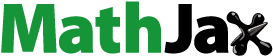
Abstract
The financial technology (fintech) industry received much attention recently. This study is the first to propose an innovative extended approach based on the Combined Compromise Solution (CoCoSo) and Interval-Valued Intuitionistic Fuzzy Set (IVIFS) models (IVIF-CoCoSo) to rank and evaluate sustainable fintech success factors in the Vietnamese fintech sector. Five success factors are collected from the existing literature to evaluate fintech firms. Using the IVIF-CoCoSo approach to rank, model, and evaluate the success factors (based on experts’ opinions) in a sustainable financial technology business, the results indicate that the agility, security, and innovative success factors are the most significant in the Vietnamese fintech sector. The study findings, and comparisons made between the results of the proposed method and other Multi-Criteria Decision Making (MCDM) methods, confirm the accuracy of the proposed method in solving the fintech success factors evaluation problem.
PUBLIC INTEREST STATEMENT
The financial services industry has converged around technology-enabled innovations, and many factors have paved the way for new entrants to challenge the conventional business models of financial services with their radically innovative and creative business models. Using a quantitative approach, this study evaluated sustainable fintech success indicators. The integration of qualitative and quantitative studies will yield results that would the impact of the key success factors on achieving sustainable business development.
2. Introduction
The financial services industry reached a new transformational pace, primarily converging around technology-enabled innovations. Factors, such as the proliferation of advanced technological innovations, an increasing focus on societal demand, data availability, a supportive regulatory environment, sufficient investor capital, and financial education paved the way for new entrants to challenge the conventional business models of financial services with their radically innovative and creative business models (Gomber et al., Citation2017). Though fintech as a concept is relatively new, its emergence and affirmation started a revolution that has already significantly impacted the financial services industry. The Economist (Gomber et al., Citation2018) notes,
The magical combination of geeks in T-Shirts and venture capitalists that has disrupted other industries has put financial services in their sights. From payments to wealth management, from peer-to-peer lending to crowdfunding, a new generation of start-ups is taking aim at the heart of the industry – and a pot of the revenues that Goldman Sachs estimates is worth $4.7 trillion dollars.
Fintech refers to companies that provide or facilitate financial services by using information technology. Currently, fintech is characterised by technology companies that disintermediate formal financial institutions and provide direct products and services to end users, often through online and mobile channels (World Economic Forum, Citation2015). Fintech is here to stay, supported by the widely emerging technologies, such as artificial intelligence (AI), blockchain, smart contracts, and machine learning, among others. Looking at precedents from other disrupted industries, such as the music and mobile phone industries, a technological revolution is undeniably underway within the financial services industry (Riemer & Johnston, Citation2019). The financial services industry is historically the most resistant to technological disruptions, thus resulting in defensive economics. The last significant disruption was the dotcom boom, which provided further evidence of the incumbents’ resilience (Miklos et al., Citation2015). This changed as fintech has become the poster child that continues to grab headlines.
The focus of fintech startups is shifting from the USA to Asia. The USA has traditionally been the destination of choice for scaleups, given its sizable market, wealth of talent, access to capital, and political stability. Today, those factors are equally, if not more so, available in Europe and Southeast Asia. The average age of fintech startups at exit in Southeast Asia is relatively lower than in Europe and the USA (Dealroom.co, Citation2017). Fintech is Southeast Asia’s (SEA) largest venture capital investment category by number of backed startups. The rise of fintech is accelerated by venture capital investment. In 2020, $1.6 billion was invested in the region, compared with only $0.2 billion five years ago (Dealroom.co, Citation2020). SEA is among the fastest growing regions for fintech in the world. (Hootsuite Inc, Citation2020) Vietnam’s digital economy hit $12 billion in 2019, and it is anticipated to record rapid growth in the coming years to reach a figure of $43 billion by 2025. In Vietnam, the biggest contributors in the internet economy are the e-Commerce and ride-hailing sectors. Particularly, homegrown marketplaces like Sendo and Tik, which compete with the likes of Lazada and Shopee, are significant contributors to the boom in digital payments. (Bain & Company, Citation2019) In six SEA countries, there are 570 million people and 360 million internet users. Every year, 22 million people enter the mobile age. As many as 50% of SEA consumers are unbanked, while 70% are either underbanked or unbanked. Today cash is still the primary means of payment. In 2019, 70% of Small and Medium Enterprise (SME) merchants accept only cash (Dealroom, Citation2020). In Vietnam, 90% of Vietnamese consumers opt to pay cash on delivery for their online purchases, a much higher proportion than in other regional markets. However, digital payment technology is evolving rapidly. Over the past five years, payments through mobile banking services in Vietnam surged by a whopping 144% annually (Tellimer Insights, Citation2020) driven by a fast growing, engaged, young, and mobile-first audience engaged in the booming internet economy. According to investors, the fintech sector is particularly appealing for the following reasons: (Wataru & Hoang, Citation2021)
Vietnam has one of the region’s highest mobile phone penetration rates at around 80% of the adult population, but a relatively low number of bank branches per capita. (UOB, PwC, SAF, Citation2021)
Regulators have shown support for fintech by issuing e-wallet licences to dozens of companies. This combination has created a fertile ground for start-ups looking to deliver financial services via smartphones. Vietnam’s market has had exponential growth, from a mere 39 firms in 2015, the size of Vietnam’s fintech industry grew dramatically to 112 in 2017, 169 in 2019, and approximately 188 by September 2021 (UOB, PwC, SAF, Citation2021). The total number of transactions via the interbank electronic payment system increased by 3.32 percent in volume and 41.37 percent in value compared to the same period in 2020. Over the same period, internet payments increased by 54.13 percent in volume and 30.70 percent in value. In the first nine months of 2021, Vietnam-based fintech companies attracted US $388 million in funding, ranking third in the industry (Wataru & Hoang, Citation2021).
Recently, many researchers have explored the critical success factors of fintech (Jinasena et al., Citation2020) using various statistical and mathematical approaches, including Data Envelopment Analysis (DEA; Yang, Citation2016) and econometric regression (Organization for Economic Co-operation and Development, Citation2020; Sonnenschein & Mundaca, Citation2016). Although prior studies used methods, such as Multiple Criteria Decision-Making (MCDM) and fuzzy sets theory, to evaluate fintech performance and indicators (Zeng et al., Citation2020; Zrobek et al., Citation2020), no study so far combines these approaches. This study is the first to take advantage of the fuzzy sets theory and MCDM methods to develop a novel hybrid approach to evaluate the sustainable success factors of the fintech sector.
The above discussion reflects the current significance of identifying success factors in the fintech sector. The factors identified and classified in this study will help fill a gap in knowledge by increasing the explanatory power of critical success factors for fintech companies. Nevertheless, there is room for further research to further test the critical success factors identified here and potentially contribute additional ones. Qualitative research on customers and fintech practitioners would be a particularly valuable contribution of this study. The LASIC principle (Lee & Teo, Citation2015) defines five important attributes of business models that can successfully harness financial technology to create a sustainable social business for financial inclusion. This study presents comprehensive indicators that can measure success in the fintech sector, and the results include useful suggestions and information for both governmental agencies and companies. The five critical success factors identified, with some variation in their degree of explanatory power, are low margin, agility, security, innovative, and compliance easy.
From a theoretical perspective, this study identifies the critical success factors for fintech companies by testing and increasing the explanatory power of Bernardo’s (Citation2017) framework of success factors, and extending it to cover the fintech sector. Consequently, this study aims to identify, rank, and evaluate the significant indicators of success in the fintech sector by integrating compromise decision-making algorithms and fuzzy sets theory. Accordingly, this study develops a hybrid method based on Interval-Valued Intuitionistic Fuzzy Sets (IVIFS) and Combined Compromise Solutions (CoCoSo) called IVIF-CoCoSo. Thus, the majority of MCDM approaches are extended in a way to be applicable to fuzzy environments. In some instances, fuzzy numbers may be still inadequate because of the natural vagueness of subjective decisions made by decision experts (DEs) or the vagueness of the complicated socio-economic situations. As decision-making problems turn out to be more complex, recognizing a distinctive alternative becomes more challenging for DEs. Combining these methods is expected to yield a robust model to evaluate and prioritise sustainable fintech success indicators. Atanassov and Gargov (Citation1989) proposed the IVIFS approach based on the notion of Intuitionistic Fuzzy Sets (IFS), to suggest an intuitive way to solve uncertain and ambiguous properties. Yazdani et al. (Citation2019) recently proposed the CoCoSo method under an integrated, simple additive weighting, and exponentially weighted product model, as a compendium of compromise solutions. The CoCoSo approach combines the compromise decision-making approach with aggregation strategies. A distance measure is considered here, which originates from the grey relational coefficient and is targeted at making the obtained results more flexible. Therefore, the weight of the alternatives is incorporated into the decision making process using three equations. Finally, an aggregated multiplication rule is used to release the ranking of the alternatives and conclude the decision-making process.
Recently, many studies have applied IVIFS in different areas as it offers several advantages. IVIFS has a greater degree of freedom and more advantages over other fuzzy set methods, and conventional IFS can deal with information that covers fuzziness and uncertainty in MCDM problems. This advantage reduces information loss. Additionally, IVIFS can handle imprecise and uncertain information in different application areas. As IVIFS can effectively solve decision-making problems with uncertainty and fuzziness, some previous studies adopted this approach to estimate success factors for sustainable development (Y. Xu & Zhang, Citation2019). However, few studies have aimed to integrate the CoCoSo and IVIFS approaches to evaluate and examine critical success factors. Particularly, the current literature includes no studies that apply the proposed hybrid method to evaluate the Vietnamese fintech sector. Therefore, this study is the first to apply the CoCoSo and IVIFS methods in developing a novel approach to rank and evaluate success factors to establish a sustainable fintech business. Thus, this study aims to achieve the following objectives:
To develop a IVIF-CoCoSo as a novel approach to identify, rank, and evaluate success factors to achieve sustainable development in the fintech sector.
To develop novel IVIF-similarity and divergence measures to compute the relative significance of decision-making setting, where DEs express their individual performance evaluation and criteria weights.
To apply five indicators from the existing literature using the IVIF-CoCoSo approach to rank, model, and evaluate the success factors in the Vietnamese fintech sector based on experts’ opinions.
3. Preliminaries
3.1. Success factors in a sustainable fintech business
In recent years, an increasing number of fintech startups have been founded in Vietnam. However, some are growing faster than others, and in general, fintech start-ups around the world are thriving (Warner, Citation2017). Fintech has revolutionized the finance industry by utilizing the most recent technological advancements to increase the capital markets and financial service providers’ efficiency (Lee & Teo, Citation2015). Consequently, fintech introduces a new business model that reduces expenses and boosts profit margins. To be profitable and sustainable, businesses will require creative applications and commercial expansion through the provision of financial services. At this juncture in the transition to fintech’s new era, financial institutions vigorously seek ways to enhance their services and products through the application of technology (Lee & Teo, Citation2015).
Numerous innovative technologies have been utilized by fintech firms. Most developing countries rely heavily on start-ups, which have been highlighted as the barometer of innovation and success in the financial sector (Duc Dang Thi Viet et al., Citation2022). Across a broad range of industries, investors, regulators, customers, and merchants place a high premium on identifying the success elements of a financially sustainable technology business (Gomber et al., Citation2017). To be profitable and sustainable, businesses will need to expand their operations by offering new fintech services. An estimated 38% of the global population does not have a formal bank account, and another 40% is underserved by banks, giving a vast market opportunity for financial institutions (Lee & Teo, Citation2015). Numerous fintech start-ups are seeking new routes to successful business models, the creation of enhanced consumer experiences, and methods that result in the transformation of services (Gomber et al., Citation2018).
3.2. A brief review of IVIFS
Atanassov and Gargov (Citation1989) pioneered the IVIFS concept as an extension of IFS to tackle uncertainty (Z. Xu & Cai, Citation2012), which is illustrated by the degrees of belongingness and non-belongingness in interval form:
3.2.1. Definition 1 (Atanassov & Gargov, Citation1989)
Let be a universe of discourse, then an IVIFS E over
is defined by:
where satisfies
. The intervals
and
denote the degrees of membership and non-membership of the element
in the set
, respectively.
For ease, suppose and
. Then,
where and
. The interval
denotes the degree of hesitancy (
) to
.
As per Definition 1, an IVIFS is categorised by the interval-valued membership and non-membership functions and articulated as a pair. For , the pair
is termed the IVIF number
. To simplify, an IVIF number (IVIFN) is typically represented as
, where
and
3.2.2. Definition 2 (Atanassov & Gargov, Citation1989)
If , then some operations can be described as below:
if
and
if
and
;
;
3.2.3. Definition 3 (Z. S. Xu & Chen, Citation2008)
Consider be an IVIFN. Then,
are the score and accuracy functions of , respectively, such that
and
. It is observed that the existing functions given in EquationEquation (2)
(2)
(2) are invalid to rank the alternatives, thus making it incapable of distinguishing the IVIFNs in terms of preference ordering. Consequently, further attention is required here to discover the precise score function to evaluate an optimal alternative from the set of feasible options. To do this, Fu et al. (Citation2018) suggested using an improved score function by considering the degree of hesitation between the IVIFNs’ belongingness and non-belongingness functions.
3.2.4. Definition 4 (Fu et al., Citation2018)
Let be an IVIFN. Then,
This is called the improved score function. Evidently, .
3.2.5. Definition 5 (Z. S. Xu & Chen, Citation2008)
Consider to be an IVIFN and
to be an arbitrary real number. Then,
Considering the idea of IVIFNs, EquationEquation (4)(4)
(4) is extended as follows:
Let be the set of IVIFNs, where
. Then, the IVIF-weighted arithmetic operator (IVIFWA) is
Similarly, the IVIF-weighted geometric operator (IVIFWG) is
3.2.6. Definition 6 (Mishra & Rani, Citation2018)
The IVIF-decision matrix is normalised based on the relation
where and
are the benefit and cost attributes, respectively, and
.
Similarity measures are a significant tool to measure the degree of resemblance between elements by comparing the information captured by the IVIFSs.
3.2.7. Definition 7 (Z. S. Xu & Chen, Citation2008)
A mapping is said to be a similarity measure for IVIFS(D), if
satisfies the following requirements:
(S1)
(S2)
(S3)
(S4). For all, E, F, G,
This method makes a key contribution to the calculation of the discrimination degree as this is first time that the concept of divergence is applied to IFSs. Later, Montes et al. (Citation2015) considered the new axiomatic definition offered for the IF-divergence measurement.
Definition 8 (Montes et al., Citation2015):
A mapping (D)
can be a divergence measure for IVIFSs if it satisfies the following axioms:
(D1).
(D2). if and only if
;
(D3). for every
(D4). for every
.
3.3. IVIF-similarity and divergence measures
The IF-similarity measure is a significant method of describing the degree of resemblance between elements (Mishra & Rani, Citation2018; Z. S. Xu & Chen, Citation2008). Based on the IVIFSs’ novelty in practical application, different IVIF-similarity measures were studied by multiple authors from different perspectives and applied to a variety of disciplines, including medical diagnosis, decision making, and image processing problems. Z. S. Xu and Chen (Citation2008) generalised several formulae of IVIF-similarity measures from IFSs. Fu et al. (Citation2018) developed an IVIF-similarity measure and used it to evaluate MCDM and medical diagnosis problems. Pekala and Balicki (Citation2017) introduced inclusion and similarity measures by using the order of IVIFSs connected with lexicographical order, and further examined some interesting properties. Mishra and Rani (Citation2018) proposed a bi-parametric IVIF-similarity measure to determine the dominance degree of alternatives and applied the TODIM (Portuguese acronym for Interactive and Multi-Criteria Decision Making) method to evaluate the problem of plant location selection. Dhivya and Sridevi (Citation2019) introduced a new IVIF-similarity measure based on the mid values of transformed triangular fuzzy numbers (TFNs). Luo and Liang (Citation2018) introduced novel IVIF-similarity measures according to the transformed IVITFNs and implemented them to solve pattern recognition and medical problems. Mishra and Rani (Citation2018) developed IVIF-information measures based on the exponential functions and discussed the weights here. Based on the advantages and usefulness of the IF-similarity measures, we propose similarity measures for IVIFSs to support the proposed weights determining the method. Subsequently, the outcomes of the IVIF-similarity measures are discussed via mathematical proofs. Based on Song et al. (Citation2014), we extend the similarity measure for IVIFSs.
Theorem 1 Function is a valid similarity measure for IVIFSs (D).
Here, corresponding to Jain and Chhabra (Citation2016), the new IVIF-divergence measure is
The Aggregated Sum Product Assessment (WASPAS) approach was applied to uncertain correlative MCDM reservoir flood control management problems under IVIFSs. The divergence measure’s symmetric form is
To express the superiority of the developed IVIF-similarity measure, it is compared with the existing similarity measures. The comparison is employed according to the widely implemented counter-intuitive cases.
4. Proposed IVIF-CoCoSo method
In this section, we develop an extended CoCoSo approach to solve MCDM problems portrayed by the IVIFSs. The proposed method extends the Multiple Attribute Decision—Making (MADM) method in Yazdani et al. (Citation2019) to comprise multiple DEs and the hesitation degree of IVIFNs in the MCDM process. It is assumed that the evaluations of DEs with respect to alternatives for different criteria with criteria weights are determined in terms of IVIF-decision matrices. Additionally, the weights-related information of DEs, specified by the decision-makers, is articulated as IVIFNs. First, the DE’s numeric weights are computed based on the IVIF-divergence measure (Mishra et al., Citation2020), and thereafter, the individual relative importance. Subsequently, we implement the proposed IVIF-similarity measure to calculate optimal objective criteria weights. Further, the combined weights (objective and subjective weights) are applied to implement the extended IVIF-CoCoSo approach.
In the MCDM process, the prime objective is to choose the most suitable alternative from a discrete set of alternatives
,
under the criterion set
Let
be the set of DEs. The IVIF-decision matrix and the relative importance of criteria are established based on the preference judgements of DEs in linguistic terms (LTs). Then, the LTs are transformed into IVIFNs as shown in . The procedure of the novel IVIF-CoCoSo approach is as follows:
Table 1. The board of experts and their detail
Table 2. Fintech industry evaluation criteria
Table 3. Linguistic terms for assessing the alternatives
•Step 1. Construct the IVIF-decision matrix for a group of DEs. Construct a performance evaluation matrix
for each DE with respect to the criterion set.
•Step 2. Calculate the relative importance of the DE.
o Step 2.1. Construct each normalised IVIF-decision matrix that can be obtained by the DEs based on EquationEquation (7)(7)
(7) , as follows:
where is a normalised IVIF-decision matrix for each DE.
o Step 2.2. Compute the IVIF preference value (IVIFPV ) for each DE using the following relations:
where is the mean of the normalised IVIF-value matrix given by
o Step 2.3. Evaluate the IVIF overall preference value for the DEs corresponding to the deviation value
in IVIFPV as follows:
o Step 2.4. Construct the IVIFP selection matrix for the DEs; the minimum normalised value matrix
is
and
o Step 2.5. Calculate the relative importance of each DE as
•Step 3. Obtain the aggregated IVIF (AIVIF) decision matrix.
Let be the decision matrix for the
DE in the form of decision linguistic variables, which is then transformed into the IVIFN such that
. Subsequently, to aggregate all the individual equations and construct a single equation, we must establish an aggregated IVIF-decision matrix. To do this, let
, where
, is the aggregated IVIF-decision matrix and
, such that
•Step 4: Construct the normalised AIVIF-decision matrix.
Based on , the normalised AIVIF-decision matrix
is evaluated and given by
•Step 5. Compute the criteria weights.
Criteria weights are either considered to be completely known by the DEs, with a subjective assessment in terms of IVIFN; partly known (or partially uncertain) weights; or fully unknown weight information. These weights depend on the DE’s observations and a variety of other subjective aspects. Now, the combination of two varieties of criteria weight information is discussed as follows:
o Step 5.1. Subjective weight evaluation.
Here, we establish a subjective weight assessment matrix for the
expert, given by
where is an assessment weight of the criterion
to be assessed by the
expert,
. The subjective criterion weight is computed by
Let be the assessment weight of the
expert, where
is an IVIFN. Now, the aggregated subjective weight of the criteria is calculated as
, and by using EquationEquation (5)
(5)
(5) , we obtain:
where
is an IVIFN.
Next, corresponding to the obtained criteria weights , the average subjective weights
can be evaluated by the Definition
[EquationEquation (3)
(3)
(3) ].
o Step 5.2. Completely unknown weights: IVIF-similarity measure.
When the criteria weights are fully unknown, the IVIF-similarity measure weight method is applied to obtain the criteria weights. During the MCDM process, the degree of uncertainty of the judgment assessment by the DEs is likely to be as low as possible. Thus, the maximum is the minimum weight allotted to a criterion and vice versa. Hence, the objective criteria weights are evaluated by
Therefore, completely unknown criteria weights, deciphered through the similarity measure approach, are achieved in terms of crisp numbers as to be used in the further process.
o Step 5.3. Calculate the criteria aggregate weights through the subjective and objective assessment weights:
where is the coefficient aggregated weight mechanism and
.
•Step 6. Compute the weighted sum and power weight comparability sequences.
The weighted sum measure (WSM) for each alternative, that is, the sum of the weighted comparability sequence, is
The weighted product measure (WPM) for each alternative; that is, amount of the power weight of comparability sequence is computed by
•Step 7. Relative weights or balanced compromise scores of the alternatives.
Here, three appraisal score strategies are used to produce the relative weights of the alternatives, which are derived as follows:
where is the coefficient of the compromise decision mechanism, and
. Generally, we take
. It is interpreted that EquationEquation (28)
(28)
(28) expresses the arithmetic mean of the sums of the WSM and WPM scores, while EquationEquation (29)
(29)
(29) expresses a sum of the relative scores of WSM and WPM when compared to the best. EquationEquation (30)
(30)
(30) releases the balanced compromise of the WSM and WPM model scores.
•Step 8. Compute the final aggregation compromise index of the alternatives.
The final ranking index , to specify the importance of the alternative, is determined by (as more significant as better)
Finally, rank the alternatives through the increasing sorting of the aggregation compromise index .
•Step 9. End.
5. Case study: The Vietnamese Fintech industry
Fintech gained significant momentum and traction both in terms of the start-up ventures’ volume and funding levels. This rapidly accelerated the funding of fintech companies from US$17.0 billion in 2015 to US$40.6 billion in 2018 (PwC, Citation2019). Given this exponential growth among fintech companies, the knowledge gap in evaluating the industry success factors is of great interest and must be addressed. However, publications on fintech success are primarily derived from magazines and newspapers (Tucker, Citation2018). The scientific research on fintech focused primarily on categorising fintech and developing a consensual definition. Thus, the literature and research so far focused more on the descriptive study of fintech (Puschmann, Citation2017).
The questions of why and how some fintech companies are successful have merely been raised, providing limited empirical evidence about fintech success (Lee & Teo, Citation2015; Warner, Citation2017). In 2022, a comprehensive database of all fintech companies in Vietnam was established (Duc Dang Thi Viet et al., Citation2022). The study found that the financial services industry could be divided into six distinct categories and 12 sub-sectors. The study also investigated each company in the Vietnamese fintech sector to understand its unique business features. However, it left some questions unanswered, especially regarding which factors are important to develop a sustainable fintech business. Another question of great interest is the fintech sector’s development since then. The study laid the groundwork for a process of recognising essential factors when identifying future sustainable fintech businesses and explaining the Vietnamese fintech sector’s development. Thus, this study fundamentally aims to fill this gap in knowledge by conducting a literature review; collecting and analysing data to analyse the fintech sector’s development; and identifying, recognising, and classifying success factors for fintech companies.
Our study was launched at the end of 2021; at this stage of the proposed model, many round-table meetings had been organized with the board members. The selection criteria and decision options used in the study were determined with input from the board of experts. The board members were selected from a pool of highly qualified professionals, and several criteria were considered for this selection. Being a specialist in the field of the fintech industry was determined as the primary requirement by the researchers. The second requirement was having advanced level professional experience (i.e., working in the industry for at least 7 years as a senior executive or company owner) and the third requirement was being a member of the board of a professional association. With offers the first comprehensive and detailed examination of expert’s opinion over an extended period if needed.
The selected members of the board of experts are currently in determinative roles in FinTech services, and all of them are the executive board members of the professional association. Hence, their opinions and evaluations are sufficient to make a reasonable and realistic assessment. Additionally, including members with less expertise and knowledge than these top senior executive members may result in the inclusion of certain excessive and undesirable evaluations. This can affect the reliability of the evaluation process. The list of the members of the board of experts are given in below. The data used in the decision-making process were obtained from a team of managers. The team identified the criteria for the assessment process and accepted the criteria as proposed by Lee and Teo (Citation2015).
These experts were all participants in an in-person meeting; first, open-ended questions were directed to them and they were asked to prepare a list of the criteria and options essential in an assessment of fintech success factors. After these lists were collected, repetitive criteria and options were eliminated, and the final selection criteria and alternatives were obtained. These criteria and definitions are presented in .
When assessing the fintech industry, it is necessary to design a reliable evaluation system to ensure the effectiveness of the results (Sisinni et al., Citation2018). The evaluation criteria for fintech companies are constructed as . provides the descriptions of the five criteria. The integrated framework of the developed method is given in .
Figure 1. The framework of interval-valued intuitionistic fuzzy-combined compromise solution approach for fintech industry evaluation.
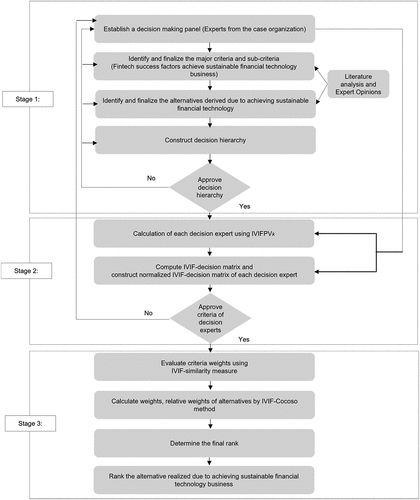
Suppose that there are ten fintech enterprises, A = {A1 (Axon Active), A2 (FPT Software), A3 (TPP Technology), A4 (IMT Solutions), A5 (MoMo), A6 (KilimoFinance), A7 (Nextpay), A8 (Zalopay), A9 (VayMuon), A10 (InnoTech)} to assess in terms of fintech success factors. After strict screening by fintech experts, the five criteria— (Low margin),
(Asset light),
(Security),
(Innovative), and
(Compliance easy)—best reflect the fintech enterprise solutions. According to the characteristics of fintech enterprise solutions, they are benefit criteria too. Additionally, the weight information
for the five criteria is given based on the knowledge and experience of financial experts. gives evaluations for fintech enterprise solutions in linguistic terms.
Subsequently, we employ the developed algorithm by the VC
software to obtain the overall aggregation and combined compromise ranking results of the alternatives.
Step 1: According to the experts’ knowledge and experience, the linguistic matrix is shown in , prepared using the evaluation scale in .
Steps 2–3: The IVIF-decision matrix (Z) about a group of DEs is constructed and the DEs’ relative importance is calculated.
Steps 4–5: The aggregated IVIF (AIVIF) decision matrix is obtained and the normalised AIVIF-decision matrix is constructed.
Steps 6–7: The criteria weights and weighted sums and power weight comparability sequences are computed.
Steps 8–9: The final aggregation compromise index and relative weights or balanced compromise scores of the alternatives are computed.
6. Results
The weights of the ten DEs regarding fintech’s critical factors are evaluated using the proposed modified IVIF preference selection approach based on the proposed IVIF-divergence measure. In this regard, the experts’ weights are calculated for IVIFPV , IVIF overall preference value
, IVIFP selection matrix
, and the minimum normalised value matrix
. The results obtained from the evaluation mentioned earlier are illustrated in .
Table 4. Computational results: IVIFPV and decision weights (DEs)
The IVIF-aggregated decision matrix for fintech-critical factors is created and represented in . Remember that all the criteria are of the benefit type; thus, the normalisation of the aggregated IVIF-decision matrix is not required. Hence,
.
Table 5. The criteria weights assessed by decision experts
Additionally, the weights of the assessment criteria are determined by the proposed IVIF-similarity measure method. Furthermore, the experts’ judgements and preferences for the evaluation criteria’s weights are applied. Finally, the subjective weight matrix is prepared. Using Equationequations (22)
(21)
(21) , (Equation23
(22)
(22) ), and (Equation24
(23)
(23) ), we calculate each criterion’s average subjective weight
.
Based on the weights defined by the DEs’ weights and EquationEquation (25)(25)
(25) , each criterion’s objective weight is evaluated below based on the proposed IVIF-similarity measure:
Using EquationEquation (26)(26)
(26) , the combined weight (wj) of each criterion (with aggregating weight mechanism coefficient
) is
Using DE weights and EquationEquations (27)(27)
(27) & (Equation29
(29)
(29) ), the WSM
and WPM
measures, and their score values—
and
—are determined for each fintech enterprise. Based on EquationEquations (29
(29)
(29) )—(Equation31
(31)
(31) ), the relative weights or balanced compromise scores
and
(with
) are computed using the compromise decision mechanism coefficient
of fintech enterprises, as given in .
Table 6. Overall aggregation and combined compromise ranking results of the alternative with
Further, the aggregation compromise index (with
) of the fintech enterprises is evaluated according to EquationEquation (32)
(32)
(32) and presented in . Finally, A5 is found to be the best firm alternative, while A10 is the worst choice.
Table 7. Degree of importance for criteria weights with aggregated weight mechanism coefficient for sensitivity analysis
6.1. The advantage of the proposed method
The proposed approach utilises a comparability sequence, after which the weights are aggregated using two mechanisms. One of them follows the multiplication rule, while the second describes the weighted power of the distance from the comparability sequence. To validate the preference order, we define three different measures (aggregation strategy) for a given alternative. Ultimately, the cumulative equation reports a ranking. There is no algorithm among the existing MCDM tools that supports this kind of aggregation. Each strategy would offer a ranking score, which would then be further improved by a complete ranking index. This procedure is based on a combination of compromise attitudes.
A modified preference selection index method has been proposed under an interval-valued intuitionistic fuzzy setting to determine the weights of the professional energy experts, resulting in more realistic individual measure degrees of DEs, unlike Xie et al. (Citation2021). The degree of hesitancy is considered independently significant in the complete implementation procedure, while the alternatives are ranked using the transaction degrees of all three parameters.
Evaluation of criteria weights is one of the major challenges in the decision-making procedure. In the proposed approach, the objective weights, as expressed by the DEs (calculated by the novel similarity measure and the subjective weights), are combined; the aggregated weights utilise the extended CoCoSo method.
6.2. Sensitivity analysis
Here, we present a sensitivity analysis for the sets of criterion weights and the different values of the aggregated weight mechanism coefficient . The values of
are varied to investigate the sensitivity of approach to the criteria’s degree of importance. Further, presents ten sets of degrees of the criteria’s significance over the different values of
, which are then implemented in the sensitivity analysis. We find that agility
has the highest degree of importance for
, while the security base
has the highest degree of importance at
.
7. Discussion
This study proposes a novel fuzzy MCDM method called IVIF-CoCoSo to rank and evaluate a model of success factors that help achieve a sustainable fintech business. A total of five success factors are collected from the existing literature to evaluate sustainable fintech. It is challenging to make effective decisions in uncertain conditions and a dynamic environment. It is even more complex to evaluate the success factors in the fintech sector because the evaluation process involves many different, potentially conflicting, criteria. A compromise between these criteria is required for the problem to be solved. To overcome challenges and problems like the one addressed in this study, the most efficient approach is the use of the fuzzy assessments articulated in linguistic terms.
This study evaluated sustainable fintech based on the comprehensive indicators of sustainable development in the fintech sector. According to the current fintech literature, five leading success factors were low margins, asset light, security, innovative, and compliance easy. The ranking of the indicators based on different values of the decision-making coefficient are shown in .
Figure 2. Caption: Ranking order of criteria with the aggregated weight mechanism coefficient for sensitivity analysis.
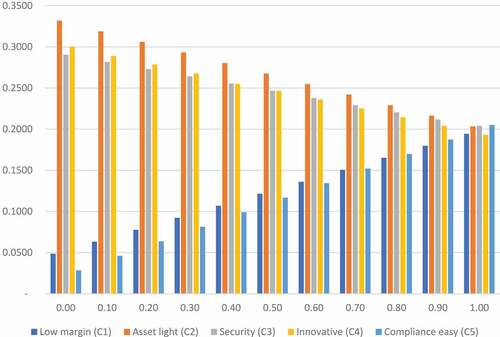
The results indicate that asset light (C2) is the most important part of sustainable fintech business development. Thus, it ranked first for ,
and second at
Additionally, in terms of the ranking of the company’s indicators, asset light is ranked first for company
. Moreover, in company
, innovative has the best rank among other indicators. A key factor for sustainability in the financial technology business is an asset-light model for operations and consumption and how it changes in terms of time, place, and across sectors.
developed their business through electronic applications; thus, with no need for physical branches or counters,
requires little physical infrastructure investment and off-line risk management. This complements both LASIC principles (Lee & Teo, Citation2015). Therefore, by keeping assets light: 1) margins can be kept low, and 2) businesses can scale easily without building physical branches.
The second rank for sustainability in a fintech business was related to the security (C3) base indicators for , while it ranked third for
,
, and ranked first for
. Further, in terms of the indicator rankings of companies, the security base indicators had the top rank in company
. The financial services sector handles sensitive information about individuals and enterprises. With the emergence of fintech, more data is now available in digital formats, which makes it easier to analyse and generate insights but also makes the data more susceptible to security breaches. According to PwC’s Global Fintech Survey 2016, almost 56% of the respondents identified information security and privacy as threats to the rise of fintech (PwC, Citation2016).
The third rank for sustainability in a fintech business was the innovative (C4) dimensions of the indicators for , while it ranked second from
. Further, in terms of the indicator rankings of companies, the innovative dimensions of the indicators of life quality had the top rank in company
. Technologies such as smart contracts, zero-knowledge proof, and distributed data storage and exchange, which are key to existing fintech innovations such as digital wallets, digital assets, decentralised finance (DeFi), and non-fungible tokens (NFT), will continue to drive disruptive business models in financial services (Dick et al., Citation2021).
The fourth rank for sustainability in a fintech business was assigned to the low margin (C1) context and characteristics for , while it ranked fifth for
,
Further, in terms of the indicator rankings of companies, low margin had the best rank in company
. Low profit margin is a key characteristic in the initial phase of a fintech business (Lee & Teo, Citation2015). Financial service providers should not look solely at current profitability numbers and fail to look at the big picture. Instead, we should think strategically by considering the long-term impact and growth potential of the various businesses within the ecosystem (Wiwanto, Citation2020). According to Accenture, even successful fintech companies take 8 to 14 years to become profitable. Many spend a significant amount of time ‘waiting in the lobby”, which implies there is a significant probability of the company going out of business. It is all centred around the idea of obtaining a large mass of users while attaining a high burn rate and high customer demand.
The fifth rank for sustainability in a fintech business was assigned to the compliance easy (C5) indicators for ,
, while it ranked fourth for
Further, in terms of the indicator rankings of companies, the compliance easy indicators had the top rank in company
. According to Deloitte (Citation2019), there is increasing pressure on FinTech firms to elevate their risk management capabilities, including the development of a responsive operational risk and compliance program. In response, many fintechs are working to achieve robust risk and compliance capabilities.
Furthermore, low margin (C1) for and the Compliance easy (C5) for
2 are low. Finally, the alternatives for
and
are in the top rank in terms of sustainability indicators for fintech businesses. The asset light (C2) and innovative (C4) of
, while the security (C3) base of
and the low margin (C1) of
are high. Therefore, both alternatives
and
retained the top rank. Low margin (C1) and Innovative (C4) for
Asset light (C2) for
and Compliance easy (C5) for
are low. Hence, alternatives
and
are ranked the worst under the sustainability indicators for a fintech business. Therefore, these are outperformed by the fintech indicators using the proposed IVIF-CoCoSo method.
8. Practical and managerial implication
The current paper proposes a novel and powerful mathematical tool for solving many decision-making problems. The proposed model is maximally consistent, and it is not affected by the rank reversal problem. Thus, it provides a more reliable and flexible evaluation environment for decision-makers. Moreover, it eliminates many structural problems and gaps in the existing literature. The FinTech firms have advantages that work in their favour during evaluation such as the success criteria, the innovation success factor followed by targeting the unbanked and good product quality. For incumbents, product quality and distribution are key factors. Keeping in mind these advantages, fintech service firms can consider a more reasonable success criteria alternative and improve sustainable fintech services while increasing profits and productivity and reducing risks and costs. In addition, practitioners can implement it easily without the need for advanced mathematical knowledge as it has a practical algorithm. It can be applied by practitioners to solve decision-making problems encountered in the fintech industry. Moreover, it can be useful for authors who perform future studies on this issue. In this study, the set of criteria were identified together with highly experienced experts of the related industry by following a methodological frame. These criteria are updated and compatible with real-life decision-making problems and requirements. Both practitioners and authors can utilise them to evaluate real-life decision-making problems encountered in the fintech industry.
9. Limitations, future research directions, and conclusions
This study introduces a novel approach based on CoCoSo and IVIFSs called IVIF-CoCoSo to rank and evaluate the indicators of fintech in the sustainable development of the Vietnamese fintech sector. A survey method was used to evaluate and rank the success criteria based on experts’ opinions and a literature review. In this regard, five indicators from the existing fintech evaluation literature are used. The data for evaluating sustainable fintech is collected from ten experts in the fintech field. The analysis results found that agility and security and were ranked first, followed by innovative, low margin, and compliance easy.
Despite the strengths of the study, some limitations can be opportunities for future research. First, this study evaluated the fintech success indicators for an emerging country. Thus, there may be a sampling bias. Future research could extend this method to the financial and non-financial sectors of other emerging and developed countries. Second, future studies could also evaluate fintech success indicators based on different performance measurements.
This study used a quantitative approach to evaluate sustainable fintech success indicators; therefore, the integration of qualitative and quantitative studies will make the study results better capable of clarifying the impact of the key success factors on achieving sustainable business development. Third, this study evaluates fintech indicators at the firm-level; therefore, future studies might also consider fintech evaluation at the country level. Fourth, this study used a limited number of experts for the process of criteria weighting; therefore, to further improve the research robustness, future studies can use more experts to evaluate and rank the success indicators. Fifth, this study developed the CoCoSo method based on the IVIF approach. Further studies can develop this method based on other fuzzy set environments such as IFSs, hesitant fuzzy linguistic term sets, hesitant fuzzy preference relations, granular fuzzy rough sets, type-2 and type-1 fuzzy sets, interval-valued hesitant fuzzy sets, interval type-2 fuzzy sets, interval type-2 polygonal fuzzy sets, etc. All these fuzzy sets could potentially be used in future studies.
Data Availability Statement (DAS)
The data that support the findings of this study are openly available in Mendeley Data at http://doi.org/10.17632/yr8wn5y8s4.1, reference number yr8wn5y8s4.1.
Acknowledgements
This work was supported by the Posts and Telecommunications Institute of Technology, Vietnam (https://www.ptit.edu.vn).
Disclosure statement
No potential conflict of interest was reported by the author(s).
Additional information
Funding
Notes on contributors
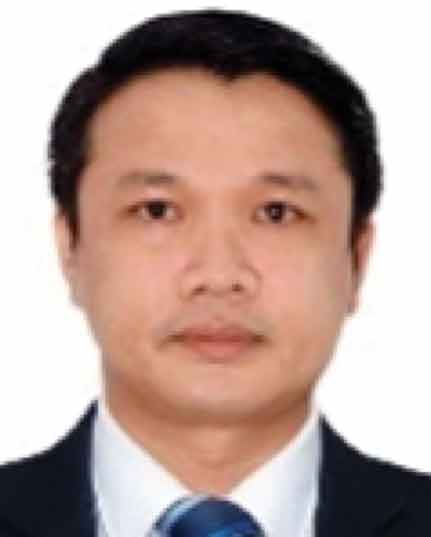
Van Phuoc Nguyen
Van Phuoc Nguyen is a lecturer at Posts and Telecommunications Institute of Technology, teaching courses in E-Business Management, International Business. He has published twenty-six scientfic papers. Research interests: technology management, operations research, operations and production management, innovation management, supply chain management, decision making theory, optimization, strategies, developing alternatives of sustainable development for business and management in industry. Nguyen’s recent publications include articles on challenges of Artificial Intelligence and Fintech.
References
- Atanassov, K., & Gargov, G. (1989). Interval valued intuitionistic fuzzy sets. Fuzzy Sets and Systems, 31(3), 343–28. https://doi.org/10.1016/0165-0114(89)90205-4
- Bain & Company. (2019). The future of Southeast Asia’s digital financial services. https://www.bain.com/insights/fufilling-its-promise/
- Bernardo, N. (2017). The future of FinTech: Integrating finance and technology in financial services. Palgrave Macmillan. https://doi.org/10.1007/978-3-319-51415-4
- Dang Thi Viet, D., Nguyen Thanh, L., & Nguyen Duc Hoai, A. (2022). Extending UTAUT2 in Mobile money adoption and actual use behavior: An empirical research in Vietnam during the Covid-19. Industrielle Beziehungen. Zeitschrift für Arbeit, Organisation und Management, 10(4), 139. https://doi.org/10.53384/inbe.101390943.2779.1862003510
- Dealroom.co Report. (2017). €90 billion in European VC-backed exits between 2014 and 2016. Is it enough?. https://dealroom.co/blog/record-e37-billion-in-european-vc-backed-exits-in-2016-is-it-enough
- Dealroom.co Report. (2020). The future of fintech in Southeast Asia. https://dealroom.co/blog/the-future-of-fintech-in-southeast-asia
- Deloitte. (2019). Fintech risk and compliance management: A framework to empower the organization. https://www2.deloitte.com/content/dam/Deloitte/us/Documents/finance/us-fintech-risk-and-compliance-management.pdf
- Dhivya, J., & Sridevi, B. (2019). A novel similarity measure between intuitionistic fuzzy sets based on the mid points of transformed triangular fuzzy numbers with applications to pattern recognition and medical diagnosis. Applied Mathematics-A Journal of Chinese Universities, 34(2), 229–252. https://doi.org/10.1007/s11766-019-3708-x
- Dick, F., Feng, H., Louis, L., Qu, J., & Shek, A. (2021,November,9). Seven technologies shaping the future of fintech. McKinsey Company. https://www.mckinsey.com/cn/our-insights/our-insights/seven-technologies-shaping-the-future-of-fintech
- Drucker, P. F., Christensen, C. M., & Govindarajan, V.; Harvard Business Review. (2013). HBR’s 10 must reads: On innovation.
- Focardi, R., & Martinelli, F. (1999). A uniform approach for the definition of security properties. Lecture Notes in Computer Science. International Symposium on Formal Methods, 794–813. https://doi.org/10.1007/3-540-48119-2_44
- Fu, S., Qu, X., Zhou, H., & Fan, G. (2018). A multi-attribute decision-making model using interval-valued intuitionistic fuzzy numbers and attribute correlation. International Journal of Enterprise Information Systems, 14(1), 21–34. https://doi.org/10.4018/IJEIS.2018010102
- Gomber, P., Kauffman, R. J., Parker, C., & Weber, B. W. (2018). On the Fintech revolution: Interpreting the forces of innovation, disruption, and transformation in financial services. Journal of Management Information Systems, 35(1), 220–265. https://doi.org/10.1080/07421222.2018.1440766
- Gomber, P., Koch, J., & Siering, M. (2017). Digital finance and FinTech: Current research and future research directions. Journal of Business Economics, 87(5), 537–580. https://doi.org/10.1007/s11573-017-0852-x
- Hootsuite Inc. (2020). Digital 2020: A comprehensive look at the state of the internet, mobile devices, social media, and ecommerce. https://www.hootsuite.com/resources/digital-2020
- Jain, K. C., & Chhabra, P. (2016). New series of information divergence measures and their properties. Applied Mathematics and Information Sciences, 10(4), 1433–1446. https://doi.org/10.18576/amis/100422
- Jinasena, D. N., Spanaki, K., Papadopoulos, T., & Balta, M. E. (2020). Success and failure retrospectives of FinTech projects: A case study approach. Information Systems Frontiers, 221, 1–16/10079. https://doi.org/10.1007/s10796-020-10079-4
- Lee, D. K. C., & Teo, E. G. S. (2015). Emergence of fintech and the Lasic principles. SSRN Electronic Journal, 3(3), 1–29. https://doi.org/10.2139/ssrn.2668049
- Luo, M., & Liang, J. (2018). A novel similarity measure for interval-valued intuitionistic fuzzy sets and its applications. Symmetry, 10(10), 441. https://doi.org/10.3390/sym10100441
- Miklos, D., Somesh, O., Tunde, K., & Kausik, R. (2015). Cutting through the FinTech noise: Markers of success, imperatives for banks. McKinsey Company. https://www.mckinsey.com/industries/financial-services/our-insights/cutting-through-the-noise-around-financial-technology
- Mishra, A. R., & Rani, P. (2018). Interval-valued intuitionistic fuzzy WASPAS method: Application in reservoir flood control management policy. Group Decision and Negotiation, 27(6), 1047–1078. https://doi.org/10.1007/s10726-018-9593-7
- Mishra, A. R., Rani, P., Pandey, K., Mardani, A., Streimikis, J., Streimikiene, D., & Alrasheedi, M. (2020). Novel multi-criteria intuitionistic fuzzy SWARA–COPRAS approach for sustainability evaluation of the bioenergy production process. Sustainability, 12(10), 4155. https://doi.org/10.3390/su12104155
- Montes, I., Pal, N. R., Janiš, V., & Montes, S. (2015). Divergence measures for intuitionistic fuzzy sets. IEEE Transactions on Fuzzy Systems, 23(2), 444–456. https://doi.org/10.1109/TFUZZ.2014.2315654
- Organization for Economic Co-operation and Development. (2020). Multidimensional review of Vietnam: Towards an integrated, transparent and sustainable economy. OECD Publishing. https://doi.org/10.1787/367b585c-en OECD Development Pathways.
- Pekala, B., & Balicki, K. (2017). Interval-Valued intuitionistic fuzzy sets and similarity measure. Iranian Journal of Fuzzy Systems, 14(4), 87–98. https://doi.org/10.22111/ijfs.2017.3327
- Puschmann, T. (2017). Fintech. Business and Information Systems Engineering, 59(1), 69–76. https://doi.org/10.1007/s12599-017-0464-6
- PwC. (2016). Security challenges in the evolving fintech landscape. https://www.pwc.in/assets/pdfs/consulting/cyber-security/banking/security-challenges-in-the-evolving-fintech-landscape.pdf
- PwC. (2019). Crossing the lines: How fintech is propelling FS and TMT firms out of their lanes. PwC Global Fintech Report. https://www.pwc.com/gx/en/industries/financial-services/assets/pwc-global-fintech-report-2019.pdf
- Riemer, K., & Johnston, R. B. (2019). Disruption as worldview change: A Kuhnian analysis of the digital music revolution. Journal of Information Technology, 34(4), 350–370. https://doi.org/10.1177/0268396219835101
- Schwartz, E. S., & Moon, M. (2000). Rational pricing of internet companies. Financial Analysts Journal, 56(3), 62–75. https://doi.org/10.2469/faj.v56.n3.2361
- Sisinni, E., Saifullah, A., Han, S., Jennehag, U., & Gidlund, M. (2018). Industrial Internet of things: Challenges, opportunities, and directions. IEEE Transactions on Industrial Informatics, 14(11), 4724–4734. https://doi.org/10.1109/TII.2018.2852491
- song, Y., Wang, X., Lei, L., & Xue, A. (2014). A new similarity measure between intuitionistic fuzzy sets and its application to pattern recognition. abstract and Applied Analysis, 2014(8), 1–11/384241. https://doi.org/10.1155/2014/384241
- Sonnenschein, J., & Mundaca, L. (2016). Decarbonization under green growth strategies? The case of South Korea. Journal of Cleaner Production, 123(6), 180–193. https://doi.org/10.1016/j.jclepro.2015.08.060
- Tellimer Insights. (2020). Vietnam digital payments: On the cusp of rapid change. https://tellimer.com/article/vietnam-digital-payments-on-the-cusp-of-rapid
- Tucker, D. (2018, October 8). Elsevier connect, Elservier. https://www.elsevier.com/connect/the-fast-moving-world-of-fintech-is-now-a-fast-growing-research-topic
- UOB, PwC, SAF. (2021). FinTech in ASEAN 2021: Digital takes flight. https://singaporefintech.org/fintech-in-asean-2021/
- Warner, J. (2017). Future of fintech. Consumer survey 2017. Telegraph Media Group. https://www.telegraph.co.uk/content/dam/business/spark/Fintech/Telegraph_Fintech_Report2017.pdf
- Wataru, S, & Hoang, L. (2021, August, 18). Vietnam emerges as South-East Asia’s next fintech battleground. Financial Times. https://www.ft.com/content/98bb236b-8eb4-4ac2-9d5d-02d992fddfd2
- Wiwanto, F. (2020, April, 2). Three Factors Driving The Rise Of Fintech And What The Banking Industry Can Learn From Them. Forbes Media. https://www.forbes.com/sites/forbesfinancecouncil/2020/04/02/three-factors-driving-the-rise-of-fintech-and-what-the-banking-industry-can-learn-from-them/?sh=6ccb5fc86d76
- World Economic Forum. (2015). The global competitiveness report 2015. http://www3.weforum.org/docs/gcr/2015-2016/Global_Competitiveness_Report_2015-2016.pdf
- Xie, J., Ye, L., Huang, W., & Ye, M. (2021). Understanding FinTech platform adoption: Impacts of perceived value and perceived risk. Journal of Theoretical and Applied Electronic Commerce Research, 16(5), 1893–1911. https://doi.org/10.3390/jtaer16050106
- Xu, Z., & Cai, X. (Eds.). (2012). Intuitionistic fuzzy information aggregation. In Intuitionistic fuzzy information aggregation (pp. 1–102). Springer-Verlag Berlin Heidelberg. https://doi.org/10.1007/978-3-642-29584-3_1
- Xu, Z. S., & Chen, J. (2008). An overview of distance and similarity measures of intuitionistic fuzzy sets. International Journal of Uncertainty, Fuzziness and Knowledge-Based Systems, 16(4), 529–555. https://doi.org/10.1142/S0218488508005406
- Xu, Y., & Zhang, J. (2019). Study on evaluation of the foreign trade sustainable development with interval-valued intuitionistic fuzzy information. Journal of Intelligent and Fuzzy Systems, 37(2), 1929–1935. https://doi.org/10.3233/JIFS-179254
- Yang, Z. (2016). An analysis of knowledge-power structure and orientalism, Proceedings of the 2016 2nd International Conference on Economics, Management Engineering and Education Technology (ICEMEET 2016) (pp. 377–379). https://doi.org/10.2991/icemeet-16.2017.78.
- Yang, C., & Liu, H. M. (2012). Boosting firm performance via enterprise agility and network structure. Management Decision, 50(6), 1022–1044. https://doi.org/10.1108/00251741211238319
- Yazdani, M., Wen, Z., Liao, H., Banaitis, A., & Turskis, Z. (2019). A grey combined compromise solution (CoCoSo-G) method for supplier selection in construction management. Journal of Civil Engineering and Management, 25(8), 858–874. https://doi.org/10.3846/jcem.2019.11309
- Zeng, S., Hu, Y., Balezentis, T., & Streimikiene, D. (2020). A multi-criteria sustainable supplier selection framework based on neutrosophic fuzzy data and entropy weighting. Sustainable Development, 28(5), 1431–1440. https://doi.org/10.1002/sd.2096
- Zrobek, S., Kovalyshyn, O., Renigier-Biłozor, M., Kovalyshyn, S., & Kovalyshyn, O. (2020). Fuzzy logic method of valuation supporting sustainable development of the agricultural land market. Sustainable Development, 28(5), 1094–1105. https://doi.org/10.1002/sd.2061