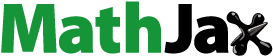
Abstract
Six generations (parents, first and second filial generations and two backcrosses) of two cross combinations of Maize (Zea mays L.) were studied for nature of gene action and correlated response for the five quantitative traits (viz., days to silking, days to maturity, ear height, cob weight per plant and grain yield per day). Significant role of dominance gene action was found for the inheritance of most of the traits. Additive gene action and epistatic interaction were also evident for the inheritance of different traits. A test of homogeneity of simple linear correlation coefficient from different generations revealed the existence of significantly different extent of association between the traits in different cross combinations. The significant correlations in parents were different from that of filial generations. Similarly, the expressivity of genes was found changed in segregating and non-segregating generations too for significantly associated traits. Based on the mode of inheritance and association of these traits, the choice of breeding procedures would be more effective for the improvement of parental material.
PUBLIC INTEREST STATEMENT
Maize with highest average productivity among cereals and wider adaptability is surpassingly also a cheap source of nutrition and used as staple food, livestock feed/forage, and industrial raw material in developing countries. Increased utilisation as poultry feed and its potential use as a forage and biofuel source makes it an important future cereal crop. Breeding for improved maize varieties requires a thorough understanding of the genetic mechanisms governing yield and yield components and association between traits. Our study attempted to see the inheritance pattern of decisive quantitative traits of maize thorough the study of generation means and the paired correlated response of traits in different generations of two cross combinations. The study unveiled the existence of different levels of correlated responses in the different generations of parental materials suggesting that during the breeding procedures the selection for and against any trait would require the detailed study of its correlated response.
Competing Interests
The authors declare no competing interests.
1. Introduction
In any breeding programme, selection criteria aimed at increasing the inherent yielding ability of a crop plant may be yield per se or one or more of the morphological components of yield. An understanding of the mode of inheritance of the yield components, the correlations among them and the association between each component and yield are necessary for an intelligent choice of breeding procedures for evolving high-yielding varieties (Khodambashi, Bitaraf, & Houshmand, Citation2012). The choice of an efficient breeding procedure depends on a large extent on knowledge of the genetic system controlling the character to be selected (Bitaraf, Khodambashi, & Houshmand, Citation2010; Azizi, Rezai, & Saeidi, Citation2006).
Quantitative characters in crop plants are often controlled by many genes; therefore, the inheritance of these traits mostly depends upon the gene action and epistatic interaction as well as environmental effects. Additive and dominance effects of gene action for a particular quantitative trait are most important to plant breeders as compared to epistasis since genes of additive and dominance nature can be exploited easily for a desired character in a certain breeding population (Nadarajan & Gunasekaran, Citation2005).
Generation mean analysis belongs to the quantitative biometric methods based on measurements of phenotypic performances of certain quantitative traits on as many as possible plant individuals in basic experimental breeding generations (parental, filial, backcross and segregating generations). As it was outlined by Kearsey and Pooni (Citation1998), generation mean analysis is a useful technique in plant breeding for estimating main gene effects (additive and dominance) and their digenic (additive x additive, additive x dominance and dominance x dominance) interactions responsible for the inheritance of quantitative traits. The estimation of genetic effects can help the plant breeder to decide the breeding procedures appropriate for the improvement of quantitative traits. The results of the estimates of the genetic components suggest for the selection of a particular breeding procedure for the improvement of the trait. In case additive component is high, reliance should be placed on synthetic breeding, whereas for the higher estimate of the dominance effect, the breeding objective should be towards development of hybrids for commercial purpose. For the high estimate of epistatic component, more reliance should be placed on selection between lines and families (Singh & Narayanan, Citation2013).
The association between traits is an important aspect to deal with in breeding programmes because genetic change due to selection for a given trait may change positively or negatively other traits (Vencovsky & Barriga, Citation1992). In addition, in most breeding programmes, the strategy is based on selection for several traits simultaneously and, therefore, knowledge about the genetic association between traits is inevitably useful for the establishment of selection criteria. A study on correlations between the traits in oil flax showed that the traits under study were in different extent of association in parents and progeny generations (Manner, Citation1958). The basic causes of genetic correlation are pleiotropy and linkage disequilibrium (Falconer, Citation1964; Hallauer & Miranda Filho, Citation1995). During the selection for a particular trait in the parent materials used, the performance and dependence of different generations devised from them could be of interest to plant breeder for effective selection of breeding procedures. Correlated responses are indeed a commonplace of selection experiments. Grain yield can be improved through indirect selection on the basis of yield components (Chandra, Islam, & Barman, Citation2004). Increase in one component might have a positive or negative effect on other components. This occurrence is a direct consequence of their interdependence during ontogenetic development of plants which is reflected through genetic correlations and compensation abilities (Grafius, Citation1978; Hamid & Grafius, Citation1978). Favourable combinations of yield contributing characters may improve yielding capacity. However, the direct selection for a quantitative trait may be associated with the indirect selection of the other traits, called correlated traits. Considering these facts, an attempt has been made in this experiment to see the inheritance pattern of five plant traits of maize through the study of generation means and the paired correlated response of these traits in different generations.
2. Materials and methods
Two cross combinations, viz., CM-500 X HKI-335 and BAUIM-2 X HKI-577 (abbreviated as C-I and C-II, respectively, in succeeding pages), were used to generate the six generations, namely, parents (P1 and P2), first and second filial generations (F1 and F2) and backcross generations (BC1 and BC2). CM-500, HKI-335, BAUIM-2 and HKI-577 are inbred lines which were sourced from the directorate of maize research (New Delhi), Antigua Gr.1 (Karnal), Birsa Agricultural University (Ranchi) and Karnal, respectively. These six generations of two crosses were raised and planted in a randomized block design (RBD) in three replications at maize research plot of Birsa Agricultural University, Ranchi, Jharkhand, India, during the autumn 2014. The experimental plot size comprised two rows for non-segregating P1, P2 and F1 generations, four rows each for BC1 and BC2 generations and six rows for F2 generation of each cross combinations. The rows were 4 m long with row spacing of 70 and 25 cm between plants accommodating 15–17 plants per row. Recommended package of practices was followed for fertilizers and other plant protection measures. A randomly selected 10 plants from each of the P1, P2 and F1, while 20 and 40 plants for each of the backcross generations and F2 generations, respectively, from each replication for both the cross combinations, were used to record data on individual plant basis for five plant characters, viz., days to silking (DS), days to maturity (DM), ear height (EH, cm), cob weight per plant (CWT, g) and grain yield per day (GY/day, g), calculated as grain yield per plant (g)/no. of days from silking to maturity, i.e. harvest day.
All the generation means were subjected to variance analysis (ANOVA) for RBD to see if the significant differences exist among the generations and significant traits were then subjected to individual scaling test (Mather & Jinks, Citation1982) and joint scaling test (Cavalli, Citation1952). A significant scale test would suggest for the presence of epistatic interaction and need of the six-parameter model of gene effect estimation. Gene effects were estimated based on the method proposed by Hayman (Citation1958) and Jinks and Jones (Citation1958). These analyses were done using the online statistical package of CCSHAU, Hariyana (OPSTAT, http://hau.ernet.in/about/opstat.php).
In the present investigation, a comparative study of correlations between the traits was done in six different generations from both the cross combinations. Correlation coefficients were calculated between all the traits by substituting corresponding variance and covariance in the formulae given by Gomez and Gomez (1983).
where r (1, 2) = correlation coefficient between the characters 1 and 2
Cov (1,2) = covariance between the characters 1 and 2
v (1) = variance of character 1
v (2) = variance of character 2
The simple linear correlation coefficient (r) was computed for all the generations (viz., both parents-P1, P2, F1, F2, BC1 and BC2) separately for all the traits. In addition, these generations were grouped as segregating and non-segregating in another set of correlation study. The homogeneity of correlation coefficients was tested to determine whether the degree of relationship between two variables remains the same under different genetic situations following Gomez and Gomez (Citation1983) using MS-EXCEL. Due to the change in the degree and sign of association between variables, it is difficult to choose the exact correlation value and its sign. A single and pooled value of “r” which should be used as selection criteria of significantly associated variables under changing genetic constitution was computed by the following steps:
Step-1—For each computed r value, z value was calculated using formula
, where ln = natural logarithm and r = simple linear correlation coefficient between two variables
Step-2—Computation of weighted means of the “z” value as:
where ni (i = 1,…, k) is the number of paired observations in the ith set of data in the computation of the ri value and ni is same for all genetic constitution. zw = weighted mean and zi = calculated z value.
(c) Step-3—Computed the chi-square value as
. The computed chi-square value was compared with tabulated one at (k-1) degrees of freedom. For significant chi-square value, the hypothesis of homogeneity was rejected.
(d) Set-4— In case chi-square test was not significant, the simple linear correlation coefficient over different genetic constitution was obtained from test of the corresponding value of r and Zw table or through the following formula
where rp = pooled linear correlation coefficient over the different genetic constitution and e = exponential.
3. Results and discussion
The six generations from both the crosses were found to have variability in their mean performance and the population was of differing variability, which is evident in Table . Analysis of variance revealed that generations were significantly differing for all the five traits in both crosses, except for DS in C-II (Table ). In subsequent analyses, scaling test for these significant traits was performed using software, OPSTAT, and found to be significant for any of the four scales (viz., A, B, C and D) or more and/or chi-square test for joint scaling test (Table ). The gene effects (additive and dominance) and epistatic interactions (additive X additive, additive X dominance and dominance X dominance) were estimated in three- and five-parameter model for non-significant scale test and significant scale test, respectively (Table ). The only trait, DM in C-II, was found to be non-significant scale test, and a three-parameter model of additive dominance was adequate to describe the total variance. The dominance action (h) was found to be significantly higher in magnitude than any other gene effects for all the traits in both the crosses except for CWT and GY/day in C-II. For these traits, dominance action was playing a major role in inheritance. EH, CWT and GY/day in C-I and DM in C-II were also having significant estimates of additive gene action (d) too. Estimates of slight additive effects are possible due to high degree of dispersion of increasing alleles between parents (Mather & Jinks, Citation1982). The results of the important role of non-additive gene action for flowering and maturity traits were in accordance with the findings of Shahrokhi, Khorasani, and Ebrahimi (Citation2013), Abadi, Khorasani, Sar, Movafeg, and Golbashi (Citation2011) and Sher, Iqbal, Khan, Yasir, and Rahman (Citation2012). The differential extent of significant non-allelic interactions was also found to be important in describing the inheritance pattern in different traits. CWT and GY/day in C-II were found to be inherited through the major role of additive X additive (i) and dominance X dominance (l) interaction. The results of CWT and GY/day for the significantly important role of interaction effects in inheritance were in concurrence to the results of Sujiprihati, Saleh, and Ali (Citation2003) and El-Badawy and El. (Citation2012) in maize and Golparvar (Citation2012) in wheat for per day productivity, respectively. DS in C-I and EH in C-II were found to have duplicate and complementary gene interaction, respectively, since the dominance (h) and dominance X dominance (l) effects were significant with opposite signs in DS and same sign in EH (Mather & Jinks, Citation1982). The frequent appearance of epistasis is mostly indicative of greater genetic diversity between the parents. The results of the estimates of the genetic components suggest for the selection of a particular breeding procedure for the improvement of the trait. Selection procedures are influenced by several genetic mechanisms. In cross-pollinated crops like maize, selection for individuals having the extreme phenotype for a trait or a group of traits selected for favours alleles showing dominance in the appropriate directions, and genes showing “desirable” epistatic interactions will also be selected for. Selection for the intermediate phenotype and against the extreme phenotypes, accumulates alleles showing additive gene action (Hayes, Immer, & Smith, Citation1955).
Table 1. Generation-wise mean, variance, variance of mean and coefficient of variation (CV) for five traits in two cross combinations
Table 2. ANOVA (MSS) for five traits of six generation means in two crosses
Table 3. Individual scale and chi-square (Xp2) test for five traits in two cross combinations
Table 4. Gene effects for five traits in two cross combinations
The correlation coefficient matrices for five traits under study in six generations of both cross combinations showed a greater extent of differences in the magnitude and direction (Table ). CWT and GY/day were found positively correlated with the highest magnitude of “r” in all the matrices (making the higher estimate of coefficient of determination “r2“), suggesting that the parental materials and their devised generations were significantly differing for these traits and these traits were capable in defining the large portion of variability present between them. DS and DM were found negatively correlated with the CWT and GY/day separately in parental generations and their respective backcrosses only. However, EH was found to be positively correlated with both the traits. Several researchers have also found the variability in the extent and direction of correlated response between different quantitative traits in different plant materials. Saidaiah, Satyanarayana, and Kumar (Citation2008) in 40 F1 generation of maize showed that correlation coefficient of grain yield with plant height, EH and 100-seed weight was positive and significant. The positive significant correlation of seed yield was found with ear weight, 100-seed weight, plant height and number of leaves per plant in maize inbreds (Eleweanya, Uguru, Ene-Obong, & Okocha, Citation2005). As a general rule, the significant correlations (r) were found to be decreased in F2 generation compared to F1 in both the crosses. The decreased “r” value may be due to the increased variability within the F2 population through inbreeding of F1. For almost all the generations, the correlation coefficients between CWT and GY/day were high (>0.9) in C-II. The reason behind may be that both the traits were found to have the same type of epistatic gene interaction as the only components of genetic variability present and total variability could be described mostly through these traits.
Table 5. Generation-wise correlation coefficients (r) and test of homogeneity for five traits in both crosses
When the generations were grouped as segregating and non-segregating, more number of paired relationships were found significant, and the relationships between the traits were differing in their magnitude, but the directions were not altered (Table ). The decreased magnitude of “r” in segregating generations compared to non-segregating may be attributed due to the increased variability within the segregating generations. Such a decrease makes the decreased “r2“ estimate, suggesting that the pair of traits would not be sufficient in describing the existing variability in the population. The change in existing variability in segregating generations would have been occurred due to the breakage of several genic relationships like linkage and non-allelic interactions by the action of segregation and recombination. In the perusal of correlation coefficient matrices of parents (P1 & P2 combined together; physical mixture of genotypes) and F1 generation (genetic mixture of genotypes), the paired relationships varied in their extent and significance level (Tables and 5). When genes from different sources (parents) came together, they behaved differently, and it may be due to the differential arrangements of gene sequences or the change in gene expression of the particular trait when the genes came together in the F1 generation. The different arrangements of genes together in F1 would appear that the action of structural genes mediating the production of different enzymes may be switched on or off (Cavalli, Citation1952).
Table 6. Group-wise correlation coefficients (r) and test of homogeneity for five traits in both crosses
As evident in this study, the extent and direction of the correlation coefficient between the traits are liable to vary in different genetic constitution. So, to see whether the degree of association relationships of the traits changes significantly or not with the change in the gene frequencies and gene sequences in the different generations, the test of homogeneity of correlation coefficient was attempted. The non-significant chi-square test in test of homogeneity of correlations among the six generations for all the paired relationships for both crosses except CWT and GY/day in C-II may be ascribed to the significant role of pleiotropic action of polygenes for not changing the correlation coefficient (being homogeneous) through the generations (Table ). The pleiotropic actions are not liable to change through the generations. In C-II, the only relationship between CWT and GY/day was found to be heterogeneous for different generations, and it suggests that the selection for a trait cannot be relied on the single correlation value for different generations. A negative correlation between traits would arise whenever two biosynthetic chains utilize a common precursor and arise indeed from competition for this precursor. Equally, a positive correlation may be produced due to changes in the action of genes whose chief effect is on the supply of precursor (Cavalli, Citation1952). When partitioning the generations into segregating and non-segregating, the extent of the correlation coefficient was found varied in both crosses and so some traits were found significant chi-square test (Table ). The variation in phenotypic expression of paired traits in segregating generations might be due to immediate segregation; the linkage relations of the genes may also have their effect on the correlation. The linkage has no effect on the means of non-segregating families. For those paired relations having non-significant chi-square test, the pooled value of correlation coefficients throughout the generations was calculated (Table , 6 and 6), and these values could be used as a selection criterion in any generation of the respective parental materials. Similarly, the homogeneity test for parents (P1+ P2) versus F1 also revealed the existence of both homogeneous and heterogeneous correlation coefficients for different paired relations in both the crosses (Table ). For the heterogeneous paired relations, the selection of a parent for a particular trait could not be done efficiently for hybridization programme unless its response in F1 is not known.
4. Conclusion
Different maize plant quantitative traits showed differential way of their inheritance pattern in different parental materials. Based on the type of gene action and interaction present, the improvement of maize can be done through the selection of breeding procedures for the particular trait of interest. Further, the expressivity of genes behaves differently at different genetic levels; the gene expression based on gene frequency and their arrangement changes in generations. The existence of different types of gene action and interactions at the different extent of their magnitudes in different crossing materials may have been also contributing to the phenotypic expression of the correlated traits at changing genetic constitution. The existence of different levels of correlated responses in the different generations (i.e. heterogeneity in correlation coefficient) suggested that during the breeding procedure, the selection for and against any trait would require the detailed study of the correlated response of the parents in different generations of cross-pollinating crops like maize.
Conflicts of Interest
The authors declare that there are no conflicts of interest in relation to the publication of this research paper.
Correction
This article has been republished with minor changes. These changes do not impact the academic content of the article.
Acknowledgements
This study was a part of the M.Sc. thesis research of the first author. The first author highly acknowledges the Directorate of Agricultural Research and Education (DARE), Ministry of Agriculture, Government of India and Government of Nepal, for providing opportunity to pursue the M.Sc. (Ag.) programme in Department of Plant Breeding and Genetics, Birsa Agricultural University (BAU), Kanke, Ranchi, under the Nepal Aid Fund Scholarship Scheme-2013. The authors also acknowledge the ICAR and the BAU for providing research platform and materials.
Additional information
Funding
Notes on contributors
Madhav Pandit
The authors are researchers who seek to develop a high-yielding, stable and farmer-preferred varieties of crops of future such as maize, millets, legumes and fodder and pasture crops through conventional, molecular and participatory plant breeding approaches. The research group emphasizes on genetic diversity assessment and evaluation of future smart food crops and livestock crops directed to the genetic improvement of crops, especially for yield, nutritional quality as well as biotic and abiotic stress breeding towards climate change adaptation and resilience of climate change. Manigopa Chakraborty is a chief scientist cum university professor who designed and conceptualized the experiment and has vast experience of various participatory and molecular plant breeding approaches; working together for improvement of agricultural production and productivity; and conducts farmer’s participatory research that helps the improvement of communities’ livelihood and technology.
References
- Abadi, J. M., Khorasani, S. K., Sar, B. S., Movafeg, S., & Golbashi, M. (2011). Estimation of combining ability and gene effects in forage maize (Zea mays L.) using line x tester crosses. Journal of Plant Physiology and Breeding, 1(1), 63–73.
- Azizi, F., Rezai, A. M., & Saeidi, G. (2006). Generation mean analysis to estimate genetic parameters for different traits in two crosses of corn inbred lines at three planting densities. Journal of Agricultural Science and Technology, 8, 153–15.
- Bitaraf, N., Khodambashi, M., & Houshmand, S. (2010). Genetic analysis, correlation and path analysis for seed yield and its components in lentil (Lens culinaris Medik.). Journal of Lentil Research, 4, 20–25.
- Cavalli, L. L. (1952). An analysis of linkage in quantitative inheritance. Waddington, London: HM Stationary Office.
- Chandra, D., Islam, M. A., & Barman, C. D. (2004). Variability and interrelationship of nine quantitative characters in F5 bulks of five wheat crosses. Pakistan Journal of Biological Sciences, 7(6), 1040–1045. doi:10.3923/pjbs.2004.1040.1045
- El-Badawy, M., & El., M. (2012). Estimation of genetic parameters in three maize crosses for yield and its attributes. Asian Journal of Crop Science, 4(1), 127–138. doi:10.3923/ajcs.2012.127.138
- Eleweanya, N. P., Uguru, M. I., Ene-Obong, E. E., & Okocha, P. I. (2005). Correlation and path coefficient analysis of grain yield related characters in maize (Zea mays L.) under umudike conditions of South Eastern Nigeria. Agro-Science, 4(1), 24–28.
- Falconer, D. S. (1964). Introduction to quantitative genetics. New York: Ronald Press.
- Golparvar, A. R. (2012). Heritability and mode of gene action determination for grain filling rate and relative water content in hexaploid wheat. Genetika, 44(1), 25–32. doi:10.2298/GENSR1201025G
- Gomez, K. A., & Gomez, A. A. (1983). Statistical procedures for agricultural research. New Delhi, India: Wiley India (P) Ltd.
- Grafius, J. E. (1978). Multiple characters and correlated response. Crop Science, 18(6), 931–934. doi:10.2135/cropsci1978.0011183X001800060004x
- Hallauer, A. R., & Miranda Filho, J. B. (1995). Quantitative genetics is maize breeding(pp. 468). Ames: Iowa State University Press.
- Hamid, Z. A., & Grafius, J. E. (1978). Developmental allometry and its implication to grain yield in barley. Crop Science, 18(1), 83–86. doi:10.2135/cropsci1978.0011183X001800010022x
- Hayes, H. K., Immer, F. R., & Smith, D. C. (1955). Methods of plant breeding. New York: Mc Graw Hill Book Company.
- Hayman, B. I. (1958). The separation of epistatic from additive and dominance variation in generation means. Heredity, 12(3), 371–390. doi:10.1038/hdy.1958.36
- Jinks, J. L., & Jones, R. M. (1958). Estimation of the components of heterosis. Genetics, 43(2), 223–234.
- Kearsey, M. J., & Pooni, H. S. (1998). The genetical analysis of quantitative traits. Stanley London: Chapman and Hall.
- Khodambashi, M., Bitaraf, N., & Houshmand, S. (2012). Generation mean analysis for grain yield and its related traits in lentil. Journal of Agricultural Science and Technology, 14, 609–616.
- Manner, R. (1958). Correlation studies between the parents and the progeny generations in oil flax. Hereditas, 44(1), 37–64. doi:10.1111/j.1601-5223.1958.tb03471.x
- Mather, K., & Jinks, J. L. (1982). Biometrical Genetics. London: Chapman and Hall Ltd.
- Nadarajan, N., & Gunasekaran, M. (2005). Quantitative genetics and biometrical techniques in plant breeding. New Delhi, India: Kalyani Publishers.
- Saidaiah, P., Satyanarayana, E., & Kumar, S. S. (2008). Association and path coefficient analysis in maize (Zea mays L.). Agricultural Science Digest, 28(2), 79–83.
- Shahrokhi, M., Khorasani, S. K., & Ebrahimi, A. (2013). Study of genetic components in various maize (Zea mays L.) traits, using generation mean analysis method. International Journal of Agronomy and Plant Production, 4(3), 405–412.
- Sher, H., Iqbal, M., Khan, K., Yasir, M., & Rahman, H. (2012). Genetic analysis of maturity and flowering characteristics in maize (Zea mays L.). Asian Pacific Journal of Tropical Biomedicine, 2(8), 621–626. doi:10.1016/S2221-1691(12)60108-7
- Singh, P., & Narayanan, S. S. (2013). Biometrical techniques in plant breeding. New Delhi, India: Kalyani Publishers.
- Sujiprihati, S., Saleh, G. B., & Ali, E. S. (2003). Heritability, performance and correlation studies on single cross hybrids of tropical maize. Asian Journal of Plant Science, 2(1), 51–57. doi:10.3923/ajps.2003.51.57
- Vencovsky, R., & Barriga, P. (1992). Genetica biometrica no fito melhoramento. Ribeirao Preto. Sociedade Brasileira de Genética.