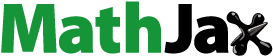
Abstract
Evaluating stability performance and range of adaptation is becoming increasingly important. If cultivars are to be selected for a large group of environments, stability and mean yield across all environments are more important than yield for specific environments. This study was conducted to assess the stability of 3 extra early maize under high and low nitrogen environments. The experiments were conducted at two locations in 2009 and 2010 wet season under high and low N applications. Additive Main Effect and Multiplicative Interaction (AMMI) and GGE biplot were used to assess the genotype-by-environment interactions. The mean square analysis reveals a significant difference for environments effect and a lack of significant mean squares for varietal effects under high and low N and across the research environments. 2004-SYNEEW gave the highest grain yield under combined analysis. Environment sum of squares under AMMI model accounted for about 98.79% of the treatment sum of squares. The first PCA scores accounted also for about 98.75% of the interaction effect. 2004-SYNEEW had the least PCA scores and the most stable with yield above average under the GGEbiplot model. Both models identified 2004-SYNEEW as the most stable variety.
Public Interest Statement
Maize is a major staple in Nigeria but the yield obtained in farmers field is very low. This is majorly due to low soil fertility and low rate of fertilizer application using both mineral and organic fertilizers. The Northern Guinea Savanna is the major maize producing region in Nigeria and other Sub-Saharan African Countries. Recently, production of maize is on the increase due to availability of early/extra early maize. Extra early maize has the potential to escape end of season drought which is usually experienced in Sudan Savanna as a result of short duration of rainfall. This study therefore aimed to identify extra early maize genotypes that are high yielding under low fertility soils and can maintain this yield across different locations in Sudan Savanna of Nigeria. The research identified a potential extra early maize variety that was high yielding and stable under high and low nitrogen application.
Competing Interests
The authors declare no competing interest.
1. Introduction
Conduct of multi-environment trials (MET) for all major crops throughout the world is important in achieving high yielding and stable cultivars (Fikere, Tadesse, & Letta, Citation2008; Yan, Cornelius, Crossa, & Hunt, Citation2001). The main purpose of MET is to identify superior cultivars for recommendation to farmers and to identify environments that best represent the target environment for a particular variety (Yan et al., Citation2001). If cultivars are to be selected for a large group of environments, stability and mean yield across all environments are more important than yield for specific environments (Piepho, Citation1996). Several statistical methods have been developed for anlaysing GEI or phenotypic stability (Becker & Leon, Citation1988; Crossa, Citation1990; Lin, Binns, & Lefkovitch, Citation1986; Piepho, Citation1998; Westcott, Citation1986). These methods can be divided into two major groups, univariate and multivariate stability (Lin et al., Citation1986). Joint regression is the most popular among univariate methods because of its simplicity of calculation and application (Aremu, Citation2005; Ariyo, Citation1987; Ariyo & Ayo-Vaughan, Citation2000; Eberhart & Russell, Citation1996), whereas Additive Main Effect and Multiplicative Interaction (AMMI) and Genotype and genotype-by-environment (GGE) biplot methodology have recently being used as the main alternative multivariate approach to the joint regression analysis in many breeding programs (Annicchiarico, Citation1997; Badu-Apraku et al., Citation2013, Citation2015; Yan & Kang, Citation2003).
The AMMI model combines the conventional analysis of variance for additive main effects with the principal components analysis (PCA) for the non-additive residuals into a single analysis (Crossa, Gauch, & Zobel, Citation1990; Gauch, Citation1993; Piepho, Citation1996; Zobel, Wright, & Gauch, Citation1988). This model has proved superior and more effective especially when both the main effects and interactions are important. AMMI analysis has been reported to have significantly improved the probability of successful selection (Gauch & Zobel, Citation1997) and was considered appropriate if one is interested in predicting genotype yields in specific environments (Annicchiarico, Citation1997). Thus, it helps in summarizing the pattern and relationship of genotypes, environment and their interaction in a graphical way (Gauch & Zobel, Citation1996). Gauch (Citation1992) reported that AMMI is effective for several purposes such as understanding GEI, improving the accuracy of yield estimates, increasing the probability of successively selecting genotypes with the highest yields, imputing missing data and increasing the flexibility and efficiency of experimental designs. Ultimately, these advantages imply larger selection gains in breeding research and more reliable recommendations in Agronomy (Ntawuruhunga, Rubaihayo, Whyte, Dixon, & Osiru, Citation2001).
The “GGE biplot” methodology developed by Yan and Kang (Yan & Kang, Citation2003) is a recent addition to the tools for analysis of MET. “GGE” refers to the genotype main effect (G) plus the genotype × environment interaction (GE), which are the two sources of variation that are relevant to cultivar evaluation. The biplot methodology (Gabriel, Citation1971) simultaneously displays both the genotypes and the environment in a graphic form and inferences about their interactions can be made (Nwachukwu, Ikeorgu, & Asiedu, Citation2006). GGE biplot is superior to the AMMI model in mega-environment analysis and genotype evaluation because it explains more G + GE interaction and has the inner-product property of the biplot (Yan, Kang, Ma, Woods, & Cornelius, Citation2007). The GGE biplot technique has been extensively used in the analysis of multi-location trials of wheat (Yan et al., Citation2001), yam (Nwachukwu et al., Citation2006), rice (Sanni, Citation2008), okra (Adekoya, Citation2008), cowpea (Akande & Balogun, Citation2009) and maize (Badu-Apraku, Fontem, Akinwale, & Oyekunle, Citation2011; Badu-Apraku et al., Citation2013, Citation2015).
The possible impact of genotype-by-environment interaction (GEI) analysis on maize grain yield improvement can be used to identify the constraints to productivity and hence formulate strategies for breeding and agronomic research. Performance of maize under different fertilization will go a long way in assisting small scale farmers in increasing their productivity per unit area. The research was conducted to study the stability performance of three commonly cultivated extra early maize varieties under high and low nitrogen level in the Sudan Savanna.
2. Materials and methods
The field trials were conducted in the 2009 and 2010 rainy seasons at the Bayero University, Kano (BUK) Agricultural Research Farm (11°59′N; 8°25′E; 466 m asl) and Komau Village, Shanono Local Government Area, Kano State (12°03.53′N; 8°06′E; 543 m asl), both located within the Sudan Savanna agro-ecological zone of Nigeria. Three extra maize varieties (TZEEW-POPX, 2004-SYNEEW, and 2000-SYNEEW) and two levels of N for low (30 kg N ha−1) and high (120 kg N ha−1) application were used (Table ). The varieties were laid out in Randomized Complete Block Design and replicated three times. The plot size was 21 m2 (5.25 × 4 m) comprising of 7 ridges (0.75 m apart) of 4 m length and the net plot used for taking measurements was 9 m2 so as to eliminate border row effects. The maize varieties were planted at a spacing of 75 cm between rows and 25 cm within rows. Two seeds per hole were sown and later thinned to one seedling per stand at two weeks after planting. Nitrogen Fertilizer was applied in the form of urea as per treatment in two splits (2 and 6 WAS). Phosphorus (P2O5) and potassium (K2O) were applied using SSP and MOP, respectively. The experimental fields were cleared, harrowed, ridged and thereafter sprayed with a pre-emergence herbicide Primextra (Atrazine + Metolachlor) at the rate of 4 L per hectare before planting. Supplementary hoe weeding was carried out.
Table 1. Characterization of the 8 environments used in investigation
Composite soil samples were collected at each of the locations, described, characterized and classified to the sub-group level. All soil samples were air-dried on trays. After drying, the clods were broken using a porcelain mortar and the ground soil sieved through a 2 mm mesh sieve. The sieved samples were stored in plastic containers and taken to the laboratory for physical and chemical analysis using standard procedure (IITA, Citation1982). Data was recorded on plant height, days to anthesis and silking, anthesis silking interval (ASI), shelling percentage, grain yield and stover yield. The data collected were analyzed separately for each nitrogen level using analysis of variance (ANOVA). A combined ANOVA was done across the nitrogen levels. In the analysis, environments and replications were considered as random effect while varieties were considered as fixed effects. The analyses were done using SAS 9.2 (SAS Institute, Citation2010). The data collected were further subjected to Additive Main effect and Multiplicative Interaction (AMMI) analysis using Genstat 16th Edition. In this analysis, each experiment was considered an environment, therefore making a total of eight environments. For any particular genotype - environment, the main effect equals the cultivar mean plus the environment mean minus the grand mean. The interaction is the cultivar IPCA score multiplied by the environment score. When a cultivar and an environment have the same sign on their respective first IPCA axes, their interaction is positive; if different, their interaction is negative. An AMMI biplot is a graph where aspects of both varieties and environments are plotted on the same axes so that interrelationship can be visualized. Varieties or environments with large PCA 1 scores, either positive or negative have large interactions, whereas varieties with PCA 1 score of zero or nearly zero have small interaction (Crossa, Citation1990).
The AMMI statistical model equation used was:
where Yger = the trait rating of genotype g in environment e for replicate r; μ = the grand mean; αg = the mean deviation of genotype g (genotype means minus grand mean); βe = the mean deviation of the environment e; n = the number of PCA axes retained in the model; λn = the singular value for PCA axis n; ygn = the genotype g eigenvector value for PCA axis n; δen = the environment e eigenvector values for PCA axis n; pge = the AMMI residuals and Єger = the residual error.
The eigenvectors are scaled as units of error and are unitless, whereas λ has the units of the yield.
The data collected were also subjected to the “GGE” biplot analysis to visualize the GE interaction. The analyses was done using GGEbiplot, a Windows application that fully automates biplot analysis (Yan et al., Citation2001). In this biplot, the genotype main effect are the primary effects and the first principal component (PC1) derived from subjecting the residual to singular value decomposition (SVD) of the environment-centered data as the secondary effect (Mandel, Citation1961).
Mandel (Citation1961) presented the following model for the analysis:
where Yij = the average yield of genotype i in environment j; βj = the average yield of all genotypes in environment j; αi = the main effect of genotype i; bj = the regression coefficient of the environment centered yields (i.e. Yij − βj) within environment j on the genotype main effects (αi); λ1 Σi1ηj1 = the first principal component from singular value decomposition (SVD) of the residual.
3. Results
The soils at both locations were classified as Typic Plinthustalfs using the USDA Soil Taxonomy (Soil Survey Division Staff, Citation2002). The pH of the soil in BUK was 6.2, which makes the soils slightly acidic (Table ). At Komau the soil pH was 5.5 which makes the soils strongly acidic with a loamy texture (Table ). The soil also had a low total N content (0.68 g kg−1), low organic carbon (8.22 g kg−1) and low available P (8.75 mg kg−1). At Komau, the soil had total N content (0.65 g kg−1). The organic carbon and available P contents were even lower than the values at BUK (7.32 g kg−1 and 6.25 g kg−1, respectively).
Table 2. Physico-chemical properties of sites
The mean squares of some agronomic traits of maize under high nitrogen level are presented in Table . Significant mean squares were observed between the environments across all trait except for plant height. There was a lack of significant mean squares between varieties for the traits measured except for ASI. The mean squares for interaction between environment and varieties were not significant. Significant mean was observed for all traits measured across environments except for ASI and this was the only significant trait across varieties under low nitrogen (Table ). In the combined analysis (Table ), there was a lack of significant mean squares for ASI across all environments. Plant height and ASl had significant mean squares for varieties. Generally, there was no significant mean squares for GEIs at both level of nitrogen applications and across the combined levels of applications.
Table 3. Mean squares of some agronomic traits of maize under high nitrogen level
Table 4. Mean squares of some agronomic traits of maize under low nitrogen level
Table 5. Mean squares of some agronomic traits of maize across environments
The mean performance of the varieties for some agronomic traits are presented in Table . The mean plant height under high N was higher than low N by about 28% . The mean plant height across the environments was 1.45 m. 2004-SYNEEW produced the tallest plants under high N (1.67 m) while TZEEW-POPX was the highest under low N (1.30 m). But across the management conditions, 2004-SYNEEW produced the tallest plants (1.47 m). The coefficient of variation under the three conditions was about 10%.
Table 6. mean performance of some agronomic traits of maize under high N, low N and across environments
The mean days to anthesis and silking under high N were 47.9 and 50.7 days, respectively. Under low N the mean days to anthesis and silking were 54.1 and 57.3 days, respectively. Days to anthesis and silking of 51.1 and 53.9 respectively was observed across environments. The mean shelling percentage of the varieties under high N was about 20% more than the mean shelling percentage under low N (Table ). The mean shelling percentage across the research environment was 9% less than the mean under high N and 11% greater than the mean under low N. The mean grain yield of the varieties obtained under high N was more than that obtained under low N by a margin of 57%. Two varieties 2004-SYNEEW and 2000-SYNEEW produced above average yields (2,604.82 and 2,594.30 kg ha−1) in the combined analysis across environments. While the yield of TZEEW-POPX was close to the average yield.
Table 7. Mean performance of yield and yield components traits of maize under high and low nitrogen level
Grain yield across the environments was 27% less than the grain yield under high N. There was a highly significant mean squares for treatments and environments (Table ). The mean squares for genotypes (varieties) and interactions were not significant. The first PCA score accounted for 98.75% of the interaction sum of squares while the environments accounted for 98.79% of the treatments sum of squares. The mean yield of the varieties ranges from 2,547 to 2,605 kg ha−1 for TZEEW-POPX and 2004-SYNEEW, respectively (Table ). 2004-SYNEEW had the least first PCA scores while 2000-SYNEEW had the highest scores. Table shows the interactive PCA scores of environments. The mean yield ranges from 391 to 4,781 kg ha−1 for E7 and E1, respectively. E4 had the largest PCA scores followed by E1 while E7 had the least.
Table 8. AMMI analysis of variance
Table 9. Interactive PCA scores of varieties
Table 10. Interactive PCA scores of environments
From the GGE biplot, 2004-SYNEEW was more stable and had a yield above average (Figure ). 2004-SYNEEW had a good performance in E4 while 2000-SYNEEW in E8. The performance of TZEEW-POPX was low but was relatively good at E5 and E7 (Figure ). From Figure , E5 and E7 are related and E4 and E1 are also related.
4. Discussion
The significant difference observed for the environments under high N is an indication that the environments were highly variable and this may be due to differences in rainfall of the environments as shown in Figure (A)–(D). The lack of significant difference for varieties implies that the varieties had similar performance and this was also evident by the non-significant variety by environment interactions observed. Similar trend was observed under low N. The lack of significant differences between the varieties indicates that the response of these varieties to N is the same. This may also indicates similar physiological responses of the maize varieties. Shaibu, Rabiu, and Adnan (Citation2015) reported lack of significant differences in physiological responses of maize grown under the same environmental condition with uniform fertilizer application. The combined analysis across the eight environments also indicates that each environment is unique and this may be partly due to the rainfall observed at Komau in 2009.
Figure 4. Rainfall distribution of the experimental sites (A) Komau 2009, (B) Komau 2010, (C) Kano 2009, and (D) Kano 2010.
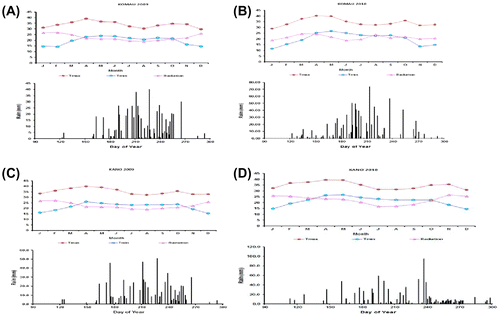
Grain yield of 2004-SYNEEW was the highest under low N and across the research environments but was the least under high N although there was no significant difference between the varieties. This could be possibly due to inherent ability of the variety to tolerate low nitrogen application.
From the AMMI analysis, the environment accounted for about 98% of the sum of squares while genotype was only 2%. This is in accordance with the report of Kaya, Akcura, and Taner (Citation2006), Farshadfar, Mohammadi, Aghaee, and Vaisi (Citation2012) and Mohamed, Said, and Amein (Citation2013). In their study, the effects of E, G and GEI account for 81, 7.3 and 11.7% of the total treatments variation respectively. According to Gauch and Zobel (Citation1997), in standard METs, environment effect acts 80% of the total sum of treatments and 10% effect of genotype and interaction. The large first PCA score indicates the adequacy of AMMI model in interpreting the interactions. The variety 2004-SYNEEW had the least IPCA scores which is an indication that it is most stable among the three varieties. Across the environments, E5 with the least IPCA score was also a good tester environment.
Further analysis using GGEbiplot revealed that 2004-SYNEEW was the most stable variety and produced yield of about 1.8 t/ha even under low N application.
5. Conclusion
Choice of variety depends on the stability of the variety and high yield. The high cost of fertilizer have always been a problem to small scale farmers and farmers are willing to accept varieties with high yield under low to moderate fertilization. Based on the findings of this study, 2004-SYNEEW was a good choice because of its stability across the various fertilization levels. Application of low N can still be an alternative to small scale farmers with low resources because of the mean yield observed under low N. However, the yields under low N are not stable as such will vary under different edaphic environments and climatic conditions. AMMI model and GGEbiplot were in agreement in terms of identifying stable variety and ideal environments.
Additional information
Funding
Notes on contributors
A.S. Shaibu
A.S. Shaibu is a PhD scholar in Plant Breeding at the Federal University of Agriculture, Abeokuta, Ogun, Nigeria and lecturer at the Department of Agronomy, Bayero University, Kano, Nigeria. He has interest in stability analysis, biometry, molecular breeding and crop modelling.
A.A. Adnan
A.A. Adnan is a PhD Scholar at Katholieke Universatate de Leuven in the Faculty of BioScience Engineering and a lecturer at the Department of Agronomy, Bayero University, Kano, Nigeria. His research interest includes crop physiology and systems modelling.
J.M. Jibrin
J.M. Jibrin is a professor of soil fertility in the Department of Soil Science, Bayero University, Kano, Nigeria. His areas of research are soil fertility management, plant nutrition and systems modelling.
References
- Adekoya, M. A. (2008). Evaluation of variation, inter-character correlation and performance of okra, Abelmoschus esculentus L. Moench (M. Agric. Dissertation, 111 pp.). University of Agriculture, Abeokuta.
- Akande, S. R., & Balogun, M. O. (2009). Multi-locational evaluation of cowpea grain yield and other reproductive characters in the forest and southern Guinea Savanna agro-ecologies of Nigeria. Electronic Journal of Environmental, Agricultural and Food Chemistry, 8, 526–533.
- Annicchiarico, P. (1997). Joint regression vs AMMI analysis of genotype × environment interactions for cereals in Italy. Euphytica, 94, 53–62.10.1023/A:1002954824178
- Aremu, C. O. (2005). Diversity, selection and genotype × environment interaction in cowpea (Vigna unguniculata (L.) Walp) (PhD thesis, 125 pp.). University of Agriculture, Abeokuta.
- Ariyo, O. J. (1987). Multivariate analysis and the choice of parents for hybridization in okra (Abelmoschus esculentus (L.) Moench). Theoretical and Applied Genetics, 74, 361–363.10.1007/BF00274718
- Ariyo, O. J., & Ayo-Vaughan, M. A. (2000). Analysis of genotype × environment interaction in okra (Abelmoschus esculentus (L.) Moech). Journal of Genetics and Breeding, 54, 33–40.
- Badu-Apraku, B., Fontem, L. A., Akinwale, R. O., & Oyekunle, M. (2011). Biplot analysis of diallel crosses of early maturing tropical yellow maize inbreds in stress and nonstress environments. Crop Science, 51, 173–188.10.2135/cropsci2010.06.0366
- Badu-Apraku, B., Oyekunle, M., Fakorede, M. A. B., Vroh, I., Akinwale, R. O., & Aderounmu, M. (2013). Combining ability, heterotic patterns and genetic diversity of extra-early yellow inbreds under contrasting environments. Euphytica, 192, 413–433.
- Badu-Apraku, B., Fakorede, M. A. B., Oyekunle, M., Yallou, G. C., Obeng-Antwi, K., Haruna, A., ... Akinwale, R. O. (2015). Gains in grain yield of early maize cultivars developed during three breeding eras under multiple environments. Crop Science, 55, 527–539. doi:10.2135/cropsci2014.11.0783
- Becker, H. C., & Leon, J. (1988). Stability analysis in plant breeding. Plant Breeding, 101, 1–23.10.1111/pbr.1988.101.issue-1
- Crossa, J. (1990). Statistical analyses of multi-location trials. Advances in Agronomy, 44, 55–85.10.1016/S0065-2113(08)60818-4
- Crossa, J., Gauch, H. G., & Zobel, R. W. (1990). Additive main effects and multiplicative interaction analysis of two international maize cultivar trials. Crop Science, 30, 493–500.10.2135/cropsci1990.0011183X003000030003x
- Eberhart, S. A., & Russell, W. A. (1996). Stability parameters for comparing varieties. Crop Science, 6, 36–40.
- Farshadfar, E., Mohammadi, R., Aghaee, M., & Vaisi, Z. (2012). GGE biplot analysis of genotype × environment interaction in wheat–barley disomic addition lines. Australian Journal of Crop Science, 6, 1074–1079.
- Fikere, M., Tadesse, T., & Letta, T. (2008). Genotype-environment interactions and stability parameters for grain yield of faba beans (Vacia faba L.) genotypes grown in south eastern Ethiopia. International Journal of Sustainable Crop Production, 3, 80–87.
- Gabriel, K. R. (1971). The biplot graphic display of matrices with application to principal component analysis. Biometrika, 58, 453–467.10.1093/biomet/58.3.453
- Gauch, H. G. (1992). Statistical analysis of regional yield trials: AMMI analysis of factorial designs. Amsterdam: Elsevier.
- Gauch, H. G. (1993). MATMODEL version 2.0: AMMI and related analysis for two way data matrices (55 pp.). Ithaca, NY: Microcomputer power.
- Gauch, H. G., & Zobel, R. W. (1996). AMMI analysis of yield trials. In M. S. Kang & H. G. Gauch Jr. (Eds.), Genotype-by-environment interaction (pp. 85–122). Boca Raton, FL: CRC.10.1201/9781420049374
- Gauch, H. G., & Zobel, R. W. (1997). Identifying mega-environments and targeting genotypes. Crop Science, 37, 311–326.10.2135/cropsci1997.0011183X003700020002x
- IITA. (1982). Automated and semi-automated methods of soil and plant analysis manual (Series No. 7). Ibadan: Author.
- Kaya, Y., Akcura, M., & Taner, S. (2006). GGE-biplot analysis of multi- environment yield trials in bread wheat. Turkish Journal of Agriculture and Forestry, 30, 325–337.
- Lin, C. S., Binns, M. R., & Lefkovitch, L. P. (1986). Stability analysis; where do we stand? Crop Science, 26, 894–900.10.2135/cropsci1986.0011183X002600050012x
- Mandel, J. (1961). Non-additivity in two-way analysis of variance. Journal of the American Statistical Association, 56, 878–888.10.1080/01621459.1961.10482132
- Mohamed, N. E. M., Said, A. A., & Amein, K. A. (2013). Additive main effects and multiplicative interaction (AMMI) and GGE-biplot analysis of genotype × environment interactions for grain yield in bread wheat (Triticum aestivum L.). African Journal of Agricultural Research, 8, 5197–5203.
- Ntawuruhunga, P., Rubaihayo, P., Whyte, J. B. A., Dixon, A. G. O., & Osiru, D. S. O. (2001). Additive main effect and multiplicative interaction analysis for storage root yield of cassava genotypes evaluated in Uganda. African Crop Science Journal, 9, 591–598.
- Nwachukwu, J. E., Ikeorgu, G., & Asiedu, R. (2006). Multi-environment trial of newly developed white yam genotypes I: Analysis using genotype main effect and genotype-by- environment interaction (GGE biplot) model. Nigerian Journal of Genetics, 20, 49–53.
- Piepho, H. P. (1996). Analysis of genotype-environment interaction and phenotypic stability. In M. S. Kang & H. G. Gauch Jr. (Eds.), Genotype-by-environment interaction (pp. 151–174). Boca Raton, FL: CRC Press.10.1201/9781420049374
- Piepho, H. P. (1998). Methods for comparing the yield stability of cropping systems. Journal of Agronomy and Crop Science, 180, 193–213.10.1111/j.1439-037X.1998.tb00526.x
- Sanni, K. A. (2008). Genetic variation, seed dormancy and multi-locational performance of Nerica rice (Oryza sp. L.) (PhD Thesis, 158 pp.). University of Agriculture, Abeokuta.
- SAS Institute. (2010). Statistical Analysis Software (SAS). Users’ guide (60 pp.). Cary, NC: Author.
- Shaibu, A. S., Rabiu, I. U., & Adnan, A. A. (2015). Variability of root and physiological traits of different maturity groups of maize (Zea mays L.). Journal of Plant Breeding and Crop Science, 7, 233–239.
- Soil Survey Division Staff. (2002). Soil taxonomy (2nd ed.). Washington, DC: USDA-NRCS U.S. Govt. Print Office. Retrieved from http://soils.usda.gov/classification/taxonomy/main.html
- Westcott, B. (1986). Some methods of analysing genotype—environment interaction. Heredity, 56, 243–253.10.1038/hdy.1986.37
- Yan, W., & Kang, M. S. (2003). GGE biplot analysis: A graphical tool for breeders, geneticist and agronomists. Boca Raton, FL: CRC Press.
- Yan, W., Cornelius, P. L., Crossa, J., & Hunt, L. A. (2001). Two types of GGE biplots for analyzing multi-environment trial data. Crop Science, 41, 656–663.10.2135/cropsci2001.413656x
- Yan, W., Kang, M. S., Ma, B., Woods, S., & Cornelius, P. L. (2007). GGE biplot vs AMMI analysis of genotype-by-environment data. Crop Science, 47, 643–655.10.2135/cropsci2006.06.0374
- Zobel, R. W., Wright, M. T., & Gauch, H. G. (1988). Statistical analysis of a yield trial. Agronomy Journal, 80, 388–393.10.2134/agronj1988.00021962008000030002x