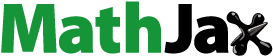
Abstract
Elastomers are the class of materials that are widely used in a variety of industrial, commercial, and consumer applications due to their unique mechanical properties, including high elasticity, high flexibility, and high resilience. However, there are many challenges in machining of elastomers such as poor surface finish, build up of heat, degradation of elastomers, etc. To overcome these challenges, cryogenic cooling assistance has been introduced as a means of improving the machinability of elastomers. This paper presents a soft computing approach for optimizing the surface roughness and cutting force during turning of elastomers under different lubrication conditions. Three types of elastomers, namely Nitrile Rubber (NBR), Polyurethane Rubber (PU), and Neoprene Rubber (CR), are studied, and a cryogenic fluid delivery system is employed to improve the machining process. Taguchi’s L27 array is used to vary the input parameters, and a Back-Propagation Artificial Neural Network (BPANN) model is developed to predict the cutting force and surface roughness. The cutting force and surface roughness are analyzed under different cooling conditions, cutting speeds, feeds, and depths of cut for various elastomers. The results show that changes in cutting conditions significantly affect the cutting force and that the type of lubrication used affects the cutting force by altering the material’s physical properties. Cutting force is significantly influenced by cutting conditions, and NBR requires the highest cutting force compared to PU and CR. Further, at a cutting speed of 55 m/min, a feed of 0.11 mm/rev, and a depth of cut of 0.25 mm, the cutting force for NBR (85.1 N), while for PU (75.1 N) and CR (80.3 N), respectively. Finally, with LN2 lubrication conditions, the Cutting Force decreased by 45% and Surface Roughness decreased by 16.9%. This study provides insights into the factors affecting the elastomer machining process, which can be useful for optimizing the machining process parameters and improving machining efficiency.
1. Introduction
Elastomers are a class of polymeric materials that are characterized by their ability to undergo large deformations and return to their original shape after the stress is removed (Mirza, Citation2016). These materials are widely used in a variety of industrial, commercial, and consumer applications due to their unique mechanical properties, including high elasticity, flexibility, and resilience (Ting, Citation2010). One of the most common applications of elastomers is in the manufacture of rubber products, such as tires, hoses, seals, and gaskets (Heinrich & Gurin, Citation2014). Elastomers are also used in the production of adhesives, coatings, and composites, as well as in the medical and pharmaceutical industries (Rehak, Citation2014). The properties of elastomers are dependent on their chemical composition and molecular structure. Generally, elastomers have a low modulus of elasticity, high elongation at break, and low tensile strength (Hager & Schubert, Citation2016). The mechanical behavior of elastomers is often described by their stress-strain curves, which exhibit a nonlinear relationship between stress and strain (Urban, Citation2012). Elastomers can be classified into three main types: thermosetting, thermoplastic, and thermoplastic elastomers (TPEs) (Bhattacharya, Citation2014). Thermosetting elastomers, such as natural rubber, undergo irreversible cross-linking when heated, while thermoplastic elastomers, such as styrene-butadiene-styrene (SBS) copolymers, can be melted and reshaped multiple times (Ghosh & Arya, Citation2007b). TPEs are a hybrid of the two and exhibit both thermoplastic and elastomeric behavior (Bridgwater, Citation1999). In summary, elastomers are a versatile class of materials with unique mechanical properties that make them ideal for a wide range of applications. Their chemical composition and molecular structure can be tailored to suit specific requirements, and their use is expected to continue to grow in the coming years (Hillmyer & Long, Citation2015). The machinability of elastomers is an important consideration in many industrial applications, particularly those involving the fabrication of rubber products. Due to their high elasticity and low tensile strength, elastomers can be difficult to machine using conventional cutting methods (Ghosh & Arya, Citation2007a). The cutting process can cause heat to build up, leading to thermal degradation of the material and reducing the quality of the final product (Crawford & Scott, Citation2012). To overcome these challenges, cryogenic cooling assistance has been introduced as a means of improving the machinability of elastomers (Joshi & Sheikh, Citation2003b). Cryogenic cooling involves the use of liquid nitrogen or carbon dioxide to cool the cutting tool and workpiece during machining, reducing the temperature and mitigating the effects of heat buildup (Joshi & Sheikh, Citation2003a). Cryogenic cooling can have several benefits for the machining of elastomers, including improved surface finish, reduced tool wear, and increased productivity (Pandey et al., Citation2012). Additionally, cryogenic cooling can help to minimize the risk of thermal damage to the material, allowing for more precise and consistent cuts (Lawal, Citation2005). Despite these advantages, cryogenic cooling is not without its drawbacks. The equipment required can be expensive, and the process can be time-consuming and difficult to implement in some settings (Coolegem & Kruth, Citation2005). Furthermore, cryogenic cooling can pose safety hazards, as the extremely low temperatures involved can cause skin and eye damage if proper precautions are not taken (Hadfield & Jain, Citation2004). In summary, the machinability of elastomers can be challenging due to their unique mechanical properties, but cryogenic cooling assistance has emerged as a promising solution. Cryogenic cooling can improve the quality of the final product and increase productivity while minimizing the risk of thermal damage. To optimize and predict the process parameters numerous statistical and soft computing approaches are available. Taguchi’s Design of Experiments (TDOE) offers a systematic approach to analyse multiple factors, their interactions, impact on output characteristics using Orthogonal Arrays and Signal to Noise ratios (Hegde et al., Citation2022; Jayashree et al., Citation2018; Karthik et al., Citation2022; Shetty et al., Citation2022). It efficiently identifies significant parameters further reducing the number of trials. However, the advent of Machine Learning has revolutionized machining optimization. Artificial Neural Networks (ANNs) possess the ability to discern intricate relationships within extensive datasheets, making them highly suitable for predicting machining parameters (Cay et al., Citation2012; Kara et al., Citation2020, Citation2023). This paper presents a soft computing approach for optimizing the surface roughness and cutting force during turning of elastomers under different lubrication conditions. Three types of elastomers, namely Nitrile Rubber (NBR), Polyurethane Rubber (PU), and Neoprene Rubber (CR), are studied, and a cryogenic fluid delivery system is employed to improve the machining process. Taguchi’s L27 array is used to vary the input parameters, and a Back-Propagation Artificial Neural Network (BPANN) model is developed to predict the cutting force and surface roughness. The cutting force and surface roughness are analyzed under different cooling conditions, cutting speeds, feeds, and depths of cut for various elastomers.
2. Methodology
2.1. Materials
In this study, elastomers, namely, Nitrile Rubber (NBR), Polyurethane Rubber (PU), and Neoprene Rubber (CR) are used (Figure ). Figure presents the microstructure of workpiece materials. These elastomers are procured from RS Aurora Rubber Corporation, Secunderabad, Telangana, with dimensions of 50 mm diameter and 250 mm length. Carbide inserts are used as cutting tools (Table ) for machining elastomers. The selection of cutting tools is based on the experimental conditions, and the specifications of the inserts are chosen accordingly.
Figure 2. Microstructure of workpiece materials (a) Nitrile Rubber (b) Polyurethane Rubber (c) Neoprene Rubber.
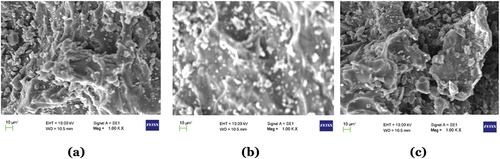
Table 1. Specifications of the cutting tool
2.2. Cryogenic fluid delivery system
Cryogenic cooling is used during turning of elastomers. A special nozzle delivery system (Figure ) is designed to deliver cryogenic cooling to the cutting zone. This system uses cryo-compatible steel pipe with insulation connected to the liquid nitrogen (LN2) tank. Compressed air is passed to the LN2 tank to aid the LN2 flow from the nozzle. The nozzle is placed in such a way that the jet of LN2 hits the cutting zone. Additionally, 1,1,1,2 Tetrafluoroethane is also used as coolant. During the machining process, the cutting forces are measured using a Kistler dynamometer which measures the force applied to the cutting tool during machining. The surface roughness of the machined surface is measured using the TalysurfSurtonic 3+ instrument with a range of 1.00 mm to 9.99 microns.
2.3. Design of experiments
The turning input parameters, such as Cutting Speed (m/min), Feed (mm/rev), Depth of Cut (mm), lubrication conditions (Dry, Tetrafluoroethane and LN2) and Elastomers (NBR, PU & CR) are varied using Taguchi’s L27 array. Taguchi’s L27 array allows for a systematic evaluation of the effects of each parameter and their interactions on the output parameters. By varying these parameters, the optimal combination of parameters can be identified which will lead to the best output parameters.
2.4. Back propagation artificial neural network
Based on the provided data, a BPANN (Back-Propagation Artificial Neural Network) model is developed to predict the cutting force and surface roughness during machining of elastomers. The input variables are cutting speed, feed, depth of cut, lubrication conditions, and elastomer material, and the output variables are cutting force and surface roughness. Figure presents the structure of BPANN model used. The BPANN model can be used to optimize the cutting process parameters for elastomer machining to achieve desired cutting force and surface roughness values. Sample code to train a BPANN (back-propagation artificial neural network) model in Python is given to predict the cutting force and surface roughness based on the input parameters provided (Figure ).
To develop the BPANN model, the following steps are followed:
Data preprocessing: The data is cleaned and preprocessed by removing any missing values or outliers. The categorical variables (lubrication conditions and elastomer type) are converted into numerical values using one-hot encoding.
Data splitting: The data is split into training and testing sets. The training set is used to train the BPANN model, while the testing set is used to evaluate its performance.
Model training: The BPANN model is trained using the training set. The model architecture is defined, and the hyperparameters are tuned to optimize the performance of the model.
Model evaluation: The performance of the BPANN model is evaluated using the testing set. The evaluation metrics are mean absolute error (MAE) and root mean square error (RMSE) for both cutting force and surface roughness.
Model deployment: Once the BPANN model is trained and evaluated, it is deployed to make predictions on new data.
The code uses the “MLPRegressor” class from scikit-learn to create BPANN models with one hidden layer of 27 neurons. The “Standard Scaler” class is used to standardize the input data and the “train_test_split” function is used to split the data into training and testing sets. Data with 20 sets of experimental results of Cutting Force (N) and Surface Roughness (microns) is used as training set while dataset with 9 sets of experimental results is used as test set to validate the BPANN model. Log’ Sigmoid is used as a transfer function and is presented in Equation 1.
where AVGx – weighted sum of input of xth processing elements and is denoted by Equation 2.
where (x, y)—processing elements; wxy – weight of connection; oy – output of yth processing element; wbi – bias weight;
Further, three different learning algorithms LM (Levenberg-Marquardt), GD (Gradient Descent), SCG (Scaled Conjugate Gradient) are compared with each other for best results by calculating respective RMSE (EquationEquation 3(3)
(3) ), R2 (EquationEquation 4
(4)
(4) ) and MEP (EquationEquation 5
(5)
(5) ) (Table ). The number of neurons was increased from 9 to 27 (9-18-27) in three steps to ensure the accuracy of the output.
Table 2. Statistical data for selection of learning algorithm
where T—Target Value; O—Output; N—Number of samples; Ty – predicted output; Oy – desired output.
3. Results and discussions
Studying the cutting force and surface roughness during turning of elastomers under different cooling conditions is crucial in identifying the optimal cooling conditions for cryogenic machining of elastomers. The results obtained from the analysis are important in optimizing the machining process parameters to achieve the desired cutting forces and improve the machining efficiency. Back Propagation Artificial Neural Network can be effectively used in optimization of the input parameters and prediction of output parameters. Optimization and prediction of cutting force and surface roughness during turning of elastomers (NBR, PU and CR) is further discussed.
3.1. Cutting force
The experimental values presented show the cutting force for different cutting conditions such as cutting speed, feed, depth of cut, and lubrication conditions for various elastomers. The cutting force is measured in Newton (N). The results of the cutting force experiment (Figures ) demonstrate that changes in cutting conditions significantly affect the cutting force. At a cutting speed of 55 m/min, a feed of 0.11 mm/rev, and a depth of cut of 0.25 mm, the cutting force is highest for NBR (85.1 N) compared to PU (75.1 N) and CR (80.3 N). NBR has a complex molecular structure which makes it more resistant to deformation, and a higher cutting force is generated. However, PU and CR are less resistant to deformation, resulting in a lower cutting force. Further, the type of lubrication used affects the cutting force by altering the material’s physical properties. When turning at a speed of 55 m/min, a feed of 0.25 mm/rev, and a depth of cut of 0.75 mm, using liquid nitrogen (LN2) lubrication, the cutting force is reduced compared to Dry and TFE (Tetrafluoroethane) lubrication conditions for all the elastomers.
From the Main Effects plot (Figure ) it can be concluded that Lubrication Conditions parameter has highest impact on Cutting Force. Further, at (125 m/min) Cutting Speed, (0.18 mm/rev) Feed, (0.5 mm) Depth of Cut and for PU elastomer material under LN2 cooling conditions lowest cutting force is observed. Figure shows the microscopic image and Figure presents the Cutting Force (N) signals of PU Elastomer Material under (125 m/min) cutting speed, (0.18 mm/rev) feed, (0.50 mm) depth of cut and LN2 Conditions. From ANOVA results (Table ), it can be seen that Lubrication Conditions have highest effect (79.03%) on the cutting force. Other parameters and their combinations are not considered to be significantly effecting the cutting force for the selected set of input parameters.
Figure 10. Microscopic image of PU elastomer material under (125 m/min) cutting speed, (0.18mm/rev) feed, (0.50 mm) depth of cut and LN2 conditions.
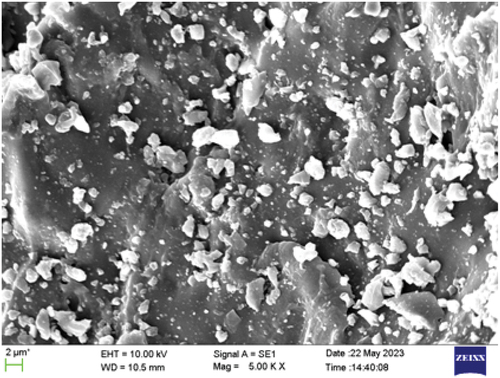
Figure 11. Cutting force (N) signals for PU elastomer material under (125m/min)cutting speed, (0.18mm/rev)feed, (0.50mm)depth of cut and LN2 conditions.
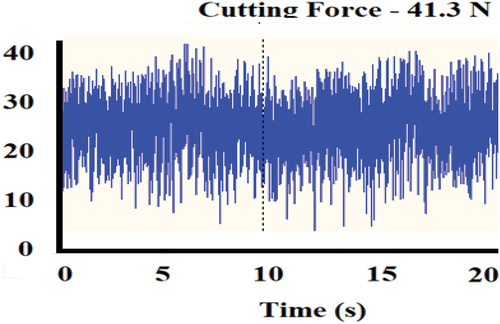
Table 3. ANOVA for cutting force (N)
3.2. Surface roughness
The surface roughness results from the experiment are presented in Figures . The lubrication conditions and elastomer material used in the experiment have a significant effect on surface roughness. When using dry lubrication conditions, the surface roughness was higher compared to TFE or LN2 conditions. Among the elastomers, NBR was found to have the highest surface roughness values compared to PU and CR. The depth of cut also has an impact on surface roughness. However, the cutting speed and feed did not show a significant influence on surface roughness. At the molecular level, surface roughness is influenced by several factors such as the plastic deformation of the material, the nature of the lubrication, and the contact between the cutting tool and the material. The physical properties of the material, such as its hardness and elasticity, also have an impact on surface roughness. Higher surface roughness values are often associated with greater plastic deformation of the material and a more significant transfer of material to the cutting tool, resulting in the formation of chips.
From the Main Effects plot (Figure ) it can be seen that lubrication conditions have significant impact on surface roughness compared to Cutting Speed, Feed, Depth of Cut and Elastomer Material. From the graph, we can observe that the Cutting Speed (125 m/min), Lubrication Conditions (LN2), and Elastomer Material (PU) have a significant impact on the Surface Roughness. The optimal levels for Feed and Depth of Cut are 0.18 mm/rev and 0.25 mm, respectively. Figure presents the microstructure of turned surfaces of the elastomers (NBR, PU and CR). From ANOVA results (Table ), it can be seen that Lubrication Conditions have highest effect (54.113%) on the surface roughness. Other parameters and their combinations are not considered to be significantly affecting the surface roughness for the selected set of input parameters.
Figure 16. Microstructure of turned surfaces of (a) Nitrile Rubber (NBR) (b) Polyurethane Rubber (PU) (c) Neoprene Rubber (CR) under (125m/min)cutting speed, (0.18mm/rev)feed, (0.50mm)depth of cut and LN2 cooling conditions.
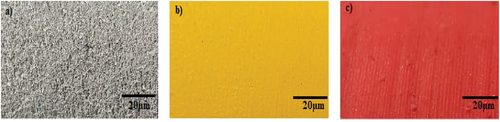
Table 4. ANOVA for surface roughness
3.3. Verification of experimental results using BPANN
The results of the experiment with different cooling conditions have been validated using a Back Propagation artificial neural network. Training dataset and Test dataset are provided in Tables . This network was trained with a learning factor of 0.7 and 5000 epochs, which resulted in accurate predictions of the cutting force and surface roughness. Table presents observations of output response. Figures presents validation of TDOE experimental output responses using BPANN.
Table 5. Training set for BPANN
Table 6. Test set for BPANN prediction
Table 7. Observations of output response
Based on the predictions, a regression equation for predicting Cutting Force and Surface Roughness is presented in Equation 6 and Equation 7, respectively. RMSE for Cutting Force (0.012564 training); (0.003489 test) and Surface Roughness (0.013415 training); (0.002598 test) along with MEP for Cutting Force (2.112144 training); (0.685894 test) and Surface Roughness (2.154877 training); (0.721296 test) justifies the prediction.
where A—Cutting Speed, B—Feed, C—Depth of Cut, D—Lubrication Condition, E—Elastomer Material.
Based on the percentage errors, it can be concluded that the BPANN model has successfully predicted the values of Cutting Force and Surface Roughness for a combination of input process parameters during turning of different elastomer materials under varying lubrication conditions.
3.4. Chip formation analysis
When turning elastomers, chips are produced as a result of the deformation and cutting of the material. The size and shape of the chips can vary depending on several factors, including the type of elastomer, the cutting conditions, and the cooling conditions. In this experimentation, the cooling conditions were varied, and the resulting chips were analyzed. Dry cooling conditions resulted in longer ribbon-like chips, which indicates that the material experienced more shearing and deformation before breaking. The elastomer materials also have a higher tendency to stick to the cutting tool, resulting in longer chips. Figure presents the formation of chips during turning of various elastomers under different cooling conditions, i.e. Dry, Tetrafluoroethane (TFE) and Liquid Nitrogen (LN2). Tetrafluoroethane cooling resulted in medium-long chips. This is due to the fact that it provides moderate cooling capacity, which allows for a balance between deformation and breaking. This cooling method also helps reduce the tendency of the material to stick to the cutting tool. Liquid nitrogen cooling resulted in fine chips. This is due to the fact that liquid nitrogen provides the most significant cooling capacity, which results in the material becoming more brittle and leads to rapid deformation. Liquid nitrogen is composed of nitrogen molecules in a liquid state at an extremely low temperature of around −196 °C. When applied to the elastomer material, this intense cooling causes the nitrogen molecules to absorb heat rapidly from the material’s surface, leading to a significant reduction in temperature. This extreme cold temperature induces changes in the material’s behavior at the atomic and molecular level. The molecular structures of elastomers consist of polymer chains held together by intermolecular forces, such as van der Waals forces and weak hydrogen bonds. These forces maintain the flexibility and resilience of elastomer. However, when subjected to the extreme cold of liquid nitrogen, the intermolecular forces weaken considerably. As a result, the elastomer’s molecular structure becomes more rigid and brittle. The reduced flexibility and increased brittleness of the elastomer material due to liquid nitrogen cooling lead to a fundamental change in its deformation behavior during the turning process. As the cutting tool interacts with the material, the weakened intermolecular forces and increased rigidity make it easier for the material to break apart along predetermined fracture planes. This controlled fracturing results in the formation of fine chips rather than longer, ribbon-like chips seen under other cooling conditions. This cooling method also helps reduce friction between the material and the cutting tool. The chip formation analysis during turning of elastomers provides an insight into the material’s properties and behavior under different cutting and cooling conditions.
4. Conclusions
From the experimental investigation and artificial neural network of cutting force and surface roughness of elastomers during cryogenic machining, the following conclusions are drawn:
Liquid nitrogen (LN2) lubrication reduces the cutting force for all the elastomers compared to dry and TFE lubrication. At a cutting speed of 55 m/min, a feed of 0.25 mm/rev, and a depth of cut of 0.75 mm, the cutting force was lowest when using LN2 lubrication. For NBR, PU, and CR, the cutting force is 61.3 N, 58.8 N, and 57.2 N, respectively. With LN2 lubrication conditions, the Cutting Force decreased by 45% and Surface Roughness decreased by 16.9%.
NBR has the highest surface roughness values compared to PU and CR. Further, dry lubrication results in higher surface roughness values than TFE or LN2 lubrication. The depth of cut also has a significant impact on surface roughness.
Liquid nitrogen cooling has resulted in formation of fine chips due to the most significant cooling capacity provided, which makes the elastomer material more brittle and leads to rapid deformation.
Back propagation artificial neural network was trained with a learning factor of 0.7 and 5000 epochs, which has resulted in accurate predictions of the cutting force and surface roughness values with 97.94% and 99.12% accuracy, respectively. LM learning algorithm has proven successful in predicting output with 27 neurons using MLP Regressor under BPANN architecture.
The utilization of liquid nitrogen cooling holds paramount significance in the process of turning elastomers, as it enables controlled chip formation by inducing brittleness in the material, reducing adhesion to cutting tools, minimizing friction and heat generation, and providing insights into material behavior. This results in the production of fine chips, enhancing machining precision, tool life, and overall process efficiency.
Disclosure statement
No potential conflict of interest was reported by the author(s).
References
- Bhattacharya, A. (2014). Introduction to elastomers. In Handbook of thermoplastic elastomers (2nd ed.) Elsevier.
- Bridgwater, A. V. (1999). Thermoplastic elastomers. In The polymer technology group handbook (2nd ed.) CRC Press.
- Cay, Y., Cicek, A., & Kara, F. (2012). Prediction of engine performance for an alternative fuel using artificial neural network. Applied Thermal Engineering, 37, 217–17. https://doi.org/10.1016/j.applthermaleng.2011.11.019
- Coolegem, B. W. T., & Kruth, J. P. (2005). Cryogenic machining of polymers and composites: A review. Journal of Materials Processing Technology, 167(2–3), 463–477.
- Crawford, R. J., & Scott, J. L. (2012). Machinability of polymers. In Polymer science: A comprehensive reference (pp. 334–338). Elsevier.
- Ghosh, S. K., & Arya, S. P. S. (2007a). Machining of elastomers. In Handbook of polymer testing (2nd ed.). John Wiley & Sons, Inc.
- Ghosh, S. K., & Arya, S. P. S. (2007b). Thermoplastic elastomers: Chemistry and properties. Journal of Macromolecular Science, Part C: Polymer Reviews, 47(4), 526–545.
- Hadfield, M., & Jain, A. (2004). Cryogenic machining of polymers and composites: Health and safety issues. Journal of Materials Processing Technology, 153-154, 582–588.
- Hager, M. D., & Schubert, U. S. (2016). Elastomers for Engineering: An overview. Macromolecular Materials and Engineering, 301(1), 10–20.
- Hegde, A. L., Shetty, R., Chiniwar, D., Naik, N., Nayak, R., & Nayak, M. (2022). Optimization and prediction of mechanical characteristics on vacuum sintered Ti-6Al-4V-SiCp composites using Taguchi’s Design of experiments, response surface methodology and random forest regression. Journal of Composites Science, 6(11), 339. https://doi.org/10.3390/jcs6110339
- Heinrich, G., & Gurin, A. L. (2014). Elastomer Technology. In Handbook of polymer science and technology (4th ed.). John Wiley & Sons, Inc.
- Hillmyer, M. A., & Long, T. E. (2015). Thermoplastic elastomers. In Encyclopedia of polymer science and technology. John Wiley & Sons, Inc.
- Jayashree, P. K., Sharma, S. S., Shetty, R., Mahato, A., & Gowrishankar, M. C. (2018). Optimization of TIG welding parameters for 6061Al alloy using Taguchi’s design of experiments. Materials Today: Proceedings, 5(11), 23648–23655. https://doi.org/10.1016/j.matpr.2018.10.154
- Joshi, S. S., & Sheikh, A. H. (2003a). Cryogenic machining of polymeric materials: Experimental investigations. International Journal of Machine Tools and Manufacture, 43(3), 301–312.
- Joshi, S. S., & Sheikh, A. H. (2003b). Cryogenic machining of polymers and composites: A review. Journal of Materials Processing Technology, 142(2), 738–753.
- Kara, F., Bayratkar, F., & Savas, F. (2023). Experimental and statistical investigation of the effect of coating type on surface roughness, cutting temperature, vibration and noise in turning of mold steel. Journal of Materials and Manufacturing, 1, 31–43.
- Kara, F., Karabatak, M., Ayyildiz, M., & Nas, E. (2020). Effect of machinability, microstructure and hardness of deep cryogenic treatment in hard turning of AISI D2 steel with ceramic cutting. Journal of Materials Research and Technology, 9(1), 969–983. https://doi.org/10.1016/j.jmrt.2019.11.037
- Karthik, S. R., Londe, N. V., Shetty, R., Nayak, R., & Hegde, A. (2022). Optimization and prediction of hardness, wear and surface roughness on age hardened stellite 6 alloys. Manufacturing Review, 9(2), 10. https://doi.org/10.1051/mfreview/2022008
- Lawal, G. A. (2005). Cryogenic machining of polymers: A review. Journal of Materials Processing Technology, 169(3), 477–489.
- Mirza, P. (2016). Elastomers. In Reference module in materials science and materials engineering. Elsevier.
- Pandey, P. M., Dwivedi, D. K., & Lal, M. (2012). An overview of cryogenic machining of elastomers. International Journal of Advanced Manufacturing Technology, 62(5–8), 529–541.
- Rehak, P. (2014). Elastomers in medical applications. In Handbook of thermoplastic elastomers (2nd ed.). Elsevier.
- Shetty, R., Gurupur, P. R., Hindi, J., Hegde, A., Naik, N., Ali, M. S. S., Patil, I. S., & Nayak, M. (2022). Processing, Mechanical characterization and electric discharge machining of stir cast and spray forming based al-Si alloy reinforced with ZrO2 particulate composites. Journal of Composites Science, 6(11), 323. https://doi.org/10.3390/jcs6110323
- Ting, K. C. K. (2010). Elastomers and Rubber elasticity. In The Wiley encyclopedia of packaging technology. John Wiley & Sons, Inc.
- Urban, M. W. (2012). “Elastomers,” in Polymer Science: A comprehensive reference. Elsevier.