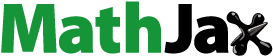
Abstract
The main activities in a remanufacturing system includes core acquisitions, remanufacturing operations, and remarketing. Core acquisitions are challenging for remanufacturers because remanufacturing in closed-loop supply chains is characterized by uncertainty in quality, timing and volume. The uncertainty is generally defined as incomplete or incomplete information. Sorting of quality incoming products in the remanufacturing system is a complex problem. On account of all quality conditions can be related either directly or indirectly, making it difficult to define certain conditions individually. Considering that the acquisition process involves many conflicting assessment criteria and subjective-qualitative considerations, an integrated method is needed to prioritize criteria selected. Therefore, this study makes a major contribution to research on multi-criteria sorting problem by developing a new hybrid method. This study focuses on development of a multi-criteria quality sorting model in the remanufacturing system based on a hybrid approach, namely Decision Making Trial Evaluation and Laboratory (DEMATEL) Analytical Network Process (ANP) and Grey Clustering. The DEMATEL approach is proposed to evaluate and to develop the dependency of criteria, and ANP method is utilized for weighting the criteria as well as Grey Clustering is proposed for modelling of uncertainty issues. The model was successful as it was able to identify the most important criteria which proposed. This work has found that maintenance history is the most important attribute in assessing the quality of incoming cores. This finding has important implication for selecting the best maintenance strategy of heavy-duty equipment during the use phase.
1. Introduction
Remanufacturing is a useful strategy for sustainable used product recovery which potential benefits on economy, environment, and society. It has been widely used in circular economy to improve resource loops by salvaging value of worn-out products. This was considered as the most unique product recovery since the significant value of discarded products is preserved by extending the product lifetime (Teixeira et al., Citation2022). The term “product” is generally understood to mean anything being supplied in order to meet customer needs and expectations (Prabowo et al., Citation2020). However, the remanufactured product must meet at least the same performance as the original product (as good as new) as a representation of the customer’s requirements.
Remanufacturing can be defined as a system for extending the lifecycle of worn-out products (also called core) back to use phase condition which is as good as new quality level. It encompasses core acquisitions, remanufacturing operations, and remarketing. It is possible for remanufactured product to have new quality level as a result more work content during remanufacturing operation, such as reconditioning and part upgrade. By contrast, remanufactured product performance differs from refurbishment in final quality level. Refurbishment is the process of restoring a used product to only operating condition quality level. In contrast to remanufacturing, however, no upgrade of parts was required in refurbishment. A well-known example of remanufacturing in heavy-duty equipment, notable and clearly distinguishable from refurbishment and other value retention processes type activities. The characteristics of heavy-duty equipment for remanufacturing occur effectively at the component level, due to more complex equipment (Parker et al., Citation2015). This is different from other sectors where remanufacturing occurs effectively at the system level of product. A vital aspect in heavy-duty equipment remanufacturing is how to classify the incoming core based on their quality level.
Many challenging issues in remanufacturing systems are not encountered in traditional manufacturing systems. The complexity and uncertainties related with the process of handling worn-out products may cause remanufacturing more challenging, due to required extensive decision-making practices, various techniques and methods have been developed (Rizova et al., Citation2020). The literature on remanufacturing system has highlighted several types of uncertain worn-out products input, namely: quality, timing, and volume (Goltsos et al., Citation2018; Mashhadi et al., Citation2015; Wei et al., Citation2015). These uncertainties are associated with incomplete information and variations.
The uncertain quality implies risk of remanufacturing cost as well as cost of quality. The highest quality variability of acquired cores and the possibility of non-remanufacturability of particular cores, necessitates prioritizing in core sorting processes (Gavidel & Rickli, Citation2015). Many factors were identified to be influencing quality uncertainty of incoming cores have been explored in several studies (Mustajib et al., Citation2019; Sakao & Sundin, Citation2018). In recent years, there has been an increasing interest in managing the uncertain quality in remanufacturing system. In the literature on quality acceptance criteria of incoming cores, the relative importance of the criteria is debated. Previous studies of sorting problem have not deal with dependency among the criteria (Mustajib et al., Citation2021). The impact of usage condition on physical condition is understudied, particularly for determining the quality grade of incoming cores. This indicates a need to understand the various judgment of independency that exist among the criteria (also called dimensions).
To better understand criteria interaction, Gölcük and Baykasoʇlu (Citation2016) grouped dependency into three broad types: structural dependency, causal dependency, and preferential dependency. The dominance and dependency relations in the structure of the criterion are referred to as structural dependency. This can be illustrated exactly by using Analytical Hierarchy Process (AHP) and Analytical Network Process (ANP) decision-making practices. ANP is a type of AHP that allows for more complicated interdependencies among criteria. Having been taken into account interdependencies (i.e., addition of feedback loops) in the model, a super-matrix can be generated (Hsueh & Lin, Citation2015). Saaty and Vargas (Citation2013) point outed that the ANP is a generalization of the AHP that considers both inner (among elements in a cluster) and outer (among elements in different clusters) relationships dependency. Meanwhile, the term causal dependency refers to cause and effect relationships. Decision Making Trial Evaluation and Laboratory (DEMATEL) is a good illustration of causal dependency. DEMATEL concerns with evaluating interdependent relationships among factors into an understandable structural model of the system, dividing it into a cause group and an effect group, and identifying the essential ones using a visual model (Si et al., Citation2018). Moreover, preferential dependency can be loosely described as preference orders of alternatives are changed when the level of criteria are adjusted.
This paper proposes a new methodology for multicriteria sorting problem of incoming cores and examines the dependency between the criteria. The purpose of this investigation is to explore the relationship among technological condition, physical condition and usage condition by using on DEMATEL. A Hybrid approach is utilised, by integrating DEMATEL, ANP and grey clustering method to establish the quality classes of incoming cores. There is an important difference between most study and this study. Previous study (Nguyen et al., Citation2020) in the field hybrid method of Grey DEMATEL and ANP have focused on proposing grey criteria or grey interactions in problem structuring to optimize evaluation and selection. In this study focus on obtaining final grey clustering results in assignment problem of objects. The DEMATEL method was applied to determine the network structure while taking into account the causal interrelationships. Then, ANP was employed in order to obtain weights of criteria. Finally, grey clustering was used to assign set of incoming cores into predefined classes. Thus, the primary research questions and research objectives of this study are presented in .
Table 1. Summary of research questions and research objectives
Therefore, this study makes a major contribution to research innovation on multi-criteria sorting problem in remanufacturing system under uncertain quality of incoming core by developing a new hybrid method. In this method the contribution is the use of DEMATEL to identify the relationships among quality criteria that should be included in the sorting problem. It allows Decision Maker (DM) to focus on those quality criteria that are important from operational point of view in remanufacturing system. The proposed approach specific for remanufacturing not for other form of value retention processes (refurbishment, repair), due to difference quality classified incoming core will be need difference treatments in remanufacturing operations.
The main sections addressed in this paper are divided into five sections. The first section deals with the context, background and/or importance of the topic of remanufacturing. This part presents an issue, problem, or controversy in the field of multi-criteria sorting problem of quality uncertainty in remanufacturing system. The second section presents literature review and the hybrid DEMATEL–ANP and grey clustering method adopted for the study. The third section provides the development of proposed model. The fourth section of this paper will examine the case study of sorting problem in heavy-duty equipment remanufacturer as numerical analysis of the proposed model as well as the findings of the research. The fifth section provides conclusion.
2. Literature review
In the literature, the term uncertainty has been used to refer to situations in which incomplete information. To better define the uncertainty, Y. Yang et al. (Citation2019) categorised this as being two different groups: subjective and objective. An interpretation, such as linguistic descriptions, are frequently tend to be subjective uncertainty. This type of uncertainty also called as epistemic uncertainty, which may be caused by imperfect information or imprecise data collection. The most prevalent examples of incomplete information are when data regarding system elements (parameters) is missing/unavailable (S. Liu et al., Citation2016a). This is because humans’ inability to correctly measure and model the physical environment (Akbari et al., Citation2020). Epistemic uncertainty is sometimes also called functional uncertainty, limited information or informative uncertainty (Y. Li et al., Citation2013). On the other hand, objective uncertainty or aleatory uncertainty is caused by inherent uncertainty or randomness of nature as the object itself, not by the description. Stochastic uncertainty and natural variability are another term for aleatory uncertainty (Y. Li et al., Citation2013). Dealing with various categories of uncertainties, many methods have been developed to handle these, such as (Liu et al., 2016a): probability statistics, fuzzy sets, rough sets and grey systems theory (GST). A classic example of a decision model under uncertainty is probabilistic model. This is exemplified in the work undertaken by Gavidel and Rickli (Citation2015). However, building probabilistic model of uncertainty requires a lot of data than GST based model. Researchers have recently made significant efforts in the field of uncertainty quantification to address issues where there is a lack of knowledge as limited data availability. By way of illustration, Liu et al. (Citation2016) recommended GST in modelling and data analysis. In view of the GST is a superior method for handling uncertainty that specializes at mathematical analysis of systems with uncertain data. It is illustrated in that with a limited amount of data, the grey system can generate possible outcomes.
In terms of main remanufacturing activities, there are three main activities in a remanufacturing system including: core acquisitions, remanufacturing operations, and remarketing. In the case of process of evaluating whether or not to remanufacture an end-of-life of products, also known as remanufacturability assessment, is a crucial stage of the remanufacturing activity, since it is difficult and has a high degree of uncertainty (Akano et al., Citation2021; Errington & Childe, Citation2013). Lack of incoming core data can lead to uncertainty during core acquisition. In addition, the quality attributes of remanufactured parts is difficult to express using statistical distribution, making remanufacturing quality control more challenging (Ma et al., Citation2018).
Zhang et al. (Citation2021) argued that many aspects must be addressed when evaluating the remanufacturing feasibility of incoming cores since the structural complexity of the worn-out products and the uncertainty of quality. Moreover, the incompleteness information and inherent variances in the quality of acquired worn-out products, commonly referred to as cores, are a major barrier for remanufacturing companies (X. X. Li et al., Citation2016). Therefore, quality uncertainty of incoming cores becomes a fundamental issue in remanufacturing systems. Sorting and inspection in reverse logistics system are essential activities to assess the quality condition of incoming cores as to their reusability or remanufacturability (Mahmoudi et al., Citation2014). However, these activities are labour-intensive and time-consuming may cause high labour cost (Guide & Wassenhove, Citation2001). These activities would have been more efficient if remanufacturer had used information and communication technology. The digitalisation in remanufacturing systems using 4.0 technology by implementing Internet of Things (IoT), Radio Frequency Identification (RFID), and cloud computing, as well as big data analytics have benefits to improve the capability to obtain actual conditions of used product for quality dependent acquisition (Kerin & Pham, Citation2019; Teixeira et al., Citation2022). Unfortunately, these approaches do not always guarantee applicable or relevant for many remanufacturing companies. Errington and Childe (Citation2013) emphasized that these methods are only powerful for those remanufacturer companies or original equipment manufacturing (OEM) who originally manufactured the products or having a control over its design, wishing to apply such technology would have to invest with a very long-term payback. For the most of remanufacturers, this seems to be prone to make automation unaffordable (Charter & Gray, Citation2007). As a result, remanufacturing frequently involves human judgment and evaluation.
A decision made at one remanufacturing activity will have a significant impact on decisions made at subsequent activities, which will have a significant impact on remanufacturing outcomes (Sitcharangsie et al., Citation2019). Mashhadi and Behdad (Citation2017) emphasized that quality grading and sorting policies play a key role to provide immediate solutions in remanufacturing systems to handle this source of uncertainty during cores acquisition. The term sorting problem has come to be used to refer to the assignment of each alternative to predetermined ordered classes (Alvarez et al., Citation2021). Different methods have been proposed to classify the quality of incoming cores.
Sorting problem may be defined as the branch of multicriteria decision making (MCDM) which studies the assignment of alternatives (observations/objects) into predefined homogenous groups/classes (Zopounidis & Doumpos, Citation2002). The multi-dimensional description of alternatives, which are evaluated in terms of non-homogenous and conflicting criteria is an essential feature of multi criteria sorting problems (Kadziński & Ciomek, Citation2021). The differences and limitations of previous studies on the subject sorting problem in remanufacturing compared to the current study are highlighted in .
Table 2. Comparison of previous studies for sorting problem with the proposed model
The problem of ranking the alternatives for implementing in sorting process of reverse logistics was studied by Hsueh and Lin (Citation2015). To obtain optimal alternatives for the sorting process, an ANP model was proposed by considering benefits, opportunities, costs, and risks (BOCR). To classify the extent of remanufacturing operational excellence’s sustainability, Golinska et al. (Citation2015) developed a decision-making tool based on grey system theory. It has been demonstrated that remanufacturers could be classified into three categories, each of which corresponded to a different level of sustainability. In a follow-up study, Golinska-dawson et al. (Citation2018) proposed mixed for the sustainability assessment of the remanufacturing process by applying grey decision making for small and medium sized remanufacturing company based on three levels of sustainability. Gavidel and Rickli (Citation2015) proposed a statistical model for sorting an extreme core arrivals under stochastic behaviour.
In the subsequent research using a grey clustering approach, Xin (Citation2016) evaluated the remanufacturability of used parts the automotive components. It has been revealed that the proposed indexes can be used to do effective evaluations. To enhance decision making in remanufacturing operation, Mashhadi and Behdad (Citation2017) proposed a new sorting approach by incorporating product life cycle information based on both internal and external factors, such as future component reusability, product identity data, and product health status, as well as market trends. Meanwhile, Shu San (Citation2019) used DEMATEL to assess the remanufacturability of mobile phones among the factors: innovation rate, residence time, product residual value, and obsolescence. To provide a systematic sorting and production planning for remanufacturing of used clothing, Lin et al. (Citation2020) developed a multi-objective programming model for minimizing the cost of remanufacturing cost and maximizing the recycle rate to reduce environmental impact. In recent study, Saiz et al. (Citation2021) investigated an ensemble learning-based for automated inspection as well as classification system for the automotive part remanufacturing. In a study conducted by Mishra et al. (Citation2021), it was shown that by enabling an integrative coefficient correlation maximisation approach (CCMA) and whitenization weight functions (WWF) clustering model are able to solve the problem of classifying core based on quality factors evaluating circularity at the product level.
In all the studies reviewed here, decision makings in sorting problem are recognised as crucial issue in remanufacturing systems. On account of sorting the incoming cores prior to remanufacture is an essential element of the remanufacturing system, removing inappropriate cores as soon as possible is able to prevent wasteful processing. Unfortunately, few researchers have addressed the complex problem of multi-criteria sorting for the uncertain quality of incoming cores. Recently, an integrated approach by coupling AHP, entropy and grey clustering to obtain efficient procedure of multi-criteria sorting problem have been developed by Mustajib et al. (Citation2021). The considered quality criteria for sorting model of incoming cores were as follows: technological, physical, as well as usage conditions. In the model, grey possibility function was used to handle the epistemic uncertainty in quality criteria of incoming core due to unavailability or incompleteness of information. However, this model was based on the AHP’s independence assumption, ignored the interdependence and feedback among criteria as well as sub criteria of the system. In view of a hierarchical structure of AHP, there is no feedback from a lower to a higher level in a linear top-down interaction, while the network structure of ANP is made up of many elements and groupings of elements that are simultaneously interconnected (Ishizaka, Citation2019). The study of quality sorting problem would have more useful if the interrelationship among quality criteria was explored. In the present study we proposed to employ the DEMATEL to address the interactions and feedback among the quality criteria of for sorting incoming cores. Within ANP, DEMATEL technique were used to construct a network model. These analytical procedures and the results obtained from them are described in the next section.
3. Methodology
As far as sorting is concerned, sorting problems are extremely related to MCDM in terms of handling complex problems deal with uncertainty, conflicting criteria, working with lot of alternatives and considering multiple dimensions. The fundamental instrument of scientific research to solve complex problems has been analysis, which breaks down a problem into its basic parts to analyse their behaviour (Saaty & Vargas, Citation2013). AHP is one of the most well-known method for the problem structuring, as well as most common procedures for determining weights in multicriteria decision making problem. Its goal is to assist decision-makers in making an effective and consistent decisions. A major advantage of AHP is that allowing for a hierarchical structure of the criteria and sub-criteria in weighting. However, there are certain weakness in some circumstances with the use of AHP. The main disadvantage of AHP is that unable to handle interdependency among the criteria in the complex problem. Moreover, AHP not able to assign the incoming core into predetermined quality classes in sorting problem. To overcome these problems, ANP was proposed in this work for its ability to deal with dependencies within a criterion (inner dependencies) and across criteria (outer dependencies). In addition, ANP was adopted to obtain the weights in the interdependency among the criteria of sorting problem on incoming cores.
Moreover, the ANP weights were used in grey clustering method to classify the incoming cores into definable quality classes using grey possibility functions. Grey clustering is defined by S. Liu et al. (Citation2016) as a technique constructed for sorting observation objects into specified classes using grey incidence matrices or grey possibility functions. Several factors are known to play a role in determining quality level of worn-out product. To evaluate incoming core quality currently available quality attributes based on previous study have been proposed by Mustajib et al. (Citation2021), the list of the derived quality attributes as well as citations is presented in .
Table 3. Evaluation criteria for incoming core
Here, we refer quality dimension as the main criteria to assess core condition. While, the quality attributes are sub criteria of quality dimension in which refers to fundamental both quantitative or qualitative measures of the core.
The main quality dimensions are based on:
Technological condition can be loosely described as the dimension of core quality, which is concerned with the used product’s technology characteristics, such as: obsolescence, upgradability, multiple life-cycle, and disassemblability.
Physical condition, refers to the dimension of core quality, which deals with end of life product conditions attributed by; damage level, completeness of components, traceability of identity or ease of identification, dimensional and geometric tolerance.
Usage condition, may be regarded with the dimension of core quality deals with intensity of the use environment and the user’s behaviour in utilizing a product, which consists of three attributes: frequency of use, or operating condition, maintenance history, and remaining useful of life.
It can be seen from conceptual network diagram of proposed model, the relationships of network include:
The causal relationships among core quality dimensions: technological condition, physical condition and usage condition to explore how these core quality dimensions relate with one another.
The causal relationships among core quality attributes within each core quality dimensions to understand the relationships among the attributes in each quality dimension: how they relate and impact in which attributes affects another.
Weighting the core quality attributes in the quality sorting of incoming core determine which core quality attributes is the most important toward each core quality dimensions.
In this work, we propose structural network model based-on ANP. The proposed model involves three quality dimensions and eleven quality attributes which were adopted from Mustajib et al. (Citation2021). Prior to develop ANP, DEMATEL method was employed to acquire criteria structure by incorporating cause and effect relationships.
The proposed hybrid method is described in . The first stage in this method is problem structuring to identify relevant quality attributes in the sorting problem of incoming cores, as well as to establish relationships between the attributes. The problem structuring plays an important role in the proposed method to indicate the concepts associated with hierarchies or networks, which allow to represent the relationships among problem elements.
The problem structuring stage results in attributes grouping and structurally networking the decision-makers’ aims. Problem structure is defined as the relationships between criteria in a decision problem. DEMATEL is particularly useful in identifying the causal relationships and the the judgments on the correlations among elements by structural modeling methods, therefore suitable for studying essential factors in complex system in remanufacturing cases (Ahmed et al., Citation2016; Bhatia & Srivastava, Citation2018; Deng et al., Citation2015; Shu San, Citation2019; Singhal et al., Citation2018). The DEMATEL method is used to develop the relationships between quality attributes to build a network relationship map (NRM). The procedure of DEMATEL can be summarized in the following steps:
Gathering experts’ judgment and generating the average matrix.
Based on the proposed quality attributes, a questionnaire was developed to obtain expert judgments in order to compare the relative importance degrees and to finalize the essential attributes in quality sorting of incoming cores. The experts were asked to respond questions using a 5-point Likert scale ranging from 0 if “no influence”; 1 if “very low influence”; 2 if “low influence”; 3 if “high influence”; 4 if “very high influence”. The goal of this questionnaire was to eliminate the proposed attributes with low ratings and no impact on quality sorting criteria/quality attributes of incoming cores. For this reason, the single direct influence matrix presented by the k-th expert. These evaluations form an n × n matrix for each expert’s judgment where the
is the score given by the k-th expert associated with quality attribute cp has influence on quality attribute cq. To incorporate all judgments from K experts, the direct-relation matrix
is calculated using EquationEq. (1)
(1)
(1) by averaging each expert’s scores.
(2) Calculating the normalized direct relation matrix (D)
The normalized direct-relation matrix can be acquired by normalizing matrix Z. The matrix was normalised using EquationEq. (2)
(2)
(2) and (Equation3
(3)
(3) ) as follows
The value of each element in matrix D falls between 0 and 1. All elements in the matrix D are complying with and at least one p such that
(3) Deriving total relation matrix
By using the normalized direct relation matrix (D), the total-relation matrix T can be obtained by using EquationEq. (4(4)
(4) ), in which I denotes the identity matrix, where
p,q = 1, 2, …,n.
when
The equation represents summation both of the direct effects and the indirect effects. The total relation matrix (T) is presented in .
(4) Determining the dispatcher and receiver group.
By determining the sums of rows and columns of matrix T it can be obtained dispatcher and receiver groups. The dispatcher (D) is calculated from the D-R which have positive values and higher influence on one another. They are assumed to indicate higher priority and are called dispatcher groups (D), where R is the sum of columns and D is the sum of rows in matrix T. Calculate the sums of rows r and columns c of the total relationship matrix T. The vector r and c can be obtained as follows:
where symbol′ express a transposed matrix. Moving on now to consider rp be the sum of the p-th row in matrix T. Further, rp express total direct and indirect effects that the quality attribute p has given to other quality attributes. Turning now to cq defines the sum of q-th column in matrix T. Moreover, cq represents the total effects, both direct and indirect received by a quality attribute q given by other quality attributes. With regard to p=q, then the term (rp + cp) express a degree of importance of the quality attribute, and the term (rp – cp) represents the net effect that the quality attribute contributes to the system in relation to other quality attributes. If the term (rp – cp) is positive, the quality attribute p is net causer (Dispatcher), and if the previous representation is negative quality attribute p is a net receiver (Receiver).
(5) Set up a threshold value
Subsequently, to construct the network relationship map (NRM), set a threshold value θ and obtain NRM. On the basis of expert opinion is determined the threshold value θ, which filters out negligible or small effects in the matrix T.
(6) Obtaining the network relationship map (NRM)
Table 4. Average direct relation matrix
Table 5. Normalized direct relation matrix (D)
Finally, NRM can be obtained by mapping all coordinate sets of to visualize the complex interrelationship and provide information to judge which are the most important quality attributes and how influence affected quality attributes.
Having NRM obtained, ANP method was employed in the evaluation stage for weighting by determining the quality clustering weights of each quality attributes. Based on pairwise comparisons, the ANP evaluates the influence between criteria. In general, the ANP procedure can be summarized in four steps as follows:
(1) Converting a problem into a topic network structure & constructing a network model.
At this step, the quality criteria of sorting problem incoming core can be transformed into a network model. This network structure can be obtained through previous NRM of DEMATEL method.
(2) Formulating a pairwise comparison matrix and determining the eigen vectors of interdependencies.
The quality attributes in each group of quality dimension are pair-wisely compared. The groups are also compared based on their significance and influence on achieving objectives, as well as interdependencies between each group’s quality dimensions. In ANP concepts, the effect of quality dimensions on each other can be presented using the eigenvector. Experts were asked to respond the relative importance of the quality attributes using a 9-point Saaty scale ranging from 1 where is “equally important” to 9 which express “extremely more important”. Geometric mean approximation of the judgment of all experts is calculated from the matrix to reduce sum of errors in priorities derivation. In this step, the internal importance vector, which express the relative importance of groups of quality dimension, is presented using EquationEq. (7(7)
(7) )
where A is the pairwise comparison matrix of the quality dimension, w is the eigenvector and λmax is the largest eigenvalue. If A presents a consistency matrix, then the eigenvector X can be calculated using the following equation.
Where I is an identity matrix; the consistency index (CI) and consistency ratio (CR) are used to check the consistency of the pairwise comparison matrix. The CI and CR values can be determined as:
where “n” is the number of quality attributes and “RI” express the average consistency index for numerous random entries of same order reciprocal matrices. If CR ≤ 0.1, then the pairwise comparison matrix is consistent; otherwise, decision makers would be asked to reconsider the pairwise comparisons in case of 0.10.
(3) Generating super-matrixes and the limit matrix.
To obtain the global priorities of the system with interdependent influences, the local priority vectors are entered in the relevant columns of a matrix, commonly referred to as a super-matrix. Let us consider that k = 1, 2,.,K corresponds to set of group of quality dimension () which is each group including n quality attributes:
. These elements of quality attributes can be applied to develop a supermatrix in Equationeq. (11
(11)
(11) ), and pair-wisely be compared using Saaty’ scale.
(4) Determining weights and the limit
The weighted super-matrix is determined by multiplication of the un-weighted super-matrix values in the group matrix. The limit super-matrix has the same structure as the weighted super-matrix, however the limit super-matrix has the same columns as the weighted super-matrix. The final priority of all elements in the matrix can be derived by normalizing each block of this super-matrix.
The last stage of decision problem in sorting using hybrid method is to assign the considered incoming cores into predefined homogeneous classes of quality. To avoid inaccuracies in results, the data are captured under a grey environment. The grey possibility functions were used in this stage to represent the epistemic uncertainty in the quality evaluation. To capture epistemic uncertainty, we treat the Xsr. as imperfect information/data or imprecise variables, where s = 1, 2,.S corresponds to set of incoming cores (a), and r = 1, 2,.R corresponds to quality attributes (c), respectively. Meanwhile, the quality classes are denoted by j = 1, 2,. J. As it is shown in that to estimate the imprecise variables, grey clustering uses a whitenization weight function to define the grey number’s preference for the possible values it could take. The standard whitenization weight function is a continuous function with defined starting and ending points that rises on the left and declines on the right (Liu & Lin, Citation2010).
4. Results and discussion
4.1. Results
In order to validate the effectiveness of the proposed approach, the multi-criteria sorting problem of the heavy-duty equipment component is taken as a case study. The growing market of heavy-duty equipment has been utilized by business players in the heavy-duty remanufacturer specializes in construction and mining equipment, such as: hydraulic excavator, dumb truck, and bulldozer, the supporting parts are various types of hydraulics cylinders (Simatupang, Citation2013). Remanufacturing components of heavy equipment to be done, due to the basic materials for components were imported by the country and sustainability reasons in manufacturing.
As was explained in the previous section, the following stages of the proposed method were undertaken for this study. In order to report the results and to discuss the finding, this section are divided into three parts based on the general stages of the proposed method as follows:
5. Problem structuring
The development of interrelationship among criteria of the quality conditions of incoming cores can be obtained by examining the conceptual framework of network model using DEMATEL. First of all, the results of the DEMATEL average matrix derived by averaging the responses data gathered from 19 expert judgements containing the pairwise comparisons are presented in . After that, by calculating the normalized direct relation matrix (D) in , the total-relation matrix T in can be obtained by using EquationEq. (4)(4)
(4) . Moreover, based on the adopted threshold value θ, it can be filtered minor effects in matrix T, based on which it will be obtained NRM, as shown on , which facilitates understanding of the relationships in the considered system. The sum of the values of matrix T was calculated and multiplied by the number of quality attributes squared. We considered relations with a threshold θ greater than 0.41 when creating the NRM. These relations can be seen in in bold. The threshold value is 0.41, which is derived by averaging all of the entries in matrix T.
Table 6. Total relation matrix (T)
Table 7. The significant relationship in total relation matrix of DEMATEL
provides the NRM, and the relationships that were plotted on the graph were those previously highlighted in bold in . It is apparent from the that quality attributes; 4 (disassemblability), 1 (obsolescence), and (7) traceability of identity are not affected by the others. As shown in this figure, the major importance of the eight quality attributes can be prioritized as 3 > 10 > 5 > 9 > 2 > 11 > 8 > 6 based on (D + R) values in the x axis, where R (Receiver) is the column sum and D (Dispatcher) is the row sum of matrix T (please see formulas 4, 5, 6). As it is shown in the NRM that multiple lifecycles is the most important quality attribute, while dimensional and geometrical tolerance is the least important quality attribute.
On the other hand, in terms of the significant relationship of eight quality attributes, it can be seen from the network relationship map in that based on (D − R) values the critical quality attributes of incoming core are generally categorised into two types: the dispatcher, also known as causes, and the receiver or effects. Our study found four major causes as dispatcher. These are: maintenance history, frequency of use, damage level, and completeness of components. In contrast, a number of quality attributes were identified to be four major effects (receiver), which are: multiple lifecycles, upgradeability, remaining useful life, dimensional and geometrical tolerance.
Having all the significance of quality attributes and the relationships have been identified, it will now move on to the next process to evaluate their importance through the ANP model to be combined with the Grey Clustering method by applied in a numerical experiment. The input of ANP model is based on the output of the DEMATEL process. The definition of the relationship network between criteria can be obtained from significant total relation as shown in . It is shown in that each colour represents the different dispatcher, for example: for every orange-coloured lines represent the dispatcher line from the multiple lifecycle criteria, which means multiple lifecycle criteria will affect the other criteria represented by the connected orange coloured line to the receiver criteria. The coloured rectangular boxes represent the inner dependence within criteria. For example: every orange colour represents the dispatcher from the multiple lifecycle criteria. It shows when the multiple lifecycle rectangular box is also orange means there is inner dependence within the multiple lifecycle criteria itself based on the DEMATEL result.
6. Evaluation
In order to evaluate the relative weights for the quality dimensions and the quality attributes of incoming cores, we examined the network relationship map (NRM) using ANP model. Moreover, the conceptual model of the ANP decision network is then can be built on Super Decision software as shown in . Therefore, assessment using pairwise comparisons were obtained by the Super Decision. The second questionnaire was designed to measure the weights in ANP to explain the importance of criteria. The total number of responses for this question was three experts. These experts consist of middle management of heavy-duty remanufacturer companies. Further analysis showed that all respondents’ answers are declared consistent based on consistency ratio (CR). The next step is to accommodate all of the respondents’ answers into a single value using the geometric mean.
All of the data were exactly and completely obtained from expert’s judgment based on ANP, therefore the weighting was successful. As a result, the final ANP weights computation was performed using Super Decision Software as detailed in . Specifically, shows that the current study found that past maintenance history of incoming core is the most important criteria for quality sorting.
Table 8. ANP final weight results
7. Assignment
To illustrate the effectiveness and applicability of the assignment process using grey clustering procedure, a numerical study for sorting incoming cores on sorting is presented here. First of all, in an attempt to exemplify the use of grey clustering in the assignment stage in accordance with previous study used whitentization weight function on the r-th quality attribute with the j-th class. To express the epistemic uncertainty, we use Xsr as imprecise variables for s-th incoming core and r-th quality attribute. below illustrates numerical example for sorting incoming cores based on the observed value in line with Mustajib et al. (Citation2021) with some adjustments. Furthermore, the grey fixed weight clustering coefficient (σs) can be calculated as follows:
When they arrived at a remanufacturing facility, the incoming cores were graded in terms of three quality classes (j = 3). This system of classification is useful because it can be categorised into best quality class, middle quality class and worst quality class. It has been reported that consumer will receive (Toromont-Reman, Citation2021): a full credit from remanufacturer for best quality with less remanufacturing processes needed, a partial credit middle quality with more remanufacturing processes needed, and no core refund for worst quality to be scraped or recycling.
After that, the clustering weight vector is calculated with the weight coefficient for each class ( for the s-th core:
Based on the calculation of the clustering weight vector for each s-th core (as), then the clustering coefficient matrix can be obtained for incoming core (set, S) incoming core (aS):
The last step is assigning the s-th core into the appropriate class based on the clustering coefficient matrix. Thus, the s-th core belongs to j* class if:
Generally speaking, shows the summary computations for grey fixed weight cluster coefficient for each class ). What can be clearly seen in the is the dominance of assignment of incoming cores in the best quality class. In summary, these results show that the proposed hybrid model can be applied to classify the quality condition of incoming cores in remanufacturing systems.
Table 9. The observed value of each quality attributes on each incoming core s (Xsr) based on Mustajib et al. (Citation2021
) and weights after ANP
Table 10. Maximum grey fixed weight cluster coefficient for each class for cores assignment
7.1. Discusions
Strong evidence of significance was found when the results were obtained based on the judgment of 12 experts of DEMATEL, when eight quality attributes are identified as critical in the quality sorting of incoming cores, which are listed in . Further analysis based on DEMATEL showed that there was significant interdependency among the quality attributes. The most surprising attributes of the data is that three attributes were removed because the insignificant impact of all quality attributes: obsolescence, disassemblability, and traceability of identity.
With respect to the insignificant relationship of three quality attributes, it is important to discuss as follows. First, obsolescence is not to be basic issue in remanufacturing of heavy-duty equipment. One reason why obsolescence is not to be fundamental quality issue for remanufacturing of heavy-duty equipment is that heavy-duty equipment are durable products with long life cycles. The obsolescence may have been an important factor in remanufacturing for short life-cycle product with rapid technology innovation. In the literature, short life-cycle product has been associated with rapid obsolescence as the emergence of new technology innovation. This is exemplified in the case study of remanufactured electronic product undertaken by Shu San (Citation2019). Second, disassemblability is minor importance aspect for determining the quality incoming core of heavy-duty equipment. On account of the availability of recent disassembly technology and workforce skills can always cope with the complexities of disassembling the cores can lead to only minor problem disassemblability. The effectiveness of the various disassembly techniques have been exemplified in a report by Duflou et al. (Citation2008). Third, traceability of identity plays only a minor role as quality attribute in multicriteria sorting problem of incoming core. The quality criteria of incoming core appeared to be unaffected by traceability of identity. It seems possible that this result is due to there is no need tracking system used to trace the identity information of incoming core.
The findings on NRM suggest that heavy-duty remanufacturer should pay much attention to four quality attributes causes rather than quality attributes receivers. In view of maintenance history, frequency of use, damage level, and completeness of components are important driving factors of multiple lifecycles, upgradeability, remaining useful life, dimensional and geometrical tolerance.
It is interesting to note that in this study that past maintenance history of incoming core is the most important criteria for quality sorting. The results based on ANP weights of this study indicate that maintenance history is the most important quality attribute in assessing the quality of incoming cores based on ANP weights. It is respectively followed by upgradability as the second, and multiple life-cycle as the third important should be considered, while frequency of use is the least important quality attribute. Due to it is believed that high quality level of incoming core resulting from proper maintenance strategies during the usage phase of product. Previous maintenance history of the used product’s is a significant criterion for estimating the remaining lifetime and deciding whether or not to reuse a component (Diallo et al., Citation2017). This results are not similar to that of previous research (Mustajib et al., Citation2021) who found multiple life-cycle as the most important criteria in assessing the quality of incoming cores based on AHP weights. This difference could have affected by the weighting in hierarchical model and network model.
Another important finding was that the vital aspect of upgradability in quality attribute of incoming core. Upgrade operation is associated with quality improvement actions that could be contributed to rejuvenate them for subsequent lifecycles. The results of this study also indicate that multiple life-cycle is an important contributing factor in sorting process. In view of sustainable product design with multiple life-cycle characteristic can result in sustainability for long term to improve resource loops by salvaging value of worn-out products.
In the final stage of the sorting model, incoming cores were successful assigned into grey classes showed the dominance of assignment of incoming cores into the best quality class. Overall, these results indicate that a multi-criteria quality sorting model based on DEMATEL and Analytical Network Process (ANP) combined with grey clustering using a possibility function can be applied to evaluate incoming cores in remanufacturing systems under uncertain quality condition.
In summary, this study strengthens the idea that interactions and feedback among the quality criteria for sorting incoming cores. These results corroborate the ideas of Tzeng and Shen (Citation2017), who suggested that new hybrid MCDM approaches which are needed to overcome unrealistic assumptions of complex problem. The hybrid MCDM can be used to recommend for improving performance the crucial criteria in addition to sorting. Strategies to enhance this hybrid MCDM might involve a decision support system. It is possible that the system will improve in the long term.
8. Conclusions
The aim of the present research was to examine interrelationship among criteria of the quality conditions of incoming cores, and to obtain the relative weights for the criteria and sub-criteria, as well as to provide assignment model on sorting of incoming core under incomplete information.
The most obvious finding to emerge from this study is that eight quality attributes are identified as critical in the quality sorting of incoming cores. These may be divided into two groups: the causes, and the effects. Four major causes are maintenance history, frequency of use, damage level, completeness of components. On the other hand, a number of quality attributes were identified to be four major effects are multiple-lifecycle, upgradeability, remaining useful life, dimensional and geometric tolerance.
Moreover, this study has identified that maintenance frequency is the most important criteria in assessing the quality of incoming cores based on ANP weights. It is respectively followed by upgradability as the second, and multiple life-cycle as the third important should be considered in quality sorting of incoming cores. The study has found that generally that a multi-criteria quality sorting model based on DEMATEL and Analytical Network Process (ANP) combined with grey clustering using a possibility function can be applied to evaluate the quality condition of incoming cores in remanufacturing systems.
The present study has confirmed the findings of Mustajib et al. (Citation2021) which found that technological, physical, as well as usage conditions are important quality factor in core acceptance criteria. These findings of case study may be somewhat limited by type of included remanufactured product. More in detail, study with more focus on short life-cycle product is therefore suggested. Further research is also needed to develop decision support system for multi-criteria sorting problem based on DEMATEL and Analytical Network Process (ANP) combined with grey clustering to overcome quality uncertainty in core acquisition. To develop of a decision support system of multi-criteria sorting problem on uncertain quality of incoming cores, additional development will be needed that a database containing relevant data for the situation is part of the data management subsystem.
Disclosure statement
No potential conflict of interest was reported by the author(s).
Additional information
Funding
References
- Ahmed, S., Ahmed, S., Shumon, M. R. H., Quader, M. A., Cho, H. M., & Mahmud, M. I. (2016). Prioritizing strategies for sustainable end-of-life vehicle management using combinatorial multi-criteria decision making method. International Journal of Fuzzy Systems, 18(3), 448–29. https://doi.org/10.1007/s40815-015-0061-0
- Akano, D. I., Ijomah, W., & Windmill, J. (2021). Stakeholder considerations in remanufacturability decision-making: Findings from a systematic literature review. Journal of Cleaner Production, 298, 126709. https://doi.org/10.1016/j.jclepro.2021.126709
- Akbari, N., Jones, D., Arabikhan, F., Jones, D., & Arabikhan, F. (2020). Goal programming models with interval coefficients for the sustainable selection of marine renewable energy projects in the UK. European Journal of Operational Research, 293(2), 748–760. https://doi.org/10.1016/j.ejor.2020.12.038. .
- Alvarez, P. A., Ishizaka, A., & Martínez, L. (2021). Multiple-criteria decision-making sorting methods: A survey. Expert Systems with Applications, 183(March), 115368. https://doi.org/10.1016/j.eswa.2021.115368
- Amezquita, T., Hammond, R., Saiazar, M., & Bras, B. (1995). Characterizing the remanufacturability of engineering systems. Proceedings of the ASME Design Engineering Technical Conference, 1(1993), 271–278. https://doi.org/10.1115/DETC1995-0036
- Badurdeen, F., Aydin, R., & Brown, A. (2018). A multiple lifecycle-based approach to sustainable product configuration design.pdf. Journal Of Cleaner Production, 200, 756–769. https://doi.org/10.1016/j.jclepro.2018.07.317
- Barquet, A. P., Rozenfeld, H., & Forcellini, F. A. (2013). An integrated approach to remanufacturing: Model of a remanufacturing system. Journal of Remanufacturing, 3(1), 1–11. https://doi.org/10.1186/2210-4690-3-1
- Bensmain, Y., Dahane, M., Bennekrouf, M., & Sari, Z. (2018). Optimal preventive remanufacturing planning of production equipment under operational and imperfect maintenance constraints: A hybrid genetic algorithm based approach. Reliability Engineering & System Safety, 185, 546–566. https://doi.org/10.1016/j.ress.2018.09.001
- Bhatia, M. S., & Srivastava, R. K. (2018). Analysis of external barriers to remanufacturing using grey-DEMATEL approach: An Indian perspective. Resources, Conservation and Recycling, 136(December 2017), 79–87. https://doi.org/10.1016/j.resconrec.2018.03.021
- Chakraborty, K., Mondal, S., & Mukherjee, K. (2017). Analysis of product design characteristics for remanufacturing using fuzzy AHP and axiomatic design. Journal of Engineering Design, 28(5), 338–368. https://doi.org/10.1080/09544828.2017.1316014
- Charter, M., & Gray, C. (2007). Remanufacturing and product design. Designing for the 7th Generation, 6(3/4), 375–392. https://doi.org/10.1504/IJPD.2008.020406
- Deng, Q., Liu, X., & Liao, H. (2015). Identifying critical factors in the eco-efficiency of remanufacturing based on the fuzzy DEMATEL method. Sustainability, 7(11), 15527–15547. https://doi.org/10.3390/su71115527
- Diallo, C., Venkatadri, U., Khatab, A., & Bhakthavatchalam, S. (2017). State of the art review of quality, reliability and maintenance issues in closed-loop supply chains with remanufacturing. International Journal of Production Research, 55(5), 1277–1296. https://doi.org/10.1080/00207543.2016.1200152
- Duflou, J. R., Seliger, G., Kara, S., Umeda, Y., Ometto, A., & Willems, B. (2008). Efficiency and feasibility of product disassembly: A case-based study. CIRP Annals - Manufacturing Technology, 57(2), 583–600. https://doi.org/10.1016/j.cirp.2008.09.009
- Dulman, M. T., & Gupta, S. M. (2018). Maintenance and remanufacturing strategy: Using sensors to predict the status of wind turbines. Journal of Remanufacturing, 8(3), 131–152. https://doi.org/10.1007/s13243-018-0050-1
- Errington, M., & Childe, S. J. (2013). A business process model of inspection in remanufacturing. Journal of Remanufacturing, 3(1), 1–22. https://doi.org/10.1186/2210-4690-3-7
- Gao, Y., Feng, Y., Wang, Q., Zheng, H., & Tan, J. (2018). A multi-objective decision making approach for dealing with uncertainty in EOL product recovery. Journal of Cleaner Production, 204, 712–725. https://doi.org/10.1016/j.jclepro.2018.09.080
- Gavidel, S. Z., & Rickli, J. L. (2015). Triage as a core sorting strategy in extreme core arrival scenarios. Journal of Remanufacturing, 5(1). https://doi.org/10.1186/s13243-015-0020-9
- Gavidel, S. Z., & Rickli, J. L. (2017). Quality assessment of used-products under uncertain age and usage conditions. International Journal of Production Research, 55(23), 7153–7167. https://doi.org/10.1080/00207543.2017.1349954
- Go, T. F., Wahab, D. A., & Hishamuddin, H. (2015). Multiple generation life-cycles for product sustainability : The way forward. Journal of Cleaner Production, 95, 16–29. https://doi.org/10.1016/j.jclepro.2015.02.065
- Gölcük, I., & Baykasoʇlu, A. (2016). An analysis of DEMATEL approaches for criteria interaction handling within ANP. Expert Systems with Applications, 46, 346–366. https://doi.org/10.1016/j.eswa.2015.10.041.
- Golinska, P., Kosacka, M., Mierzwiak, R., & Werner-Lewandowska, K. (2015). Grey Decision Making as a tool for the classification of the sustainability level of remanufacturing companies. Journal of Cleaner Production, 105, 28–40. https://doi.org/10.1016/j.jclepro.2014.11.040
- Golinska-dawson, P., Kosacka, M., Mierzwiak, R., & Werner-lewandowska, K. (2018). The mixed method for sustainability assessment of remanufacturing process using grey decision making. In P. Golinska & D. F. Kübler (Eds.), Sustainability in remanufacturing operations (pp. 125–139). Springer. https://doi.org/10.1007/978-3-319-60355-1
- Goltsos, T. E., Ponte, B., Wang, S., Liu, Y., Naim, M. M., & Syntetos, A. A. (2018). The boomerang returns? Accounting for the impact of uncertainties on the dynamics of remanufacturing systems. International Journal of Production Research, 1–34. https://doi.org/10.1080/00207543.2018.1510191
- Guide, V. D. R., & Wassenhove, L. N. (2001). Managing product returns for remanufacturing. Production and Operations Management, 10(2), 142–155. https://doi.org/10.1111/j.1937-5956.2001.tb00075.x
- Hsueh, J. T., & Lin, C. Y. (2015). Constructing a network model to rank the optimal strategy for implementing the sorting process in reverse logistics: Case study of photovoltaic industry. Clean Technologies and Environmental Policy, 17(1), 155–174. https://doi.org/10.1007/s10098-014-0770-3
- Ishizaka, A. (2019). Analytic hierarchy process and its. In New perspectives in multiple criteria decision making (1st ed., pp. 81–93). https://doi.org/10.1007/978-3-030-11482-4: Springer International Publishing.
- Jiang, Z., Wang, H., Zhang, H., Mendis, G., & Sutherland, J. W. (2019). Value recovery options portfolio optimization for remanufacturing end of life product. Journal of Cleaner Production, 210, 419–431. https://doi.org/10.1016/j.jclepro.2018.10.316
- Kadziński, M., & Ciomek, K. (2021). Active learning strategies for interactive elicitation of assignment examples for threshold-based multiple criteria sorting. European Journal of Operational Research, 293(2), 658–680. https://doi.org/10.1016/j.ejor.2020.12.055
- Kerin, M., & Pham, D. T. (2019). A review of emerging industry 4 . 0 technologies in remanufacturing. Journal of Cleaner Production, 237, 117805. https://doi.org/10.1016/j.jclepro.2019.117805.
- Kosacka, M. (2018). Sustainability in remanufacturing operations. In K. F. G.-D. P (Ed.), Sustainability in remanufacturing operations (pp. 25–45). Springer. https://doi.org/10.1007/978-3-319-60355-1
- Li, Y., Chen, J., & Feng, L. (2013). Dealing with uncertainty: A survey of theories and practices. IEEE Transactions on Knowledge and Data Engineering, 25(11), 2463–2482. https://doi.org/10.1109/TKDE.2012.179
- Li, X., Li, Y., & Cai, X. (2016). On core sorting in RMTS and RMTO systems: A newsvendor framework. Decision Sciences, 47(1), 60–93. https://doi.org/10.1111/deci.12152
- Lin, C. R., Chen, M. T., Tseng, M., Jantarakolica, T., & Xu, H. (2020). Multi-objective production programming to systematic sorting and remanufacturing in second-hand clothing recycling industry. Symmetry, 12(1161), 1–22. https://doi.org/10.3390/sym12071161
- Liu, S., & Lin, Y. (2010). Grey systems: Theory and applications. Springer-Verlag.
- Liu, M., Liu, C., & Zhu, Q. (2014). Optional classification for reassembly methods with different precision remanufactured parts. Assembly Automation, 34(4), 315–322. https://doi.org/10.1108/AA-03-2014-023
- Liu, S., Yang, Y., & Forrest, J. (2016). Grey data analysis: methods, models and applications. Springer-Verlag. https://doi.org/10.1007/978-981-10-1841-1
- Ma, J., Kremer, G. E. O., & Ray, C. D. (2018). A comprehensive end-of-life strategy decision making approach to handle uncertainty in the product design stage. Research in Engineering Design, 29(3), 469–487. https://doi.org/10.1007/s00163-017-0277-0.
- Ma, J., Wang, Q., & Zhao, Z. (2018). RLS-based quality control method for reassembly under uncertainty. Production Engineering, 12(3–4), 481–490. https://doi.org/10.1007/s11740-018-0826-z
- Mahmoudi, H., Fazlollahtabar, H., & Zhou, Z. (2014). An integer linear programming for a comprehensive reverse supply chain. Cogent Engineering, 1(939440), 1–14. https://doi.org/10.1080/23311916.2014.939440
- Mashhadi, A. R., Esmaeilian, B., & Behdad, S. (2015). Uncertainty management in remanufacturing decisions: A consideration of uncertainties in market demand, quantity, and quality of returns. ASCE-ASME Journal of Risk and Uncertainty in Engineering Systems Part B: Mechanical Engineering, 1(4 Suppl 1), 129–132. https://doi.org/10.1115/1.4029759
- Mashhadi, A. R., & Behdad, S. (2017). Optimal sorting policies in remanufacturing systems: Application of product life-cycle data in quality grading and end-of-use recovery. Journal of Manufacturing Systems, 43, 15–24. https://doi.org/10.1016/j.jmsy.2017.02.006
- McManus, H., & Hastings, D. (2005). A framework for understanding uncertainty and its mitigation and exploitation in complex systems. INCOSE international symposium, 1–20.
- Mishra, A., Verma, P., & Tiwari, M. K. (2021). A circularity-based quality assessment tool to classify the core for recovery businesses. International Journal of Production Research, 1–19. https://doi.org/10.1080/00207543.2021.1973135
- Morse, E., Dantan, J. Y., Anwer, N., Söderberg, R., Moroni, G., Qureshi, A., Jiang, X., & Mathieu, L. (2018). Tolerancing: Managing uncertainty from conceptual design to final product. CIRP Annals, 67(2), 695–717. https://doi.org/10.1016/j.cirp.2018.05.009
- Mustajib, M. I., Ciptomulyono, U., & Kurniati, N. (2019). Determining factors of quality uncertainty and its control analysis in remanufacturing system. International Journal of Applied Science Engineering, 16(1), 35–45. https://doi.org/10.6703/IJASE.201906
- Mustajib, M. I., Ciptomulyono, U., & Kurniati, N. A novel multi-criteria sorting model based on ahp-entropy grey clustering for dealing with uncertain incoming core quality in remanufacturing systems. (2021). Applied Sciences, 11(6), 2731. Applied Sciences (Switzerland), 11(6. https://doi.org/10.3390/app11062731
- Nguyen, P. H., Tsai, J. F., Nguyen, H. P., Nguyen, V. T., & Dao, T. K. (2020). Assessing the unemployment problem using A grey MCDM model under COVID-19 impacts: A case analysis from Vietnam. Journal of Asian Finance, Economics and Business, 7(12), 53–62. https://doi.org/10.13106/JAFEB.2020.VOL7.NO12.053.
- Parker, D., Riley, K., Robinson, S., Symington, H., Tewson, J., Jansson, K., Ramkumar, S., & Peck, D. (2015, October). Remanufacturing market study. European Remanufacturing Network. EC--09404ERNWP2.2.docx
- Prabowo, R., Singgih, M. L., Karningsih, P. D., & Widodo, E. (2020). New product development from inactive problem perspective in Indonesian SMEs to open innovation. Journal of Open Innovation: Technology, Market, and Complexity, 6(1), 20. https://doi.org/10.3390/JOITMC6010020
- Rizova, M. I., Wong, T. C., & Ijomah, W. (2020).November 2019 A systematic review of decision-making in remanufacturing. Computers & Industrial Engineering, 147, 106681. https://doi.org/10.1016/j.cie.2020.106681
- Saaty, T. L., & Vargas, L. G. (2013). Decision making with the analytic network process: economic, political, social and technological applications with benefits, opportunities, costs and risks (2nd ed.). Springer.
- Saiz, F. A., Alfaro, G., & Barandiaran, I. (2021). An inspection and classification system for automotive component remanufacturing industry based on ensemble learning. Information, 12(489), 489. https://doi.org/10.3390/info12120489
- Sakao, T., & Sundin, E. (2018). How to improve remanufacturing?—A systematic analysis of practices and theories. Journal of Manufacturing Science and Engineering, 141(2), 021004. https://doi.org/10.1115/1.4041746
- Sharma, V., Garg, S. K., & Sharma, P. B. (2015). Remanufacturing process: The case of heavy equipment support services. International Journal of Services and Operations Management, 22(1), 40. https://doi.org/10.1504/IJSOM.2015.070882
- Shi, J., Li, T., & Liu, Z. (2015). A three-dimensional method for evaluating the remanufacturability of used engines. International Journal of Sustainable Manufacturing, 3(4), 363. https://doi.org/10.1504/IJSM.2015.073838
- Shu San, G. (2019). Analyzing remanufacturability of mobile phones using DEMATEL approach. Jurnal Teknik Industri, 21(1), 33–42. https://doi.org/10.9744/jti.21.1.33-42
- Si, S. L., You, X. Y., Liu, H. C., & Zhang, P. (2018). DEMATEL technique: A systematic review of the state-of-the-art literature on methodologies and applications. Mathematical Problems in Engineering, 2018, 1–33. https://doi.org/10.1155/2018/3696457. 2018(1.
- Simatupang, T. M. (2013). Heavy Equipment Supply Chain in Indonesia. In Logistics and supply chain in Indonesia : Emerging practices (1st ed.). School of Busisness and Management, Institut Teknologi Bandung 44 .
- Singhal, D., Tripathy, S., & Kumar Jena, S. (2018). DEMATEL approach for analyzing the critical factors in remanufacturing process. Materials Today: Proceedings, 5(9), 18568–18573. https://doi.org/10.1016/j.matpr.2018.06.200
- Sitcharangsie, S., Ijomah, W., & Wong, T. C. (2019). Decision makings in key remanufacturing activities to optimise remanufacturing outcomes: A review. Journal of Cleaner Production, 232, 1465–1481. https://doi.org/10.1016/j.jclepro.2019.05.204
- Suhariyanto, T. T., Wahab, D. A., & Rahman, M. N. A. (2017). Multi-Life Cycle Assessment for sustainable products: A systematic review. Journal of Cleaner Production, 165 1 , 677–696. https://doi.org/10.1016/j.jclepro.2017.07.123
- Teixeira, E. L. S., Tjahjono, B., Julião, J., & Julião, J. (2022). Demystifying the digital transition of remanufacturing: A systematic review of literature. Computers in Industry Journal, 134(103567), 1–14. https://doi.org/10.1016/j.compind.2021.103567
- Toromont-Reman, Core Acceptance Criteria. (2021). In Toromont Reman. Retrieved February 12, 2021, from https://www.toromontcat.com/docs/default-source/reman/customer-version-core-handbook.pdf
- Töyrylä, I. (1999). Realising the potential of traceability - A case study research on usage and impacts of product traceability. Acta Polytechnica Scandinavica Mathematics and Computing Series, 1999 211.
- Tzeng, G.-H., & Shen, K.-Y. (2017). New concepts and trends of hybrid multiple criteria decision making (1st ed.). CRC Press.
- Wang, H., Jiang, Z., Zhang, H., Wang, Y., Yang, Y., & Li, Y. (2019). An integrated MCDM approach considering demands-matching for reverse logistics. Journal of Cleaner Production, 208(3), 199–210. https://doi.org/10.1016/j.jclepro.2018.10.131
- Wei, S., Tang, O., & Sundin, E. (2015). Core (product) Acquisition Management for remanufacturing: A review. Journal of Remanufacturing, 5(4), 1–27. https://doi.org/10.1186/s13243-015-0014-7
- Xin, J. (2016). Evaluation of auto parts remanufacturing by grey cluster model. Grey Systems: Theory and Applications, 6(3), 296–308. https://doi.org/10.1108/GS-08-2016-0022
- Xu, F., Liu, X., Chen, W., Zhou, C., & Cao, B. (2018). An ontology and AHP based quality evaluation approach for reuse parts of end-of-life construction machinery. Mathematical Problems in Engineering 2018. https://doi.org/10.1155/2018/3481030.
- Yang, S. S., Nasr, N., Ong, S. K., & Nee, A. Y. C. (2017). Designing automotive products for remanufacturing from material selection perspective. Journal of Cleaner Production, 153 1 , 570–579. https://doi.org/10.1016/j.jclepro.2015.08.121
- Yang, Y., Liu, S., & Xie, N. (2019). Uncertainty and grey data analytics data analytics. Marine Economics and Management, 2(2), 73–86. https://doi.org/10.1108/MAEM-080209-0006.
- Zhang, X., Zhang, H., Jiang, Z., & Wang, Y. (2016). A decision-making approach for end-of-life strategies selection of used parts. International Journal of Advanced Manufacturing Technology, 87(5–8), 1457–1464. https://doi.org/10.1007/s00170-013-5234-0
- Zhang, L., Zhao, X., Ke, Q., Dong, W., & Zhong, Y. (2021). Disassembly line balancing optimization method for high efficiency and low carbon emission. International Journal of Precision Engineering and Manufacturing - Green Technology, 8(1), 233–247. https://doi.org/10.1007/s40684-019-00140-2
- Zhang, X., Tang, Y., Zhang, H., Jiang, Z., & Cai, W. (2021). Remanufacturability evaluation of end-of-life products considering technology, economy and environment: A review. Science of the Total Environment, 764(16), 142922. https://doi.org/10.1016/j.scitotenv.2020.142922
- Zhou, J., Huang, P., Zhu, Y., & Deng, J. (2012). A quality evaluation model of reuse parts and its management system development for end-of-life wheel loaders. Journal of Cleaner Production, 35(November), 239–249. https://doi.org/10.1016/j.jclepro.2012.05.037
- Zopounidis, C., & Doumpos, M. (2002). Multicriteria classification and sorting methods : A literature review. European Journal of Operational Research, 138(2), 229–246. https://doi.org/10.1016/S0377-2217(01)00243-0