covid positive or negative. The proposed model uses the dataset of patients infected with Coronavirus, in which the radiologist indicated multilobar involvements in the chest X-rays. A total of 6500 images have been considered for the study. The convolutional network (CNN) model was trained and a validation accuracy of 94% is obtained.
PUBLIC INTEREST STATEMENT
The COVID-19 pandemic has resulted in millions of deaths across the world. Patients affected by Coronavirus may be found suffering with respiratory problems. In the study the infectious disease are identified from the chest radiography images. The presented computer-aided diagnosis (CADX) technique uses chest X ray, since X ray modality is a very commonly available diagnostic modality. The algorithm developed with the current deep learning technique is very useful for Doctors during the screening of COVID −19 patients. The designed convolutional network (CNN) model was trained, and the validation accuracy obtained are indicating that the method suggested is a promising approach for screening COVID-19 patients. However, with further improvements it can even be used to predict the severity.
1. Introduction
The COVID-19 pandemic has led to more than 200 million infected cases and 4 million deaths across the world. The World Health Organization (WHO) publicly acknowledged the outbreak of a health emergency of global concern at the end of January 2020 and claimed a pandemic on March 11, 2020 (https://www.who.int). The pandemic is triggering a massive epidemic, with a significant effect on the health and lives of many people worldwide. Early detection of this deadly disease is very important. In emergent countries, the most commonly authenticated RT-PCR test for COVID-19 diagnosis is insubstantial, which adds to a rise in illness rates and postponement of important prevention steps. As available resources are limited, hospitals are becoming increasingly overloaded with an exponentially expanding percentage of patients. Perhaps one of the best ways is to identify this disease from chest radiography images (Corman V. M.et al., Citation2020; Fang, Y. et al. Citation2020; M Farooq, Citation2020).
The uncontrolled spread of the SARS-CoV-2 virus is one of the major problems. The RT-PCR test is presently the global standard for diagnosing COVID-19 infection (Gridhar, A. et al., Citation2021). The SARS CoV-2 RT-PCR test identifies viral RNA. A positive result indicates that the coronavirus is present. This process of Polymerase Chain Reaction includes three major steps, that is Denaturation, Annealing, and Elongation, which are repeated 40 times for confirmation of the results. Validation is done by means of genetic relatedness to SARS-CoV-2. In this process, false negatives are a major problem. Sample collection processes, reagent contamination, improper laboratory technique, improper transport, or storage are the biggest contributors to false-negative results, thereby misleading the results. As these processes are time-consuming, an easy diagnostic tool is necessary. The effectiveness of these assays varies and is influenced by the assessment itself, viral inoculum, sample collection location, and testing time. Furthermore, low viral count and sampling errors might have an impact on RT-PCR conclusions. As a result, these procedures have a significant false-positive rate and may need to be repeated two or three times well before outcomes are eventually validated. It may take several hours to days to obtain the results. Building a reliable diagnostic tool for COVID-19 detection is of paramount importance in this scenario (Corman V. M. et al., Citation2020; Kermany, D. S.,et al. Citation2018; Salehi, S., et al. Citation2020).
Accurate Covid estimation and prediction are crucial during this current pandemic. Big Data analytics can be used to develop a suitable model. It will reduce the risk of virus spread and is also cost-effective. No test gives a 100% accurate result (Watson, J. et al., Citation2020). Even the RT-PCR test is insufficient to detect the virus at a very early stage, which adds to a rise in illness rates and postponement of important prevention steps (Wikramaratna et al., Citation2020). To ensure the safety of people and less spread of the virus, a diagnostic and prognostic tools are required. Hence, a fully automated framework is necessary for the early detection of the virus. Currently, there are a limited number of publicly available COVID-19 data in the raw DICOM format. DICOM is the accepted format for displaying data in Medical Imaging. The data is transformed from DICOM to basic visual formats, generally the JPEG and PNG standards that result in loss of informational value and hence creating a lot of bias in our prediction. With the latest development in the neural network, a suitable and easy approach using a deep learning network can be developed.
2. Literature
On the 31 December 2019, the WHO Office in China was notified of instances of pneumonia with an unfamiliar cause in Wuhan City, Hubei Province. On 7 January 2020, Chinese officials declared a new coronavirus as the causal agent (https://www.who.int). The current standard represents the key technique for diagnosis using synthetic nucleic acid technology and is based on the virus’s near genetic relatedness to the SARS coronavirus (Corman Victor M et al., Citation2020). Over 4.1 million new cases and 84000 new fatalities were recorded as of 17 May 2021, representing a 14 percent and 2 percent decline in new cases and fatalities, respectively, relative to the earlier week. Detecting patients affected with Coronavirus illness is crucial. COVID-19 illness is commonly diagnosed using swab assessments, depending on existing health technology breakthroughs (Corman Victor M et al., Citation2020).
Scientists from the GDCDC (Guangdong Provincial Center for Disease Control and Prevention) discovered that the variants develop faster inside the respiratory tracts of infected patients. The most infectious among the different variants of the coronavirus is the delta variant, as it has the power to transmit rapidly with respect to other variants of coronavirus (Baisheng B. Li et al., Citation2021). The viral load in the Delta virus-infected patients was a thousand times greater than those in the earlier alpha or beta strain infections. The spread of the virus has increased due to the rapid transmission of more infective variants, relaxation of social measures, rises in social mixing, and uneven distribution of vaccines. Table lists several vaccines approved by the World Health Organization to prevent the spread of COVID-19.
Table 1. Vaccines on emergency use list approved by WHO (https://www.who.int)
Currently, the RT-PCR test is considered as the golden standard, it is inefficient and unreliable because of the time consumption and sampling errors that it makes. The sensitivity of these assays has been demonstrated to be inadequate for the early identification of the virus (Z. Lin L. Li et al., Citation2020). So, a more accurate and reliable, faster diagnostic technique is necessary. The coronavirus infects the upper respiratory tract as well as the respiratory organ. COVID-19 features appear as bilateral, subpleural, ground-glass opacities in radiography images, ill-defined margins, and quite bulky in the right lobe at the lower side on lung radiography images. Abnormal lung radiography scans might be evident even in affected patients with no symptoms (Shi, H. et al., Citation2020; Khan, A. I. et al., Citation2020). Lesions can rapidly progress into ground-glass opacities within 1–3 weeks after being symptomatic, rising at about 2 weeks (Shi, H. et al., Citation2020). Well over 38 covid patients among 114, who recovered acute coronavirus illness-based pneumonia, had fibrotic-like alterations in their lungs six months later, according to a follow-up CT. Old age, prolonged hospitalizations, arrhythmias, acute respiratory distress syndrome, and use of ventilators were all linked to these alterations (Han, X., et al., Citation2021).
Chest X-ray imaging has shown to be beneficial for diagnosing lung disorders and thus is a beneficial tool in the diagnosis of COVID-19. Deep learning models can be utilized to categorize Covid positive and Covid negative using the chest X-ray images. The computed tomography (CT) has a sensitivity, which is substantially greater than RT-PCR testing (HX Bai et al., Citation2020). CT scanners, however, are not generally available. X-rays are less expensive, more widely available, and less hazardous than CT scans since the radiation dose is lower. Because portable instruments exist, X-ray imaging may be conducted bedside, reducing the risk of infection greatly. AP projections are done on seriously unwell patients, AP and PA data are frequently combined in databases, which might create a bias (Tabik, S. et al., Citation2020). From a medical standpoint, it’s difficult to do X-ray radiography on the patient’s chest in a single projection because this is dependent on the patient’s state. Various deep learning algorithms using chest X-ray images have been extensively studied in the literature. Studies aimed at implementing models that imitated human intellect and abilities to finalize solutions (Piccinini, G. Citation2004). The artificial intelligence techniques may contribute significantly improve the diagnostic facility in case of COVID-19 problems (Shah, S. et al., Citation2022).
A Convolutional Neural Network (CNN) Model for the categorization of chest X-ray images is reported (Kesim et al., Citation2019). The pre-trained CNN models are used in real-world applications, the authors devised a small-sized CNN architecture. The authors reported an average accuracy of 86% in their tests. Turkoglu, M. (Citation2021) proposed an ELM-based network for detecting coronavirus illness in chest CT scan data with an accuracy of 98.36%. A two-stage method is proposed for detecting COVID-19 instances while distinguishing them from bacterial pneumonia, viral pneumonia, and healthy normal individuals (Jain, G. et al., Citation2020). The first stage model distinguishes virus-produced pneumonia, bacteria-driven pneumonia, and healthy individuals, with an accuracy of 93.01%. The X-ray images of virally caused pneumonia were examined further to see if COVID-19 was present. The second-stage model for detecting the presence of COVID-19 performs exceptionally well, with a 97.22% accuracy.
The method proposed by Ozturk, T. et al. (Citation2020) is intended to provide a reliable diagnosis for binary and multi-class categorization. The suggested model has a classification accuracy of 98.08% for binary classes and 87.02% for multi-class cases. The DenseNet model was used as a classifier in their study. Minaee, S. et al. (Citation2020) created a collection of 5,000 chest X-ray images divided into three categories: COVID-19, Non-COVID normal, and Non-COVID other illnesses. They programmed four prominent convolutional neural networks, ResNet18, ResNet50, SqueezeNet, and DenseNet-121, to accomplish a three-class classification with an overall specificity of 90% and sensitivity of 97.5%.
It has been demonstrated that the differences in attributes of medical data from a vast image database and extracting the deep features has become increasingly challenging. As a result, a suitable system for retrieving medical images must be developed (Sampathila, N., et al., Citation2020). Chowdhury, M.E. et al. (Citation2020) created a big dataset of X-ray radiographs of 1579 normal, 1485 viral, and 423 COVID-19 positive pneumonia patients. For 2 distinct categorization schemes, 8 different pre-trained DL networks were trained, validated, and tested. One classification model has been utilized to detect COVID-19 and normal X-ray radiographs, while the other was conditioned to classify normal, viral pneumonia, and COVID-19 pneumonia images. To investigate the influence of image augmentation on this problem, both studies were examined with and without image augmentation techniques. The classification accuracy was 99.7%.
By using deep CNN, Rahman, T. et al. (Citation2021) investigated the impact of several image-enhancing approaches on the automatic detection of COVID-19 from Chest X-Ray (CXR) images. Without segmentation, the classification accuracy was determined to be 96.29% and 95.11% with segmentation. The object detection method using the YOLO model is found to be an interesting automatic detection approach for disease diagnosis using an image-based approach (Khandekar, R. et al., Citation2021). The diagnosis of coronavirus utilizing different CT scans, X-rays, and other pattern analysis, approaches are analyzed. The machine learning-based drug and vaccine development for COVID-19 is also explored. The management of pandemics was also investigated. The primary goal of this paper was to undertake a thorough examination of machine learning applications that combat the lethal virus. This article aids researchers in analyzing the data patterns linked to COVID-19, as well as preparing for a future epidemic caused by new strains (K Chadaga et al., Citation2021).
Elisha Goldstein et al. (Citation2020) studied the classifier using the pre-trained ResNet50 model. Based on similarities to the input image, the nearest neighbor algorithm was used to identify similar test images. The model was evaluated and achieved an accuracy of 89.7%. Asif et al. (Citation2020) suggested a pre-trained model called Inception V3 with transfer learning was suggested for utilizing chest X-ray radiographs to detect coronavirus pneumonia in infected individuals. It has a 98% classification accuracy. In a study, Maghded, H. S. et al. (Citation2020) provided a smartphone-based strategy for alerting individuals when they are near a COVID-19-infected region. The suggested model makes use of positioning data and distance measures from nearby registered users. Maghded,, H. S. et al. (Citation2020) suggested a novel framework for detecting COVID-19 utilizing smartphone sensors. The AI-enabled framework interprets the signal data from smartphone sensors to determine the severity of the condition. Aysen et al. (2021) studied a unique technique for the simultaneous localization, severity grading, and detection of COVID-19 from CXR images by generating infection maps. Experiments indicate that state-of-the-art segmentation networks can be trained to localize COVID19 infection with an F1-score of 83.20%. This method detected COVID-19 with a sensitivity of 94.96% and a specificity of 99.88%.
The study done by Apostolopoulos, I.D. et al. (Citation2020) aimed to assess the effectiveness of CNN designs for image categorization in medical applications. The identification of COVID-19 is obtained with an overall accuracy of 97.82% using transfer learning. In the study done by Hall et al. (Citation2020), ResNet50 and a deep CNN were adjusted in 10-fold cross-validation using 102 COVID-19 cases and 102 pneumonia patients. The total accuracy was found to be 89.2%. The absence of information regarding where the COVID-19, as well as the limited data, set size, are both limitations in this early investigation. Narin, A. et al. (Citation2021) utilized five pre-trained CNN models, such as ResNet50, ResNet101, ResNet152, InceptionV3, and Inception-ResNetV2 for detecting coronavirus pneumonia infected patients utilizing Chest X-rays. Based on the findings, it’s clear that the pre-trained ResNet50 model has the best classification performance of about 96.1%. Importantly, combining real-time RT-PCR with clinical characteristics, particularly lung scans, may make disease treatment easier (Tahamtan, A. et al., Citation2020). Asif et al. (Citation2020) suggested a DCNN-based Inception V3 model with transfer learning for detecting coronavirus pneumonia in infected patients using chest X-rays, and it was found to be more than 96% accurate.
The application of successively pruned deep learning model ensembles for identifying pulmonary symptoms of COVID-19 using chest X-rays, was demonstrated by Rajaraman, S. et al. (Citation2020). The weighted average of the best-performing pruned models considerably increases the performance, resulting in an accuracy of 99.01%. Ismael, A. M. et al. (Citation2021) utilized deep-learning-based methods to categorize COVID-19 and normal chest X-ray images in their research. ResNet18, ResNet50, ResNet101, VGG16, and VGG19 were employed to extract deep features. The overall accuracy for the deep features derived from the ResNet50 model was 94.7%, which was the highest of all the findings.
Wang et al. (Citation2017) demonstrated that using a proven unified weakly supervised multi-label classification and localization framework, many widely occurring thoracic illnesses can be identified and even spatially localized. Lakhani P. et al. (Citation2017) have utilized, deep learning with DCNN and have proved accurate classification from chest images. Sethy, P. K. et al. (Citation2020) demonstrated SVM-based identification of coronavirus using X-ray images and has an accuracy of 95.38%.
The main objective of the proposed work is to develop a classification tool using the conventional neural network extracting deep features. The approach helps in the screening of COVID-19 samples using X-ray images.
3. Methodology
Computational thinking is the major step in solving a research problem. It is important to think about a problem and find a solution to it, using computers. At first, we have to identify if the problem is solvable. We need to require a more conceptual approach to solve the problem before we can come up with a solution or write an algorithm. Then, using an appropriate programming language, we would apply the logical method and direct it to the computer. As a result, we might assume that computational thinking is the process of creating a blueprint and then putting it into action.
In this research work by using deep learning multi-layered networks, we classify chest images as covid positive or negative. We use chest X-rays for the detection of COVID-19 as they are an easily accessible tool, less hazardous than CT since the radiation doses are lower and less expensive. Due to the many advantages of X-rays over CT, we use X-ray images for our study. For the proposed model, we have used the dataset of patients affected with COVID-19, from a publicly accessible platform (https://www.kaggle.com/tawsifurrahman). Kaggle enables its users to access a wide range of datasets in a web-based environment. Figure depicts the chest x-rays of 4 patients, in which the multilobar involvement in the X-rays of two infected patients.
Figure 1. Chest X-ray Images of 4 patients (i) Normal (ii) Affected with coronavirus.(courtesy: https://www.kaggle.com/tawsifurrahman)
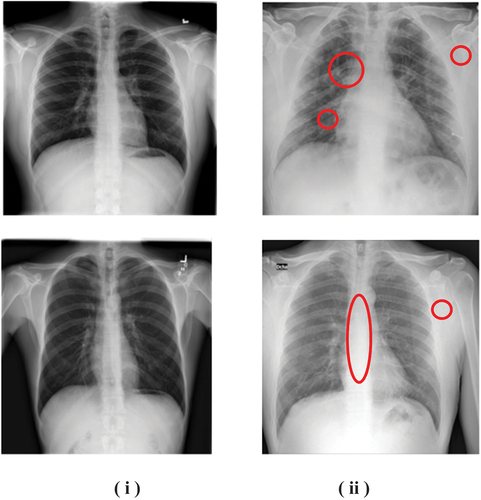
We have selected 6500 images consisting of both the cases (COVID-19 positive and negative) of which 5000 images are used for training and the remaining 1500 images are used for testing the model. The images are in Portable Network Graphics (PNG) file format having a resolution of 299,299 pixels. In this research work, we use modern algorithms to differentiate whether the input sequences of chest X-ray images are covid positive or not. Shown in Figure are the chest X-ray images of four subjects among two are infected with coronavirus. The designed multi-layered deep learning methodologies are useful for automating the diagnosis of infected and uninfected patients.
Deep learning models give more accurate outputs, and especially for image classifications. We extracted the features from the input. Then we trained the model and then evaluated for understanding the performance of the model. We utilized Google Colab GPU for the current research work. The input images used are of size 299 × 299 pixels in resolution. A total of 89,401 neurons forms the input layer. The ReLu (Rectified Linear Unit) activation is set for the neurons. In this work, the CNN model was evaluated experimentally using Python on a Windows 10-based system with an Intel i3 processor.
4. Deep Learning (DL) architecture
Deep Learning is a subset of Artificial Intelligence. Figure depicts the basic architecture of deep learning neural network.
Deep learning also helps in interpreting unstructured data. Since it concerns neural networks with several hidden deep layers that facilitate learning, deep learning can be called a subset of machine learning, which includes methods motivated by the resemblance of the human brain, such as artificial neural networks that adapt from vast quantities of data. Deep learning is particularly effective for assessing complex, rich, and multidimensional data such as speech, videos, and images.
The Figure represents the model for classification of patients affected with coronavirus where C1, C2, C3, and C4 represent the first, second, third, and fourth convolution layers, respectively. P1, P2, P3, and P4 represent the pooling layers used in this model. R1, R2, R3, and R4 represent the ReLu activation function.
5. Convolutional neural networks
The Convolutional Neural Networks (CNN) are the core models in Deep Learning (DL). Such models are built to adapt from data free from any of the utilization of user-defined characteristics. CNNs are advanced and enlarged versions of Artificial Neural Networks (ANNs). CNNs are defined as the deepened network that occurs as a result of extending the number of hidden layers in neural network. Generally, 2-D filters are been used in the design of CNN. CNN has been a widely utilized approach, particularly in medical image analysis and research (Goldstein et al. Citation2020; Tajbakhsh, N., Citation2016).
6. Convolution Layer (CL)
The convolution layer, as its term suggests, is crucial to the network’s operation. This layer is involved with a majority of the calculation and is said to be the core unit of a network. The settings of the layer are centered on the usage of training kernels. These kernels are generally low in spatial dimensions, yet they unfold across the entire dimension of the data deeply. Identifying the kernels that perform best for the network based on a specific set of input data is the ability to design a CNN model.
Kernels are the main factors in the CL that are dynamically learned during the training process. When the input data reaches a convolution layer, the layer convolves with each filter all over the data’s spatial dimensions to create a 2D activation feature map as illustrated in Figure .
7. Pooling
CNN have convolution layers, in addition to this it has layers, such as pooling layers (PL). It implies that the convolution layer’s outputs serve as inputs to the network’s pooling layers. Pooling techniques reduce the size of feature maps by using various functions to summarize subregions, such as taking the highest intensity value as shown in Figure .
Pooling layers attempt to lower the dimensionality of the data over time, reducing the coefficients as well as the model’s complexity.
8. Flattening Layer (FL)
The flattening layer converts mapped data to a one-dimensional array as shown in Figure . The FL is an advanced form of downsampling in which a feature map of dimension X x Y is down-sampled into a 1 × 1 array by directly calculating the average of all the components in each feature map, but the information of feature maps is preserved. The Flattened layer is an input to the dense layer or the fully connected layer.
8.1. Fully connected layer
The output of computations in the deep network is achieved by a fully connected layer (FC layer). In an FC layer, the output has interconnections to all the activations in the preceding layer. As a result, their activations have to be determined using a matrix operation and a bias offset. Even though we can retrieve features of the input data from the output of the CL and PL, using an FC layer is to process combinations of the features, and also it’s a low-cost function.
Deep learning requires a large number of matrix computations that may be extensively parallelized and hence accelerated on GPUs. A GPU can have any number of cores, but a CPU typically has a limited number of cores. Two difficulties restrict GPU’s technical feasibility: long training times and restricted GPU memory, which is deeply affected as the size of the network rises. The cost function evaluates the compliance of the deep network’s output predictions. Cross entropy is an extensively used cost function for classification problems. The Mean Squared Error function cannot be used for complex problems, alternatively Cross entropy function may be used (Gridhar A. et al. Citation2021).
Gradient Descent is a streamlining approach that minimizes the cost function or error to enhance DL network-based models. It is used to update the parameters of our model. The gradient descent algorithm processes by calculating the gradient of the cost function. According to the variations in the cost function, a gradient can evaluate variations in the weight. The maximum the gradient is, the sharper the slope and the more rapid, a model can adapt. But if the slope is zero, the model stops learning. We have used Adam (Adaptive Moment Estimation) optimizer in this model. It’s a method for minimizing error that uses a gradient descent optimizer. It uses projections of the moment of gradients to measure adaptive learning thresholds for various parameters. Adam optimizer helps in improving CNN classification ability. Adam preserves previous information or data. In the same way that momentum preserves a gradually decaying mean of past gradients, Adam preserves previous gradients. The DL network is trained by organizing the data available into the training set and testing set. A total of 6500 images, with a training subset of 5000, and the testing subset of 1500 images are used in this study. The CNN is trained with an epoch of 120 and the batch size chosen was 64. The training data set using input helps in learning, while testing set are used for prediction of the model.
In this proposed work, code is developed using Python, an open-source programming language. It includes the “Numpy” package, which simplifies math operations. Python enables easy data handling involves process such as data augmentation and classification. Tensorflow and Keras are important for designing applications-based deep learning frameworks. Tensors are the best suitable form of representing data in DL. The TensorBoard user-based visualization application allows to keep track of models while being trained.
Tensor flow is an open-source library for significant machine learning and numerical computing. The functions such as tensorflow.keras.layers and tensorflow.keras.models are being used in this context. We have access to different classes in these modules. Both layers are inherited from the Class Layer. The Sequential model is a stack of layers that is based on the presumption that the model has a precisely single input and a single output. Data augmentation is a technique for manually expanding the range of datasets.
To construct a new model, the Class sequentially groups a linear sequence of layers into a model. A layer named Class add adds up a list of inputs. The 2D convolution layer is represented by the Class Conv2D. Maxpooling2D is a class that performs operations on 2D spatial data. The Class Dropout aims to avoid overfitting. Dropout is the most efficient and popular regularization algorithm. It randomly removes some neurons from layers to reduce overfitting.
The Flatten class flattens the input, but the batch size will not be affected. Sigmoid, Step function, Softmax, and ReLU (Rectified Linear Unit Function) are the most common activation functions. ReLU is chosen over other functions because it can train network models faster. ReLU function outputs the input, only if it’s positive. The hidden layer computation was performed with the Dense class and the ReLu activation function. Since we want binary outputs, the ReLu activation function is replaced by the Sigmoid function for the output. The Cross entropy loss between real and expected labels is calculated by BinaryCrossentropy. In Convolution Neural Networks, the input data is driven through a series of layers called the hidden layers. The major steps involved in the multi-layered neural networks are illustrated in Figure . The input image is initially convolved with a feature detector, that is, the filter to obtain corresponding feature maps. Generally, 3 × 3 filters have proven to have better results. The next step is to progressively reduce the spatial size of representation called pooling. This is to ease the Computation process and to make the image readable to the system. Each Convolution is followed by Pooling. Here, we have used 4 Map Dimensions, which are 32, 64, 64, and 128. As 32 has increased to 64, we can say that the dimensional volume has increased. The Fully connected layer (FC) functions with a flattened signal, which ensures that each response is related to every neuron. The suggested DL model comprises weighted layers, in which there are four convolutional layers and one FC layer. A total of 1,735,937 parameters were extracted using CNN Model with a training period of three hours.
The convolutional layers are ensued by ReLU and Max pooling layers. ReLU is the standard activation function in Image categorization. In this study, we have trained CN with 120 epoches. The FC layer is fed as an input to the Sigmoid classifier, which gives the ultimate results if the patient is covid-positive or not. The sigmoid function is a suitable output unit for the binary classification problem. The output is labeled as covid positive or covid negative depending on the features evaluated by the network model on the input image.
9. Algorithm
Initially, Import Necessary Packages in Python.
1: Initialize the Learning rate, number of epochs, and Batch Size.
2: Build Dataset (COVID-19 and Normal Chest X-ray Dataset). Split the data into training and testing sets
3: Load the Data and pre-process it.
4: Build the CNN.
5: Train the Model.
6: Evaluate Model and Visualize the Model Performance.
7: Predict and Evaluate Results.
The performance of the CNN is studied with the estimation of sensitivity, specificity, and F1 scores. The equations for the calculation of performance are represented in Equationequation (1)(1)
(1) to (4);
Here, True Positives, True Negatives, False Positives, and False Negatives are represented by TP, TN, FP, and FN, respectively.
10. Result
The DL model is trained by sorting the data available into the training set and testing set. The training dataset is utilized to train the DL model, and the testing dataset is utilized for validation processes. Initially, the model was trained for 50 epochs. Shown in Figures are the plots of the accuracy and cost function, respectively (for 50 epochs). A total of 6500 chest X-ray radiography images, with a training subset of 5000, and the testing subset of 1500 images are used in this study. The X-ray images are rescaled during the pre-processing phase. The accuracy and cost function are studied with the plots shown in Figures respectively.
The training accuracy was noted to be 90% and the validation accuracy was 89%. Figure shows the increase in validation accuracy with respect to the training accuracy, which is a good sign that indicates the ability of the model in prediction. The training accuracy for 120 epochs is 96% and the validation accuracy of the model is 94%.
represent the cost function for training the model for 50 and 120 epochs, respectively. The performance of the classifier is estimated. The results are being compared in the given Table , which indicates that our model can classify the randomly selected images at about 94% classification accuracy with only one true positive as the false prediction.
Table 2. Comparative studies on methodology and results
The proposed DL model achieves an overall classification accuracy. The Batch size chosen was 64, to make the network better. The network model was trained with a 96% accuracy, and the model was validated, giving an accuracy of 94%. This result indicates the ability of the model to diagnose covid-positive patients. Table compares various methodology and the result. The results are also compared with the proposed work using CNN. The most widespread performance measures in deep learning are accuracy, precision, recall, and F1 score. The values of precision, recall, and F1 score are calculated as shown in Table .
Table 3. Classification report
Degerli, A., et al. (Citation2021) presented work on COVID-19 infection Map Generation and detection from Chest X-Ray Images, and they reported an F1 score of 83.2%. In the proposed work, the F1 score is found improved to 90%. The proposed computer-aided design approach is an intelligent approach using CNN and is a promising method to detect Covid-19 positive and Covid-19 negative cases.
11. Conclusion
The COVID-19 pandemic has caused many deaths across the world, and many research groups have come up with various screening facilities for diagnosing the Covid-19 subjects. Among them, all the solutions are not very much accurate, found improvements. However, the early detection of this deadly disease is very important. In the present study, we discussed a computer-based solution for the prediction of COVID-19 subjects, using X-ray images utilizing the Deep Learning method. The Deep learning model used CNN has proven its capability to detect the covid class with a reasonably good accuracy of 94% and a F1-score value of 90%. The X-ray-based modality is thus playing a major role in the screening of various infections of the lung, and further improvising the AI (artificial intelligence) based early-stage detection algorithms will make the modality more robust.
correction
This article has been republished with minor changes. These changes do not impact the academic content of the article.
Acknowledgements
We would like to acknowledge the Department of Biomedical Engineering Department, Manipal Institute of Technology, MAHE, Manipal.
Disclosure statement
No potential conflict of interest was reported by the author(s).
Additional information
Funding
Notes on contributors
Shahanaz Abdul Gafoor
Shahanaz Abdul Gafoor (Biomedical Engineering), Manipal Institute of Technology, MAHE, Manipal. Her current interest includes Deep learning Applications, Rehabilitation Engineering, Pattern Recognition, and Bio-Informatics.
Niranjana Sampathila
Niranjana Sampathila is a senior member of IEEE and Fellow of IE India. He has more than 25 years of experience in research and academics in the area of Healthcare and applications. Presently working at Manipal Institute of Technology, Manipal Academy of Higher Education, Manipal, India. Research interests: Medical Informatics and healthcare applications.
Madhushankara M
Madhushankara M has more than 12 years of experience in research and academics. Presently working at Manipal School of Information Sciences, Manipal Academy of Higher Education. Research areas: Machine Learning, and Speech technology, Low power VLSI, and Portable medical equipment.
Swathi K S
Swathi K S has more than 10 years of teaching experience. Presently working at Manipal Institute of Management, Manipal Academy of Higher Education, Manipal, India. Research interests: Health Services Management, Healthcare Quality Management, and Health Informatics.
References
- Apostolopoulos, I. D., & Mpesiana, T. A. (2020). Covid-19: Automatic detection from x-ray images utilizing transfer learning with convolutional neural networks. Physical and Engineering Sciences in Medicine, 43(2), 635–18. https://doi.org/10.1007/s13246-020-00865-4
- Asif, S., & Wenhui, Y. (2020). Automatic detection of COVID-19 using X-ray images with deep convolutional neural networks and machine learning. medRxiv.
- Asif, S., Wenhui, Y., Jin, H., & Jinhai, S. (2020, December). Classification of COVID-19 from chest X-ray images using deep convolutional neural network. In 2020 IEEE 6th International Conference on Computer and Communications (ICCC), (pp. 426–433). IEEE.
- Bai, H. X., Hsieh, B., Xiong, Z., Halsey, K., Choi, J. W., Tran, T. M. L., … Liao, W. H. (2020). Performance of radiologists in differentiating COVID-19 from non-COVID-19 viral pneumonia at chest CT. Radiology, 296(2), E46–E54. https://doi.org/10.1148/radiol.2020200823
- Chadaga, K., Prabhu, S., Vivekananda, B. K., Niranjana, S., Umakanth, S., & Pham, D. T. (2021). Battling COVID-19 using machine learning: A review. Cogent Engineering, 8(1), 1958666. https://doi.org/10.1080/23311916.2021.1958666
- Chouhan, V., Singh, S. K., Khamparia, A., Gupta, D., Tiwari, P., Moreira, C., Damaševičius, R., & De Albuquerque, V. H. C. (2020). A novel transfer learning based approach for pneumonia detection in chest X-ray images. Applied Sciences, 10(2), 559. https://doi.org/10.3390/app10020559
- Chowdhury, M. E., Rahman, T., Khandakar, A., Mazhar, R., Kadir, M. A., Mahbub, Z. B., Islam, K. R., Khan, M. S., Iqbal, A., Emadi, N. A., Reaz, M. B. I., & Islam, M. T. (2020). Can AI help in screening viral and COVID-19 pneumonia? IEEE Access, 8, 132665–132676. https://doi.org/10.1109/ACCESS.2020.3010287
- Corman, V. M., Landt, O., Kaiser, M., Molenkamp, R., Meijer, A., Chu, D. K., Bleicker, T., Brünink, S., Schneider, J., Schmidt, M. L., Mulders, D. G., Haagmans, B. L., van der Veer, B., van den Brink, S., Wijsman, L., Goderski, G., Romette, J.-L., Ellis, J., Zambon, M., … Drosten, C. (2020). Detection of 2019 novel coronavirus (2019-nCoV) by real-time RT-PCR. Eurosurveillance, 25(3), 2000045. https://doi.org/10.2807/1560-7917.ES.2020.25.3.2000045
- Degerli, A., Ahishali, M., Yamac, M., Kiranyaz, S., Chowdhury, M. E., Hameed, K., Hamid, T., Mazhar, R., & Gabbouj, M. (2021). COVID-19 infection map generation and detection from chest X-ray images. Health Information Science and Systems, 9(1), 1–16. https://doi.org/10.1007/s13755-021-00146-8
- Fang, Y., Zhang, H., Xie, J., Lin, M., Ying, L., Pang, P., & Ji, W. (2020). Sensitivity of chest CT for COVID-19: Comparison to RT-PCR. Radiology, 296(2), E115–E117. https://doi.org/10.1148/radiol.2020200432
- Farooq, M., & Hafeez, A. (2020). Covid-resnet: A deep learning framework for screening of covid19 from radiographs. arXiv preprint arXiv:2003. 14395.
- Giridhar, A., & Sampathila, N. (2021, March). Application of artificial intelligence to predict the degradation of potential mRNA vaccines developed to treat SARS-CoV-2. In International Conference on Machine Learning and Big Data Analytics, (pp. 85–94). Springer, Cham.
- Goldstein, E., Keidar, D., Yaron, D., Shachar, Y., Blass, A., Charbinsky, L., … Eldar, Y. C. (2020). Covid-19 classification of x-ray images using deep neural networks. arXiv preprint arXiv:2010. 01362.
- Hall, L. O., Paul, R., Goldgof, D. B., & Goldgof, G. M. (2020). Finding covid-19 from chest x-rays using deep learning on a small dataset. arXiv preprint arXiv:2004. 02060.
- Han, X., Fan, Y., Alwalid, O., Li, N., Jia, X., Yuan, M., Li, Y., Cao, Y., Gu, J., Wu, H., & Shi, H. (2021). Six-month follow-up chest CT findings after severe COVID-19 pneumonia. Radiology, 299(1), E177–E186. https://doi.org/10.1148/radiol.2021203153
- Harmon, S. A., Sanford, T. H., Xu, S., Turkbey, E. B., Roth, H., Xu, Z., Turkbey, E. B., Yang, D., Myronenko, A., Anderson, V., Amalou, A., Blain, M., Kassin, M., Long, D., Varble, N., Walker, S. M., Bagci, U., Ierardi, A. M., Stellato, E., … Turkbey, B. (2020). Artificial intelligence for the detection of COVID-19 pneumonia on chest CT using multinational datasets. Nature Communications, 11(1), 1–7. https://doi.org/10.1038/s41467-020-17971-2
- Ismael, A. M., & Şengür, A. (2021). Deep learning approaches for COVID-19 detection based on chest X-ray images. Expert Systems with Applications, 164, 114054. https://doi.org/10.1016/j.eswa.2020.114054
- Jain, G., Mittal, D., Thakur, D., & Mittal, M. K. (2020). A deep learning approach to detect Covid-19 coronavirus with X-ray images. Biocybernetics and Biomedical Engineering, 40(4), 1391–1405. https://doi.org/10.1016/j.bbe.2020.08.008
- Kaggle, https://www.kaggle.com/tawsifurrahman
- Kermany, D. S., Goldbaum, M., Cai, W., Valentim, C. C., Liang, H., Baxter, S. L., McKeown, A., Yang, G., Wu, X., Yan, F., Dong, J., Prasadha, M. K., Pei, J., Ting, M. Y. L., Zhu, J., Li, C., Hewett, S., Dong, J., Ziyar, I., … Zhang, K. (2018). Identifying medical diagnoses and treatable diseases by image-based deep learning. Cell, 172(5), 1122–1131. https://doi.org/10.1016/j.cell.2018.02.010
- Kesim, E., Dokur, Z., & Olmez, T. (2019, April). X-ray chest image classification by a small-sized convolutional neural network. In 2019 scientific meeting on electrical-electronics & biomedical engineering and computer science (EBBT), (pp. 1–5). IEEE.
- Khan, A. I., Shah, J. L., & Bhat, M. M. (2020). CoroNet: A deep neural network for detection and diagnosis of COVID-19 from chest x-ray images. Computer Methods and Programs in Biomedicine, 196, 105581. https://doi.org/10.1016/j.cmpb.2020.105581
- Khandekar, R., Shastry, P., Jaishankar, S., Faust, O., & Sampathila, N. (2021). Automated blast cell detection for acute lymphoblastic leukemia diagnosis. Biomedical Signal Processing and Control, 68, 102690, . https://doi.org/10.1016/j.bspc.2021.102690
- Lakhani, P., & Sundaram, B. (2017). Deep learning at chest radiography: Automated classification of pulmonary tuberculosis by using convolutional neural networks. Radiology, 284(2), 574–582. https://doi.org/10.1148/radiol.2017162326
- LeCun, Y., Kavukcuoglu, K., & Farabet, C. (2010, May). Convolutional networks and applications in vision. In Proceedings of 2010 IEEE international symposium on circuits and systems, (pp. 253–256). IEEE.
- Li, L., Qin, L., Xu, Z., Yin, Y., Wang, X., Kong, B., … Xia, J. (2020). Artificial intelligence distinguishes COVID-19 from community acquired pneumonia on chest CT. Radiology.
- Li, B., Deng, A., Li, K., Hu, Y., Li, Z., Xiong, Q., … Lu, J. (2021). Viral infection and transmission in a large well-traced outbreak caused by the Delta SARS-CoV-2 variant. MedRxiv.
- Maghded, H. S., Ghafoor, K. Z., Sadiq, A. S., Curran, K., Rawat, D. B., & Rabie, K. (2020, August). A novel AI-enabled framework to diagnose coronavirus COVID-19 using smartphone embedded sensors: Design study. In 2020 IEEE 21st International Conference on Information Reuse and Integration for Data Science (IRI), (pp. 180–187). IEEE.
- Maghdid, H. S., Asaad, A. T., Ghafoor, K. Z., Sadiq, A. S., Mirjalili, S., & Khan, M. K. (2021, April). Diagnosing COVID-19 pneumonia from X-ray and CT images using deep learning and transfer learning algorithms. In Multimodal Image Exploitation and Learning 2021 (Vol. 11734, pp. 117340E). International Society for Optics and Photonics.
- Minaee, S., Kafieh, R., Sonka, M., Yazdani, S., & Soufi, G. J. (2020). Deep-covid: Predicting covid-19 from chest x-ray images using deep transfer learning. Medical Image Analysis, 65, 101794. https://doi.org/10.1016/j.media.2020.101794
- Narin, A., Kaya, C., & Pamuk, Z. (2021). Automatic detection of coronavirus disease (covid-19) using x-ray images and deep convolutional neural networks. Pattern Analysis and Applications, 1–14. https://doi.org/10.1007/s10044-021-00984-y
- Ozturk, T., Talo, M., Yildirim, E. A., Baloglu, U. B., Yildirim, O., & Acharya, U. R. (2020). Automated detection of COVID-19 cases using deep neural networks with X-ray images. Computers in Biology and Medicine, 121, 103792. https://doi.org/10.1016/j.compbiomed.2020.103792
- Piccinini, G. (2004). The First computational theory of mind and brain: A close look at mcculloch and Pitts' “logical calculus of ideas immanent in nervous activity”. Synthese, 141(2), 175–215. https://doi.org/10.1023/B:SYNT.0000043018.52445.3e
- Rahman, T., Khandakar, A., Qiblawey, Y., Tahir, A., Kiranyaz, S., Kashem, S. B. A., Islam, M. T., Al Maadeed, S., Zughaier, S. M., Khan, M. S., & Chowdhury, M. E. (2021). Exploring the effect of image enhancement techniques on COVID-19 detection using chest X-ray images. Computers in Biology and Medicine, 132, 104319. https://doi.org/10.1016/j.compbiomed.2021.104319
- Rajaraman, S., Siegelman, J., Alderson, P. O., Folio, L. S., Folio, L. R., & Antani, S. K. (2020). Iteratively pruned deep learning ensembles for COVID-19 detection in chest X-rays. IEEE Access, 8, 115041–115050. https://doi.org/10.1109/ACCESS.2020.3003810
- Salehi, S., Abedi, A., Balakrishnan, S., & Gholamrezanezhad, A. (2020). Coronavirus disease 2019 (COVID-19): A systematic review of imaging findings in 919 patients. American Journal of Roentgenology, 215(1), 87–93. https://doi.org/10.2214/AJR.20.23034
- Sampathila, N., & Martis, R. J. (2020). Computational approach for content‐based image retrieval of K‐similar images from brain MR image database. Expert Systems, e12652. https://doi.org/10.1111/exsy.12652
- Sethy, P. K., & Behera, S. K. (2020). Detection of coronavirus disease (covid-19) based on deep features.
- Shah, S., Mulahuwaish, A., Ghafoor, K. Z., & Maghdid, H. S. (2022). Prediction of global spread of COVID-19 pandemic: A review and research challenges. Artificial Intelligence Review, 55(3), 1607–1628. https://doi.org/10.1007/s10462-021-09988-w
- Shi, H., Han, X., Jiang, N., Cao, Y., Alwalid, O., Gu, J., Fan, Y., & Zheng, C. (2020). Radiological findings from 81 patients with COVID-19 pneumonia in Wuhan, China: A descriptive study. The Lancet Infectious Diseases, 20(4), 425–434. https://doi.org/10.1016/S1473-3099(20)30086-4
- Tabik, S., Gómez-Ríos, A., Martín-Rodríguez, J. L., Sevillano-García, I., Rey-Area, M., Charte, D., Guirado, E., Suarez, J. L., Luengo, J., Valero-Gonzalez, M. A., Garcia-Villanova, P., Olmedo-Sanchez, E., & Herrera, F. (2020). COVIDGR dataset and COVID-SDNet methodology for predicting COVID-19 based on chest X-ray images. IEEE Journal of Biomedical and Health Informatics, 24(12), 3595–3605. https://doi.org/10.1109/JBHI.2020.3037127
- Tahamtan, A., & Ardebili, A. (2020). Real-time RT-PCR in COVID-19 detection: Issues affecting the results. Expert Review of Molecular Diagnostics, 20(5), 453–454. https://doi.org/10.1080/14737159.2020.1757437
- Tajbakhsh, N., Shin, J. Y., Gurudu, S. R., Hurst, R. T., Kendall, C. B., Gotway, M. B., & Liang, J. (2016). Convolutional neural networks for medical image analysis: Full training or fine tuning? IEEE Transactions on Medical Imaging, 35(5), 1299–1312. https://doi.org/10.1109/TMI.2016.2535302
- Turkoglu, M. (2021). COVID-19 detection system using chest CT images and multiple kernels-extreme learning machine based on deep neural network. IRBM, 42(4), 207–214. https://doi.org/10.1016/j.irbm.2021.01.004
- Wang, X., Peng, Y., Lu, L., Lu, Z., Bagheri, M., & Summers, R. M. (2017). Chestx-ray8: Hospital-scale chest x-ray database and benchmarks on weakly-supervised classification and localization of common thorax diseases. In Proceedings of the IEEE conference on computer vision and pattern recognition, (pp. 2097–2106).
- Watson, J., Whiting, P. F., & Brush, J. E. (2020). Interpreting a covid-19 test result. BMJ (Clinical Research Ed.), 369, m1808. https://doi.org/10.1136/bmj.m1808
- Wikramaratna, P., Paton, R. S., Ghafari, M., & Lourenco, J. (2020). Estimating false-negative detection rate of SARS-CoV-2 by RT-PCR. MedRxiv.
- World Health Organization (WHO), https://www.who.int
- Worldometers. https://www.worldometers.info/coronavirus/