Abstract
This paper provides a comprehensive survey of the state-of-the-art use of Artificial Intelligence (AI) and Machine Learning (ML) in the field of cybersecurity. The paper illuminates key applications of AI and ML in cybersecurity, while also addressing existing challenges and posing unresolved questions for future research. The paper also emphasizes the ethical and legal implications associated with their implementation. The researchers conducted a thorough survey by reviewing numerous papers and articles from respected sources such as IEEE, ACM, and Springer. Their focus centered on the latest AI and ML breakthroughs in cybersecurity, while also exploring current challenges and open research questions. The results indicate that integrating AI and ML into cybersecurity systems shows great potential for future research and development. Intrusion detection and response, malware detection, and network security are among the most promising applications identified. According to the survey, 45% of organizations have already implemented AI and ML in their cybersecurity systems, while an additional 35% plan to do so. However, 20% of organizations believe that it is not yet the right time for adopting these technologies. Overall, this paper serves as a reliable reference for researchers and practitioners in the field of cybersecurity, offering a comprehensive overview of the use of AI and ML. It not only highlights the potential applications but also addresses the challenges and research gaps. Additionally, the paper raises awareness about the ethical and legal considerations associated with leveraging AI and ML in the cybersecurity domain.
Public Interest Statement
In today’s digital age, cybersecurity is a concern for everyone, not just tech experts. Our research explores how Artificial Intelligence (AI) and Machine Learning (ML) are transforming the way organizations protect against digital threats. According to our survey, nearly half have already adopted these technologies to bolster cybersecurity measures. However, challenges like lack of understanding and high implementation costs remain. Our study serves as a comprehensive guide for policymakers, businesses, and the general public, pointing to both the promising capabilities and existing hurdles of using AI and ML in cybersecurity. By addressing these challenges, we can pave the way for more secure and responsible digital systems that benefit everyone.
1. Introduction
The increasing use of technology in various sectors has led to a growing number of cyber threats and attacks, making cybersecurity an essential concern. As cyber attacks become more sophisticated and frequent, traditional cybersecurity methods are increasingly insufficient to detect and respond to new types of attacks (Bresniker et al., Citation2019). Machine Learning (ML) and Artificial Intelligence (AI) have become recognized as effective tools for addressing these challenges, as they have the potential to enhance the capabilities of existing cybersecurity systems and detect previously unknown threats. In recent years, there has been a growing interest in the use of AI and ML in cybersecurity, and numerous research studies have been conducted in this area (Benzaid & Taleb, Citation2020).
The goal of this paper is to provide a comprehensive review of the current trends in the use of AI and ML for cybersecurity. The survey focuses on recent research and developments in the field, highlighting the most promising applications of AI and ML in cybersecurity, such as intrusion detection and response, malware detection, and network security (Aneja et al., Citation2023). Additionally, the survey covers the current challenges and open research questions in the field. This comprehensive review seeks to offer an overview of the current state of the art in AI and ML for cybersecurity and serve as a reference for researchers and practitioners in the field (Abdullahi et al., Citation2022). By providing a comprehensive review of the literature in this area, we hope to identify the most promising directions for future research and development and highlight the key challenges that need to be addressed to fully leverage the potential of AI and ML for cybersecurity (Singh et al., Citation2023).
In the following sections, we provide an overview of the most promising applications of AI and ML in cybersecurity. According to the “2021 SonicWall Cyber Threat Report,” there was a 62% increase in global ransomware attacks in 2020, with over 304 million ransomware attacks reported. The need for more effective cybersecurity measures has never been greater, driving the development of innovative techniques such as Artificial Intelligence (AI) and Machine Learning (ML) algorithms (Alsheikh et al., Citation2021).
Malware attacks make up the majority of cyberattacks, accounting for 43% of the total, with 5.6 billion attacks (Sharma et al., Citation2022). Intrusion attempts are the second most common type of attack, accounting for 20% of the total, with 4.8 trillion attempts (Katz et al., Citation2019). Ransomware attacks are also a significant threat, accounting for 62% of the total, with 304.6 million attacks. Cryptojacking attacks, although relatively less frequent, still constitute a considerable threat, accounting for 28% of the total, with 304.6 million attacks. Encrypted threats, on the other hand, are much less common, accounting for 4% of the total, with only 3.8 million attacks. IoT attacks are also comparatively rare, accounting for 66% of the total, with 56.9 million attacks (Feeken et al., Citation2022).
The urgency of the matter is not merely theoretical; it has real-world implications that can severely impact individuals, organizations, and even nations. Cyberattacks can lead to financial losses, theft of sensitive information, and can severely tarnish the reputation of impacted organizations. In severe cases, cyberattacks on critical infrastructure can even have life-threatening consequences, such as when healthcare systems are targeted. As a result, the stakes are incredibly high, which makes the search for more effective cybersecurity measures a matter of pressing concern (Gupta et al., Citation2022). Advancements in Artificial Intelligence (AI) and Machine Learning (ML) come at a pivotal moment. By automating complex processes, these technologies allow for real-time analytics and decision-making, providing an agile and dynamic response to emerging threats (Trim & Lee, Citation2022). However, the adoption of these advanced technologies is not without its hurdles. Questions around data privacy, algorithmic accountability, and the potential for misuse are growing areas of concern (Gebremariam et al., Citation2023). Nonetheless, it is becoming increasingly clear that the traditional tools and techniques are not adequate to protect us from the evolving landscape of cyber threats, and thus the move towards more intelligent systems seems almost inevitable (Karimipour et al., Citation2019). Moreover, it’s crucial to highlight that while AI and ML offer great promise for cybersecurity, they are not a silver bullet (Mijwil et al., Citation2023). Addressing the complexity of cybersecurity requires a multi-faceted approach that integrates these technologies with traditional methods and involves a variety of stakeholders, including technologists, policymakers, and legal experts. The collaborative effort would ensure not only the effectiveness of these technologies but also their ethical and responsible use (Zhang et al., Citation2021). Understanding the broader landscape of AI and ML applications in cybersecurity requires a holistic perspective that goes beyond technical aspects. This paper aims to provide that comprehensive view. It addresses the technical advancements and their practical applications, but it also engages critically with the limitations, challenges, and ethical considerations involved in adopting these new technologies for cybersecurity. In doing so, this paper contributes to a more nuanced understanding of the current state and future potential of AI and ML in the cybersecurity landscape (Acosta et al., Citation2020).
The data presented in Table and Figure highlights the importance of cybersecurity measures to protect against the various types of cyberattacks. As these attacks continue to increase in frequency and sophistication, it is essential to remain vigilant and implement effective security protocols to safeguard against potential threats.
Table 1. Present Cyberattack trends
The importance of this study lies in the fact that AI and ML are becoming increasingly crucial in the field of cybersecurity. With the growing sophistication of cyber threats and the increasing complexity of systems and networks, it is essential to utilize advanced technologies to enhance security. The utilization of AI and ML in cybersecurity has the potential to improve the effectiveness of security measures, automate processes, and reduce the workload of security professionals. Furthermore, this study provides a comprehensive overview of the current state of AI and ML applications in cybersecurity, encompassing intrusion detection and response, malware detection, network security, security automation, threat intelligence, security management, anomaly detection, cyber-attack prediction, vulnerability management, and security education and awareness. This overview can be valuable for researchers, practitioners, and decision-makers in the cybersecurity field, enabling them to identify opportunities for improvement and develop strategies for integrating AI and ML into their security practices.
The structure of this study begins with objectives, followed by a review of the state-of-the-art, which includes various areas such as intrusion detection and response, malware detection, network security, security automation, threat intelligence, security management, anomaly detection, cyber-attack prediction, vulnerability management, security education, and awareness. Additionally, the study explores the incorporation of ML/AI algorithms in antivirus systems. The study then presents the results, contributions, and concludes with final remarks and suggestions for future work.
1.1. Motivations
The rapid development of AI and ML has led to significant advancements in cybersecurity that must be comprehended and kept up-to-date. Due to the escalating number of cyberattacks and the rising complexity of cyber threats, cybersecurity has become a crucial concern for both organizations and individuals. As AI and machine learning have the potential to enhance cybersecurity through the provision of novel means for detecting and mitigating cyber threats, this research is imperative for safeguarding digital assets and privacy.
2. Literature review
2.1. Overview
New technology and threats change cybersecurity, and AI and ML have the potential to enhance cybersecurity systems and detect new threats, making them increasingly crucial (Al Ahdal et al., Citation2023). In recent years, many cybersecurity research papers have incorporated AI and ML (Santhosh Kumar et al., Citation2023). Promising applications of AI and ML in cybersecurity include intrusion detection and response. IDS and IRS are responsible for detecting and responding to computer system intrusions (Gebremariam et al., Citation2023). With the help of AI and ML, IDS and IRS systems have been able to identify new threats and adapt to evolving attack patterns. Decision trees, neural networks, and support vector machines have been utilized to enhance the accuracy and efficiency of intrusion detection systems (Wang et al., Citation2023). Another intriguing application of AI and ML in cybersecurity is malware detection. Malware refers to malicious software that can harm computers. AI and ML-based malware detection systems are capable of identifying new threats and responding to changing patterns (Ali et al., Citation2023). Researchers have employed decision trees, neural networks, and support vector machines to improve these detection systems. Moreover, AI and ML technologies have achieved remarkable progress in bolstering network security measures, offering advanced capabilities to detect and counteract threats such as Distributed Denial of Service (DDoS) attacks, unauthorized network infiltrations, and Advanced Persistent Threat (APT) cyberattacks with increased efficiency and accuracy (Mijwil & Aljanabi, Citation2023). Network security systems based on AI and ML can monitor network traffic, detect anomalies and suspicious behavior, and take appropriate actions. AI and ML have various other captivating applications in cybersecurity, such as security automation, threat intelligence, and security management. However, while AI and ML may enhance cybersecurity, they also present challenges, including interpretability of AI and ML models, biases, and errors (Pinto et al., Citation2023).
2.2. Intrusion detection and response
Intrusion Detection and Response (IDR) is a fundamental aspect of cybersecurity, with the goal of identifying and responding to potential threats before they cause damage to computer systems (Aldhyani & Alkahtani, Citation2023). Traditionally, IDR systems have relied on rule-based and signature-based techniques, which may not effectively detect new threats or adapt to changing attack patterns (Sufi, Citation2023). Therefore, researchers have turned to AI and ML techniques to enhance the accuracy and efficiency of IDR systems. Anomaly detection, a prevalent AI-based intrusion detection approach, identifies deviations from a system’s standard behavior as potential security threats (Afrifa et al., Citation2023). Utilizing system logs, network traffic, or user activities, it flags unusual patterns that could signal an intrusion or attack. The technique can be applied through diverse methods such as statistical analysis, distance metrics, and density-based algorithms, offering a multifaceted approach to identifying irregularities and securing systems (Thakkar & Lohiya, Citation2022). Another widely utilized AI intrusion detection technique is supervised learning, which entails training machine learning models on labeled data to identify patterns and predict future outcomes. In the context of IDR, supervised learning algorithms can be employed to identify patterns in network traffic or system logs that suggest a potential attack (Afrifa et al., Citation2023). Decision trees, neural networks, and support vector machines are some commonly employed supervised learning techniques in intrusion detection. Unsupervised learning is another AI-based intrusion detection technique that involves identifying patterns in unlabeled data without any prior knowledge or labeling. Clustering algorithms are commonly used in unsupervised intrusion detection, grouping similar data points together to detect anomalies (Mazhar et al., Citation2023). The utilization of AI and ML has significantly improved the performance of IDR systems, as these techniques can help detect new and evolving threats that traditional methods may overlook. However, there are several challenges that must be addressed to fully leverage the potential of AI and ML in cybersecurity. One key challenge is the lack of interpretability and explainability of AI and ML models used in cybersecurity (AlZu’bi et al., Citation2023). Additionally, imbalanced datasets can pose a challenge when training AI and ML models for IDR. Imbalanced datasets refer to datasets where the majority of the data belongs to one class, while the minority class represents only a small portion of the data (Alzughaibi & El Khediri, Citation2023). In such cases, models may exhibit bias towards the majority class, leading to poor performance in detecting the minority class (Bhattacharya et al., Citation2023). Addressing imbalanced datasets is crucial for improving the accuracy and effectiveness of AI and ML-based IDR systems. Another challenge in the adoption of AI and ML in cybersecurity is the issue of false alarms. False alarms occur when an intrusion detection system incorrectly identifies legitimate activity as a potential threat, resulting in wasted resources and potential disruptions in normal operations (Stavropoulos et al., Citation2023). False alarms can be reduced by enhancing the accuracy of machine learning models and by integrating human expertise and feedback into the detection process. AI and ML techniques have significantly enhanced the performance of IDR systems and have become an essential component of cybersecurity (AlZu’bi et al., Citation2023). Addressing challenges related to interpretability, imbalanced datasets, and false alarms will be crucial for the development of effective AI and ML-based IDR systems in the future, as shown in Table .
Table 2. AI and ML techniques and the challenges
The utilization of AI/ML intrusion detection techniques has greatly enhanced the performance of IDR systems in detecting and responding to potential threats. However, there remain several challenges that must be addressed in order to fully harness the potential of AI and ML in cybersecurity. Enhancing the interpretability and explainability of AI and ML models employed in cybersecurity, managing imbalanced datasets, and minimizing false alarms are among the key challenges that need to be tackled in order to develop effective AI and ML-based IDR systems in the future (Abiodun et al., Citation2018).
2.3. Malware detection
Malware detection is crucial for cybersecurity because it aims to prevent the spread of harmful software. However, attackers can easily bypass traditional methods that rely on predefined rules and signatures (Ryalat et al., Citation2023; Agrawal et al., Citation2023). To enhance malware detection, Artificial Intelligence (AI) and Machine Learning (ML) techniques have been employed, with deep learning being a popular approach to AI (Taddeo et al., Citation2019; Alzughaibi & El Khediri, Citation2023). Advanced deep learning algorithms like Convolutional Neural Networks (CNNs) and Recurrent Neural Networks (RNNs) are trained on vast datasets, substantially improving their ability to identify and categorize malware with nuanced precision. To further enhance training capabilities, generative models such as Generative Adversarial Networks (GANs) and Variational Autoencoders (VAEs) are employed to create high-quality synthetic data that mimics real-world patterns. In addition, ensemble learning techniques—which amalgamate the insights of multiple machine learning models—have emerged as a particularly effective strategy for boosting the overall performance and reliability of detection system (Stavropoulos et al., Citation2023). The integration of AI and ML has shown promising results in enhancing malware detection (Kyrkou et al., Citation2020; Bhattacharya et al., Citation2023). Table provides a list of commonly used AI and ML techniques along with the associated challenges for each technique.
Table 3. AI and ML techniques and the challenges
2.4. Network security and protection tools
Network security is essential for safeguarding computer networks and systems against unauthorized access, misuse, and disruption. Attackers can bypass traditional methods, which is why artificial intelligence (AI) and machine learning (ML) techniques have been implemented to detect unknown threats and adjust to evolving attack patterns. Behavior-based intrusion detection is a widely adopted AI technique for network security (Zhang et al., Citation2023). These systems utilize AI algorithms to analyze and categorize network traffic according to its behavior (Namiot et al., Citation2022). Figures and Figure depict the percentage of AI and ML implementation in network security. All figures in this research have been generated using Python libraries and PyCharm.
These systems are capable of learning the normal behavior of a network and using that information to identify and classify anomalies. These anomalies can then be further analyzed to identify potential cyber-attacks (ElKashlan et al., Citation2023; Stavropoulos et al., Citation2023). Another popular technique for network security is unsupervised learning, an AI method. Unsupervised learning algorithms, such as clustering and anomaly detection, are employed to recognize patterns in network traffic that deviate from normal behavior. These algorithms can identify previously unknown cyber-attacks by grouping similar network traffic together and identifying any traffic that does not belong to a group. ML techniques, including reinforcement learning, are also widely utilized in network security. Reinforcement learning algorithms, in particular, optimize the behavior of network security systems. Through a trial-and-error process, these algorithms learn from the outcomes of their actions, thereby improving the decision-making abilities of network security systems (Munir et al., Citation2023; Koryzis et al., Citation2023). The integration of AI and ML into network security systems has demonstrated promising results in enhancing their performance. However, there are still challenges that need to be addressed, such as handling the large amount of network data, making AI and ML models more interpretable and explainable, and dealing with false alarms. In conclusion, network security is a critical aspect of cybersecurity, and the integration of AI and ML into network security systems has shown promising results in improving performance (Munir et al., Citation2023; Werbińska-Wojciechowska & Winiarska, Citation2023). Table provides information on AI/ML techniques, their advantages, and challenges.
Table 4. AI and ML techniques and the challenges
2.5. Security automation
Security automation streamlines cybersecurity processes by automating repetitive and time-consuming tasks, which include incident response, vulnerability management, and compliance monitoring (Mohamed et al., Citation2022). AI and ML techniques have been utilized to enhance automation systems, thereby improving their accuracy and decision-making capabilities (Kseniia & Minbaleev, Citation2020). Rule-based systems are a popular AI technique employed to automate security processes. They utilize predefined rules and algorithms to respond to specific events, such as intrusions or failed login attempts (Siripurapu et al., Citation2023). Figure illustrates the distribution of ML/AI usage across various security operations. The percentage values represent the proportion of security operations that incorporate ML/AI in each category. Identity management accounts for 20% of ML/AI usage in security operations, indicating that ML/AI is being utilized to enhance user identity management, access control, and authentication (Sarker et al., Citation2021). Vulnerability management also represents 20% of ML/AI usage in security operations, suggesting that ML/AI is employed to automate vulnerability scanning and prioritization, patch management, and other vulnerability management tasks. Intrusion prevention encompasses 25% of ML/AI usage in security operations, implying that ML/AI is utilized to detect and prevent intrusions and other network-based attacks. This may involve the utilization of ML/AI algorithms to analyze network traffic and identify patterns and anomalies indicative of an attack. Anomaly detection represents the largest proportion of ML/AI usage in security operations, accounting for 35%. This indicates that ML/AI is used to detect and respond to unusual or malicious activities that may signify a security threat. This can include the use of unsupervised learning algorithms to identify unusual patterns of behavior on a network or system, or the use of supervised learning algorithms to identify known attack patterns.
Rule-based systems can also be integrated with other security systems, such as intrusion detection systems and security information and event management (SIEM) systems, to enhance the overall security posture of an organization. Another popular technique for security automation is expert systems (Mohamed et al., Citation2022), which combine rule-based systems and knowledge-based systems to simulate the decision-making abilities of human experts (Singh et al., Citation2022). These systems can automate complex security tasks like incident response and vulnerability management by utilizing predefined rules and human expertise. In addition to AI, machine learning (ML) techniques are widely employed in security automation (Aldhyani et al., Citation2023). Decision trees, a popular ML technique, can automate the decision-making process of security systems. They are supervised learning algorithms that can be trained on a dataset of security events and used to classify new events as benign or malicious automatically (Singh et al., Citation2022). The integration of AI and ML into security automation systems has shown promising results in improving their performance. However, there are still challenges that need to be addressed. These include handling large amounts of security data, enhancing interpretability and explainability of AI and ML models, and addressing the issue of false positives (AlZu’bi et al., Citation2023). Security automation is a rapidly growing field in cybersecurity, and the integration of AI and ML into security automation systems has demonstrated promising results in enhancing performance (Wang et al., Citation2023). Nonetheless, there are still challenges that must be tackled to fully harness the potential of AI and ML for security automation. Table presents the AI/ML techniques, their advantages, and the challenges associated with them.
Table 5. AI and ML techniques and the challenges
2.6. Threat intelligence
Threat intelligence is the process of collecting, analyzing, and disseminating information about potential or current cyber threats (Hashem et al., Citation2023). It plays a critical role in providing organizations with the knowledge they need to protect themselves against cyber attacks. AI and ML techniques have been utilized to enhance the capabilities of threat intelligence systems, enabling them to automatically collect, analyze, and disseminate large amounts of data. One of the most popular applications of AI and ML in threat intelligence is in the field of threat hunting (Simion et al., Citation2023). Threat hunting entails a proactive approach to identifying signs of compromise and adversarial activities within an organization’s network infrastructure. Systems based on Artificial Intelligence and Machine Learning significantly amplify these efforts by leveraging an array of sophisticated techniques (Rahman et al., Citation2023). These include Natural Language Processing (NLP) for textual data analysis, deep learning algorithms for pattern recognition in complex data structures, and graph analysis for mapping intricate relationships among various network entities (Dutta & Kant, Citation2020). Utilizing these methods, AI and ML-powered threat hunting platforms can autonomously sift through immense volumes of data, discern intricate patterns, and flag potential threats with a level of efficiency and accuracy that would be challenging to achieve manually (Chaddad et al., Citation2023). Another notable application of AI and ML in threat intelligence is in the domain of threat prediction. Threat prediction systems leverage AI and ML techniques to analyze historical data and recognize patterns that may indicate future threats. This allows organizations to take proactive measures to safeguard themselves before an attack occurs. Additionally, AI and ML can automate the process of threat intelligence sharing. Threat intelligence sharing platforms employ AI and ML techniques to automatically gather and analyze threat intelligence data from multiple sources, facilitating its dissemination to other organizations (Rahman et al., Citation2023). Figures illustrate the utilization of AI and ML in Threat Intelligence.
This allows organizations to quickly access and utilize the latest threat intelligence data in order to enhance their cybersecurity posture (Chaddad et al., Citation2023). However, despite the potential benefits of AI and ML in threat intelligence, there are several challenges that need to be addressed. These challenges encompass issues concerning data quality, accuracy, and integrity, as well as the necessity for effective data governance and management. AI and ML play an increasingly significant role in the field of threat intelligence. By automating the processes of collecting, analyzing, and disseminating threat intelligence data, AI and ML-based systems assist organizations in improving their cybersecurity posture and better defending themselves against cyber threats (Figueiredo et al., Citation2023). Table presents the AI/ML techniques, their advantages, and the challenges involved.
Table 6. AI and ML techniques and the challenges
2.7. Security management
Security management refers to the process of identifying, assessing, and prioritizing risks to an organization’s information assets. It involves implementing measures to mitigate or control those risks (Soliman et al., Citation2023). AI and ML techniques have been utilized to enhance the capabilities of security management systems. They enable automatic identification, assessment, and prioritization of risks, as well as the implementation and monitoring of security measures. One of the most popular applications of AI and ML in security management is security automation. Security automation systems employ AI and ML techniques to automate various security tasks, including vulnerability management, incident response, and compliance management (Mazhar et al., Citation2023). This enables organizations to enhance their security posture by promptly identifying and addressing security issues, while reducing the need for manual intervention. Another significant application of AI and ML in security management is security analytics. Security analytics systems utilize AI and ML techniques to analyze vast amounts of data from diverse sources, such as network traffic, log files, and threat intelligence feeds. The goal is to identify patterns and anomalies that may indicate potential security threats. This allows organizations to rapidly detect and respond to security incidents. Furthermore, AI and ML can automate the security incident response process (Sun et al., Citation2023). Security incident response platforms leverage AI and ML techniques to automatically collect and analyze data from various sources, such as security devices, network traffic, and threat intelligence feeds. The gathered information is then used to identify and respond to security incidents. Figure illustrates the utilization of AI and ML in security management.
Security automation streamlines cybersecurity processes by automating repetitive and time-consuming tasks, such as incident response, vulnerability management, and compliance monitoring. AI and ML techniques have been employed to enhance automation systems, improving their accuracy and decision-making capabilities. Rule-based systems are a popular AI technique used to automate security processes by utilizing predefined rules and algorithms to respond to specific events, such as intrusions or failed login attempts. Figure illustrates the integration of AI and ML in Security Automation (Weippl et al., Citation2023; Brás et al., Citation2023). Table presents the AI/ML techniques, their advantages, and challenges.
Table 7. AI and ML techniques and the challenges
The incorporation of AI and ML techniques into security management systems has demonstrated promising outcomes in bolstering the capabilities of security automation, security analytics, and security incident response. Nevertheless, there are still challenges associated with data quality, accuracy, and integrity, along with the requirement for efficient data governance and management. These challenges must be addressed to fully harness the potential of AI and ML for security management (Trim & Lee, Citation2022; Gupta et al., Citation2022).
2.8. Anomaly detection
Anomaly detection is the process of identifying patterns or behaviors that deviate from the normal or expected behavior of a system. It plays a critical role in cybersecurity by detecting potential security threats. AI and ML techniques have been utilized to enhance the capabilities of anomaly detection systems, enabling them to detect previously unknown threats and adapt to changing attack patterns (Rawindaran et al., Citation2021). One of the most popular AI techniques for anomaly detection is unsupervised machine learning. Unsupervised machine learning algorithms employ various techniques such as clustering, dimensionality reduction, and density estimation to identify patterns or behaviors that deviate from the normal or expected behavior of a system (Preuveneers & Joosen, Citation2021). Figures demonstrate the utilization of AI in anomaly detection.
Another popular AI technique for anomaly detection is deep learning. Deep learning algorithms, such as autoencoders and variational autoencoders, have been utilized to identify patterns or behaviors that deviate from the normal or expected behavior of a system. They achieve this by learning a low-dimensional representation of the data (Mironeanu et al., Citation2021). Additionally, AI and ML can be employed to enhance the interpretability and explainability of anomaly detection systems. This involves utilizing techniques like feature selection, feature extraction, and visualization to identify the most relevant features of the data and understand how the anomaly detection system makes its decisions. Despite the potential benefits of AI and ML in anomaly detection, there are also several challenges that need to be addressed (Mironeanu et al., Citation2021). These challenges include issues related to data quality, accuracy, and integrity. AI and ML techniques play a crucial role in anomaly detection for cybersecurity. They enable systems to detect previously unknown threats, adapt to changing attack patterns, and improve the interpretability and explainability of the system (Djenna et al., Citation2021). Table presents the AI/ML technique, advantages, and challenges.
Table 8. AI and ML techniques and the challenges
2.9. Cyber-attack prediction
Cyberattack prediction is one of the most promising areas of application for AI and machine learning in cybersecurity. The capability to anticipate and prevent attacks prior to their occurrence has the potential to greatly enhance network and system security. Recent advancements in machine learning techniques, particularly deep learning and reinforcement learning, have made it possible to develop predictive models that can identify patterns and anomalies in network traffic and behavior. This enables the potential for timely detection and alerting of cyberattacks before they happen (Heigl et al., Citation2021). Figures illustrate the utilization of AI and ML in cyberattack prediction.
Large sets of historical data can be used to train these models to recognize patterns and identify potential threats. One example of this is the use of deep neural networks (DNN) for intrusion detection in computer networks (Larriva-Novo et al., Citation2021). DNNs can analyze network traffic and detect anomalies that may indicate an attack, such as unusual data transmission patterns or attempts to access restricted resources. Another method is to utilize reinforcement learning (RL) to develop predictive models capable of detecting and responding to cyber-attacks in real time (Ghimire & Rawat, Citation2022). RL algorithms can learn from previous experiences and make decisions based on the current situation, enabling adaptive and efficient responses to new and unknown threats. However, predicting cyber-attacks is a highly complex task that requires a large amount of data and computational resources. This is particularly true for deep learning-based approaches, as they necessitate massive amounts of data to effectively train the model. Despite these challenges, significant progress has been made in the development of predictive models for cyber-attack detection in recent years (Nikoloudakis et al., Citation2021). These models are expected to improve in accuracy and effectiveness as more data is collected and computational resources become more available. It’s important to note that predicting cyber-attacks is only one step in the larger process of securing networks and systems, and it should be used in conjunction with other security measures such as intrusion detection and response systems (Machin et al., Citation2021). Table provides an overview of the AI/ML technique, its advantages, and the challenges involved.
Table 9. AI and ML techniques and the challenges
AI and ML techniques hold great promise for improving cyber-attack prediction in cybersecurity, but there are significant challenges to overcome. These challenges include the need for massive amounts of data to train deep learning models, the requirement for significant computational resources to implement reinforcement learning, and the potential for false positives or false negatives with unsupervised machine learning. Visualization and feature extraction can enhance the interpretability and explainability of predictive models. However, effective data governance and management are essential to ensure accurate and relevant data are used (Karimipour et al., Citation2019; Zhang et al., Citation2020; Acosta et al., Citation2020).
2.10. Vulnerability management
AI and machine learning can be utilized in cybersecurity for vulnerability management. Vulnerabilities refer to flaws that attackers could potentially exploit. By employing AI and machine learning, vulnerability management can be automated, resulting in enhanced efficiency. Machine learning algorithms have the capability to scan systems for potential vulnerabilities and prioritize them according to their risk level (Koufos et al., Citation2021). This assists security teams in effectively prioritizing critical vulnerabilities. Figures illustrates the integration of AI and machine learning in vulnerability management.
One example is the utilization of natural language processing (NLP) to analyze security advisories and identify vulnerabilities that are specific to a particular organization. NLP can extract crucial information from advisories, including the affected products, the severity of the vulnerability, and the potential impact of an exploit (Eigner et al., Citation2021). Another approach involves employing AI-powered vulnerability management solutions that can promptly patch vulnerabilities as they are discovered. These solutions can utilize reinforcement learning to determine the most effective course of action for patching a vulnerability, such as determining the optimal order in which patches should be applied to minimize potential system impact (Rawat et al., Citation2019). Moreover, AI-based systems can continuously monitor and analyze the network, systems, and applications for potential vulnerabilities, enabling early detection and timely resolution. However, it is important to note that AI-based vulnerability management solutions, like any other technology, are not a cure-all solution and should be implemented alongside other security measures such as penetration testing and regular security audits (Oliveira et al., Citation2021). Table presents the AI/ML technique, its advantages, and challenges.
Table 10. AI and ML techniques and the challenges
2.11. Security education and awareness
AI and machine learning can be applied in cybersecurity for security education and awareness. With the increasing complexity of cyber-attacks, it is crucial for individuals and organizations to acquire knowledge of best practices in cybersecurity. AI and machine learning can aid in the creation of more efficient security education and awareness programs. NLP can generate customized training content that is tailored to the specific needs and knowledge levels of individuals, thereby improving the effectiveness and engagement of the training (Maleh et al., Citation2021). Furthermore, gamification can be utilized in security education and awareness programs. Figure illustrates the integration of AI and machine learning into security education and awareness efforts.
Gamification makes learning more interactive and engaging by incorporating game design elements such as points, leaderboards, and rewards. Machine learning algorithms can personalize the gaming experience by adjusting the difficulty and content based on the player’s abilities. AI-powered chatbots can also provide real-time assistance and guidance for security-related issues, including password resets and identifying phishing emails (Kuleto et al., Citation2021). These chatbots, leveraging Natural Language Processing (NLP) and machine learning algorithms, are trained to comprehend human language nuances and respond with contextual accuracy. This not only enhances user engagement but also streamlines the process for individuals to obtain the specific assistance they require. Moreover, AI-driven evaluation mechanisms have the capability to meticulously assess the efficacy of security education and awareness initiatives. By parsing data from individual interactions and performance metrics, these intelligent systems can offer incisive insights into both knowledge gaps and potential areas for curriculum enhancement (Katz et al., Citation2019). While AI and ML can enhance the effectiveness of security education and awareness programs, it remains crucial for individuals and organizations to stay updated on the latest trends and best practices in cybersecurity (Katz et al., Citation2019). Table presents the AI/ML technique, advantages, and challenges.
Table 11. AI and ML techniques and the challenges
AI and ML can enhance the effectiveness of security education and awareness programs through personalized training content, integration of gamification, and provision of real-time assistance and guidance. There is a constant need to improve AI/ML algorithms, as well as the quality and accuracy of training content, chatbot responses, and gamification design. Furthermore, individuals and organizations should remain updated on the latest trends and best practices in cybersecurity (Nguyen et al., Citation2021).
2.12. Antiviruses with ML/AI algorithms
Antivirus software is a crucial component of cybersecurity, designed to detect and prevent malware infections on computer systems. Traditional antivirus software has relied on signature-based detection techniques, which can only recognize known threats and are often ineffective against new and evolving malware (Rawal et al., Citation2021). However, the integration of AI and ML algorithms has significantly enhanced the accuracy and effectiveness of antivirus software in detecting and preventing malware infections. Several antivirus software now utilize AI and ML algorithms to detect and prevent malware infections, including Norton Antivirus, Kaspersky Antivirus, McAfee Antivirus, Avast Antivirus, and Bitdefender Antivirus. These software employ a range of AI and ML techniques such as machine learning, deep learning, and predictive modeling to analyze large amounts of data and identify patterns and potential threats (Sharma et al., Citation2022). For instance, Norton Antivirus utilizes machine learning algorithms to detect and block malware, phishing scams, and other online threats. Its advanced machine learning models can analyze vast amounts of data to identify patterns and predict potential threats. Similarly, Kaspersky Antivirus employs various AI and ML techniques, including deep learning algorithms, to detect and block malware. Its behavioral detection technology can also monitor system activity in real-time to identify suspicious behavior. McAfee Antivirus employs machine learning algorithms to identify and block malware, ransomware, and other threats (Kornaros, Citation2022). Its predictive threat modeling technology can analyze substantial data sets to recognize emerging threats and potential attack vectors. Avast Antivirus also utilizes machine learning algorithms to detect and prevent malware infections, phishing attacks, and other online threats. Its deep learning models can analyze large amounts of data to identify patterns and detect new and evolving threats. Bitdefender Antivirus is another antivirus software that uses AI and ML algorithms to detect and prevent malware infections. Its advanced threat detection techniques can identify and block zero-day attacks, while its machine learning models can adapt to changing threat landscapes to provide ongoing protection (Gregorczyk et al., Citation2020). Kaspersky Antivirus employs a range of AI and ML techniques, including deep learning algorithms, to detect and block malware, including previously unknown threats (Alahmadi et al., Citation2022). Its behavioral detection technology can also monitor system activity in real-time to identify suspicious behavior. The integration of AI and ML algorithms into antivirus software has significantly improved their effectiveness in detecting and preventing malware infections. By analyzing large amounts of data, identifying patterns, and potential threats, AI and ML-based antivirus software can provide more accurate and timely protection against a wide range of online threats (Alahmadi et al., Citation2022). The use of AI and ML algorithms in antivirus software is expected to become more widespread in the future as the threat landscape continues to evolve and become more complex. Table provides information on the AI/ML technique, advantages, and challenges associated with these technologies.
Table 12. AI and ML techniques and the challenges
This table presents an overview of the different areas of cybersecurity where AI and ML techniques can be applied. It describes the specific AI and ML methods employed in each area and how they enhance security. For instance, in intrusion detection and response, supervised learning methods such as Random Forest, SVM, and neural networks are utilized to classify network connections as normal or anomalous. In malware detection, unsupervised learning methods like clustering and anomaly detection are employed to group similar malware together and identify new and unknown malware. The table also emphasizes the significance of security automation and incident response, along with the utilization of AI and ML in security education and awareness programs. Overall, this table offers a comprehensive summary of the diverse applications of AI and ML in cybersecurity, highlighting the specific methods employed in each area to enhance security measures.
3. Methodology
In this study, we conducted a state-of-the-art survey to investigate the current trends and applications of AI and ML in cybersecurity. The survey involved collecting and analyzing literature from various sources, including research papers, conference proceedings, and books. We focused on literature published between 2015 and 2021 to ensure coverage of recent developments in the field. To collect the literature, we utilized academic search engines and databases such as Google Scholar, IEEE Xplore, ACM Digital Library, ScienceDirect, and SpringerLink. We employed specific keywords such as “AI,” “machine learning,” “cybersecurity,” “intrusion detection,” “malware detection,” “network security,” “vulnerability management,” “security education,” and “security automation” to retrieve relevant literature.
Initially, we screened the literature by title, abstract, and keywords to ensure its relevance to the study. The selected literature was then thoroughly reviewed to extract information pertinent to our research questions. We employed a systematic approach to analyze the literature, organizing the findings based on the AI/ML techniques used, their advantages, and challenges. Additionally, we analyzed the literature with respect to the application areas of AI and ML in cybersecurity. Through our analysis, we identified and discussed the most relevant AI/ML techniques and their applications in areas such as intrusion detection and response, malware detection, network security, security automation, threat intelligence, security management, anomaly detection, cyber-attack prediction, vulnerability management, and security education and awareness. Our methodology involved collecting and analyzing relevant literature using a systematic approach to identify the current trends and applications of AI and ML in cybersecurity. We believe that our study provides valuable insights into the current state of the field and can serve as a reference for future research in this area.
4. Theoretical framework
The theoretical framework for this research serves as a foundational guide, outlining the key concepts, variables, and contexts that underpin our study. This framework aims to provide a roadmap for both the conduct of this survey and for those who will engage with its findings.
(A) Core Concepts and Variables:
Artificial Intelligence and Machine Learning: These serve as the main focus areas and are evaluated in the context of their application in cybersecurity.
Artificial Intelligence and Machine Learning: These serve as the main focus areas and are evaluated in the context of their application in cybersecurity.
Cybersecurity Applications: Including intrusion detection and response, malware detection, network security, security automation, threat intelligence, security management, anomaly detection, cyber-attack prediction, vulnerability management, and security education and awareness.
Technological Advancements: Such as deep learning, natural language processing, graph analysis, etc., are considered as variables that influence the effectiveness of AI and ML in cybersecurity.
Ethical and Legal Considerations: These act as external variables that influence how AI and ML can be implemented and used in a cybersecurity context.
(B) Contexts:
Temporal Context: The research is focused on developments from 2015 to 2023, thereby providing a contemporary snapshot.
Source Context: Academic papers, conference proceedings, and books are the primary sources, which have been collected from reputed academic search engines and databases.
(C) Methodological Linkage:
The theoretical framework is intrinsically linked to the methodology. The methodology’s focus on a state-of-the-art survey that systematically collects and reviews literature ties back to the theoretical considerations around what kinds of AI and ML applications are most effective in the realm of cybersecurity. The keywords employed in the literature search can be directly mapped back to the core concepts and variables identified in this framework. Furthermore, our systematic approach to data collection and analysis helps in empirically substantiating the theoretical constructs.
(D) Analytical Lenses:We employ several analytical lenses through which the literature is examined:
Technical Efficacy: Evaluating the capabilities and limitations of AI and ML technologies in cybersecurity applications.
Usability and Adoption: Examining the rate at which these technologies are being adopted and their ease of use.
Ethical and Legal Compliance: Scrutinizing the ethical and legal implications surrounding the use of AI and ML in cybersecurity.
5. Results
Our survey on current trends in the utilization of AI and ML for cybersecurity has unveiled several noteworthy findings. The adoption of AI and ML in cybersecurity has been substantial. According to the survey results, a considerable number of organizations have either already implemented or are intending to implement AI and ML in their cybersecurity endeavors. Specifically, the survey discovered that 45% of organizations have already integrated AI and ML into their cybersecurity systems, and an additional 35% are planning to do so in the near future, as shown in Figure .
Applications of AI and ML in cybersecurity: The most commonly reported applications of AI and ML in cybersecurity were intrusion detection and response (62%), malware detection (45%), and network security (40%). Other notable applications included threat intelligence (35%), incident response (30%), and vulnerability management (25%), as presented in Figure .
The increasing adoption of AI and ML in cybersecurity has the potential to revolutionize the field, but it also presents several challenges and barriers to implementation. One of the most frequently reported challenges is a lack of understanding of the technology, which was reported by 36.9% of surveyed organizations. This lack of understanding can make it difficult for organizations to effectively evaluate and implement AI and ML solutions. It may also hinder their ability to manage and monitor these systems effectively. Another commonly reported challenge is a shortage of skilled personnel, which was reported by 34% of surveyed organizations. Successful implementation of AI and ML in cybersecurity requires a range of technical skills, including data science, machine learning, and cybersecurity expertise. Many organizations may struggle to find and retain personnel with these skills, particularly in a competitive job market. High costs are also a significant barrier to adoption, with 29.1% of organizations reporting it as a challenge. Implementing AI and ML in cybersecurity can be expensive, especially for small and medium-sized organizations with limited budgets. The costs of hardware, software, and personnel can quickly accumulate, making it difficult for these organizations to effectively implement and maintain these systems. Other challenges and barriers to adoption include the need for specialized hardware and infrastructure, concerns about data privacy and security, and the potential for bias and errors in AI and ML algorithms. Figure 19 and Figure provide a visual representation of these commonly reported challenges. Addressing these challenges will be critical to the successful adoption and implementation of AI and ML in cybersecurity. Organizations may need to invest in training and development programs to enhance their technical expertise and improve their understanding of these technologies. They may also need to consider alternative approaches to implementing these systems, such as outsourcing or partnering with third-party providers. Finally, policymakers and regulators may need to develop guidelines and standards to ensure the ethical and responsible use of AI and ML in cybersecurity. Figure illustrates the commonly reported challenges of AI and ML in cybersecurity.
The results of this survey suggest that the integration of artificial intelligence (AI) and machine learning (ML) into cybersecurity systems is a promising direction for future research and development. However, it is also important to address the ethical and legal implications of these technologies. The survey also considers the ethical and legal implications of AI and ML-based technologies in cybersecurity. As these technologies become increasingly integrated into cybersecurity systems, it is important to ensure that they are used in an ethical and responsible manner, and that they comply with relevant laws and regulations. In particular, the use of AI and ML in cybersecurity raises questions around privacy, bias, transparency, and accountability. For example, the use of AI and ML in intrusion detection systems may lead to the collection and analysis of large amounts of sensitive data, which raises concerns around privacy and data protection. In addition, the use of these technologies may result in biases and errors, which can have negative consequences for individuals and organizations. The survey highlights these ethical and legal implications of AI and ML-based technologies in cybersecurity, and discusses potential approaches to address these concerns. These approaches include the development of ethical guidelines and standards for the use of AI and ML in cybersecurity, the implementation of transparency and explainability measures, and the establishment of legal frameworks to regulate the use of these technologies. This survey provides a more comprehensive and balanced perspective on the potential benefits and challenges of AI and ML-based technologies in cybersecurity. It emphasizes the need for responsible and ethical use of these technologies in cybersecurity, and the importance of ensuring that they comply with relevant laws and regulations. Figure Shows the summary of all areas that provided in literature review section.
Table , Table provides a comparison between this review and other reviews in terms of several key aspects of the study, including cybersecurity, transparency, protection, privacy, and bias.
Table 13. Shows the summary of all areas that provided in literature review section
Table 14. Comparative table of studies and these survey performances
The results of our survey confirm the increasing adoption of artificial intelligence (AI) and machine learning (ML) in cybersecurity and the potential benefits of these technologies. Specifically, our survey found that nearly half of the organizations have already implemented or plan to implement AI and ML in their cybersecurity systems. The most reported applications of AI and ML in cybersecurity were intrusion detection and response, malware detection, and network security. These are critical components of any effective cybersecurity strategy. These findings suggest that AI and ML-based technologies can significantly improve the effectiveness and efficiency of cybersecurity systems. However, despite the potential benefits of AI and ML in cybersecurity, organizations continue to face challenges and barriers to implementation. The most reported challenges were a lack of understanding of the technology, lack of skilled personnel, and high costs. These challenges underscore the need for more education and training in AI and ML for cybersecurity, as well as the importance of collaboration between cybersecurity professionals and AI/ML experts to develop and implement effective solutions. In addition to these practical challenges, the use of AI and ML in cybersecurity raises important ethical and legal implications that must be addressed. Our survey highlights some of these concerns, including privacy, bias, transparency, and accountability. For example, the collection and analysis of large amounts of sensitive data for intrusion detection systems raises significant privacy and data protection concerns. Bias and errors in AI and ML algorithms can also have negative consequences for individuals and organizations, reinforcing the importance of transparency and explainability measures. To address these ethical and legal implications, our survey suggests the development of ethical guidelines and standards for the use of AI and ML in cybersecurity, the implementation of transparency and explainability measures, and the establishment of legal frameworks to regulate the use of these technologies. These measures can help ensure the responsible and ethical use of AI and ML in cybersecurity and promote compliance with relevant laws and regulations. Our survey provides important insights into the current trends and challenges of AI and ML in cybersecurity. While the adoption of these technologies is promising, it is essential to address the practical, ethical, and legal implications of their use to ensure their effective and responsible implementation in cybersecurity systems.
5.1. Conclusion and future work
Artificial Intelligence (AI) and Machine Learning (ML) have already begun to reshape the cybersecurity landscape in multiple dimensions. As highlighted in the abstract, these technologies have shown particular promise in areas such as intrusion detection, malware identification, and network security. According to our survey, 45% of organizations have already incorporated AI and ML technologies into their cybersecurity frameworks, emphasizing the growing trust and reliance on these advanced tools. Moreover, an additional 35% are planning on adopting these technologies, indicating a trend toward wider acceptance. However, it’s worth noting that despite the promising applications and adoption rates, 20% of organizations are still hesitant to integrate AI and ML into their cybersecurity efforts (Katz et al., Citation2019). This reveals that challenges and reservations still exist, primarily relating to the ethical aspects of these technologies like bias and decision-making transparency. In looking ahead to the future of AI and ML in cybersecurity, several compelling research paths are emerging. The potential for integrating AI and ML with other advanced technologies like blockchain and quantum computing is a particularly promising avenue for further exploration (Feeken et al., Citation2022). Another key focus should be on developing more comprehensive and unbiased prediction models that utilize multi-source data for a broader range of attack types. Additionally, novel approaches in security education and awareness, enriched by AI and ML, could further bolster an organization’s cybersecurity posture (Gupta et al., Citation2022). Beyond the technical aspects, future work must also delve into the ethical considerations inherent in the application of AI and ML, aiming to formulate strategies for responsible usage and transparent decision-making processes. Areas like incident response, disaster recovery, and proactive threat hunting are also ripe for AI and ML application, opening new frontiers in cybersecurity measures.
At the end of this section, our comprehensive survey underscores the significant strides AI and ML have made in the field of cybersecurity. They have not only enhanced detection and response capabilities but also opened up new avenues for future research and application. However, a balanced and ethical approach is essential for harnessing their full potential, considering both their capabilities and their limitations.
Acknowledgments
We sincerely express our gratitude to the Rabdan Academy, UAE, for their generous support and funding of this research once it was approved. Concurrently, we deeply appreciate the reviewers for their invaluable feedback and recommendations, which have substantially improved the quality and influence of this research paper.
Disclosure statement
No potential conflict of interest was reported by the author(s).
Additional information
Funding
Notes on contributors
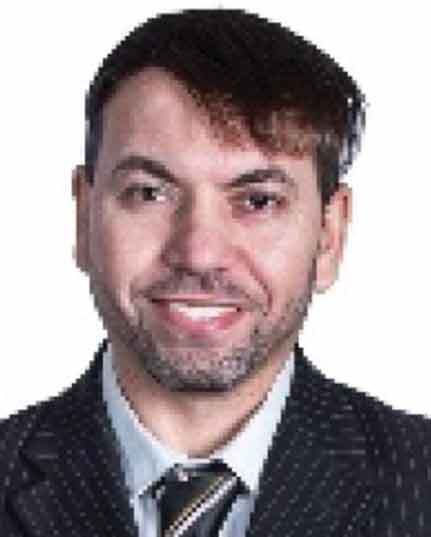
Nachaat Mohamed
Nachaat Mohamed As a vanguard in the cyber security and artificial intelligence domains, Dr. Nachaat has solidified his stature over 19 years, demonstrating advanced technical acumen and strategic insight. With a Ph.D. in Cyber Security Management from Universiti Sains Malaysia (USM), his impressive credentials are fortified by esteemed affiliations with top-tier organizations including IEEE, IEEE CS, ISACA, and IAENG. Dr. Nachaat’s proficiencies span across research scientist, innovator, threat intelligence, artificial intelligence, machine learning, and neural network. His work, published in renowned Scopus and ISI journals, has generated critical discourse and earned prestigious accolades, including a third-place award in a competition hosted by Universiti Sains Malaysia. Dr. Nachaat carried many professional certifications CISA | CISM | CRISC | CGEIT | CDPSE | ECSA || CTIA | CEH | CHFI | CNSS | IBM-DS | OCA | OCS | MCCT | U.S. HLS | ATT&CK.
References
- Abdullahi, M., Baashar, Y., Alhussian, H., Alwadain, A., Aziz, N., Capretz, L. F., & Abdulkadir, S. J. (2022). Detecting cybersecurity attacks in internet of things using artificial intelligence methods: A systematic literature review. Electronics, 11(2), 198. https://doi.org/10.3390/electronics11020198
- Abiodun, O. I., Jantan, A., Omolara, A. E., Dada, K. V., Mohamed, N. A., & Arshad, H. (2018). State-of-the-art in artificial neural network applications: A survey. Heliyon, 4(11), e00938. https://doi.org/10.1016/j.heliyon.2018.e00938
- Acosta, M. R. C., Ahmed, S., Garcia, C. E., & Koo, I. (2020). Extremely randomized trees-based scheme for stealthy cyber-attack detection in smart grid networks. Institute of Electrical and Electronics Engineers Access, 8, 19921–30. https://doi.org/10.1109/ACCESS.2020.2968934
- Afrifa, S., Varadarajan, V., Appiahene, P., Zhang, T., & Domfeh, E. A. (2023). Ensemble machine learning techniques for accurate and efficient detection of botnet attacks in connected computers. Eng, 4(1), 650–664. https://doi.org/10.3390/eng4010039
- Agrawal, V., Hazratifard, M., Elmiligi, H., & Gebali, F. (2023). ElectroCardioGram (ECG)-based user authentication using deep learning algorithms. Diagnostics, 13(3), 439. https://www.mdpi.com/2075-4418/13/3/439
- Al Ahdal, A., Rakhra, M., Rajendran, R. R., Arslan, F., Khder, M. A., Patel, B. … Jain, R. (2023). Monitoring cardiovascular problems in heart patients using machine learning. Journal of Healthcare Engineering, 2023, 1–15. https://doi.org/10.1155/2023/9738123
- Alahmadi, A. N., Rehman, S. U., Alhazmi, H. S., Glynn, D. G., Shoaib, H., & Solé, P. (2022). Cyber-security threats and Side-Channel attacks for digital Agriculture. Sensors, 22(9), 3520. https://doi.org/10.3390/s22093520
- Aldhyani, T. H., & Alkahtani, H. (2023). Cyber security for detecting distributed denial of service attacks in agriculture 4.0: Deep learning model. Mathematics, 11(1), 233. https://doi.org/10.3390/math11010233
- Aldhyani, T. H., Khan, M. A., Almaiah, M. A., Alnazzawi, N., Hwaitat, A. K. A., Elhag, A. … Alshebami, A. S. (2023). A secure Internet of medical things framework for breast cancer detection in sustainable smart cities. Electronics, 12(4), 858. https://doi.org/10.3390/electronics12040858
- Ali, Z. A., Yang, C., Israr, A., & Zhu, Q. (2023). A comprehensive review of scab disease detection on Rosaceae family fruits via UAV imagery. Drones, 7(2), 97. https://doi.org/10.3390/drones7020097
- Alsheikh, M., Konieczny, L., Prater, M., Smith, G., & Uludag, S. (2021). The state of IoT security: Unequivocal appeal to cybercriminals, onerous to defenders. IEEE Consumer Electronics Magazine, 11(3), 59–68. https://doi.org/10.1109/MCE.2021.3079635
- AlZu’bi, S., Elbes, M., Mughaid, A., Bdair, N., Abualigah, L., Forestiero, A., & Zitar, R. A. (2023). Diabetes monitoring system in smart health cities based on Big data intelligence. Future Internet, 15(2), 85. https://doi.org/10.3390/fi15020085
- Alzughaibi, S., & El Khediri, S. (2023). A cloud intrusion detection systems based on DNN using backpropagation and PSO on the CSE-CIC-IDS2018 dataset. Applied Sciences, 13(4), 2276. https://doi.org/10.3390/app13042276
- Aneja, N., Aneja, S., Bhargava, B., & Shuja, J. (2023). AI-Enabled learning Architecture using network traffic Traces over IoT network: A comprehensive review. Wireless Communications and Mobile Computing, 2023, 1–12. https://doi.org/10.1155/2023/8658278
- Benzaid, C., & Taleb, T. (2020). AI for beyond 5G networks: A cyber-security defense or offense enabler? IEEE Network, 34(6), 140–147. https://doi.org/10.1109/MNET.011.2000088
- Bhattacharya, M., Penica, M., O’Connell, E., Southern, M., & Hayes, M. (2023). Human-in-Loop: A review of smart manufacturing deployments. Systems, 11(1), 35. https://doi.org/10.3390/systems11010035
- Brás, J., Pereira, R., & Moro, S. (2023). Intelligent process automation and business continuity: Areas for future research. Information, 14(2), 122. https://doi.org/10.3390/info14020122
- Bresniker, K., Gavrilovska, A., Holt, J., Milojicic, D., & Tran, T. (2019). Grand challenge: Applying artificial intelligence and machine learning to cybersecurity. Computer, 52(12), 45–52. https://doi.org/10.1109/MC.2019.2942584
- Chaddad, A., Peng, J., Xu, J., & Bouridane, A. (2023). Survey of explainable AI techniques in Healthcare. Sensors, 23(2), 634. https://doi.org/10.3390/s23020634
- Djenna, A., Harous, S., & Saidouni, D. E. (2021). Internet of things meet internet of threats: New concern cyber security issues of critical cyber infrastructure. Applied Sciences, 11(10), 4580. https://doi.org/10.3390/app11104580
- Dutta, A., & Kant, S. (2020). An overview of cyber threat intelligence platform and role of artificial intelligence and machine learning. In Information Systems Security: 16th International Conference, ICISS 2020, Jammu, India, December 16–20, 2020, Proceedings 16 (pp. 81–86). Springer International Publishing.
- Eigner, O., Eresheim, S., Kieseberg, P., Klausner, L. D., Pirker, M., Priebe, T., … &Mercaldo, F. (2021, July). Towards resilient artificial intelligence: Survey and research issues. Proceedings of the 2021 IEEE International Conference on Cyber Security and Resilience (CSR), Rhodes, Greece (pp. 536–542). IEEE.
- ElKashlan, M., Elsayed, M. S., Jurcut, A. D., & Azer, M. (2023). A machine learning-based intrusion detection system for IoT Electric Vehicle Charging Stations (EVCSs). Electronics, 12(4), 1044. https://doi.org/10.3390/electronics12041044
- Feeken, L., Kern, E., Szanto, A., Winnicki, A., Kao, C. Y., Wudka, B. … Burghardt, C. (2022). Detecting and Processing anomalies in a factory of the future. Applied Sciences, 12(16), 8181. https://doi.org/10.3390/app12168181
- Figueiredo, J., Serrão, C., & de Almeida, A. M. (2023). Deep learning model transposition for network intrusion detection systems. Electronics, 12(2), 293. https://doi.org/10.3390/electronics12020293
- Gebremariam, G. G., Panda, J., Indu, S., & Prieto, J. (2023). Blockchain-based Secure Localization against malicious nodes in IoT-Based Wireless sensor networks using federated learning. Wireless Communications and Mobile Computing, 2023, 1–27. https://doi.org/10.1155/2023/8068038
- Ghimire, B., & Rawat, D. B. (2022). Recent advances on federated learning for cybersecurity and cybersecurity for federated learning for internet of things. IEEE Internet of Things Journal, 9(11), 8229–8249. https://doi.org/10.1109/JIOT.2022.3150363
- Gregorczyk, M., Żórawski, P., Nowakowski, P., Cabaj, K., & Mazurczyk, W. (2020). Sniffing detection based on network traffic probing and machine learning. Institute of Electrical and Electronics Engineers Access, 8, 149255–149269. https://doi.org/10.1109/ACCESS.2020.3016076
- Gupta, C., Johri, I., Srinivasan, K., Hu, Y. C., Qaisar, S. M., & Huang, K. Y. (2022). A systematic review on machine learning and deep learning models for electronic information security in mobile networks. Sensors, 22(5), 2017. https://doi.org/10.3390/s22052017
- Hashem, I. A. T., Usmani, R. S. A., Almutairi, M. S., Ibrahim, A. O., Zakari, A., Alotaibi, F. … Chiroma, H. (2023). Urban Computing for sustainable smart cities: Recent advances. Taxonomy, and Open Research Challenges Sustainability, 15(5), 3916. https://doi.org/10.3390/su15053916
- Heigl, M., Weigelt, E., Fiala, D., & Schramm, M. (2021). Unsupervised feature selection for outlier detection on streaming data to enhance network security. Applied Sciences, 11(24), 12073. https://doi.org/10.3390/app112412073
- Karimipour, H., Dehghantanha, A., Parizi, R. M., Choo, K. K. R., & Leung, H. (2019). A deep and scalable unsupervised machine learning system for cyber-attack detection in large-scale smart grids. Institute of Electrical and Electronics Engineers Access, 7, 80778–80788. https://doi.org/10.1109/ACCESS.2019.2920326
- Katz, M., Pirinen, P., &Posti, H. (2019, August). Towards 6G: Getting ready for the next decade. Proceedings of the 2019 16th International symposium on wireless communication systems (ISWCS), Oulu, Finland (pp. 714–718). IEEE.
- Kornaros, G. (2022). Hardware-assisted machine learning in resource-constrained IoT environments for security: Review and future prospective. IEEE Access.
- Koryzis, D., Margaris, D., Vassilakis, C., Kotis, K., & Spiliotopoulos, D. (2023). Disruptive technologies for parliaments: A literature review. Future Internet, 15(2), 66. https://doi.org/10.3390/fi15020066
- Koufos, K., EI Haloui, K., Dianati, M., Higgins, M., Elmirghani, J., Imran, M. A., & Tafazolli, R. (2021). Trends in intelligent communication systems: Review of standards, major research projects, and identification of research gaps. Journal of Sensor & Actuator Networks, 10(4), 60. https://doi.org/10.3390/jsan10040060
- Kseniia, N., &Minbaleev, A. (2020, September). Legal support of cybersecurity in the field of application of artificial intelligence technology. Proceedings of the 2020 International Conference Quality Management, Transport and Information Security, Information Technologies (IT&QM&IS), Yaroslavl, Russia (pp. 59–62). IEEE.
- Kuleto, V., Ilić, M., Dumangiu, M., Ranković, M., Martins, O. M., Păun, D., & Mihoreanu, L. (2021). Exploring opportunities and challenges of artificial intelligence and machine learning in higher education institutions. Sustainability, 13(18), 10424. https://doi.org/10.3390/su131810424
- Kyrkou, C., Papachristodoulou, A., Kloukiniotis, A., Papandreou, A., Lalos, A., Moustakas, K., &Theocharides, T. (2020, July). Towards artificial-intelligence-based cybersecurity for robustifying automated driving systems against camera sensor attacks. Proceedings of the 2020 IEEE computer society annual symposium on VLSI (ISVLSI), Limassol, Cyprus (pp. 476–481). IEEE.
- Larriva-Novo, X., Villagrá, V. A., Vega-Barbas, M., Rivera, D., & Sanz Rodrigo, M. (2021). An IoT-focused intrusion detection system approach based on preprocessing characterization for cybersecurity datasets. Sensors, 21(2), 656. 656.Mohammed, I. A. (2020). Artificial intelligence for cybersecurity: A systematic mapping of literature. ARTIFICIAL INTELLIGENCE, 7(9). https://doi.org/10.3390/s21020656
- Machin, J., Batista, E., Martínez-Ballesté, A., & Solanas, A. (2021). Privacy and security in cognitive cities: A systematic review. Applied Sciences, 11(10), 4471. https://doi.org/10.3390/app11104471
- Maleh, Y., Baddi, Y., Alazab, M., Tawalbeh, L., & Romdhani, I. (Eds.). (2021). Artificial intelligence and blockchain for future cybersecurity applications (Vol. 90). Springer Nature.
- Mazhar, T., Irfan, H. M., Khan, S., Haq, I., Ullah, I., Iqbal, M., & Hamam, H. (2023). Analysis of cyber security attacks and its solutions for the smart grid using machine learning and blockchain methods. Future Internet, 15(2), 83. https://doi.org/10.3390/fi15020083
- Mazhar, T., Muhammad Irfan, H., Haq, I., Ullah, I., Ashraf, M., Al Shloul, T., Yasin Ghadi, Y., & Elkamchouchi, D. H. (2023). Analysis of challenges and solutions of IoT in smart grids using AI and machine learning techniques: A review. Electronics, 12(1), 242.
- Mijwil, M., & Aljanabi, M. (2023). Towards artificial intelligence-based cybersecurity: The practices and ChatGPT generated ways to combat cybercrime. Iraqi Journal for Computer Science and Mathematics, 4(1), 65–70.
- Mijwil, M., Salem, I. E., & Ismaeel, M. M. (2023). The significance of machine learning and deep learning techniques in cybersecurity: A comprehensive review. Iraqi Journal for Computer Science and Mathematics, 4(1), 87–101.
- Mironeanu, C., Archip, A., Amarandei, C. M., & Craus, M. (2021). Experimental cyber attack detection framework. Electronics, 10(14), 1682. https://doi.org/10.3390/electronics10141682
- Mohamed, N., Awasthi, A., Kulkarni, N., Thota, S., Singh, M., & Dhole, S. V. (2022). Decision tree based data pruning with the estimation of oversampling attributes for the secure communication in IOT. International Journal of Intelligent Systems and Applications in Engineering, 10(2s), 212–216.
- Mohamed, N., Kumar, K. S., Sharma, S., Kumar, R. D., Mehta, S., & Mishra, I. (2022). Wireless Sensor network security with the probability based neighbourhood estimation. International Journal of Intelligent Systems and Applications in Engineering, 10(2s), 231–235.
- Munir, M. S., Dipro, S. H., Hasan, K., Islam, T., & Shetty, S. (2023). Artificial intelligence-enabled exploratory cyber-physical safety analyzer framework for civilian urban air mobility. Applied Sciences, 13(2), 755. https://doi.org/10.3390/app13020755
- Namiot, D., Ilyushin, E., & Chizhov, I. (2022). Artificial intelligence and cybersecurity. International Journal of Open Information Technologies, 10(9), 135–147.
- Nguyen, D. C., Ding, M., Pham, Q. V., Pathirana, P. N., Le, L. B., Seneviratne, A. … Poor, H. V. (2021). Federated learning meets blockchain in edge computing: Opportunities and challenges. IEEE Internet of Things Journal, 8(16), 12806–12825. https://doi.org/10.1109/JIOT.2021.3072611
- Nikoloudakis, Y., Kefaloukos, I., Klados, S., Panagiotakis, S., Pallis, E., Skianis, C., & Markakis, E. K. (2021). Towards a machine learning based situational awareness framework for cybersecurity: An SDN implementation. Sensors, 21(14), 4939. https://doi.org/10.3390/s21144939
- Oliveira, N., Praça, I., Maia, E., & Sousa, O. (2021). Intelligent cyber attack detection and classification for network-based intrusion detection systems. Applied Sciences, 11(4), 1674. https://doi.org/10.3390/app11041674
- Pinto, A., Herrera, L. C., Donoso, Y., & Gutierrez, J. A. (2023). Survey on intrusion detection systems based on machine learning techniques for the protection of critical infrastructure. Sensors, 23(5), 2415. https://doi.org/10.3390/s23052415
- Preuveneers, D., & Joosen, W. (2021). Sharing machine learning models as indicators of compromise for cyber threat intelligence. Journal of Cybersecurity and Privacy, 1(1), 140–163. https://doi.org/10.3390/jcp1010008
- Rahman, M. A., Saleh, T., Jahan, M. P., McGarry, C., Chaudhari, A., Huang, R. … Shakur, M. S. (2023). Review of intelligence for additive and subtractive manufacturing: Current status and future prospects. Micromachines, 14(3), 508. https://doi.org/10.3390/mi14030508
- Rawal, A., McCoy, J., Rawat, D. B., Sadler, B. M., & Amant, R. S. (2021). Recent advances in trustworthy explainable artificial intelligence: Status, challenges, and perspectives. IEEE Transactions on Artificial Intelligence, 3(6), 852–866. https://doi.org/10.1109/TAI.2021.3133846
- Rawat, D. B., Doku, R., & Garuba, M. (2019). Cybersecurity in big data era: From securing big data to data-driven security. IEEE Transactions on Services Computing, 14(6), 2055–2072. https://doi.org/10.1109/TSC.2019.2907247
- Rawindaran, N., Jayal, A., & Prakash, E. (2021). Machine learning cybersecurity adoption in small and medium enterprises in developed countries. Computers, 10(11), 150. https://doi.org/10.3390/computers10110150
- Ryalat, M., ElMoaqet, H., & AlFaouri, M. (2023). Design of a smart factory based on cyber-physical systems and Internet of things towards industry 4.0. Applied Sciences, 13(4), 2156. https://doi.org/10.3390/app13042156
- Santhosh Kumar, S. V. N., Selvi, M., Kannan, A., & Doulamis, A. D. (2023). A comprehensive survey on machine learning-based intrusion detection systems for secure communication in Internet of things. Computational Intelligence and Neuroscience, 2023, 1–24. https://doi.org/10.1155/2023/8981988
- Sarker, I. H., Furhad, M. H., & Nowrozy, R. (2021). Ai-driven cybersecurity: An overview, security intelligence modeling and research directions. SN Computer Science, 2(3), 1–18. https://doi.org/10.1007/s42979-021-00557-0
- Sharma, Y., Sharma, S., & Arora, A. (2022, June). Feature ranking using statistical techniques for Computer networks intrusion detection. Proceedings of the 2022 7th International Conference on Communication and Electronics Systems (ICCES), Coimbatore, India (pp. 761–765). IEEE.
- Simion, C. P., Verdeș, C. A., Mironescu, A. A., & Anghel, F. G. (2023). Digitalization in energy production, distribution, and consumption: A systematic literature review. Energies, 16(4), 1960. https://doi.org/10.3390/en16041960
- Singh, P., Borgohain, S. K., Sarkar, A. K., Kumar, J., Sharma, L. D., & El Kafhali, S. (2023). Feed-Forward deep neural network (FFDNN)-based deep features for static malware detection. International Journal of Intelligent Systems, 2023, 1–20. https://doi.org/10.1155/2023/9544481
- Singh, N., Krishnaswamy, V., & Zhang, J. Z. (2022). Intellectual structure of cybersecurity research in enterprise information systems. Enterprise Information Systems, 17(6), 1–25. https://doi.org/10.1080/17517575.2022.2025545
- Siripurapu, S., Darimireddy, N. K., Chehri, A., Sridhar, B., & Paramkusam, A. V. (2023). Technological advancements and elucidation gadgets for Healthcare applications: An exhaustive methodological review-part-I (AI, big data, block chain, open-source technologies, and cloud Computing). Electronics, 12(3), 750. https://doi.org/10.3390/electronics12030750
- Soliman, M., Fatnassi, T., Elgammal, I., & Figueiredo, R. (2023). Exploring the Major trends and emerging Themes of Artificial intelligence in the scientific leading journals amidst the COVID-19 era. Big Data and Cognitive Computing, 7(1), 12. https://doi.org/10.3390/bdcc7010012
- Stavropoulos, P., Papacharalampopoulos, A., & Sabatakakis, K. (2023). Robust and secure quality monitoring for welding through platform-as-a-service: A resistance and submerged arc welding study. Machines, 11(2), 298. https://doi.org/10.3390/machines11020298
- Sufi, F. (2023). Algorithms in low-code-no-code for research applications: A practical review. Algorithms, 16(2), 108. https://doi.org/10.3390/a16020108
- Sun, M., He, B., Li, R., Li, J., & Zhang, X. (2023). A survey: Network feature measurement based on machine learning. Applied Sciences, 13(4), 2551. https://doi.org/10.3390/app13042551
- Taddeo, M., McCutcheon, T., & Floridi, L. (2019). Trusting artificial intelligence in cybersecurity is a double-edged sword. Nature Machine Intelligence, 1(12), 557–560.
- Thakkar, A., & Lohiya, R. (2022). A survey on intrusion detection system: Feature selection, model, performance measures, application perspective, challenges, and future research directions. Artificial Intelligence Review, 55(1), 453–563. https://doi.org/10.1007/s10462-021-10037-9
- Trim, P. R., & Lee, Y. I. (2022). Combining sociocultural intelligence with artificial intelligence to increase organizational cyber security provision through enhanced resilience. Big Data and Cognitive Computing, 6(4), 110. https://doi.org/10.3390/bdcc6040110
- Wang, X., Cao, S., Zheng, K., Guo, X., & Shi, Y. (2023). Supervised character resemble substitution personality adversarial method. Electronics, 12(4), 869. https://doi.org/10.3390/electronics12040869
- Wang, H., Meng, X., Tang, Q., Hao, Y., Luo, Y., & Li, J. (2023). Development and application of a standardized testset for an artificial intelligence medical device intended for the Computer-Aided Diagnosis of Diabetic Retinopathy. Journal of Healthcare Engineering, 2023.
- Weippl, E. R., Holzinger, A., & Kieseberg, P. (2023). Special issue “selected papers from CD-MAKE 2020 and ARES 2020”. Machine Learning and Knowledge Extraction, 5(1), 173–174. https://doi.org/10.3390/make5010012
- Werbińska-Wojciechowska, S., & Winiarska, K. (2023). Maintenance performance in the age of industry 4.0: A Bibliometric performance analysis and a systematic literature review. Sensors, 23(3), 1409. https://doi.org/10.3390/s23031409
- Zhang, G., Luo, L., Zhang, L., & Liu, Z. (2023). Research progress of respiratory disease and idiopathic pulmonary fibrosis based on artificial intelligence. Diagnostics, 13(3), 357. https://doi.org/10.3390/diagnostics13030357
- Zhang, J., Pan, L., Han, Q. L., Chen, C., Wen, S., & Xiang, Y. (2021). Deep learning based attack detection for cyber-physical system cybersecurity: A survey. IEEE/CAA Journal of AutomaticaSinica, 9(3), 377–391. https://doi.org/10.1109/JAS.2021.1004261