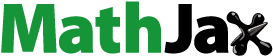
Abstract
The construction industry is the most important for the social, economic, and political development of countries. The road construction sector is the largest in the construction industry. Inappropriate road maintenance leads to an inefficient and random way of spending maintenance costs. Therefore, road maintenance needs to prevent deterioration that shortens the service life of the road. Thus, the study was focused on a road maintenance strategy evaluation and selection model by integrating Fuzzy AHP and Fuzzy TOPSIS methods. The evaluation was a multiple-criteria decision-making problem. The fuzzy AHP technique was used to determine criterion weight. To deal with the uncertain judgment of decision makers, a fuzzy TOPSIS method is applied as an evaluation tool in a fuzzy environment where the uncertain, subjectivity, and imprecise judgments of decision makers are handled with linguistic values parameterized by triangular fuzzy numbers. To achieve the objective, the data were collected from primary and secondary sources. The method of data analysis for this study was made by integrated methodology, and the analysis was made by using Microsoft Excel. The study revealed that quality, maintenance time, environment, production cost, and profit are the most important selection criteria. The finding of the study revealed that periodic maintenance was the best relative closeness value among the considered alternatives. This study recommends that the analysis method can be implemented by the Ethiopian Roads Authority to demonstrate its practicality in selecting the appropriate strategy.
PUBLIC INTEREST STATEMENT
The current study examined a road maintenance management strategy evaluation and selection model by integrating Fuzzy AHP and Fuzzy TOPSIS methods in the case of the Ethiopian Roads Authority. To achieve the objectives, a literature review was conducted to identify the key selection criteria using predefined criteria to select the appropriate road maintenance strategy used by the Ethiopian Roads Authority that must be set from published studies.
Furthermore, one of the fundamental aims of this study was to select the appropriate road maintenance strategy. In this case study, Experts Panel was used based on their subject knowledge, experience, and skill, and a panel of decision experts was invited to fill a questionnaire prepared based on a pairwise comparison matrix analysis with fuzzy scales. The findings of this study are thought to be helpful to provide a reference guide for the company, academicians, policymakers, and further investigation on the topic as required.
1. Introduction
Construction industry has long been realized as one of the most important enablers for the social, economic, and political development of countries. The industry, a separate economic sector, directly contributes to the economic expansion (Velmurugan & Dhingra, Citation2015).
The road infrastructure of Ethiopia has deteriorated and faced high maintenance costs on the aging transportation highway such that it was a serious prevention to the efforts of the Government of Ethiopia for reviving the economy toward achieving sustained poverty reduction by improving access links between production centers and markets (Mkilania, Citation2016).
According to (Mohamed, Citation2005), road maintenance is done to preserve and keep each type of roadway, roadside, and structure as nearly as possible in its original condition as constructed or as subsequently improved and the operation of highway facilities and services to provide satisfactory and safe transportation. As the road network deteriorates, the whole country loses major assets built up over the years, assets created with vast amounts of money, time, and effort (Velmurugan & Dhingra, Citation2015).
According to (Mkilania, Citation2016), there is no visible and clear picture presented that which types of road sections are carried out by what types of maintenance strategies they are considered. This means that there is no obvious connection between the maintenance activities and the fulfillment of the company’s strategic goals. Yamasaki (Citation1976) stated that the traditional way of determining maintenance needs in many cities and highway agencies depends upon visual inspection of road condition, and maintenance activities are performed as a result of user complaints when the road components become exposed to damage and the road becomes out of service.
By considering this situation, road maintenance strategy can be identified as the proposed problem to be studied. Thus, this study focused on the road maintenance management strategy evaluation and selection model by integrating fuzzy AHP and fuzzy TOPSIS methods to overcome maintenance problems in the company. The fuzzy AHP technique was used to determine criterion weight. Alternatively, a Fuzzy Technique for Order Performance by Similarity to Ideal Solution (TOPSIS) model was used to help the decision experts for evaluation and select appropriate road maintenance management strategy in a fuzzy environment where the vagueness and subjectivity are handled with linguistic values parameterized by triangular fuzzy numbers. The proposed method enables decision analysts to better understand the complete evaluation process and provide a more accurate, effective, and systematic decision support tool. The importance of this research paper is to provide a reference guide for the company in general to know the selection criteria for the appropriate road maintenance management strategies. The study provides a significant source of secondary data for future users to get knowledge, skill, and understanding of road maintenance management strategies.
The remainder of this paper is organized as follows: Section 2 describes the road maintenance management strategy selection criteria, road maintenance management strategy, Fuzzy AHP, and Fuzzy TOPSIS Multi-criteria decision-making model, and Section 3 presents how the integrated methodology of fuzzy AHP and fuzzy TOPSIS can be adopted. Section 4 shows numerical analysis and results of fuzzy AHP and fuzzy TOPSIS results along with some discussions relating to maintenance selection criteria and maintenance strategy. Section 5 presents discussions, managerial implications, and limitations. Finally, general conclusions and remarks are then presented in Section 6.
2. Literature review
The construction industry can be defined in both developed and developing countries and may be viewed as that sector of the economy which, through planning, design, construction, maintenance and repair, and operation, transforms various resources into constructed facilities (Yamasaki, Citation1976).
According to (Mkilania, Citation2016), road maintenance provides delivers a safe, serviceable, and sustainable road network taking into account the need to contribute to the wider objectives of asset management, integrated transport, and continuous improvement. Road maintenance is defined as a program to preserve, repair, and restore a system of highways with its elements to its designed or accepted configuration, but does not include rehabilitation, constructing shoulders, or widening roads (Mohamed, Citation2005).
2.1. Maintenance
Maintenance is the combination of all technical, administrative, and managerial actions during the life cycle of an item intended to retain it in or restore it to a state in which it can perform the required function under normal stated operating conditions (Mohamed, Citation2005). Maintenance provides maximization of the use of road components and the reliability of these assets to achieve operational and business objectives (Mkilania, Citation2016).
2.2. Road maintenance management
British Standards Institution (BSI) (Citation2010) defines maintenance management as all activities of the management that determine the maintenance objectives, strategies, and responsibilities. Maintenance management is concerned with good control of the maintenance function and its related areas to best assist the objectives and goals of the entire organization. Mkilania (Citation2016) and Enofe (Citation2009) define road maintenance under two subdivisions as physical maintenance and traffic services. Physical maintenance is the preservation and upkeep of a highway in its constructed condition. Traffic services are defined as the operation of a highway facility and services help to provide safe, convenient, and economical highway transportation (Enofe, Citation2009).
According to (Yamasaki, Citation1976) road maintenance management can simply be defined as managing the road maintenance activities to ensure the availability of the road components and facilities helps to keep the downtime to a minimum. Road maintenance management is essentially a management philosophy, and it should be considered a business function that provides opportunities to retain the quality, life, and value of road (Karia, Citation2014).
2.3. Road Maintenance Strategies Selection Criteria
According to (Velmurugan & Dhingra, Citation2015) maintenance strategy is a systematic approach to upkeep the facilities and components and it varies from facility to facility. According to (Rastegari & Salonen, Citation2015) maintenance strategy involves the identification, researching, and execution of many repairs, replacement, and inspection decisions and is concerned with formulating the best life plan for each unit of the road, in coordination with other functions concerned. To select the best road maintenance management strategy firstly, its maintenance management goals were first identified, and defining the criteria that can be used to evaluate the alternatives must be set (Jafari et al., Citation2008). The following criteria were identified in the literature as the road maintenance strategy selection criteria.
2.3.1. Maintenance Cost
According to Mong et al. (Citation2018), costing and budgeting for the maintenance department embrace the provision of financial information on labor and material expenditure. Maintenance cost considers the cost required to maintain the road components that are managed using different maintenance management strategies (Mwanza & Mbohwa, Citation2017).
Training: refers to the obtaining or transferring knowledge, skills, and abilities process needed to carry out a specific maintenance activity or function (Mwanza & Mbohwa, Citation2017). Maintenance training describes the formal, ongoing efforts that are made within organizations to improve the performance and self-fulfillment of their employees through a variety of methods and maintenance programs in the maintenance organization (Makinde et al., Citation2016).
Maintenance Labor Cost: the amount of all salaries paid to the workers, as well as the employee benefits and payroll taxes charged by an employer (Rastegari & Salonen, Citation2015). The maintenance labor costs are broken down into direct labor costs as salaries for all employees in maintenance, utilities, and road components, and indirect maintenance labor costs (Zafar, Citation2018).
Production Cost: The costs a company incurs for maintaining an item or providing a service that generates revenue for the company (Mong et al., Citation2018). Maintenance is carried out by the firms by using available resources as input for executing the maintenance activities as output by providing the production cost to pay for the maintenance employees.
Cost of Advising and Consulting: Provided for consulting and supervising to implement or execute maintenance works, to supervise and control their quality and progress, and to certify the completion of works (Milhem et al., Citation2014). Cost of advising and consulting is mostly a necessary component of maintenance cost regardless of the type of maintenance strategy adopted whether corrective, periodic, or predictive. However, the cost of advising and consulting varied in amount between strategies (Suzuki, Citation2018).
Cost of Inventories: The cost of inventories is examined by management as part of its evaluation of how much inventory to keep on hand for executing the maintenance strategies (Tucker, Citation2016).
2.3.2. Value-adding
Value-adding is one of the most important selection criteria in maintenance management strategy to measure the value created by the maintenance of roads (Parida & Kumar, Citation2007). This brings to the focus on doing the right things keeping in view the business objectives of the maintenance company (Suzuki, Citation2018).
Quality: Road facilities failures may cause a drop in road quality, and it does not provide the intended service for the customers (Knezevic & Knezevic, Citation2012). Quality maintenance is aimed towards customer delight through the highest quality through defect-free maintenance (Suzuki, Citation2018).
Profit: Profit is a measure of profitability which is the owner’s major interest in the income formation process of maintenance management (Tucker, Citation2016).
Maintenance time: It is critical for road maintenance and service delivery arrangements for road management to provide for efficient, effective, and economic management of the road system (Verbert et al., Citation2017). During the construction process, some assets may fail to cause delays in order delivery.
2.3.3. Maintenance Implementation
Maintenance implementation is one approach through which maintenance objectives, methods, and rules are implemented throughout programs, financial plan, and procedure (Tucker, Citation2016). The following implementation criteria are also included under road maintenance implementation criteria.
Access to Equipment and Technology: The selection of maintenance strategy considers choices of appropriate technology, accessibility of advanced equipment, and methods of construction are the most important criteria required for the execution of a maintenance task (Milhem et al., Citation2014).
Desire of workers: Some workers may not want predictive maintenance because workers do some extra duties in the predictive type of maintenance strategies (Parida & Kumar, Citation2007).
Desire of Top Management: Top managers have a priority on some maintenance activities over others and have the authority to do many things in the maintenance organization (Knezevic & Knezevic, Citation2012). Top managers are responsible for developing the organization’s strategy and being a steward of its vision and mission.
Skills and Competency Development: The key to productive employment for maintenance management. Hence, it is an important means for increased productivity, maintenance of company development, inclusive economic growth, and poverty reduction (Knezevic & Knezevic, Citation2012).
Work Order Management: The ability to direct maintenance personnel to do planned work at the right times using a written work order that allows a maintenance manager to direct the staff according to plan (Milhem et al., Citation2014).
2.3.4. Safety
Safety in maintenance is concerned with the freedom from danger, protection from or not being exposed to accidents, injuries, and health problems during the process of carrying out maintenance procedures (Makinde et al., Citation2016). The safety criterion for road maintenance management strategy selection consists of the following (Parida & Kumar, Citation2007).
Environment: safety policies and procedures maintain a healthy working environment and the whole internal and external environment (Mwanza & Mbohwa, Citation2017).
Facilities: Maintenance facilities mean a public facility, supporting maintenance, repair, material storage, and similar activities including street and similar uses of maintenance activities. Maintenance facilities are needed for the completion of maintenance tasks, such as road and special repair and overhaul facilities (Knezevic & Knezevic, Citation2012).
Safety Training: Describes the set of activities aimed at providing workers with the knowledge and skills to perform their duties safely and effectively (Mohamed, Citation2005).
Personnel Safety: Maintenance is the freedom from danger or risk from harm during the process of carrying out maintenance procedures (Mwanza & Mbohwa, Citation2017).
2.3.5. Effectiveness
Maintenance effectiveness is a maintenance performance measurement that helps to focus on maintenance objectives and quantifiable values (Galar et al., Citation2017).
The Effectiveness criterion consists of the following:
Employee satisfaction: The employee comfort and acceptability of the application provided by the maintenance monitoring activities. Employee satisfaction is used to describe what lines her employees are happy, contented, and fulfilling their desires and needs at maintenance work (Sageer et al., Citation2012).
Functionality: maintenance functionality and serviceability are the quality of the road components being able to provide the intended service for the users (Azadeh & Zadeh, Citation2015).
Maintainability: Maintainability is the measure of the ability of the road system to be retained in or restored to a specified condition when maintenance is performed by personnel having specified skill levels using prescribed procedures and resources at each prescribed level of maintenance and repair (Tadele, Citation2007).
Reliability: Ensures maintenance tasks are performed in an efficient, cost-effective, reliable, and safe manner (Tadele, Citation2007). According to (Azadeh & Zadeh, Citation2015) the reliability of road components can be defined as the probability that an item will perform a specific function.
2.4. Road maintenance management strategies
Road Maintenance management strategies can be classified as follows.
2.4.1. Routine maintenance
According to (Basahel & Taylan, Citation2016), routine maintenance is based on routine (daily) inspection of the condition of the pavement, cut and fill slopes, drainage, bridges, and other structures and facilities to monitor any defects and damage.
Activities are carried out as frequently several times as required during each year to ensure serviceability at all times and in all weathers that are funded from the recurrent budget (Basahel & Taylan, Citation2016).
Routine maintenance refers to small maintenance works to be carried out in all seasons on all roads regularly involving the cleaning and clearing of different road elements to ensure that they work properly and that damage to the road is avoided (Nedevska et al., Citation2017).
2.4.2. Periodic Maintenance
Maintenance is based on a detailed inspection performed at certain time intervals such as seasonally or yearly depending on the type and kind of facilities (Suzuki, Citation2018). According to (Basahel & Taylan, Citation2016), periodic maintenance covers all longer-term programmable operations required within the service life of the road. He also defined that periodic maintenance as operations that are occasionally required on a section of road after many years. According to (Galar et al., Citation2017), periodic maintenance operations are costly and require specific identification and planning for implementation and often require design.
2.4.3. Emergency Maintenance
(Tran Anh et al., Citation2018) stated that emergency maintenance comprises works to restore road and road-related facilities to their normal operating conditions after they are damaged by road accidents or natural causes. Emergency road maintenance activities cannot be estimated with any certainty in advance; the activities include emergency works to repair landslides, washouts, debris on the road, and broken drainage structures that result in the road being cut off or made impassable. Galar et al. (Citation2017) stated that urgent activities that must be done in response to an event are beyond the control of the maintenance personnel.
2.4.4. Preventive Maintenance
It describes actions taken to prevent premature deterioration and to retard the progression of deficiencies to reduce the rate of deterioration. Nedevska et al. (Citation2017) and Mohamed (Citation2005) defined preventive maintenance as to repair that addresses causes of deterioration leading to the need for costly rehabilitation work in the future. According to (Basahel & Taylan, Citation2016) preventive maintenance activities are conducted to correct minor defects, retard future deterioration, and maintain and improve the functional condition of the system.
2.4.5. Corrective Maintenance
According to (British Standards Institution (BSI), Citation2010), corrective maintenance activities are carried out after fault recognition and intended to put an item into a state in which it can perform a required function. Under (Hivarekar et al., Citation2020) corrective maintenance actions are taken to correct deficiencies which are potentially hazardous defects that seriously affect the road operation to keep the road within a tolerable level of serviceability.
2.4.6. Predictive Maintenance
Predictive maintenance is defined as condition-based maintenance carried out to forecast derived from the analysis and evaluation of significant parameters of the condition of the item. According to (Tran Anh et al., Citation2018), predictive maintenance is defined as conducted on the most cost-effective date after residual useful life has forecast an item. Predictive maintenance is a technique that uses condition-monitoring tools to track the performance of assets during normal operation to detect possible defects and fix them before the asset fails so maintenance can occur at just the right time (Hivarekar et al., Citation2020).
2.4.7. Reliability-Centered Maintenance
Reliability Centered Maintenance can be defined as an organized, rational process for improving the maintenance requirements of a physical resource in its operating context to understand its inherent reliability which can be attained with an effective maintenance program (Illeris, Citation2012). Reliability-centered maintenance is the optimum mix of reactive, time or interval-based, condition-based, and proactive maintenance practices to determine the maintenance requirements of the road. Afefy (Citation2010) and CitationTrojan (2018) stated that reliability-centered maintenance is a strategy that is implemented to optimize the maintenance program of a corporation or a facility.
2.5. Multi-Criteria Decision-Making Model
Multi-criteria decision-making (MCDM) has been widely used in selecting or ranking a finite set of decision alternatives characterized by multiple and usually conflicting criteria (attributes; Hivarekar et al., Citation2020).
Fuzzy MCDM performance ratings and weights are usually represented by fuzzy numbers. An alternative is calculated by aggregating all criteria weights and alternative ratings, where alternatives with a higher utility are preferred (Wang & Lee, Citation2009).
Fuzzy multiple criteria decision-making, approach can be more suitable to explain how decision experts make decisions to select the best alternatives from a set of alternatives. While crisp data are inadequate to model the real-life situations in MCDM, we apply linguistic variables to specifically describe the degrees of a criterion.
A linguistic variable is a variable which applies words or sentences in a natural or artificial language to describe its degree of value (Saeednamaghi & Zare, Citation2014).
Multi-Criteria Decision-Making is a method designed to help decision-makers conduct evaluations of alternatives characterized by numerous and conflicting factors influencing the status and evolution of a system and find compromises in the decision-making process (Hivarekar et al., Citation2020).
Prior research on the applied technique in road maintenance had some limitations; however, there are some studies that would be undertaken in other knowledge areas that are discussed in the next section. There are numerous ways to multi-criteria decision-making models. The study does, however, take into account a combined methodology of fuzzy analytical hierarchy process and fuzzy approach for order preference by similarity to ideal solution method as mentioned in the following.
2.5.1. Fuzzy Analytical Hierarchy Process
Analytic hierarchy process (AHP) is a powerful method to solve complex decision problems. Any complex problem can be decomposed into several sub-problems using AHP in terms of hierarchical levels where each level represents a set of criteria relative to each sub-problem (Kazan et al., Citation2015).
Fuzzy set theory is one of the most common methods used in multi-criteria decision-making studies. The importance of the weights and the performance ratings of the criteria are measured using this numerical scale (Saeednamaghi & Zare, Citation2014).
According to (Saeednamaghi & Zare, Citation2014), conducted a study on the selection of the most appropriate maintenance strategy in a local newspaper printing facility in Turkey by using Analytical Hierarchical Process (AHP) and the Analytical Hierarchical Process (ANP). Furthermore, Fu et al. (Citation2020) carried out a study on formulating an effectual tea assessment system to increase the credibility of the current tea evaluation mechanism by using a Fuzzy Analytic Hierarchy Process.
2.5.2. Fuzzy Technique for Order Preference by Similarity to Ideal Solution Method
TOPSIS is a multi-criteria decision analysis method, which was originally developed by Hwang and Yoon (Saghafian & Hejazi, Citation2005). Fuzzy TOPSIS helps to solve the vagueness inherent in the maintenance strategy selection problem but also increases the accuracy of the result by the implementation of a fuzzy linguistic approach.
It bases upon the concept that the chosen alternative should have the shortest distance from the positive ideal solution that maximizes the benefit criteria and minimizes the cost criteria and the farthest from the negative ideal solution that maximizes the cost criteria and minimizes the benefit criteria (Fu et al., Citation2020).
Fuzzy TOPSIS methodology is applied as an evaluation tool in some studies, where uncertain and imprecise judgments of decision makers are translated into fuzzy numbers
Momeni et al. (Citation2011) conducted a study on a Fuzzy TOPSIS-based approach for the selection of maintenance strategies in Electerofan Company is studied. Similarly, Basahel and Taylan (Citation2016) conducted a study on identifying and evaluating the factors that impact workplace safety conditions at construction sites in Saudi Arabia by using fuzzy AHP and fuzzy TOPSIS approaches.
Furthermore, Wang and Lee (Citation2009) conducted a study on the assessment of TEOG examination success by using TOPSIS multi-criteria decision-making method practice. The overall steps and procedures employed for the applied methodologies conducted in this study were briefly described in section 3.
3. Methodology
The study can employ a multi-method approach, encompassing quantitative and qualitative research to obtain information for the objective of the study. Quantitative approaches were carried out by questionnaire survey, whereas qualitative research was done by document review. In this paper, a total of 22 decision experts were chosen as the target population in the Ethiopian Roads Authority because they have background knowledge on the general design of the highway and its construction from the feasibility stage to design and then finally construction, completion, and maintenance of the road.
The sample size that can be used for this study was consensus-based MCDM methods called Experts Panel in MCDM methods. According to Tarei et al. (Citation2018), the number of experts used for carrying out MCDM methods was based on their subject knowledge, experience, skill, and personal contacts. For this study, convenience sampling was adopted because it focusing on representative samples which are willing, readily available for the study.
Primary data were collected from questionnaire survey and document analysis, and secondary data were collected from the literature review that were used to collect all the relevant data to answer the research questions.
The study questionnaire was prepared based on a pairwise comparison matrix analysis with fuzzy scales through different selection criteria in road maintenance management in the Ethiopian Roads Authority.
The questionnaires were distributed to owners, consultants and contractors in the road maintenance projects in the Ethiopian Roads Authority. The decision Experts involved in the survey invited to fill a questionnaire had several years of experience which is selected based on their subject knowledge, experience, and skill. The characteristics of the decision experts participated in survey are summarized in Table .
Table 1. Experts demographics
The overall framework and steps of the newly proposed methodology are carried out by integration of fuzzy AHP and fuzzy TOPSIS as shown in Figure .
3.1. Fuzzy multi-criteria decision-making model
Decision-making is a process of identifying and choosing alternatives based on the values and preferences of the decision-maker (Gade, Citation2014). In Multi-Criteria Decision-Making Models choosing an alternative, the overall goal depends on two or more criteria (Literate & Indonesia, Citation2020). According to Saghafian and Hejazi (Citation2005), decision-making problem is the process of finding the best option from all of the feasible alternatives. A multi-criteria decision-making problem can be concisely expressed in matrix format as:
Where
is possible alternatives among which decision-makers have to choose,
is the criteria with which alternative performance is measured,
is the rating of alternative
concerning criterion
and
is the weight of criterion
.
Multi-criteria decision-making model selection approaches for the evaluation of appropriate road maintenance management strategy used in this study can be described as follows.
3.1.1. Fuzzy Analytical Hierarchy Process
The fuzzy AHP method is an aggregated technique that was developed based on Saaty’s (Basahel & Taylan, Citation2016). The analytical hierarchy process is primarily applied to decision problems in uncertain situations with multiple criteria. (Literate and Indonesia (Citation2020) highlighted that in the analytical hierarchy process fuzzy set theory and fuzzy operation were introduced to overcome the subjectivity, inaccuracy, and fuzziness produced when making decisions.
It is impossible to achieve accurate results or assessment if the data is immeasurable. In other words, crisp data or single numerical value is insufficient to model real-life problems. This is due to human evaluation is usually vague and cannot be expressed with an exact value called fuzzy.
A fuzzy set can be defined mathematically by a membership function, which assigns each element x in the universe of discourse × a real number in the interval (Velmurugan & Dhingra, Citation2015).
A triangular fuzzy number is a special class of fuzzy numbers whose membership is defined by three real numbers, expressed as (a1, a2, a3). The triangular fuzzy numbers are represented as follows in Figure . According to Meharie et al. (Citation2019), the fuzzy set can be defined as follows:
A linguistic variable is a variable that applies words or sentences in artificial language to describe the degree of value. In this study, the triangular fuzzy numbers are used to represent the value (Siew-Hong & Kamaruddin, Citation2012).
where a1 and a3 mean the lower and upper bounds of the fuzzy number , and m is the middle value for
, The TFN can be denoted by
.
The operational laws of TFN = (a1, a2, a3) and
= (b1, b2, b3) are presented as the following equations (Sun, Citation2010).
Addition of the fuzzy number ⊕.
Multiplication of the fuzzy number ⊗
Subtraction of the fuzzy number
Division of a fuzzy number
Reciprocal of the fuzzy number
The analytic hierarchy process is one of the types in MCDMM which is a predominant approach to determine the preference weights of the key selection criteria in road maintenance management strategy. The following steps were used to implement the fuzzy analytic hierarchy process technique (Kumar et al., Citation2013).
Step 1: Analyzing Problems
The road maintenance management strategy selection criteria were determined based on the review of prior literature, and structured questionnaires were undertaken for 22 decision experts. Those experts were invited to survey hierarchical structures of analytic hierarchy process, which were established by identifying five main criteria and 21 sub-criteria and seven maintenance alternatives.
Step 2: Construct a Hierarchical Model
Using criteria, sub-criteria, and alternatives, a hierarchical structure is constructed to apply the analytic hierarchy process and fuzzy TOPSIS algorithms. Then, relationships among criteria and sub-criteria are determined and reflected in the hierarchical model shown in Figure . The hierarchical structure of the maintenance strategy selection problem includes four levels. The first level of the hierarchy represents the ultimate goal of the problem, while the second level of the hierarchy consists of five main maintenance strategy selection criteria. These criteria are decomposed into various sub-criteria. Finally, the bottom level of the hierarchy represents seven alternative maintenance strategies.
Step 3: Questionnaire Design and Survey
Based on the established hierarchical structure, a questionnaire was designed to sort out the importance levels of criteria and sub-criteria were identified based on their prior experience in the road construction sector (5 years or more), educational background, and their prior knowledge and commitment to participate in the study.
After carrying out expert’s panel selection, the criteria and sub-criteria were converted to triangular fuzzy scale to reflect the expert’s rating of importance. To compare the importance of each evaluation criterion, it was categorized into nine linguistic terms on a scale as shown in Table . The linguistic scale in the fuzzy AHP method was used to categorize the criteria (C) based on their importance.
Table 2. Fuzzy linguistic terms determined for the criterion weight comparison (Kil et al., Citation2016)
Step 4: Building pairwise comparison matrices with fuzzy scales
Pairwise comparisons are done among related criteria and sub-criteria following the scale suggested by (Annals, Citation2017). A special questionnaire form is used to complete the pairwise comparison matrix. In this comparison criteria, sub-criteria are used for comparing alternative maintenance strategies by experts in the field (Kou et al., Citation2017). The pairwise comparisons are members of the set: {1/9, 1/8, 1/7, 1/6, 1/5, 1/4, 1/3,1/2, 1,2,3,4,5,6,7,8,9}. The pairwise comparison judgments are represented by fuzzy triangular numbers denoted by ãij = (lij,mij, uij) and n(n-1)/2 judgments are required for each comparison group for a level to construct a positive fuzzy reciprocal comparison matrix Ă = {ãij} (Basahel & Taylan, Citation2016). The matrix is expressed as follows.
Where,
Step 5: The consistency test
(Huang & Ho, Citation2013) stated that consistency ratio (CR) was used to assess the reliability and credibility of the questionnaire. The consistency ratio for each decision experts was calculated by using an Excel template through using the general formula. When the value of CR ≤10% indicates a good level of consistency in the comparative judgments represented in that matrix, it is acceptable. CR value greater than 10% has resulted in the inconsistency of judgments within that matrix. This suggests that the evaluation process needs to be reviewed and repeated to improve the consistency of the questionnaire.
As proposed by (Abay et al., Citation2019) the consistency ratio is calculated as per the following steps.
Calculate the eigenvector and largest Eigenvalue (
) for each matrix of order n. Principal Eigenvalue (
) is obtained from the summation of products between each element of the Eigenvector and the sum of columns of the reciprocal matrix.
Compute the consistency index and consistency ratio for each matrix of order “n” by the formula (Vahidnia et al., Citation2008).
Where, RI is random consistency index obtained from a large number of simulations runs and varies depending upon the number of criteria as shown in Table .
n= number of criteria
Table 3. Random consistency index (RI; Vahidnia et al., Citation2008)
Step 6: Transforming fuzzy scales into triangular fuzzy numbers
The fuzzy nine-scale linguistic scaling to weigh the importance of each main-criterion and sub-criteria were transformed into triangular fuzzy numbers as shown in Table .
Table 4. The triangular fuzzy number of linguistic variables and fuzzy scales (Wang et al., Citation2019)
Step 7: Aggregating the experts’ decision
To aggregate the decision of experts, the fuzzy geometric mean was the most common operation used in fuzzy multiple criteria decision-making. The following formula can be used to transfer expert opinions into triangular fuzzy numbers (Fu et al., Citation2020).
where -is the integrated triangle fuzzy Number by N experts.
k is the i-th to the j-th factor pair comparison by expert k.
⊗- is the symbol of matrix multiplication.
= (aij1⊗ aij 2⊗ aij 3 … … … … … .⊗ aij10) 1/22
= (aij1⊗ aij 2⊗ aij 3 … … … … … .⊗ aij10) 1/22
Step 8: Calculating fuzzy geometric mean and fuzzy weight of criteria
To find the results of the fuzzy geometric mean and fuzzy weight the fuzzy analytical operation can be done by using MS-Excel for the calculation of importance coefficient is calculated using formula presented below.
where ain is fuzzy comparison value of criterion i to criterion n
ri is the geometric mean of fuzzy comparison value of criterion i to each criterion,
wi is the fuzzy weight of the i-th criterion, which can be indicated by a triangular fuzzy number.
⊕ is the symbol of matrix plus
Hence wi = (Lwi, Mwi, Uwi). Lwi, Mwi, and Uwi stand for the lower, middle, and upper values of the fuzzy weight of the i-th criterion, respectively.
Step 9: Weight priority by defuzzification
Defuzzification is used to renew the fuzzy number into a single crisp value (Fu et al., Citation2020). The center of the area converts a fuzzy weight into a non-fuzzy value and has been extensively applied in defuzzification by the following equation (Wang et al., Citation2019).
After calculating the non-fuzzy value, it can perform normalization to obtain the local weights for each criterion. The sum of local weights of the main criteria and sub-criteria on the same hierarchy was 1.00.
Step 10: Calculate the global weights of all criteria.
According to Kil et al. (Citation2016), the value of global weight equaled the value of the local weight within each main criteria multiplied by the value of local weight within each sub-criteria. The sum of global weights was also 1.00. The ranking was arranged according to the order of the global weight.
Global weight = value of the local weight within each main criteria × value of local weight within each sub-criteria (17)
3.1.2. Fuzzy Technique for Order Preference by Similarity to Ideal Solution Method
The Technique for Order of Preference by Similarity to Ideal Solution (TOPSIS) is a multi-criteria decision analysis method of compensatory aggregation that compares a set of alternatives by identifying weights for each criterion (Kore et al., Citation2017).
Fuzzy TOPSIS is based on the concept that the chosen alternative should have the shortest geometric distance from the positive ideal solution and the longest geometric distance from the negative ideal solution. Then, we use a new method to calculate the distance between two triangular fuzzy ratings. Using the idea of comparison between two fuzzy numbers, it can calculate the distance of each alternative from a fuzzy positive ideal solution and a fuzzy negative ideal solution, respectively. In other words, a new distance measure for Fuzzy TOPSIS is proposed. Finally, a closeness coefficient of each alternative is used to determine the ranking order of all alternatives. The higher value of the closeness coefficient indicates that an alternative is closer to the fuzzy positive ideal solution and farther from the fuzzy negative ideal solution simultaneously (Saghafian & Hejazi, Citation2005).
Under (Saeednamaghi & Zare, Citation2014) the steps for implementing the fuzzy TOPSIS methodology are described below.
Step 1: Determine the weighting of each evaluation criteria.
The study can employ fuzzy AHP to find the fuzzy preference weights; it is because the analysis was done by integrating fuzzy AHP and fuzzy TOPSIS methods.
Step 2: Construct the fuzzy decision matrix and choose the appropriate linguistic variables for the alternatives to criteria. The experts have their range for the linguistic variables employed in this study according to their subjective judgments.
Step 3. Aggregate the fuzzy judgment values of different evaluators regarding the same evaluation dimensions to get the aggregated fuzzy rating of alternative
under criterion
(Kore et al., Citation2017).
i = 1, 2, …, m; j =1,2, …,n
Xij = 1/k(Xij1 + Xij2 + Xij3 + XijK)
where Xij = is the rating of alternative Ai with respect to criterion Cj evaluated by K expert and
XijK = (lij, mij, uij)
Step 4: Normalize the fuzzy decision matrix
It is possible to avoid complex calculations; a linear normalization is used to convert the various measurement scales into comparable scales and that the range of each component of the normalized triangular fuzzy numbers lies within (Velmurugan & Dhingra, Citation2015). Therefore, it is possible to obtain the normalized fuzzy decision matrix denoted by Rij.
i = 1, 2, …, m; j =1, 2, …,n
Then the normalization process can be performed by using the formula:
,
and j = 1, 2 … . n is equal one.
Step 5: Construct the weighted normalized fuzzy decision matrix.
The weighted fuzzy normalized decision matrix is shown as following matrix :
Where ⊗
, ⊗ = Fuzzy multiplication
Step 6: Determine the fuzzy positive-ideal solution (FPIS) and fuzzy negative-ideal solution (FNIS). According to the weighted normalized fuzzy decision matrix, the elements are normalized positive triangular fuzzy numbers and their ranges belong to the closed interval (Velmurugan & Dhingra, Citation2015). Then, it can define the Fuzzy Positive Ideal Solution (FPIS, A+) and Fuzzy Negative Ideal Solution (FNIS, A−) as the following formula:
Where, 1, 1, 1) and
0, 0, 0), j =1, 2, …,n
Step 7: Calculate the distance of each alternative from FPIS and FNIS.
The distance of each alternative from
can be calculated as:
Where, d () is the distance measurement between two fuzzy numerical values
Let ā = (a1, a2, a3) and b = (b1, b2, b3) be two triangular Fuzzy numbers.
If =
then
=
= 1, 2, 3.
If is a triangular fuzzy number and
> 0 and
≤ 1 for A fuzzy set
(Velmurugan & Dhingra, Citation2015), then
is called a normalized positive triangular fuzzy number.
Let ā = (a1, a2, a3) and b = (b1, b2, b3) be two triangular Fuzzy numbers. The distance between them is given using the vertex method by (Kore et al., Citation2017):
Step 8: Calculate the closeness coefficient of each alternative.
The CCi is defined to determine the ranking order of all alternatives once the of each alternative have been calculated. Calculate similarities to ideal solutions. This step solves the similarities to an ideal solution by the formula:
Step 9: Ranking the alternatives
3.2. Sensitivity analysis
Sensitivity analysis is becoming increasingly widespread in many fields of engineering and sciences and has become a necessary step to verify the feasibility and reliability of a model or a method (Li et al., Citation2013).
Sensitivity analysis is a method for testing changes of the final order by a modification of the original input data or by small deviation of the original weights of the criteria.
TOPSIS does not test the effect of the weight settings, however. Sensitivity analysis is one of the ways to test the impact of weight adjustments.
Sensitivity analysis is proposed in the following steps (Jiří, Citation2018):
Perform standard TOPSIS while saving the matrices
and the resulting order of the alternatives for further use.
Where, ; k, p ∈ ℕ
Change one weight wp and adjust the other weights using the coefficient
.
Where, wp′ = wp + ∆p; p ∈ ℕ, ∆∈ ℝ, wp′ > 1
; k, p ∈ ℕ
Construct out of the changed values
=
(30)
Where is a coefficient of the correction all other weights except for the tested weight.
⊗
Calculate the new distances from the saved vectors.
Calculate the new relative closeness using the standard TOPSIS procedure.
Evaluate the new order of alternatives and check if it equals the original, saved order.
4. Case study: numerical analysis
4.1. Fuzzy analytic hierarchy process results
The main criteria, sub-criteria, and maintenance alternatives are represented in hierarchical structure of the decision problem, as shown in Figure . After the construction of the hierarchy, the different priority weights of each criteria, attributes and alternatives are calculated using the fuzzy AHP and fuzzy TOPSIS approaches. The comparison of the importance or preference of one criterion, attribute or alternative over another can be done with the help of the questionnaire. The method of calculating priority weights of the different decision alternatives is discussed below.
First, the decision-making team consisted of 22 decision experts that fill the judgment matrix about the relative importance of the criteria, and then the pairwise comparison matrices of criteria will be obtained. It can apply the fuzzy numbers defined in Table .
Calculating the consistency index and consistency ratio.
When the value of CR ≤10% indicates a good level of consistency in the comparative judgments represented in that matrix, it is acceptable. CR value greater than 10% has resulted in the inconsistency of judgments within that matrix.
This suggests that the evaluation process needs to be reviewed and repeated to improve the consistency of the questionnaire.
The consistency index (C.I.) and the consistency ratio (C.R.) for a comparison matrix are calculated using the following equations.
Where, n is the number of main criteria to be compared, is the largest eigenvalue of the comparison or judgmental matrix, and RI(n) is the random index based on n.
As an example, a fuzzy pairwise comparison matrix of the main criteria.
=5.238, n = 5, RI (n=5) = 1.12 (Vahidnia et al., Citation2008).
Therefore, the CI can be determined by using Equationequation (10)(10)
(10) , and the CR by using Equationequation (11)
(11)
(11) , of the pairwise judgmental matrix can be calculated as follows: CI = 0.092.
Thus, the judgmental matrix is acceptable.
The consistency ratios of all the other matrices are less than 10%. Thus, all the judgments are consistent.
Computing the elements of the aggregated pairwise comparison matrix of the criteria in the hierarchy was done through combining the collected data from all experts by using a geometric mean method, that is,
As a sample calculation, the aggregated fuzzy pairwise comparison values for the main criteria are shown in the following matrix Ă.
In order to determine the fuzzy geometric mean and fuzzy weights of the main criteria, the fuzzy geometric mean technique can be used in this paper by using the following equation.
Likewise, the remaining ri values are obtained as follows:
To determine the fuzzy weight of the main criteria, Equationequation (14)(14)
(14) is applied.
Similarly, the remaining fuzzy weights wi values are:
To apply the COA method to compute the BNP and BNPw values of the fuzzy weights of the main criteria: To take the BNP value of the weight of main criteria as an example, the calculation process is as follows:
Similarly, the BNP value of the remaining criteria and sub-criteria can be obtained in a similar computational procedure (see, Tables ).
Table 5. Fuzzy Linguistic terms and their numerical intervals for fuzzy TOPSIS (Basahel & Taylan, Citation2016)
Table 6. Fuzzy weights of the main-criteria with respect to the goal
Table 7. Fuzzy weights of the sub-criteria with respect to cost
Table 8. Fuzzy weights of the sub-criteria with respect to add value
Table 9. Fuzzy weights of the sub-criteria with respect to implementation
Table 10. Fuzzy weights of the sub-criteria with respect to Safety
Table 11. Fuzzy weights of the sub-criteria with respect to effectiveness
The normalized weights of the sub criteria placed at the third level in the hierarchy structure can be presented in Table .
Table 12. Weighted values and rankings considered by decision experts
4.2. Fuzzy TOPSIS Results
Step 1: Determine the weighting of evaluation criteria. The fuzzy preference weights for main criteria and sub-criteria are presented in Table .
Step 2: Construct the fuzzy-decision matrix and choose the appropriate linguistic variables for the alternatives with respect to criteria. This paper focuses on evaluation and selection of the best road maintenance management strategy determining the best road maintenance strategy, and the questionnaire has been collected completely and will start with building dataset that is collected. The evaluators have their own range for the linguistic variables employed in this study according to their subjective judgments. For each evaluator with the same importance, this study employs the method of average value to integrate the fuzzy/vague judgment values of different evaluators regarding the same evaluation criteria. The evaluators then adopted linguistic terms Table , including “Very Low Important”, “Low Important”, “Moderately Important”, “Highly Important” and “Extremely Important” to express their opinions about the rating of every person, based on the fuzzy data of the 22 decision experts are listed in Table .
Table 13. Linguistic terms and their numerical intervals for fuzzy TOPSIS (Basahel & Taylan, Citation2016)
Step 3: Normalize the fuzzy decision matrix
Using EquationEquation (18)(18)
(18) , it can normalize the fuzzy decision matrix as it was presented in Table .
Table 14. Aggregated fuzzy decision matrix for alternative by decision experts
Table 15. Normalized fuzzy decision matrix for alternative by decision experts
Step 4: Establish the weighted normalized fuzzy decision matrix.
The fourth step in the analysis is to find the weighted fuzzy decision matrix, and the resulting
Fuzzy weighted decision matrix is shown as Table .
Table 16. Weighted normalized fuzzy decision matrix by decision experts
Step 5: Determine the fuzzy positive and fuzzy negative ideal reference points.
Then, the fuzzy positive-ideal solution (FPIS) and the fuzzy negative-ideal solution (FNIS) as: A+ and A−. This is the fifth step of the fuzzy TOPSIS analysis.
A+ = [(1, 1, 1)]
A− = [(0, 0, 0)]
Step 6: Ranking the alternatives.
Once the distances of Alternatives from FPIS and FNIS are determined, the closeness coefficient can be obtained by using Equationequation (24)(24)
(24) .
In order to calculate the closeness coefficients of each of the alternatives and
calculation is used as an example as follows: The closeness coefficient of the first alternative is calculated as:
As a result of the above Fuzzy TOPSIS steps, closeness coefficients, CCi, of the seven alternatives come out to be 0.220, 0.187, 0.185, 0.181, 0.157, 0.151, and 0.142, respectively. Hence, the ranking order for periodic maintenance is higher than others, that is, periodic maintenance is the best choice considering the given criteria. The closeness coefficient scores for alternatives are numeric values and can be further utilized to indicate the degree of inferiority or superiority of the alternatives with respect to each other. In the contrary, the ranking order for reliability-centered maintenance and predictive maintenance is lower than others, which indicates that the identified maintenance management strategy cannot be provided to achieve the business objectives and the company strategic goals based on the identified selection criteria.
4.3. Validation of the proposed methodology
The analysis of the proposed methodology was done by using sensitivity analysis depending on the procedure as it was described in Section 3 to validate the robustness of the proposed methodology. The new weights for the proposed approach use EquationEquations (27(27)
(27) , Equation28
(28)
(28) , Equation29
(29)
(29) ) in cycles for sensitivity testing of each weight. The recalculated new weighted normalized fuzzy decision matrix was determined by using Equation (30). The new distances and the closeness coefficient of the alternatives were determined by using the standard TOPSIS procedure as it was presented in Table .
Table 17. Closeness coefficients and ranking
Table 18. Closeness coefficients and ranking by sensitivity analysis
The results show that there is a variation in the ordering of the alternatives based on the analysis made on sensitivity analysis to provide a robust output of TOPSIS to test the impact of weight adjustments in the alternatives. Based on the analysis made on sensitivity analysis, it can show that there were adjustments that were made on the ranking of the alternatives. However, periodic maintenance was ranked first based on the results of Fuzzy TOPSIS and sensitivity TOPSIS methods.
Therefore, from the alternative evaluation results in Table , the best alternative is periodic maintenance management strategies. This shows that periodic maintenance was the most sensitive alternative. Thus, periodic maintenance is identified as the appropriate road maintenance management strategy in the Ethiopian Roads Authority.
5. Discussions, managerial implications, and limitations
5.1. Discussion of Results
In this study, a set of 21 road maintenance selection criteria were considered. This study explored the appropriate set of selection criteria and maintenance strategy for fuzzy AHP in the case of the Ethiopian Roads Authority.
The combined methodology of Fuzzy AHP and Fuzzy TOPSIS helps to determine the appropriate selection criteria and maintenance strategy as it is presented in Figure . The decision experts weighted the value of the main criteria and sub-criteria as it is presented in Table . As it was observed in Table , the decision experts compared local weights in each main criterion and ranked add value (0.353) and cost (0.254) as the first and second most important maintenance strategy selection criteria. Thus, decision experts believe that adding value and cost should be viewed as the most important in road maintenance management strategy selection criteria. This indicates that the decision experts focus on achieving their business objectives and minimizing extra costs incurred for executing maintenance by considering these criteria. Safety (0.164) is the next most important selection criterion identified by the decision experts followed by the implementation (0.136). Contrariwise, effectiveness (0.093) was the least important selection criterion. This result indicates that the perceptions of the decision experts are influenced by the priority weights of add value, and cost overshadows this criterion in maintenance strategy selection.
Accordingly, a similar decision was made through document analysis, by using the available maintenance manual documents and technical specifications. Besides these selection criteria, efficiency and workmanship requirement should be considered in maintenance strategy selection by the Ethiopian road authority. Quality control and payment made for each type of maintenance strategy were identified from the document review. Individually, further examining each sub-criterion under the main criteria, the highest and most important selection criteria among the sub-criteria for cost category were production cost (0.251) followed by training (0.249), maintenance labor cost (0.195), and cost of inventories (0.168). On the contrary, the cost of advising and consulting (0.137) are the least important criteria. The greatest weighted value under add value criteria was quality (0.588) followed by maintenance time (0.227) and profit (0.186).
Furthermore, the desire of workers (0.234), the desire of top management (0.221), and access to equipment and technology (0.231) were identified as the most important selection criteria by the experts. Contrariwise, skills and competency development (0.198) and work order management (0.116) were identified as the least important selection criteria.
This finding can be supported by Tadele (Citation2007) that quality maintenance is aimed towards customer delight, highest quality through defect-free maintenance. Accordingly, quality maintenance will meet the expectations of the customer (Labi et al., Citation2003).
Moreover, the greatest importance of sub-criteria under the safety criteria were environment (0.398) and facilities (0.368).
Contrariwise, personnel safety (0.136) and safety training (0.098) are the least important selection criteria identified by the decision experts. Finally, the results show that the value for employee satisfaction (0.399) and functionality (0.385) were the important sub-criteria in the effectiveness (0.093) category in maintenance. On the contrary, reliability (0.127) and maintainability (0.089) were identified as the least important selection criteria.
Overall, the sub-criteria with the five highest-ranked final weights among global weights were quality (0.208), maintenance time (0.080), profit (0.066), environment (0.065), and production cost (0.064). In this study, seven road maintenance management strategies were considered. This study explored the appropriate road maintenance strategy for the fuzzy TOPSIS model in the case of the Ethiopian Roads Authority.
Table shows the closeness coefficient and ranking order of the road maintenance management strategies selected by the Ethiopian Roads Authority and considered by decision experts. As it was observed in Table , the most appropriate road maintenance management strategy in Ethiopian road authority which is ranked first as perceived by the experts was periodic maintenance with a closeness coefficient value higher than other alternatives. Hence, the results show that the higher value of the closeness coefficient was obtained for periodic maintenance which is closer to the ideal values for fuzzy TOPSIS and sensitivity Fuzzy TOPSIS.
Contrariwise, the lower value of the closeness coefficient was obtained for reliability-centered maintenance and predictive maintenance. Reliability-centered maintenance management strategies were ranked as the sixth road maintenance management strategy by experts. Predictive maintenance management strategies were ranked as the seventh road maintenance management strategy. This indicates that the closeness coefficients of reliability-centered maintenance and predictive maintenance management strategies were far from the ideal value. Therefore, periodic maintenance was selected as the best road maintenance strategy among the considered ones, which have the best closeness coefficient value by decision experts.
5.2. Managerial Implications
The outcomes of this research provide some significant implications for maintenance managers and the company in general. The maintenance managers ought to be conscious of the various magnitudes of maintenance in the road maintenance strategies and the implication that they have towards the attainment of maintenance goals. They ought to appreciate that scientific and appropriate strategy selection tools for maintenance can significantly enhance the quality of their maintenance decisions and reduce the errors made in over-or-under-estimating decision variables.
The research again provides many important practical implications to make maintenance policies and strategies for the maintenance company. The outcomes of this research prove that although it is necessary to engage in periodic maintenance strategy, it was identified as the best for the upkeep of the road by considering maintenance time, quality, profit, and maintenance environment.
5.3. Limitations
In the course of conducting this research, some knowledge was gained, which reveals the limitation of the techniques or methodologies in this research article. With this insight, it is worthwhile to outline additional methods that could be pursued in the future to extend the frontier of knowledge concerning road maintenance strategy. Here there are some limitations with existing techniques or methods that affect road maintenance management strategy evaluation and selection.
The first deficiency of the published paper is the unavailability of adequate documents for getting additional information on road maintenance selection criteria, and road maintenance strategy. Second, during the criteria selection process, in most cases, a large number of sub-criteria was considered for selection purposes, which requires high computational requirements. This also makes the developed fuzzy model more complex and time-consuming as far as the formulation of the historical database is concerned. Few studies would be conducted on the applied methodologies of fuzzy-based approaches; in the literature, through the use of fuzzy set theory is the hot research topic. Thus, the method can be used other than the knowledge area. This paper looks forward to road maintenance management strategy evaluation and selection in the Ethiopian Roads Authority among the different management aspects.
The other limitation of the proposed methodology is that it uses a limited number of decision experts for carrying out the study. Thus, the generalizability of this might be limited. Finally, in this research article, fuzzy concept has been applied. However, several other fuzzy theories may be applied to make a comparison of the results and to check the consistency of the results.
6. Conclusions and remarks
This research aims to construct a fuzzy AHP and fuzzy TOPSIS model for the evaluation and selection of appropriate road maintenance management strategies in the Ethiopian Roads Authority. The importance of the criteria for the selection of the appropriate road maintenance management strategy is evaluated by the decision experts, and the uncertainty of human decision-making is taken into account through the fuzzy concept in a fuzzy environment. From the proposed method, fuzzy AHP helps to find out that add value and maintenance cost can be identified as the most important road maintenance strategy selection criteria. The first five important road maintenance strategy selection criteria for the Ethiopian Roads Authority are quality (0.208), maintenance time (0.080), profit (0.066), environment (0.065), and production cost (0.064). On the other hand, maintainability (0.008) and reliability can be identified as the least important selection criteria and last priorities among these selection criteria. Moreover, fuzzy TOPSIS methodology helps to determine the best maintenance alternatives.
The finding of the study revealed that the periodic maintenance strategy is identified as the best and the most appropriate road maintenance management strategy by the Ethiopian Roads Authority. This might be the fact that it applies to the conditions and environment even in other countries. This is because it considers complex sustainability statistical road assessment that considers in other countries or regions. Contrariwise, reliability-centered maintenance and predictive maintenance management strategies were identified as the least important road maintenance management strategies in the company. Moreover, this approach provides a more accurate, systematic, and rational decision-support tool. Further study can apply the other prioritization techniques for finding the weight of criteria and maintenance strategy under a fuzzy environment and the results can be compared with the present method.
Finally, the findings of this study provide academicians and practitioners with insightful information on the introduction of integrated techniques in the construction industry to demonstrate its practicality would be the best area of investigation.
Data availability
The source of data used to support the findings of this study is available from the corresponding author upon request.
Disclosure statement
No potential conflict of interest was reported by the author(s).
Additional information
Funding
Notes on contributors
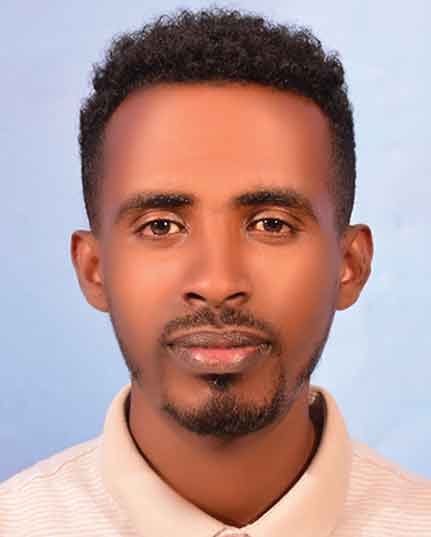
Girmay Getawa Ayalew
Girmay Getawa Ayalew is a lecturer at the Gondar Institute of Technology, University of Gondar, P.O. Box 196, Gondar, Ethiopia. He received his MSc degree in Construction Engineering and Management from the University of Gondar, Gondar, Ethiopia. His research interest includes Road Maintenance, FMDMM, and Project management.
Meseret Getnet Meharie
Meseret Getnet Meharie (Ph.D.) is a senior lecturer at Adama Science and Technology University, Adama, Ethiopia. He is an Assistant professor and also he is a guest lecturer at other universities, in Ethiopia. His research interest includes BIM, Cost Estimation of Construction Projects, AHP, Machine learning algorithm in predicting the cost of highway projects, and simulation. E-mail: [email protected]
Betelhem Worku
Betelhem Worku is a lecturer at the Gondar Institute of Technology, University of Gondar, Gondar, Ethiopia. She received her MSc degree in Construction Engineering and Management from the University of Gondar, Gondar, Ethiopia. Her research interest includes Maintenance Management and Concrete quality management. E-mail: [email protected]
References
- Abay, A., Barbieri, G., & Woldearegay, K. (2019). GIS-based landslide susceptibility evaluation using Analytical Hierarchy Process (AHP) approach: The case of Tarmaber district, Ethiopia. 11(1), 14–35.
- Afefy, I. H. (2010). Reliability-centered maintenance methodology and application: A case study. Engineering, 02(11), 863–873. https://doi.org/10.4236/eng.2010.211109
- Annals, S. (2017). Ranking alternatives by pairwise comparisons matrix and priority vector Jaroslav ramík *. 64, 85–95. https://doi.org/10.1515/saeb-2017-0040
- Azadeh, A., & Zadeh, S. A. (2015). An integrated fuzzy analytic hierarchy process and fuzzy multiple-criteria decision-making simulation approach for maintenance policy selection. (november). https://doi.org/10.1177/0037549715616686
- Basahel, A., & Taylan, O. (2016). Using fuzzy ahp and fuzzy topsis approaches for assessing safety conditions at. 6(4), 728–745. https://doi.org/10.2495/SAFE-V6-N4-728-745
- British Standards Institution (BSI). (2010). BSI standards publication maintenance maintenance terminology.
- Enofe, O. M. (2009). Improving maintenance perception in developing countries – A case study. 1–55.
- Fu, H., Chen, Y., & Wang, G., “Using a fuzzy analytic hierarchy process to formulate an E ff ectual tea assessment system,” 2020.
- Gade, P. K. (2014). Evaluation of multi criteria decision making methods for potential use in application security.
- Galar, D., Sandborn, P., & Kumar, U. (2017). Maintenance performance measurement. Maint. Costs Life Cycle Cost Anal, 191–268. https://doi.org/10.1201/9781315154183-4
- Hivarekar, N., Jadav, S., Kuppusamy, V., Singh, P., & Gupta, C. (2020). Preventive and predictive maintenance modeling. Proceedings, Annual Reliability and Maintainability Symposium, 2020-Janua, 1–23. https://doi.org/10.1109/RAMS48030.2020.9153636
- Huang, H., & Ho, C. (2016). Applying the fuzzy analytic hierarchy process to consumer decision-making regarding home stays. February 2013, 2016. 5. https://doi.org/10.4156/ijact.vol5.issue4.119
- Illeris, K. (2012). International perspectives on competence development: Developing skills and capabilities. International Perspectives on Competence Development: Developing Skills and Capabilities, (May), 1–228. https://doi.org/10.4324/9780203523032
- Jafari, A., Jafarian, M., Zareei, A., & Zaerpour, F. (2008). Using fuzzy Delphi method in maintenance strategy selection problem. 2(4), 289–298.
- Jiří, M. (2018). The robustness of TOPSIS results using sensitivity analysis based on weight tuning. IFMBE Proceedings, 68(2), 83–86. https://doi.org/10.1007/978-981-10-9038-7_15
- Karia, N. (2014). Exploring maintenance management in service sector: A case study. 3119–3128.
- Kazan, H., Karaman, E., & Akcali, B. Y. (2015). Assessment of TEOG examination success: TOPSIS multi-criteria decision-making method practice assessment of TEOG examination success: TOPSIS multi-criteria decision-making method practice. (July). https://doi.org/10.1016/j.sbspro.2015.06.371
- Kil, S., Lee, D. K., Kim, J., Li, M., & Newman, G. (2016). Utilizing the analytic hierarchy process to establish weighted values for evaluating the stability of slope revegetation based on hydroseeding applications in South Korea. https://doi.org/10.3390/su8010058
- Knezevic, J. . (2012). Quality of maintenance: Mirce Mechanics Axiom. Journal of Quality in Maintenance Engineering, 18(2), 216–226. https://doi.org/10.1108/13552511211244238
- Kore, N. B., Ravi, K., & Patil, S. B. (2017). A simplified description of FUZZY TOPSIS method for multi criteria decision making. International Research Journal of Engineering and Technology, 4(5), 2395.
- Kou, G., Ergu, D., Lin, C., Chen, Y., & Group, F. (2017). Pairwise comparison matrix in multiple criteria decision making (Vol. 4913). https://doi.org/10.3846/20294913.2016.1210694
- Kumar, M., Jayaswal, P., & Kushwah, K. (2013) Exploring fuzzy SAW method for maintenance strategy selection problem of material handling equipment. 2008, 600–605.
- Labi, S., Sinha, K., Transportation, J., Lafayette, W., Building, S. O., & Report, F. (2003). Fhwa/in/jtrp-2002/27 4. 7. 2.
- Li, P., Qian, H., Wu, J., & Chen, J. (2013). Sensitivity analysis of TOPSIS method in water quality assessment: I. Sensitivity to the parameter weights. Environmental Monitoring and Assessment, 185(3), 2453–2461. https://doi.org/10.1007/s10661-012-2723-9
- Literate, S., & Indonesia, J. I. (2020). “View metadata, citation and similar papers at core.ac.uk,” pengaruh pengguna. pasta labu kuning (cucurbita moschata) untuk substitusi tepung terigu dengan penambahan tepung angkak dalam pembuatan mie kering, pp. 274–282
- Makinde, O., Mpofu, K., & Ramatsetse, B. (2016). “Establishment of the best maintenance practices for optimal reconfigurable vibrating screen management using decision techniques . International Journal of Quality & Reliability Management Article Information , 33(October 2017), 1239–1267. https://doi.org/10.1108/IJQRM-01-2016-0004
- Meharie, M. G., Gariy, Z. C. A., Ndisya Mutuku, R. N., & Mengesha, W. J. (2019). An effective approach to input variable selection for preliminary cost estimation of construction projects. Advances in Civil Engineering, 2019, 5–9. https://doi.org/10.1155/2019/4092549
- Milhem, W., Abushamsieh, K., & Pérez Aróstegui, M. (2014). Training strategies, theories and types. Journal Accounting Business and Management, 21(1), 12–26.
- Mkilania, J. N. (2016). Factors affecting best maintenance practice in Tanzania public sector. International Journal of Mechanical Engineering and Technology, 7(3), 139–149.
- Mohamed, O. M. (2005). Identifying the barriers affecting quality in maintenance within Libyan manufacturing organisations (public sector). (february). 260. Available http://usir.salford.ac.uk/id/eprint/2172/
- Momeni, M., Fathi, M. R., Zarchi, M. K., & Azizollahi, S. (2011). A fuzzy TOPSIS-based approach to maintenance strategy selection: A case study. 8(3), 699–706.
- Mong, S. G., Mohamad, S. F., & Misnan, M. S. (2018). Maintenance management model: An identification of key elements for value-based maintenance management by local authority. (August). https://doi.org/10.14419/ijet.v7i3.25.17467
- Mwanza, B. G., & Mbohwa, C. (2017). Safety in maintenance : An improvement framework. Procedia Manufacturing, 8(October 2016), 657–664. https://doi.org/10.1016/j.promfg.2017.02.084
- Nedevska, I., Ognjenović, S., Pustovgar, A., & Murgul, V. (2017). Review on the pavement-repairing measures as a condition for the choice of road-maintenance strategy. MATEC Web of Conferences, 106, 02023. https://doi.org/10.1051/matecconf/201710602023
- Parida, A., & Kumar, U. (2007). Maintenance productivity and performance measurement.
- Rastegari, A., & Salonen, A. (2015). Strategic maintenance management: Formulating maintenance strategy. International Journal of COMADEM, 18(1), 5–14.
- Saeednamaghi, S., & Zare, A. (2014). Fuzzy topsis algorithm for multi criteria decision. 3(1), 29–37.
- Sageer, A., Rafat, S., & Agarwal, M. P. (2012). Identification of variables affecting employee satisfaction and their impact on the organization. 5(1), 32–39.
- Saghafian, S., & Hejazi, S. R., “Multi-criteria group decision making using a modified fuzzy TOPSIS procedure,” Proc. - Int. Conf. Comput. Intell. Model. Control Autom. CIMCA 2005 Int. Conf. Intell. Agents, Web Technol. Internet, vol. 2, pp. 215–220, 2005, https://doi.org/10.1109/cimca.2005.1631471.
- Siew-Hong, D., & Kamaruddin, S. (2012). Selection of optimal maintenance policy by using fuzzy multi criteria decision making method. International Conference on Industrial Engineering and Operations Management, 435–443.
- Sun, C. C. (2010). A performance evaluation model by integrating fuzzy AHP and fuzzy TOPSIS methods. Expert Systems with Applications, 37(12), 7745–7754. https://doi.org/10.1016/j.eswa.2010.04.066
- Suzuki, T. (2018). Quality maintenance. TPM Process Lndustries, 235–260. https://doi.org/10.1201/9780203735312-7
- Tadele, T. (2007). Improvement of maintenance management system a case study on by. (July).
- Tarei, P. K., Thakkar, J. J., & Nag, B. (2018). A hybrid approach for quantifying supply chain risk and prioritizing the risk drivers: A case of Indian petroleum supply chain. Journal of Manufacturing Technology Management, 29(3), 533–569. https://doi.org/10.1108/JMTM-10-2017-0218
- Tran Anh, D., Dabrowski, K., & Skrzypek, K. (2018). The predictive maintenance concept in the maintenance department of the ‘industry 4.0’ production enterprise. Foundations of Management, 10(1), 283–292. https://doi.org/10.2478/fman-2018-0022
- Trojan, F. (2018,). “Proposal of Maintenance-types classification to clarify maintenance concepts in production and operations management proposal of maintenance-types classification to clarify maintenance concepts in production and operations management (Vol. July 2017). https://doi.org/10.15341/jbe(2155-7950)/07.08.2017/005
- Tucker, E. J. (2016). Strategy implementation in organizations: A conceptual overview. 14(Allio 2005), 205–218.
- Vahidnia, M. H., Alesheikh, A., Alimohammadi, A., & Bassiri, A. (2008). Fuzzy analytical hierarchy process in GIS application. International Archives of the Photogrammetry, Remote Sensing and Spatial Information Sciences, 37(B2), 593–596.
- Velmurugan, R. S., & Dhingra, T. (2015). Maintenance strategy selection and its impact in maintenance function: A conceptual framework. International Journal of Operations & Production Management, 35(12), 1622–1661. https://doi.org/10.1108/IJOPM-01-2014-0028
- Verbert, K., De Schutter, B., & Babu, R. (2017). Timely condition-based maintenance planning for multi-component systems * timely condition-based maintenance planning for. 19.
- Wang, T., & Lee, H. (2009). Expert systems with applications developing a fuzzy TOPSIS approach based on subjective weights and objective weights. Expert Systems with Applications, 36(5), 8980–8985. https://doi.org/10.1016/j.eswa.2008.11.035
- Wang, C. N., Yang, C. Y., & Cheng, H. C. (2019). A fuzzy multicriteria decision-making (MCDM) model for sustainable supplier evaluation and selection based on triple bottom line approaches in the garment industry. Processes, 7(7), 1–13. https://doi.org/10.3390/pr7070400
- Yamasaki, M. (1976). The construction industry in developing countries Fred Moavenzadeh professor of civil engineering Janet Ann Koch Rossow Technology Adaptation Program Massachusetts Institute of Technology Spring 1975 (Second Printing Spring 1976) the. 1975.
- Zafar, B. (2018). Effective maintenance strategy is key to success for maintenance optimization programs. Reliable Consultants, (November).