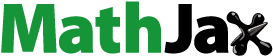
Abstract
The present case study sums up the results of an initial attempt to adapt the emerging technology of Augmented Reality (AR) for supporting routine operations performed in Danish slaughterhouse facilities. Our aim is to reveal the applicability of off-the-shelf components and programming platforms to the trimming and boning process for pork bellies. The AR technology has demonstrated lucrative applications in industrial QA procedures and even farm management applications appear to benefit from applying the technology. With the ever-increasing turnover of labour in the meat industry, we investigate here the application of AR-assisted production procedures as a potential management tool and support tool to assist a novice operator in a specific trimming operation. The case study concerns the trimming and cutting of pork bellies, a widely used and versatile procedure in the Danish pork meat industry. Many similar belly products made from similar raw materials are exported to specific customers and markets. Due to biological variability between pigs, final products are produced with variability in yield, despite the fact that the final product qualities are similar. The best management option is to use the correct raw material for each product, thus generating fewer by-products and increasing the volume/weight of the final product. The application of AR to the cutting operation appears to increase the production yield; however, the operators need training in order to benefit fully from the efficiency and capacity of the application rather than adopting the standard procedure of oral communication of instructions.
Public Interest Statement
The paper reports from an initial attempt to adapt Augmented Reality to support a food production routine performed on a Danish Slaughterhouse. To the authors’ knowledge it is for the first time demonstrated that food production routines might be optimised by this emerging technology. The pilot experiment also points out some drawbacks with the use of Smart Glasses in a production environment, drawbacks that must be addressed in future development of the AR/Smart Glass combination in order to gain the full potential of Augmented Reality in the slaughterhouse.
Competing interests
The authors declare no competing interests.
1. Introduction and literature review
Augmented Reality (AR) covers a vision-based information provision system that enhances one’s visual perception of the real world. This enhancement often includes features of the real world that are inaccessible to the operator in a specific operation. Several applications, classified here in four sections, are demonstrated in the literature (Furht, Citation2011).
(1) | The information providers of museums, cities, archaeological sites have also provided AR-based enhancements to the excavation sites and ruins. | ||||
(2) | Modern advertising and commercial information provision benefit from interactive augmentation to “passive” brochures. | ||||
(3) | Medical surgery benefits from revealing internal anatomical features of the patient during minimally invasive surgical operations. | ||||
(4) | Finally, mobile platforms have generated a vast range of applications for “edutainment” purposes. |
In the literature, AR concepts are often linked to the use of smart glasses which provide a hands-free operation option. However, this link is not a prerequisite for benefiting from the AR modality. The automotive industry has demonstrated the versatility of AR-based operator support, ranging from conventional quality assurance (QA) support and assistance to the driver of the vehicle. The QA applications have demonstrated the potential of supporting the inspection procedures for welded parts before assembly, a solution that assists the operator with the projection of relevant inspection points directed at the welded part from a head-mounted laser pointing device and a vision-based position localiser (Hofhauser, Steger, & Navab, Citation2015; Tönnis, Citation2008). The solution relies on a highly accurate tracking of the inspection part to define the transformation between the coordinate system of the welded part and the position of the projection device, a tracking that may be provided by fiducial marks on the projection helmet. The driver support includes a “Top Gun-inspired” application of the head-up display to augment the driver’s field of view with supporting information relevant for safe driving of the vehicle. The information may originate from sensors (speed, fuel level, temperature) within the vehicle or from external sensors and systems like GPS, traffic warning services or even commercial advertising sources. The automotive applications illustrate the versatility of visualisation modalities in AR. The visualisation modalities included in the present case study range from a simple passive video monitor to binocular see-through, interactive smart glasses.
The present highly interdisciplinary case study contains several elements ranging from basic meat cutting with a knife, information tracking and visualisation, and image analysis to computer tomography and automated volume segmentation of lean meat content. We explain the vital components in detail in the sections below.
To the author’s knowledge, no current applications of AR exist in the food production industry. However, other industrial applications, such as the warehouse outbound picking application, have inspired the present study because of their potential impact on error reduction. Error reduction in terms of meat trimming applications results in improved yield of the raw material, a parameter that has a significant impact on the bottom line of the slaughterhouse facility.
1.1. The application
1.1.1. Trimming and cutting of Danish pork bellies
Denmark’s annual production of approximately 20 million pigs contributes substantially to the country’s export earnings. In the low margin international market for pork meat, it is vitally important that the slaughterhouse is capable of optimising the yield of any raw material. In other words, it must select the most suitable raw material for each and every final product, using the least amount of production effort to produce the largest volume of final product.
One simple introduction to the product range may be found in the ESS FOOD catalogue (ESS Food, Citation2015), a de facto standard for final meat products covering a substantial part of the international trade in pig meat. The present case study concerns the production of three different final belly products from a selection of three different raw materials, each final product produced from each selected raw material. The final products differ in minor details, such as size, shape, fat cover thickness and deboning process. The raw material differs essentially in weight and lean meat content. Consequently, the manual process needs to adapt to differences in the raw material in order to generate a consistent quality of the final products. This operator adaptation to raw material differences is a pivotal point in this case study. Since many of the differences are concealed subsurface, the operator needs to make empirically based estimations to assess hidden details such as the subcutaneous fat thickness.
1.1.2. The management challenge
The turnover of labour presents a significant challenge for the slaughterhouse management in terms of optimising the total yield, a challenge that increases further during vacation periods. Communicating written instructions on producing a versatile range of products to novice operators is a particularly important task. The development of a technology solution that eases and optimises this task will be of specific interest to the floor management. AR-based technology may potentially provide such a solution.
One other issue related to floor management in Danish slaughterhouses is the linguistic challenge resulting from the ever-increasing range of ethnic contributions to the total workforce. A suitable AR-based solution also should address this topic.
A more fundamental challenge faced by the pig meat production industry is the somewhat coarse level of specific information related to the raw material. In the Danish meat production industry, the raw material is sorted into groups according to total lean meat content and weight of the entire carcass, and then the specific belly quality is predicted from these figures. The prediction relies on anatomical coherence between the backfat layer thickness and belly quality, a coherence that may change over time between calibrations for manual dissection trials.
1.2. The augmented reality environment
The term AR is somewhat unclear in the literature, with some authors restricting the term to use in combination with Smart Glasses and other authors using the term with greater versatility. Here, we will stick to the versatile understanding of augmenting information otherwise hidden from an operator, which is relevant for the optimisation of the procedure at hand. In this case study, we apply off-the-shelf AR components only.
1.2.1. Off-the-shelf components
The AR application is based on the Creator software suite and the Junaio display channel (Metaio GmbH, Citation2014). The suite includes several relevant tools for displaying visual information to the user. In this case study, we use text, simple lines and fat thickness maps projected onto the raw material. Tracking of individually adapted information onto the products is performed with the tracking feature included in Creator, based on an image referred to here as trackables. The trackables consist of a set of simple colour images of each product, one from each surface (the meat surface and the fat surface). Although alternative 3D-tracking features are included in the Creator package, the shape of the raw material, only a few centimetres high, compared to the distance of operation, means that the basic 2D image tracking is used for this case study.
The display channel denotes the method of providing the correct information to the operator. The display channel used is the Junaio software, a shareware package made for mobile platforms, i.e. tablets, smart phones and smart glasses. Through Junaio, the information is transmitted to the operator while the operation is being performed. The choice of information is made by a specific QR code scanned by the Junaio software, one individual QR code for each operator. Stored under each QR code, the selection of trackables for each operator is wireless accessible on a public data network server.
The information transmitted by Junaio may assume many forms; here we use written instructions, position of cutting lines and a coloured thickness 3D map of the fat cover.
1.2.2. Visualisation of thickness
The 3D maps are made from CT scanning of the raw material. The image stacks (tomograms) from the CT scanner, stored in Dicom file format, form the input to a Python segmentation programme, classifying the tissue density into three classes by simple thresholding: meat, fat and bone. The Dicom format references to a standardised density scale, the so-called Hounsfield scale (HU), which is explained below. The Hounsfield units for the raw material range from −150 to 0 HU representing fat tissue, from 0 to 120 HU representing meat, and above 120 HU representing bone. Based on this segmentation, the fat thickness is calculated in a fine grid (1 × 10 mm) and shifted to a non-linear colour-coding. The image stack also forms the basis for generating a surface mesh representing the top surface of the skin side of the raw material. The surface mesh is stored as an object file with the colour-coded fat thickness as texture parameter forming a 3D model to impose on the relevant trackable in the Creator software suite.
The 3D surface is mapped to the 2D trackable using a supervised semiautomatic process, thus creating a link between the tracked meat and the artificial augmentation. The link between the 3D surface and the 2D trackable assumes that the meat is lying flat on a table, a reasonable assumption in most cases. This removes three of the six degrees of freedom (Z-translation, X- and Y-rotation), leaving only the information available in 2D (two translational axes and one rotational axis).
1.2.3. Smart glasses
One of the modalities in the case study is smart glasses, often referred to as “a hands-free smart phone”. It is a type of wearable suitable for two-hand applications. Smart glasses may assume many forms, and a detailed review can be found in (Inbar, Citation2015).
In this study, two models of smart glasses are applied, representing monocular and binocular types of glasses. One is the Vuzix M100 (www.Vuzix.com), a wireless monocle device and the other is the Epson Moverio BT-200 see-through binocular (www.Epson.com). Both devices are off-the-shelf commercially available devices that include software development open source platforms and some generic ready-to-use options. One option included in both devices is an APP installer which makes the Junaio software accessible on the devices.
1.3. The tracking
One important parameter in AR is the tracking feature, a necessary component in the software. In this study, it is responsible for the alignment of information to the individual raw material. The alignment must be in space, since the raw material is a spatial object (like most real-life objects).
1.3.1. Vision tracking
Due to the flattened shape of the belly products, we chose to use simple RGB images that show the surface texture of the raw material. In previous experiments (Larsen, Hviid, Jørgensen, Larsen, & Dahl, Citation2014), we have shown that the surface texture is a relatively robust identifier for meat products, especially the meat side texture. We made the images in a set-up using a fixed size ratio which ensured a well-defined transformation geometry between images and the surface mesh generated from the CT scanning image stacks. The two-way mapping from augmented colour-coded surface to trackable makes it possible to update the position and orientation of the generated surface when the real meat is handled, provided it ends up lying flat on the work surface.
1.4. The CT scanning
Computer tomography is an X-ray-based imaging modality capable of revealing internal details in volume objects. It was developed for medical applications but has since emerged in other industries, e.g. homeland security (Singh & Singh, Citation2003) and veterinary clinics (Wright & Wallack, Citation2007). Very recently, RD teams in Denmark and Spain have published research work on the development of scanners for online applications in the meat industry (Mosbech, Ersbøll, & Larsen, Citation2011; Picouet, Muñoz, Fulladosa, Daumas, & Gou, Citation2014).
1.4.1. Lean meat percentage (LMP) of the raw material
CT scanning has great potential in the segmentation of fresh meat due to the high contrast between meat, fat and bone tissues. Recently, several European institutions have collaborated on developing methods to determine the lean meat content of a pig carcass using a CT-scanned image stack as input. The image stack consists of grayscale images with a fixed reference to the HU, an attenuation scale with two reference points: air equals −1,000 HU and water equals 0 HU. The tissues of the carcass are distributed on the scale with fat ranging from −150 to 0 HU, meat ranging from 0 to 120 HU and bone distributed at values above 120 HU. The modality images can be segmented into these three main tissue components, and, from the segmented volumes, a very detailed description of the spatial distribution may be made: measurement of tissue volumes, geometrical features (e.g. thicknesses, weight and distances) at anatomical fix points.
The weight measurement is based on a simple equation made from the segmented images. The individual raw material weight (W) is determined using a scale. Combining the segmented tissue volumes Vf, Vm and Vb, respectively, and the weight from the scale gives the equation (Vester-Christensen et al., Citation2008).
where bf, bm and bb represent an average density for each segmented volume. The density values are determined by simple statistical regression. Using the estimated density values, any virtual final product may be “weighed” on the computer. This feature makes CT scanning a valuable tool for setting up sorting schemes in the slaughterhouse based on the simulation of virtual cuts and cutting of the raw material (Christensen et al., Citation2010).
From the above equation, the lean meat percentage (LMP) is defined as
The sorting of the raw material can be carried out based on the weight and the LMP.
2. Applied research methods
The case study assesses the potential of using an off-the-shelf AR technique to assist manual operations in the meat production industry. Specifically, it has potential use as a management tool in
● | outlining manual procedures to untrained/novice operators | ||||
● | assessing revealed details | ||||
● | optimising yield |
The case study included six operating teams, each team providing a different kind of assistance to the cutting operation. Four of the teams included AR assistance, one team was supported by a visual monitor and the final team was assisted by oral instructions only and was used as a control team in this study.
The raw material for the study was selected from three sorting groups and was purchased from a Danish slaughterhouse. The raw material was CT-scanned on our medical scanner (Toshiba Aquilion S16). The scanner was placed in a mobile trailer, providing an air-conditioned environment at 15°C for the CT scanning and for the vision registration of both surfaces. The raw material was CT-scanned, photographed with the RGB camera from both sides and then frozen (−20°C) until the time of experiment. In the meantime, the visualisation of the fat cover was performed using segmentation and thickness measurement in a Python-based image processing software developed specifically for this experiment and using the free 3D renderer Blender to visualise the coloured texture. Linking of the surface mesh to the trackable (RGB image) was performed together with the cutting instructions on the meat side using the Creator software. The raw material for the three final products was divided randomly into six different sub-samples so that each team had access to three sub-samples, one for producing each of the three final products. The instructions for each team were stored under a QR code on a public access data server, the QR code being readable with the Junaio software. Scanning the QR code with Junaio from the smart glass or tablet gives the operators access to the information on the public data server, with the information displayed on the screen of the equipment.
The operators were untrained in using smart glasses, but they participated in a professional training course (3rd grade). They tried the vision function of the binocular glasses the day before the experimental session, but without practising the tracking of meat products. Therefore, on the day of the session the four teams with AR assistance had to familiarise themselves with the modality experience of the monocle, binoculars or tablet, including tracking of the raw material.
A simple vision monitor assisted one team. The monitor displayed the same level and type of information that was made available to the AR-assisted teams but without real-time tracking of the real object. One team was assisted by oral instructions only, given to all teams before the cutting session. This team represents the control team that uses the support tools provided for most operations in the Danish meat industry.
Before cutting the raw material, each piece of material was weighed on a scale, and, after cutting and trimming, the final product was weighed on the same scale. Each team had the same amount of time available in which to perform the operations, and therefore a simple counting of the final products indicated the capacity of the team/modality.
3. Results
The operator assistant weighed each raw material on a scale before trimming. The three sorting groups cover the weight range shown in Table .
Table 1. Weight range of the raw material for the case study
The results from the weight estimation using the CT scanner give the following relation Figure :
Figure 1. Relation between the weights of each product determined with a scale and estimated from CT scanning using the segmented images, respectively.
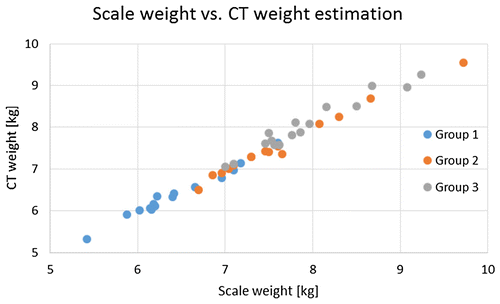
The mean difference offset is 5 g with an SD of 135 g, respectively. This result supports the application of weight estimation of virtual products based on CT scanning. The difference includes the variable loss of water during thawing of the raw material before processing.
From the CT scanning, we estimated the LMP of each raw material. The results are shown in Table .
Table 2. LMP range of the raw material for the case study
The supply of raw material took place in a random order within the sub-sample of the operator. The AR software provided the identification of each individual object despite the random order.
The tracking feature of the Creator software was challenged in this study. The meat side of the products was tracked in most cases rather than the skin/fat side. The tracking failed in the latter case, probably due to the lack of robust features on the skin/fat surface. As alternative tracking, the operators were provided with a photo of the products accounting for the base that all the trackables were made from. This alternative ensured full identification of the skin/fat side of all products, but might potentially have had an impact on the performance of the groups.
The information generated for each product was of three types: the colour-coded fat cover, the notification of recipe ID and corrective actions according to the standard recipe, where relevant, and finally position identification of the cutting lines (see Figure ). In this particular case, the size of the raw material is within the range of the final product (1,853), and therefore only a final trimming of the hip end is required, indicated by the black line. This reduces the total processing time required for this product.
Figure 2. The augmented information shown for the assisted operator to show cutting instructions (top) for the meat side and colour-coded fat layer thickness (bottom) to assist the trimming to specific thickness of the fat side of the product.
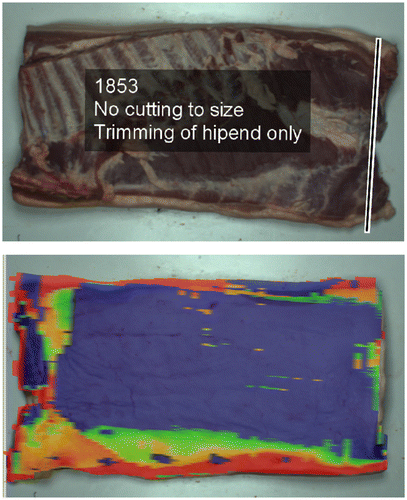
The corresponding fat side is overlaid with a colour-coding with a non-linear transformation: the blue colour indicates thicknesses below 7 mm, so no fat trimming in the blue areas is required. The range between 7 and 15 mm is indicated in green to yellow colours, and orange to red colours indicate areas with a subcutaneous fat thickness of above 15 mm. The product recipe requires a fat cover of no more than 7 mm. The operator for this particular product is therefore informed about where to trim and, using coarse measurements, how much fat needs to be removed by trimming.
The final products are weighed on the same scale after production to quantify the production yield for each operator team. The timeframe for production was the same for all teams, and therefore the number of products produced may be taken as a measure of the team capacity (products/time). The yield for each team is estimated from the ratio of the final product quantity relative to the weight of the raw material, measured in %. The higher the yield, the better the use of the raw material.
To analyse this study, the six teams are divided into three groups with respect to supervision modality: the oral supervision team, the monitor supervision team and the four AR-supported teams as one average team Table .
Table 3. Team capacity and yield for each support modality
These results lead to a very preliminary conclusion of the overall performance of the three support modalities: oral, vision monitor and AR. All AR components are off-the-shelf commercially available components, with no training of the operators in using the modality for the given task.
The product of the capacity and yield gives an indication of the ranking of the modalities, with the simple vision monitor appearing to be the most efficient support for inexperienced operators. Due to the very low capacity of AR-supported teams, the higher yield is not compensating enough to outperform the simple monitor-supported team.
4. Discussion
The case study must be considered as a first time evaluation of the accessibility of off-the-shelf products when using AR as a plant floor management tool in the food industry. The results indicate a significant yield improvement potential if the operators are supported by guidance information about the raw material. In this study, we provide support with a high level of detail and corrective actions, relevant for future installations of online CT scanners. Other sensor-based information-providing methods, e.g. ultrasound (Busk, Olsen, & Brøndum, Citation1999), radiology (Kröger, Bartie, West, Purchas, & Devine, Citation2006) or vision (Font i Furnols & Gispert, Citation2009), are already commercially available and may also provide usable information about the raw material.
The display of information used in the present case study is realised at two levels: low level with a simple vision monitor placed in front of the operator and high level via smart glasses that include AR and real-time tracking. The former method, despite its simplicity, has demonstrated a substantially increased yield, whilst maintaining a high-capacity performance. The latter has also demonstrated an increased yield, although here at the cost of a much lower capacity performance. However, this may be improved through training.
One other reason for the low capacity was the poor performance of the tracking method used for this application. Tracking is a key feature, and training is a prerequisite for a beneficial use of AR as a management tool. This is an area with considerable potential, and we believe that different methods or components may result in significant improvements.
As part of the case study, the operators were interviewed after the experiment about their perception of the emerging modalities. They felt that the (monocle) smart glasses were difficult to use, since the field of view was too unstable during the operation. One binocular user commented: “I feel remote from my hands; it’s not me doing the cutting”. The see through type of smart glasses in combination with the fat cover colour-coding may benefit from dynamic, updated information based on the performed trimming operations and a real-time adapted colour map.
5. Conclusions
From this case study, it is not possible to draw any strict conclusions, since most of the modules included in the study were off-the-shelf components. However, the study points towards some potentials and directions for future work in fulfilling the potential of AR as part of a next generation management tool for the food industry.
First of all, the study points out some of the drawbacks of the present orally based supervision of production operators in most slaughterhouses. The main drawback is the sub-optimal use of raw material. The loss of product volume may amount to substantial figures in high-capacity production lines. One other issue is the consistency over time of oral communication of cutting instructions. It appears that non-familiar recipes may gradually be converted into more well-known instructions over time, which is obviously not desirable.
Next, the study indicates the necessity of an adaptive period for getting used to the smart glasses as a prerequisite for a beneficial use as a guiding tool in manual (high speed) trimming operations. Despite a significant difference between the two types of smart glasses (monocle vs. binocular), the potential of using these emerging technologies as a beneficial management tool is not demonstrated in this study.
Thirdly, the potential of the simple monitor-based modality appears to present an attractive off-the-shelf option for high-speed process guidance when combined with the relevant information level used in this case study. The real-time tracking feature provided by the smart glasses does not appear to be a prerequisite for obtaining a better yield of the raw material. It may, however, be speculated that, without the problem discovered in this study (failed tracking and unfamiliarity with the AR equipment), even higher yields may be obtained.
Lastly, the development of improved tracking and tracing of information is a prerequisite for realising the full potential of AR-based assistance for manual food production operations and management.
Acknowledgements
This case study could not have been carried out without the generous assistance of the University College Zealand, which is responsible for the professional training of Danish butchers. Furthermore, we are deeply grateful for the painstaking assistance provided by Benny Lauritzen and Max Petersen, DMRI.
Additional information
Funding
Notes on contributors
Lars Bager Christensen
At Danish Meat Research Institute, LBC, MSc, PhD, has been involved in sensor projects throughout the entire electromagnetic spectrum since 1998. Very recently, his main effort has been application and use of detailed information of the tissue distribution in meat products to optimise a manual process in the slaughterhouse. DMRI can obtain the detailed tissue distribution by using CT or ultrasound, depending on the application and specific raw material. Presentation of the information to an operator in an intuitive manner has demonstrated the need for an interactive knife to reflect the effect of cutting. The knife, pat. appl., is developed on top of a standard whizzard knife to quantify the thickness of the trimmed fat layer and to update the coloured fat cover map accordingly.
References
- Busk, H., Olsen, E. V., & Brøndum, J. (1999). Determination of lean meat in pig carcasses with the Autofom classification system. Meat Science, 52, 307–314.10.1016/S0309-1740(99)00007-8
- Christensen, L. B., Erbou, S. G. H., Vester-Christensen, M., Hansen, M. F., Darré, M., Hviid, M., & Olsen, E. V. (2010). Optimized workflow and validation of carcass CT scanning. Proceedings of 56’th ICoMST. Jeju: ElseVier.com.
- ESS Food. (2015). The Pork catalogue. Retrieved from http://www.e-pages.dk/essfood/1/html5/1/
- Font i Furnols, M. F., & Gispert, M. (2009). Comparison of different devices for predicting the lean meat percentage of pig carcasses. Meat Science, 83, 443–446.10.1016/j.meatsci.2009.06.018
- Furht, B. (Ed.). (2011). Handbook of augmented reality. New York, NY: Springer.com. 10.1007/978-1-4614-0064-6
- Hofhauser, A., Steger, C., & Navab, N. (2015). Edge-based template matching and tracking for perspectively distorted planar objects. In Lecture notes in computer science. TU München. Retrived from far.in.tum.de/pub/hofhauser2008isvc/hofhauser2008isvc.pdf
- Inbar, O. (2015). Smart glasses market 2014: Towards 1 billion shipments. Retrieved from http://www.Augmentedreality.org
- Kröger, C., Bartle, C. M., West, J. G., Purchas, R. W., & Devine, C. E. (2006). Meat tenderness evaluation using dual energy X-ray absorptiometry (DEXA). Computers and Electronics in Agriculture, 54, 93–100.10.1016/j.compag.2006.09.002
- Larsen, A. B. L., Hviid, M. S., Jørgensen, M. E., Larsen, R., & Dahl, A. L. (2014). Vision-based method for tracking meat cuts in slaughterhouses. Meat Science, 96, 366–372.10.1016/j.meatsci.2013.07.023
- Metaio GmbH. (2014). Retrieved from http://www.metaio.com/fileadmin/upload/documents/promo_book/ARDemoBooklet-2014.pdf
- Mosbech, T. H., Ersbøll, B. K., & Larsen, R. (2011). Computed tomography in the modern slaughterhouse (Published doctoral dissertation) (p. 147). Department of Informatics and Mathematical Modelling, Technical University of Denmark, Kongens Lyngby, ( IMM-PHD-2011; No. 258).
- Picouet, P. A., Muñoz, I., Fulladosa, E., Daumas, G., & Gou, P. (2014). Partial scanning using computed tomography for fat weight prediction in green hams: Scanning protocols and modelling. Journal of Food Engineering, 142, 146–152.10.1016/j.jfoodeng.2014.06.012
- Singh, S., & Singh, M. (2003). Explosives detection systems (EDS) for aviation security. Signal Processing, 83, 31–55.10.1016/S0165-1684(02)00391-2
- Tönnis, M. (2008). Towards automotive augmented reality. TU München: München.
- Vester-Christensen, M., Erbou, S. G. H., Hansen, M. F., Olsen, E. V., Christensen, L. B., Hviid, M. S., … Larsen, R. (2008). Virtual dissection of Pig Carcasses. Meat Science, 81, 699–704.
- Wright, M., & Wallack, S. (2007). The little book of CT in veterinary medicine: A practical guide to CT technique for technicians and veterinarians. Westbrook, ME: IDEXX Laboratories.