Abstract
A methodology based on Fourier transform infrared spectroscopy with attenuated total reflectance sampling technique, combined with multivariate analysis, was developed to monitor adulteration of extra virgin olive oil (EVOO) with peanut oil (PEO). Principal components regression (PCR), partial least squares regression (PLS-R), and linear discriminant analysis (LDA) allowed quantification of percentage of adulteration based on spectral data of 192 samples. Wavenumbers associated with the biochemical differences among several types of edible oils were investigated by principal component analysis. Two sets of frequencies were selected in order to establish a robust regression model. Set A consisted on the frequency regions from 600 to 1,800 cm−1 and from 2,750 to 3,050 cm−1. Set B comprised 17 discrete peak absorbance frequencies for which the communality value was higher than 0.6. Analysis of an external set of 25 samples allowed the validation and evaluation of the predictability of the models. When using a specific set of discrete peak absorbance frequencies, the R 2 coefficients for the prediction were 0.960 and 0.977, and the root mean square error (RMSE) were 1.49 and 1.05% V/V when using the PCR or PLS-R models, respectively. LDA was successful in the binary classification presence/absence of PEO in adulterated EVOO (with 5% V/V of less of PEO). LDA provided 92.3% correct classification for the calibration set and 88.3% correct classification when cross-validated. The lowest detectable concentration of PEO in EVOO was the lowest adulteration level studied, 0.5% V/V.
Public Interest Statement
Analysis of the quality and purity of edible oils, in general, and of extra virgin olive oil (EVOO), in particular, is of great importance. Adulteration of pure EVOO either with low-priced oils, with degraded used oils or with toxic mineral oils can have great economic and social impact, and is a serious public health problem because harmful substances are delivered to the organism of unwary consumers.
Several techniques can be used in the authentication of edible oils and other foodstuff, such as enzymatic and PCR based methodologies. Absorption spectroscopy in the visible, near infrared or in the mid-infrared has enormous advantages over classical methods as they provide real-time analysis.
In this work, infrared spectroscopy combined with statistical techniques was used to develop methodologies for the quantitative analyses of mixtures of EVOO with peanut oil in order to predict the adulteration level.
1. Introduction
Extra virgin olive oil (EVOO) is a vegetable edible oil made from healthy and intact fruits of the olive tree (Olea europaea L.) only by mechanical means (crushing, malaxation, and centrifugation) and can be directly consumed unrefined. No chemicals are used in this extraction process, thus keeping its original characteristics and constituents which are lost in refined oils (Nieto, Hodaifa, & Lozano Peña, Citation2010). EVOO has being extensively studied because of its biological and sensory properties, and because is an agricultural product of paramount reputation.
The detection of adulteration of food products is important for consumers, industries, and retailers. Analysis of the quality and purity of edible oils in general and of EVOO, in particular, is of great relevance and has been the subject of research of several authors (Ben-Ayed, Kamoun-Grati, & Rebai, Citation2013). Adulteration of pure expensive edible oils either with low-priced oils, with degraded used oils or with toxic mineral oils can have great economic and social impact, and is a serious public health problem (Johnson, Citation2014). Adulteration results in the integration of harmful substances in foodstuff supplied to unwary consumers. In particular, peanuts and derivatives, such as peanut butter or peanut oil (PEO), are known sources of allergies (Al-Muhsen, Clarke, & Kagan Citation2003). Consequently, the improvement of fast and non-expensive analytical methodologies having the ability of detecting such adulterations in EVOO is a current topic of research.
Quite a few techniques have been applied for quantification of adulteration of EVOO, for example, Fourier transform infrared (FTIR) spectroscopy, real-time mass spectrometry, gas chromatography, and high-performance liquid chromatography (Kataoka, Lord, & Pawliszyn, Citation2000). Optical techniques, such as Raman, fluorescence, and absorption spectroscopy, are reagentless, non-destructive analytical techniques having an increasing number of applications in the study of foodstuff. Namely, near infrared and FTIR spectroscopy found applications across a wide range of fundamental (Movasaghi, Rehman, & ur Rehman, Citation2008) and applied sciences (De Luca, Oliverio, Ioele, & Ragno, Citation2009) and production lines in industry (Roggo et al., Citation2007).
FTIR combined with chemometric methods is a powerful analytical approach. It does require minimal sample preparation, particularly when used in conjunction with attenuated total reflectance (ATR) (Vlachos et al., Citation2006). It has been recognized as a fast analytical technique to detect and quantify the presence of adulterants because it provides important information about the presence of certain functional groups. It is also considered a “fingerprint technique,” meaning that there are no two oils with the same FTIR spectra either in the number of peaks or in the maximum peak intensities.
FTIR has been applied in the detection of EVOO adulterated with sunflower, soyabean, sesame, corn, hazelnut, corn–sunflower binary mixture, cottonseed, rapeseed, rice bran, and palm oils (PAOs) (Baeten et al., Citation2005; Groselj, Vracko, Fernández Pierna, Baeten, & Novic, Citation2008; Gurdeniz & Ozen, Citation2009; Kasemsumran, Kang, Christy, & Ozaki, Citation2005; Rohman & Man, Citation2010, Citation2012a, Citation2012b). However, there is no available research on using FTIR-ATR spectroscopy for the analysis of adulteration of EVOO with PEO.
Therefore, FTIR spectroscopy allows researchers to differentiate between authentic and adulterated oils by observing the FTIR spectra changes due to the adulteration (Rohman & Man, Citation2012c). Spectral bands have been suggested as markers for adulteration with other edible oils (Rohman, Man, & Yusof, Citation2014). Rohman, Man, Ismail and Hashim (Citation2010) used the spectral frequencies of 1,105–1,120 and 960–965 cm−1 to construct calibration models that correlate between actual and FTIR-predicted values of oil adulterants in EVOO.
The principal component regression (PCR) and the partial least square regression (PLS-R) techniques are well-known statistical methods, often used in quantitative prediction methodologies based on spectroscopic data (Martens & Naes, Citation1989). Both techniques have large acceptance in a wide range of scientific fields. The main reason is that they have been designed to meet the situation where there are many, possibly correlated, predictor variables and few samples: a situation that is common, especially in food science where developments in spectroscopy since the seventies have revolutionized chemical analysis. They are based on reduction of data dimensionality and inverse calibration, in systems where there is a possibility to calibrate for the desired component while implicitly modeling the other source of variation (Miller & Miller, Citation2005). Both techniques can be applied to full infrared (IR) spectrum; however, some regions of the IR spectrum possess very little or irrelevant information. Useless signals coming from the interferences or instrumental drifts must be ignored (Centner et al., Citation1996; Wentzell & Vega Montoto, Citation2003).
In this work, FTIR-ATR spectroscopy combined with PCR and PLS-R techniques were used to develop methodologies for the quantitative analyses of mixtures of EVOO with PEO in order to predict the adulteration level of EVOO. In order to determine frequency regions useful to distinguish EVOO from other edible oils, the infrared spectra of soya bean oil (SOO), corn oil (CO), palm oil (PAO), sunflower oil (SFO), and grapeseed oil (GSO) were measured. To investigate the possibility of creating a robust regression model, a set of continuous spectral bands and a set of discrete wavenumbers were selected.
2. Material and methods
2.1. Sample preparation
Five brands of commercial EVOO were bought from local producers (samples were collected in the olive mills). Five brands of PEO were acquired from producers specialized in cold press oils. Four brands of each of these oils were used to prepare EVOO samples with different concentrations of PEO, constituting the so-called “calibration set”, i.e. a set of samples used to build and validate the PCR and PLS-R models. The remaining brand of each oil was reserved to prepare a set of samples, referred below as the “external set”, to test the predictability of the PCR and PLS-R methods.
The EVOO/PEO samples were prepared by mixing EVOO with PEO in different volume proportions, from 0.5 to 5% V/V in 0.5% V/V steps and from 6 to 30% V/V in 2% V/V steps (total of 23 sampling points) and mixed using a vortex mixer to ensure total homogenization. Each one of the sampling points of the calibration set was represented by eight samples: two out of four PEO brands were randomly selected and mixed with all four EVOO brands. The infrared absorption spectra of a set of 192 samples (184 EVOO/PEO, 4 pure EVOO, and 4 pure PEO) was measured and constituted the calibration spectral data-set. The remaining EVOO and PEO brands originated an external set of 25 spectra (23 EVOO/PEO, 1 pure EVOO, and 1 pure PEO). The pure oils were preserved in the original package and mixed samples were stored in polyethylene terephthalate flasks. Five other types of commercially available edible oils were acquired: SOO, CO, PAO, SFO, and GSO.
2.2. FTIR-ATR measurement
Infrared spectra were collected in a “Unicam Research Series” FTIR spectrometer equipped with a single-reflection “Golden Gate” diamond ATR module, a deuterated Lalanine doped triglycene sulfatedetector, and a KBr beamsplitter. The equipment is connected to computer and controlled by WinFirst 1.1 software (Madison, USA).
FTIR-ATR measurements were performed by pipetting a small drop (~5 μl) of edible oil on top of the ATR baseplate, which was kept at 30°C. All infrared spectra, recorded in absorbance mode, were collected in the region of 500–4,000 cm−1, co-adding 128 interferograms at a resolution of 4 cm−1, the collection time being approximately 2 min. Each measurement was repeated three times and averaged using the software that controls the equipment. From each of oil samples, five replicates were analyzed.
Each spectrum was subtracted against the background spectrum and at every three scans, a new background spectrum was taken. The ATR base was carefully cleaned in situ by scrubbing with ethanol and dried with soft tissue before measuring the next sample. The cleaning method was verified by collecting a background spectrum and comparing it with the previous one.
2.3. Mathematical treatment
Baseline drifts of the spectra were corrected by using a fourth-order polynomial. Smoothing of the spectra was based on the Savitzky-Golay algorithm using a third-order polynomial and frames of seven points. Afterward, data were mean-centered and standardized using the standard normal variate transformation. A principal component analysis (PCA) was initially utilized to inspect differences between samples. PCA transforms the large number of potentially correlated variables into a smaller number of uncorrelated factors (principal components, PCs), and thus reduces the size of the data-set. PCA permitted the identification of the most important variables (wavenumbers) associated with the differences between several edible oils. Elimination of uninformative spectral variables is important to achieve more robust and less complex models, nevertheless able to predict EVOO adulteration.
For qualitative analysis, principal components contributing to the variance of the data-set were subjected to linear discriminant analysis (LDA) in an attempt to predict the likelihood of a sample belonging to a previously defined group. LDA is a statistical method used to find a linear combination of structures with the potential to characterize or separate classes of objects or observations. The resulting arrangement may be used as a linear classifier or dimensionality reduction.
For quantitative analysis, principal components considerably contributing to the variance of the data-set were regressed using PLS-R and PCR onto the referred variables.
The calibration methodology for quantification of the adulteration relied on two steps, so-called calibration and validation. In the calibration step, a mathematical model was built to establish a relation between the matrix of FTIR spectra (predictor variables) and the concentration of analytes of interest (response variables), using a set of observations usually named calibration set. In the validation step, the developed model was used to calculate the concentration of samples not used to setup the model (De Luca et al., Citation2009).
The relative performance of the established model is evaluated by the root mean square error of calibration (RMSEC), root mean square error of cross-validation (RMSECV), and multiple coefficient of determination or regression coefficient (R 2). The model selected is then used to determine the concentration of samples of an independent prediction set (or external set). The predictive ability of the model is evaluated from the root mean square of prediction (RMSEP). The lower the RMSEP value, the higher the degree of accuracy of the prediction result provided by the calibration model.
PCA, LDA, PCR, and PLS-R calculations were performed using the Excel-based “XLSTAT” V2006.06 package (Addinsoft, Inc., New York, USA) and statistical software “Unscrambler” V9.6 package (Camo, Oslo, Norway).
3. Experimental results and discussion
3.1. FTIR spectra analysis
All the spectra were collected in mid-infrared region between 500 and 4,000 cm−1. Figure displays the average FTIR spectra of five replicates of each one of the seven edible oils in the range 600–3,050 cm-1. All spectra look very similar because all vegetable oils are essentially composed by ~92% of triacylglycerols, approximately 5% of di- and monoacylglycerols and small levels of other components. There are minor differences between the oils, namely between EVOO and PEO. A closer observation, inset of Figure , reveals small band shifts and changes in the relative peak intensity (absorbance), especially at about 1,117, 2,922, and 3,007 cm−1. The band at 3,007 cm−1 can be attributed to the stretching vibration of cis-double bonds while peak at 1,117 cm−1 corresponds to the CO group vibration. Small differences in relative intensity values were exploited in the classification and quantification of EVOO adulterated with PEO, as discussed below.
3.2. Exploratory principal component analysis
Each spectrum was divided in two regions, from 600 to 1,800 cm−1 and from 2,750 to 3,050 cm−1. The regions left out of the analysis presented an overall low signal-to-noise ratio and were found to be prone to induce misclassification. In addition, variation in the laboratory atmosphere during measurement induces random spectral changes in the region between 1,800 and 2,750 cm−1.
PCA was then applied to the two selected spectral regions to investigate the similarities and differences between EVOO and other vegetable oils. It was shown that the total variance of the data-set could be explained by 29 principal components, among which the first six principal components, with eigenvalue higher than 1, explain approximately 91.6% of the total variance.
In statistics, communality is defined as the sum of the squared principal component loadings for all principal components for a given original variable. It is the variance in that variable accounted for by all the principal components. In other words, the communality measures the percentage of variance in a given variable explained by all the principal components jointly and may be interpreted as the consistency of the indicator (Abdi & Williams, Citation2010). By definition, the initial value of the communality in PCA is 1. Small communalities’ values after extraction indicate variables that do not fit well the principal component solution and should be dropped from the analysis (Field, Citation2005). According to Stevens (Citation2002), a lower limit of 0.6 should be used.
Wavenumbers (predictor variables) for which the communality value of each principal components out of the six was higher or equal to 0.6 were considered as meaningfully explaining the variance of the spectral data-set and then were considered as prospective wavenumbers associated with the chemical differences among the seven types of vegetable oils considered.
These wavenumbers belong to 17 bands with peaks at 3,007, 2,952, 2,922, 2,853, 1,745, 1,701, 1,463, 1,417, 1,376, 1,361, 1,234, 1,160, 1,117, 1,099, 1,037, 850, and 723 cm−1, which were then selected for a second PCA. The peak at 723 cm−1 is associated with a CH2 rocking mode, while the peaks at 1,099, 1,117, 1,160, and 1,234 cm−1 correspond to CO stretching vibration. The peak at 1,463 cm−1 is associated with a CH2 bending (scissoring) vibration and the peak at 1,376 cm−1 is ascribed to symmetrical bending of CH3. A large peak at 1,745 cm−1 is responsible for the CO stretching vibrations. Symmetric and asymmetric stretching vibrations of CH2 are caused by the absorbance at 2,853 and 2,922 cm−1, respectively (Downey, Citation1998; Guillén & Cabo, Citation1997; Lerma-García, Ramis-Ramos, Herrero-Martínez, & Simó-Alfonso, Citation2010).
Figure represents the score plot obtained from a PCA using the absorbance at the mentioned peaks. The first principal component (F1) explained 54.0% variance, while the second (F2) and the third (F3) explained 29.4 and 9.1%, respectively; therefore, approximately 92.6% of variance can be described by only three principal components.
As in the score plot of Figure , each oil occupy different position in the F1/F2/F3 space, the obvious conclusion is that PCA allows qualitative discrimination between the edible oils under analysis. PEO, PAO, and EVOO are clearly separated from cluster formed by CO, SO, SFO, and GSO. In particular, PEO and EVOO are well apart in the F1/F2/F3 space (they are in opposite sides of the F2 axis) thus making these oils distinguishable from each other. CO and SOO are very close to each other; therefore, they would be very difficult to distinguish using infrared spectroscopy.
The loading plot in Figure (two-dimensional plot, for the sake of clarity) reveals, that frequencies of 3,007, 2,922, 2,853, and 1,117 cm−1 and, at a much smaller extend, the frequencies of 1,745, 1,160, and 1,099 cm−1, are the most important frequencies for the formation of principal components F1 and F2. Together, they explain 84.6% of the total variance.
3.3. Supervised analysis of EVOO adulteration with PEO
The average absorption spectra of EVOO samples adulterated with PEO and the spectra of pure oils are shown in Figure , where for the sake of clarity, only the spectra of 5, 10, 15, 20, 25, and 30% V/V PEO samples are represented.
Figure 4. Average FTIR spectra of PEO/EVOO admixtures and the corresponding pure oils
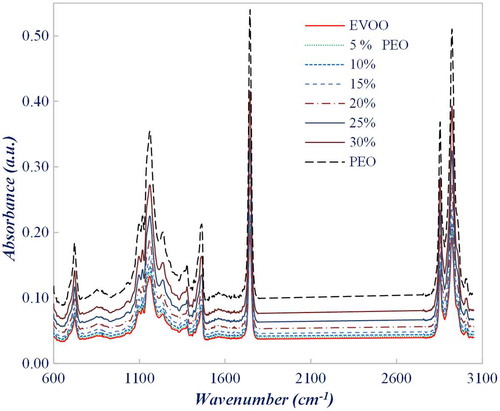
A sub-set of samples, constituted by EVOO adulterated with 5% V/V or less of PEO, pure EVOO and PE, was classified by LDA using the absorbance at the 17 frequencies referred in previous section. LDA was based in the à priori classification of each sample as EVOO, PEO/EVOO, or PEO. This classification constitutes the categorical or dependent variable. Figure shows the similarity map as defined by discriminant factors DF1 and DF2, which explained the total variance. This is a sub-set of the calibration set and was formed by four aliquots of each type of admixture.
Figure 5. Similarity map as determined by discriminant analysis using factors DF1 and DF2 for FTIR spectral data of pure EVOO, PEO, and EVOO mixed with PEO (samples with less than 5% V/V of PEO)
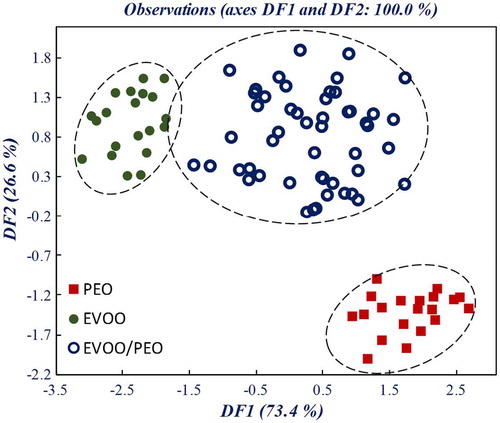
The classification in Table resulting from the LDA provided 92.3% correct classification for the calibration set and 88.3% correct classification when cross-validated. From the cross-validation results, it can be seen that the EVOO/PEO samples misclassified as pure EVOO is 10%. Notice that those samples belong to the set of samples adulterated with 0.5% V/V of PEO. Therefore, the lowest detectable concentration of PEO in EVOO was the lowest adulteration level studied, 0.5% V/V. This value is not a limitation of the experimental/theoretical methodology followed in this work; it is a consequence the fact that this is the minor value of adulteration used in this work. For the detection of adulteration of EVOO with cottonseed and rapeseed oils, detections limits of 1.4 and 1.32% V/V, respectively, were obtained (Gurdeniz & Ozen, Citation2009), values slightly higher than the obtained in the present work.
Table 1. Confusion matrix for the classification of pure EVOO, PEO, and EVOO mixed with PEO (samples with less than 5% V/V of PEO)
3.4. Model for prediction of olive oil adulteration based on FTIR spectral data
In the PCR and PLS-R calibration models, the evaluation of the linearity of the methods was carried out in order to show a proportional relationship between predictor variables (band intensity) and the percentage of EVOO adulteration with PEO.
The quality of the fitting was scrutinized by the RMSEC, multiple coefficient of determination or regression coefficient (R 2, where R is the correlation factor), and by the RMSECV (Wang, Lee, Wang, & He, Citation2006). The optimum number of factors, (principal component or latent variables for PCR and PLS-R models, respectively), was determined using Leave-One-Out cross-validation method. This is done by plotting the number of factors against the RMSECV and from this, the optimum number of factors is selected (Naes, Fearn, & Davies, Citation2002) for both PCR and PLS-R models. The optimum number of factors is the one that minimizes the RMSECV. The capability of the models to predict the percentage of EVOO adulteration with PEO for external samples was inspected by the RMSEP.
To build PCR or PLS-R models, regions containing significant information were selected, and the noisy signals arising from external interferences were ignored (Wang et al., Citation2006). The frequency regions used for quantification should be based on its capability to provide high correlation between actual PEO concentration and the corresponding FTIR-predicted levels.
Based on this reasoning two sets of frequencies were selected to establish an optimized regression model. Set A consisted on the frequency regions from 600 to 1,800 cm−1 and from 2,750 to 3,050 cm−1; Set B comprised the 17 peak absorbance frequencies in which the LDA was based on.
The behavior of the RMSEC and RMSECV as a function of the number of factors for PCR and PLS-R models was calculated for both sets of frequencies. The higher the number of factors, the lower the RMSEC value. A model build with such high number of factor would lead to over fitting, conducting to a very low RMSE value for the calibration samples but would give rise to high values of RMSE for an external set of samples. Table shows the number of factors corresponding to the minimum RMSECV for PCR and PLS-R and both sets of variables.
Table 2. Quality parameters of the multivariate calibrations models (PCR and PLS-R) for quantification of EVOO adulteration with PEO, using two different sets of frequencies
Figure represent the plot of the measured percentage of adulteration against the predicted values from FTIR measurements, using Set A and B of variable, respectively, which reflect the accuracy and the performance of the PCR and PLS regression models. Table also displays the quality parameters of the four multivariate calibrations models in terms of the RMSEC, RMSECV, RMSEP, and R 2 coefficients.
Figure 6. Principal component regression (PCR) and partial least squares regression (PLS‐R) among predicted and measured values of the addition level of PEO in mixtures with EVOO; (a) PCR, wavenumber Set A, (b) PLS-R, wavenumber Set A, (c) PCR, wavenumber Set B, and (d) PLS-R, wavenumber B
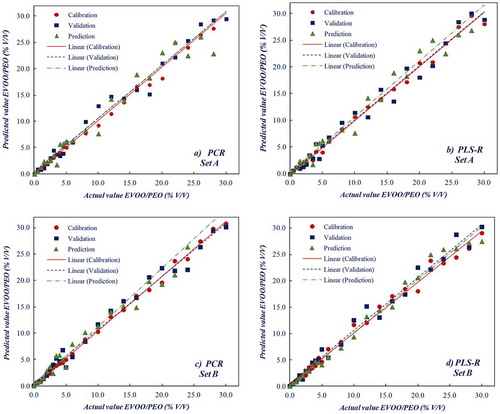
The coefficient R 2 measures the correlation between the measured values and the values predicted by the model. The closer the value to 1, the higher the correlation between the data. For PCR and PLS-R models, R 2 values are 0.960 and 0.977, respectively, for the external set of samples and for Set B of variables. As a general remark, both PCR and PLS regressions techniques offer low RMSEP values as compared with other regression techniques, such as multiple linear regression MLR (Martens & Naes, Citation1989); however the later offers better results, with a RMSEP value of 1.04% V/V.
The quantification of CO in EVOO was studied in (Rohman & Man, Citation2012b). The lowest value of RMSEC was 0.019% V/V; however, the model showed a high value of RMSEP of 2.34% V/V, a value slight higher than the obtained in the present work. In addition, the number of factors used is high (8 factors), meaning that over-fitting occurs for such model. For binary mixtures of EVOO with sunflower, corn, soybean, and hazelnut oils, Lerma-García et al. (Citation2010), developed MLR models capable of detecting a low-cost oil content in EVOO with RMSEP between 1.5 and 2.0% V/V, values of the same order of magnitude as in this work.
The minimum number of factors corresponding to optimum RMSECV is lower for Set B and for PLS regression (4 factors). Therefore, it may be concluded that it is not necessary to use data from the whole spectrum over the full mid-infrared region but only from discrete frequencies that compose Set B. Moreover, the RMSE for calibration, cross-validation, and prediction are lower for PLS-R and Set B. Under these conditions, PLS‐R provides accurate estimation of the concentration of PEO in EVOO.
4. Conclusions
In this work, we presented an exploratory study of the applicability of FTIR-ATR spectroscopy in the prediction of the adulteration level of EVOO with PEO. PCR and PLS multivariate regression techniques were found suitable for the application of a practical experimental methodology.
Using PCR, we were able to establish the most informative wavenumbers that provide distinction between edible oils. PCR and PLS-R were applied to full infrared spectrum as well as to the selected set of wavenumbers.
Validation and evaluation of the predictability of the models was attained by the analysis of a set of external samples. A root mean square error of the prediction value of ~1% V/V was obtained for a four-factor PLS regression model based on the discrete set of wavenumbers. Furthermore, LDA of spectral data was able to differentiate between pure EVOO and EVOO adulterated with 5% V/V or less of PEO. The lowermost detectable concentration was the lowest concentration studied in this work, 0.5% V/V. Additional research is necessary to find if a lower limit is attainable.
Our results indicate that FTIR spectroscopy combined with PLS-R applied to the wavenumbers of 3,007, 2,922, 2,853, 1,754, 1,160, and 1,117 cm−1 is reliable methodology for the quantification and discrimination of PEO in EVOO.
This study opens the perspective of future research with the goal to further decrease the number of wavenumbers necessary to discriminate edible oils. The use of a standard bulk FTIR spectrometer could be avoided provided that convenient electromagnetic source and detector are made available. Compact, low-weight, widely tunable laser sources in the mid-infrared are already commercially available, compatible with the some of the wavenumbers above. Moreover, using selected wavenumbers instead of the whole spectrum is an advantage from the point of view of industrial and commercial applicability of the methodology.
Correction
When this article was first published online the ORCID ID for Ana Barros was incorrect. This version has been corrected.
Cover image
Source: Author.
Additional information
Funding
Notes on contributors
José Manuel Marques Martins de Almeida
José Manuel Marques Martins de Almeida graduated in Applied Physics (Optics and Electronics) (1987) and has a PhD (1998) by University of Porto, Portugal. Since 2000, he has the position of an associate professor at the Department of Physics, Universidade de Trás os Montes e Alto Douro, Portugal and has Habilitation (2006) from the same University. Currently, he is a senior researcher in the Centre for Applied Photonics at INESC-TEC, Porto, Portugal. His present research interests include absorption and Raman spectroscopy and optical biosensors based on optical fibers for application in the food industry and life sciences.
References
- Abdi, H. , & Williams, L. J. (2010). Principal component analysis. Wiley Interdisciplinary Reviews: Computational Statistics , 2 , 433–459.
- Al-Muhsen, S. , Clarke, A. , & Kagan, R. (2003). Peanut allergy: An overview. Canadian Medical Association Journal , 168 , 1279–1285.
- Baeten, V. , Fernández Pierna, J. , Dardenne, P. , Meurens, M. , García-González, D. , & Aparicio-Ruiz, R. (2005). Detection of the presence of hazelnut oil in olive oil by FT-Raman and FT-MIR spectroscopy. Journal of Agricultural and Food Chemistry , 53 , 6201–6206.10.1021/jf050595n
- Ben-Ayed, R. , Kamoun-Grati, N. , & Rebai, A. (2013). An overview of the authentication of olive tree and oil. Comprehensive Reviews in Food Science and Food Safety , 12 , 218–227.10.1111/crf3.2013.12.issue-2
- Centner, V. , Massart, D. , de Noord, O. , de Jong, S. , Vandeginste, B. , & Sterna, C. (1996). Elimination of uninformative variables for multivariate calibration. Analytical Chemistry , 68 , 3851–3858.10.1021/ac960321m
- De Luca, M. , Oliverio, F. , Ioele, G. , & Ragno, G. (2009). Multivariate calibration techniques applied to derivative spectroscopy data for the analysis of pharmaceutical mixtures. Chemometrics and Intelligent Laboratory Systems , 96 , 14–21.10.1016/j.chemolab.2008.10.009
- Downey, G. (1998). Food and food ingredient authentication by mid-infrared spectroscopy and chemometrics. TrAC trends in analytical chemistry , 17 , 418–424.10.1016/S0165-9936(98)00042-9
- Field, A. P. (2005). Discovering statistics using SPSS . London: Sage.
- Groselj, N. , Vracko, M. , Pierna, J. , Baeten, V. , & Novic, M. (2008). The use of FT-MIR spectroscopy and counter-propagation artificial neural networks for tracing the adulteration of olive oil. Acta Chimica Slovenica , 55 , 935–941.
- Guillén, M. & Cabo, N. (1997). Characterization of edible oils and lard by Fourier transform infrared spectroscopy. Relationships between composition and frequency of concrete bands in the fingerprint region. Journal of the American Oil Chemists Society , 74 , 1281–1286.10.1007/s11746-997-0058-4
- Gurdeniz, G. , & Ozen, B. (2009). Detection of adulteration of extra-virgin olive oil by chemometric analysis of mid-infrared spectral data. Food Chemistry , 116 , 519–525.10.1016/j.foodchem.2009.02.068
- Johnson, R. (2014). Food fraud and “Economically Motivated Adulteration” of food and food ingredients. In Congresse TLo (Ed.), (Vols. 7–5700, R43358, pp. 1–40). Washington, DC: Library of Congress.
- Kasemsumran, S. , Kang, N. , Christy, A. , & Ozaki, Y. (2005). Partial least squares processing of near‐infrared spectra for discrimination and quantification of adulterated olive oils. Spectroscopy Letters , 38 , 839–851.10.1080/00387010500316189
- Kataoka, H. , Lord, H. L. , & Pawliszyn, J. (2000). Applications of solid-phase microextraction in food analysis. Journal of Chromatography A , 880 , 35–62.10.1016/S0021-9673(00)00309-5
- Lerma-García, M. J. , Ramis-Ramos, G. , Herrero-Martínez, J. M. , & Simó-Alfonso, E. F. (2010). Authentication of extra virgin olive oils by Fourier-transform infrared spectroscopy. Food Chemistry , 118 , 78–83.10.1016/j.foodchem.2009.04.092
- Martens, H. , & Naes, T. (1989). Multivariate calibration . Chichester: Wiley.
- Miller, J. N. , & Miller, J. C. (2005). Statistics and chemometrics for analytical chemistry . Edinburgh: Pearson Education.
- Movasaghi, Z. , Rehman, S. , & ur Rehman, I. (2008). Fourier transform infrared (FTIR) spectroscopy of biological tissues. Applied Spectroscopy Reviews , 43 , 134–179.10.1080/05704920701829043
- Naes, T. I. , Fearn, T. , Davies, A. M. (2002). A user friendly guide to multivariate calibration and classification . London: NIR Publications.
- Nieto, L. , Hodaifa, G. , & Lozano Peña, J. (2010). Changes in phenolic compounds and Rancimat stability of olive oils from varieties of olives at different stages of ripeness. Journal of the Science of Food and Agriculture , 90 , 2393–2398.10.1002/jsfa.v90:14
- Roggo, Y. , Chalus, P. , Maurer, L. , Lema-Martinez, C. , Edmond, A. , & Jent, N. (2007). A review of near infrared spectroscopy and chemometrics in pharmaceutical technologies. Journal of Pharmaceutical and Biomedical Analysis , 44 , 683–700.10.1016/j.jpba.2007.03.023
- Rohman, A. , & Man, Y. (2010). Fourier transform infrared (FTIR) spectroscopy for analysis of extra virgin olive oil adulterated with palm oil. Food Research International , 43 , 886–892.10.1016/j.foodres.2009.12.006
- Rohman, A. , & Man, Y. (2012a). The chemometrics approach applied to FTIR spectral data for the analysis of rice bran oil in extra virgin olive oil. Chemometrics and Intelligent Laboratory Systems , 110 , 129–134.10.1016/j.chemolab.2011.10.010
- Rohman, A. , & Man, Y. (2012b). Quantification and classification of corn and sunflower oils as adulterants in olive oil using chemometrics and FTIR spectra. The Scientific World Journal , 2012 , 1–6.10.1100/2012/250795
- Rohman, A. , & Man, Y. B. C. (2012c). Application of Fourier transform infrared spectroscopy for authentication of functional food oils. Applied Spectroscopy Reviews , 47 (1), 1–13.10.1080/05704928.2011.619020
- Rohman, A. , Man, Y. B. C. , Ismail, A. , & Hashim, P. (2010). Application of FTIR spectroscopy for the determination of virgin coconut oil in binary mixtures with olive oil and palm oil. Journal of the American Oil Chemists Society , 87 , 601–606.10.1007/s11746-009-1536-7
- Rohman, A. , Man, Y. B. C. , & Yusof, F. M. (2014). The use of FTIR spectroscopy and chemometrics for rapid authentication of extra virgin olive oil. Journal of the American Oil Chemists Society , 91 , 207–213.10.1007/s11746-013-2370-5
- Stevens, J. P. (2002). Applied multivariate statistics for the social sciences . Hillsdale, NJ: Erlbaum.
- Vlachos, N. , Skopelitis, Y. , Psaroudaki, M. , Konstantinidou, V. , Chatzilazarou, A. , & Tegou, E. (2006). Applications of Fourier transform-infrared spectroscopy to edible oils. Analytica Chimica Acta , 573–574 , 459–465.10.1016/j.aca.2006.05.034
- Wang, L. , Lee, F. , Wang, X. , & He, Y. (2006). Feasibility study of quantifying and discriminating soybean oil adulteration in camellia oils by attenuated total reflectance MIR and fiber optic diffuse reflectance NIR. Food Chemistry , 95 , 529–536.10.1016/j.foodchem.2005.04.015
- Wentzell, P. , & Vega Montoto, L. (2003). Comparison of principal components regression and partial least squares regression through generic simulations of complex mixtures. Chemometrics and Intelligent Laboratory Systems , 65 , 257–279.10.1016/S0169-7439(02)00138-7