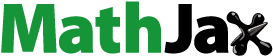
Abstract
Despite dryland areas are diverse in agro-ecology; technological recommendations are summative and based on researchers’ on-station genetic traits, which deprived the farmers’ preference, the economic and technical efficiencies. Participatory sorghum technologies evaluation was thus initiated to compare dryland sorghum technologies (Misikir and Girana-1) against the local practice in a wider scale, comprising 450 farmers from marginal districts of Wag-lasta. The agronomic, economic and preference data were collected and analysed in descriptive statistic, ANOVA, partial budgeting and weighted ranking matrix. The combined result indicated that Misikir, Girana-1 and the local sorghum provided mean grain yields of 2.9, 1.6 and 1.5 ton ha−1, respectively. Accordingly, Misikir technology has 81.3% and 93.3% yield advantage over Girana-1 and the local, respectively. The marginal rate of return (MRR) of Misikir is 477.6% but insignificant for Girana-1 by the cost higher than the local practice. The weighted ranking matrix also shows that Misikir was preferred by its earliness, seed setting performance, grain and biomass yields. Dissemination of Misikir technology is thus safely recommended. The finding further revealed that technological recommendations using on-station plot trials is dwarfing the adoption rates since farmers would hesitate to uptake as if they did not evaluate the technologies on their local context. The paper concludes that scale wide farmers’ participation is vital in future experiments for sustainable and demand-driven technology development and diffusion on top of providing feedback to the concerned agricultural scientists.
PUBLIC INTEREST STATEMENT
In dryland areas, the technological recommendations are provided for wider production domains using simply plot level on-station genetic traits, which deprive farmers’ preference, the economic and technical efficiencies. This in turn dwarves technological adoption in the marginal dryland zones worldwide. These areas contain more than seven agro-ecologies thus entails diverse production potential for different sorghum technologies. Therefore, implementing a participatory approach to evaluate technologies best suiting for the local context is an important opportunity for farmers to enhance demand-driven technology adoption, which ultimately increases production and productivity on top of providing feedback to the agricultural scientists. Besides, scale wide farmers’ participation in on-farm experiments is vital to sustainable technological development and diffusion as it approximates the real farm circumstances.
Competing Interests
The authors declare no competing interests.
1. Introduction
Sorghum (Sorghum bicolar) grows over a wide range of latitudes from 0° to 45° North and South of the equator (International Crop Research Institute for the Semi- arid Tropics [ICRISAT], Citation1991). Because of its drought resistance, sorghum is the crop of far excellence for dry regions and areas with unreliable rainfall. It is one of the important indigenous food crops and is only second to tef among cereals in the dryland areas of Ethiopia (Geremew et al., Citation2004). As sorghum is growing under a wide range of environmental conditions, the range of both biotic and abiotic production constraints are diverse, resulting in very poor performance under farmers’ circumstances (Asmiro, Ademe, Lijalem, & Tsega, Citation2016). The average national yield of 2.4 ton ha−1 is by far very low compared to 3–6 ton ha−1 achieved using improved sorghum technologies (Central Statistical Agency [CSA], Citation2015). In northeast Ethiopian dryland zones, where sorghum is the major food crop, its average productivity ranges from 1.5 ton ha−1 up to zero in sever moisture deficit seasons, which is by far lower than the national average (Central Statistical Agency [CSA, Citation2017). Because of the low amount, uneven distribution and erratic nature of the rainfall on top of fewer improved technologies fitting to diverse growing conditions and lower utilization rates, crop production is seriously affected in these areas (Ademe, Asmiro, Lijalem, & Tsega, Citation2018).
The major constraints of farmers in using improved technologies are weak linkages between farmers and research as well as limited use of extension and research results. One can still witness the persistence of subsistence agriculture with an ever more dynamic and competitive environment. This entails the risk of the existence of wider gaps between the performance of research and farm averages (Amede, Assefa, & Stroud, Citation2004). With the aim of addressing these problems, several dryland sorghum technologies were developed and released nationally having special merits. Sekota Dry Land Agricultural Research Centre has been undertaking adaptation trials on these technologies in Wag-lasta marginal areas and released two improved varieties (Misikir and Girana-1) with their production package for wider production domain (Asmiro et al., Citation2016). However, the trial was on-station at plot level and the evaluation was designed and managed by researchers merely. The recommendation was provided purely on the bases of biological standpoints, devoid of farmers’ involvement, economic efficiency, societal acceptability, and technological applicability. These factors are of course, the building blocks for wider technology diffusion in general and demand-driven knowledge tracking in particular.
Over 70% of new technologies developed for marginal dryland areas have failed to take root among farmers and remain confined to research stations. Further intensification of extension services has not also shown great promise in improving the situation (Sanghi, Citation1987). An increasing number of scientists, therefore, have recognized that there is a need to modify research methodologies in order to make it more sensitive to local conditions (Ashby, Citation1986). In recent years, agricultural researchers started working with low-income farmers in hard environmental conditions. These environments tend to be highly fragile, characterized by combinations of low and unreliable rainfall, poor and easily degradable soils, hilly topographies, lack of economic and social infrastructures (Kojo, Citation1956). As a result, technologies generated on research stations have not performed well under farm conditions and have not been widely adopted (Matlon, Citation1984).
According to Ogwal-Kasimiro, Wakulira, Kiyini, Mwebaze, and Yiga (Citation2012), to circumvent these problems and to achieve better results, responsive adaptive research trials should be established with actively participating farmers in the technology development process.
Moisture stressed areas in Ethiopia are grouped into seven agro-ecologies and covers 66% of the total area of the country entails diverse potential to different sorghum technologies (Geremew et al., Citation2004). Efforts to overcome these problems have led to the development of methods of collaborating with farmers to understand local-level farm conditions and strategies, and the processes through which small-scale cultivators adopt and adapt new agricultural technologies (Fernandez, Citation1988). This collaboration is termed as participatory technology development and its aim is to minimize the researchers’ bias and maximize the input of the farmer, based on their concerns (Sollows, Citation2012).
It is also argued that large scale on-farm trials should be simple and flexible. They should be farmer managed to approximate farm conditions. Researchers should concentrate on monitoring and measuring the experiments and refrain from imposing controls on the trials (Siriwardena, Citation1988). With simple trial design, a large number of farmers can participate and inter-farm variations be noted. These activities need to be synchronized with the activities of the farmer and not developed for the convenience of the researcher. The most important aspect of on-farm research is seen as building the relationship with the farmer (Kleene, Citation1984).
This study is, therefore, designed to provide farmers with a menu of sorghum technologies then to select and demonstrate economically feasible, technically applicable and socially acceptable technologies in a participatory approach in the marginal drylands of Wag-lasta, Ethiopia.
Therefore, findings from this participatory evaluation would provide information to the universal dryland areas about the importance of considering farmers’ participation, wider coverages as well as the economic and technical efficiency traits in technology development. Because these traits are the building blocks for farmers in identifying their favourite technologies to enhance demand-driven technology adoption, ultimately increases production and productivity.
2. Materials and methods
2.1. Description of the study area
The study was conducted at three districts (viz., Ziquala, Abergele and Lalibela) in Northeastern marginal drylands of Ethiopia for three consecutive production years (2015/16–2018/19). More specifically, Ziquala district is geographically located at 12°48ʹN and 38°47ʹE latitude and longitude, respectively (Asmiro et al., Citation2016). The district has an altitude of 1462 masl. Its annual average rainfall and temperature are 255 mm and 22°C, respectively (Dereje, Citation2004). Abergelle district is also located at 13°20ʹN and 38°58ʹE latitude and longitude, respectively. Its altitude ranges from 1150 to 2500 masl, with the annual mean temperature and rainfall of 23-43°C and 250–750 mm, displaying semi-arid nature of the district (Ademe et al., Citation2018). Lalibela district is located at 12°55ʹ559ʹ’N latitude and 38°42ʹ293ʹ’E longitude at an altitude of 2400 masl having mean annual temperature and rainfall of 26.2°C and 895.2 mm, respectively (Woreda Office of Agricultural Development [WoA, Citation2015).
The districts’ rainfall is unimodal, short and erratic that extends not more than 2 months per year, usually from the end of June to the end of August. Hence, the districts’ crop production usually fails due to low soil fertility and high moisture stress, almost every year (Ademe et al., Citation2018).
2.2. Sampling, experimental design and farmers’ participation
Two-stage sampling technique was employed to select the participant farmers. In the first stage, three districts were purposively picked to denote sorghum production areas in marginal drylands of northeastern Ethiopia. In the second stage, 50 farmers who had 0.25 ha clustered farmland on average were randomly selected from each district to host the experiment. In combination, 450 (75 female) farmers in the 3 years were involved for the scale wide participatory on-farm evaluation. Host farmers in each district were organized into farmers’ research and extension groups (FREGs) in order to ease the participatory evaluation. The group members were selected with the consultation of local agricultural experts and key informants, conversant to the areas to represent different social segments (to have diverse spectrum of age, sex and wealth status). Each group had a chairman and secretary to facilitate FREG tasks in collaboration with researchers and extension workers. A timely action plan and meeting schedule were set out by the group members to evaluate the technologies following the main physiological growth stages. Before launching the experiment, researchers organized operational platform to create awareness and to identify responsible stakeholders in the experiment. Then, memorandum of understanding (MoU) was signed between stakeholders to part duties in the whole trial course. Farmers thus provided training on basic agronomic practices and technology packages for 2 days comprising the theoretical and practical components. Training provision, technical backstopping and data collection were managed by the researchers while farmers undertook the experiment ().
The experiment was conducted using two improved sorghum technologiesFootnote1 (Girana-1 and Misikir) and adjacent to them local cultivar under traditional production practice for comparison. The treatments were laid on three un-replicated simple block considering farmers as replications. The improved technologies were planted in a row at 10 kg ha−1 seed rate along with 100 and 50 kg ha−1 diammonium phosphate (DAP) and Urea, respectively. All the required management practices were done as per the recommendation. The study was carried out for three consecutive years in order to minimize the risk of seasonal variation as well as to increase farmers’ confidence in the provided technologies. Finally, field days were held involving concerned stakeholders to evaluate and endorse the performances of different technologies to the wider community.
2.3. Data collection and analysis
Both quantitative and qualitative data were collected from farmers using a checklist as well as focus group discussions. The quantitative data (days to maturity, grain and biomass yield) were collected at the plot level. The qualitative data (socioeconomic parameters: profitability and acceptability) were also collected. On the other hand, secondary data were collected from different published and unpublished (working reports from districts) sources to triangulate and support the quantitative results. The collected data were analysed using descriptive statistics such as mean, frequency and percentage points. Besides, different methods as suggested by Yadav, Kamboj, and Garg (Citation2004) were used to analyse the technological gaps, extension gaps and the technological index among treatments using the following formulas:
Data from the treatments (Misikir, Girana-1 and Local) were subjected twice to the analysis of variance (ANOVA) followed by Tukey’s honestly significant difference (HSD) test (SPSS, Citation2007). The ANOVA table is constructed to illustrate the effects of treatments and other factors like experimental errors on the parameter values under consideration. The post hoc analysis (Tukey-HSD) carried out to compare the means of every pair of treatments in the study districts (i.e., identifying which technology has significantly larger mean as compared to other technology). The first of which was depending on agronomic records as explanatory variables and the second was depending on the indicative scores as explanatory variables. The coefficient of determination (R2) and the Tukey’s test (HSD) have been applied to significant variables in both analyses. The data of the indicative scores of sites for the three agronomic records were standardized and the sample variance (S2) was calculated by the following formula:
where S2 is sample variance, Σ is sum, xi is the term in data set (indicative scores of sampling sites), x is sample mean, and n is sample size.
The results of ANOVA (R2, F, P) and the sample variance (S2) have been taken to express the impact of the agronomic records and their order of importance, on the different treatments of the trial area (Alaa & Mahgoub, Citation2019).
Economic data (production costs and benefits) were collected to compare the cost-effectiveness of treatments. The costs of the whole package components such as improved seed, fertilizer and management practices were collected for each district in Ethiopian Birr (ETB). Yield obtained from each treatment was adjusted by 10%, and also the selling price of grain and biomass yield at the farm gate was taken. The average labour cost for improved practice (row planting and weeding) was expressed in person day, where one person day was assumed to be 8 hours of work. Finally, the economic advantage (efficiency) of each technology was calculated in the marginal rate of return (MRR) using the following formula:
where MRR = marginal rate of return, ∆NB = change in net benefits and ∆TVC = change in total variable input costs (CIMMYT, Citation1998).
To make the partial budget more useful, administering sensitivity analysis was worthwhile. Therefore, it was managed by computing the worst, most likely, and best-case scenarios of the cost-benefit sides of treatments. The worst, most likely and best case figures can be computed using a general error factor rate of 10%, or by adjusting the item that most likely to fluctuate. This is because farmers deal with market uncertainties, or not knowing weather the prices will increase or decrease by tomorrow. The sensitivity analysis hence will relax farmers’ forced decision-making built on the imperfect market information. Thus, combining partial budget and sensitivity analysis was robust enough to handle the efficiency questions of farmers on the technology package (Ademe et al., Citation2018).
Group discussion with FREG members, field visit and field days were used to evaluate the technologies. Farmers’ reaction to each technology was asked using focus group discussions (FGDs) by assigning literate farmers in each group to lead the evaluation since most participant farmers were unable to read and write. Host farmers, therefore, brainstormed to identify their main evaluation criteria to be considered in selecting best sorghum technology under the local context. Five preference parameters (viz., grain yield, earliness, seed setting performance, stalk yield and marketability in descending order) were identified and weighted on the bases of their significance. Farmers were ranking the accredited preference criteria pair-wisely and then considered the rank as weight. The scores given by farmers to each variety were multiplied by the respective weight. Products were aggregated for each variety for final selection (1, 2, 3; 1 = the best) (Russell, Citation1997).
Moreover, Spearman’s rank correlation was used to see the degree of coincidence between farmers’ preference rank with the actual value of measured attributes (Ferdous, Datta, Anal, Anwar, & Khan, Citation2016). The correlation coefficient is defined as:
where d = difference in the ranks assigned to the same phenomenon and n = number of phenomena ranked.
Finally, extension activities like diagnostic field visits and field days were undertaken to create awareness about the technology in general and the variety in particular, which can benefit the farmers in the long run (Feder, Citation2002).
3. Results and discussion
3.1. Yield and yield component performance
The local sorghum variety under farmers’ customary practice gave a mean grain yield of 1.5 ton ha−1. On the other hand, the improved sorghum technologies, Misikir and Girana-1 provided mean grain yields of 2.9 and 1.6 ton ha−1, respectively (Table ). The result thus revealed that, Misikir and Girana-1 improved sorghum technologies have an overall yield advantage of 93.3% and 6.6% respectively, over farmers’ variety under the existing practice in all sites. Sorghum productivity problem in dryland area thus could be overwhelmed by adopting efficient package practices on top of using improved varieties. Likewise, the higher technological index in Misikir technology provided evidence that necessitates a wider scope of further improvements in sorghum production (Yadav et al., Citation2004). The ANOVA result in Table , shows that statistically significant difference between treatments in grain yield, stalk yield and days to maturity across locations (p < 5%). Moreover, Tukey–HSD test in , indicates that among treatments, Misikir was best performing technology in grain yield, stalk yield and days to maturity (p < 10%).
Table 1. Duties and responsibilities of main stakeholders in the scale wide evaluation of sorghum technologies
Table 2. Yield performance, technology gap and index of different sorghum technologies
Table 3. ANOVA result on the differences in grain yield, stalk yield and maturity days
3.2. Partial budget analysis
Expenditures which were similar to treatments were not taken and analysed (citrus paribus), hence given the prevailing farm gate prices, the benefit-cost ratio was computed for grain and stalk yield on a hectare basis. The total variable cost of farmers’ practice was lower than that of improved technology. The use of improved production package for Misikir technology thus provided a higher net benefit of ETB 20 802.8 ha−1, followed by the farmers’ practice with the net benefit of ETB 12 292.4 ha−1 (Table ). The marginal rate of return (MRR) of Misikir technology was thus 477.6%. This implies that for every ETB 1.00 invested in improved technology (changing from local practice to Misikir technology), farmers can expect to recover the ETB 1.00 and obtain an additional ETB 4.78.
The economic return of Girana-1 technology is not promising thus it is rejected from further analysis due to lower net benefit than Misikir and local practice at similar and higher total variable cost, respectively. The process of rejecting dominated treatments from the further analysis is called dominance analysis (Ademe et al., Citation2018).
The sensitivity analysis is a change in the net benefit and the return on marginal capital as revenue and input prices vary by 10% above and/or below their values. Thus, a 10% change in the revenue of Misikir sorghum technology influenced the net benefit by 24.2%. Whereas, a 10% change in the total input price influenced its net benefit by less than 10%. The return of marginal capital always beats 100%, which indicates an investment in Misikir sorghum technology will be gainful. Generally, the sensitivity analysis result showed that if the price of output becomes constant and the price of inputs increase by 1023.8%, the technology has a positive return.
3.3. Farmers’ preference for different sorghum technologies
Due to their homogeneous sociocultural entities, farmers across location identified five preference parameters in common to select their best sorghum technology. The comparison result of the weighted ranking matrix thus revealed that a technology which has lower aggregated product was peaked as a primary choice. As a result, farmers preferred Misikir, local and Girana-1 technology treatments as the best, fair and worst, respectively, in all parameters (Table ).
Table 4. Tukey–HSD test to identify best performing technology in grain yield, biomass yield and days to maturity
Table 5. Partial budget and sensitivity analysis of different treatments
Table 6. Summary of farmers’ preference ranking among sorghum technologies
Spearman’s rank correlation coefficient was calculated to see the degree of coincidence between farmers’ preference rank and the actual value of measured agronomic attributes. Therefore, the degree of coincidence between farmers’ preference rank and actual values rank for grain yield, biomass yield and earliness attributes were 100%, 100% and 100% respectively (Table ). At the end of the trial, field days were organized involving model farmers, development agents, farmers from the trial areas, experts and administrative officials. A total of 750 (200 females) participants visited the trial in different sites and applaud Misikir technology for its grain and stalk yield as well as earliness traits than the rest of treatments.
Table 7. Correlation of farmers’ preference rank and the actual measured traits rank
4. Conclusion
The study was basically focused on the participatory evaluation of sorghum technologies on a wider scale to create demand-driven technology diffusion in the north-eastern drylands of Ethiopia. Two improved sorghum technologies (Misikir and Girana-1) along with the local sorghum variety under farmers’ practice were evaluated at 450 farmers’ field. The result thus revealed that the mean grain yield of Misikir sorghum technology significantly out-yielded Girana-1 sorghum technology as well as the local variety under farmers’ practice. The Tukey–HSD test also indicated that among treatments, Misikir was best performing technology in grain yield, biomass yield and days to maturity in all locations. The MRR result similarly indicated that among treatments, investing in Girana-1 improved sorghum technology was not promising due to its lowest net benefit at the higher and even equal variable input costs. The farmers’ preference ranking matrix also shows that Misikir, the local and Girana-1 technologies were preferred as first, second and third choices, respectively, by the overall preference parameters. The agronomic (ANOVA), economic (partial budget) and the weighted ranking matrix result thus indicated that farmers have preferred and perceived the higher yield potential and profitability of Misikir technology. As a result, Misikir improved sorghum technology is recommended for further dissemination in the marginal drylands of Wag-lasta. This finding tells that recommendations based on researchers’ plot level on-station trial, deprived of economic profitability evaluation as well as limited or even zero farmers’ participation leads to meagre technological adaptation and/or complete rejection. Therefore, suppliers (i.e. researchers) should deliver a basket of choice of improved varieties developed through active and scale wide farmers’ involvement for the needy to pick one, two, or all depending on their context (). Backup studies should take the farmers’ preference parameters and the feedback into account for future variety and/or technology development activities.
Acknowledgements
The farmers’ and extension personnel’s unreserved cooperation is greatly acknowledged.
Additional information
Funding
Notes on contributors
Ademe Mihiretu
Ademe Mihiretu is a citizen of Ethiopia. He is a full time researcher in Socioeconomic and Agricultural extension, Sekota Dryland Agricultural Research Center. He holds a BA degree in Development and Environmental Management Studies (Honours) from University of Gondar in 2009; and MSc in Rural Development from Haramaya University with great distinction and Excellent (A+) accreditation to his thesis, in December 2017. Ademe has practical experience and solid meticulous talent in Socioeconomic and Agricultural Extension research. He has published research articles in reputable journals (e.g. Taylor and Francis) and in ARARI’s proceeding scheme. He also reviewed scientific articles (e.g. cogent food and Agriculture) and regional Agricultural extension proceedings.
Notes
1. Technology in this study stands for full package practice comprising the recommended production components (viz., improved variety, suggested seed and fertilizer rates, inter and intra row spacing, land preparation and weeding at optimum level).
References
- Ademe, M., Asmiro, A., Lijalem, A., & Tsega, D. 2018. Participatory evaluation of hybrid sorghum technologies: In mid and low land areas of Wag-Himra zone, Eastern Amhara, Ethiopia. Proceedings of the 9th Annual Regional Conference on Completed Research Activities of Socio-Economics and Agricultural Extension Research (pp. 978-99944-927-7-0). March 9-20 2015. Bahir Dar, Ethiopia.
- Alaa, M., & Mahgoub, M. A. (2019). The impact of five environmental factors on species distribution and weed community structure in the coastal farmland and adjacent territories in the northwest delta region. Heliyon, 5, e01441. doi:10.1016/j.heliyon.2019
- Amede, A., Assefa, H., & Stroud, A. (eds.). (2004). Participatory research in action. Ethiopian experiences. Addis Ababa, Ethiopia: Ethiopian Agricultural Research Organization and the African Highlands Initiative.
- Ashby, J. A. (1986). Methodology for the participation of small farmers in the design of on-farm trials. Agricultural Administration, 22, 1–12. doi:10.1016/0309-586X(86)90103-2
- Asmiro, A., Ademe, M., Lijalem, A., & Tsega, D. (2016). Participatory evaluation of hybrid sorghum technologies: In mid and low land areas of Wag-Himra zone, Eastern Amhara, Ethiopia’. Journal of Agricultural Extension and Rural Development, 8(5), 80–88.
- Central Statistical Agency (CSA). (2015). Agricultural sample survey 2014/15 (Report on area and production of crops (private peasant holdings, meher season) (Vol. I). Addis Ababa, Ethoiopia: National annual report.
- Central Statistical Agency (CSA). (2017). Agricultural sample survey 2016/17. Report on area and production of crops (private peasant holdings, meher season) (Vol. I). Addis Ababa, Ethoiopia: National annual report.
- CIMMYT. (1998). From agronomic data to farmer recommendations, an economics training manual (Completely revised ed.). Mexico. D.F: Economic programm.
- Dereje, A. (2004). Preliminary survey of livestock production systems in Ziquala woreda, Wag-himra zone of Amhara regional state (Msc thesis). Alemaya University of Agriculture, Alemaya, Ethiopia.
- Feder, G. (2002). Adoption of agricultural innovations in developing countries. A Survey of Economic Development and Cultural Change, 32, 255–298.
- Ferdous, Z., Datta, A., Anal, A. K., Anwar, M., & Khan, M. R. (2016). Development of home garden model for year round production and consumption for improving resource-poor household food security in Bangladesh. NJAS - Wageningen Journal of Life Sciences, 78, 103–110. doi:10.1016/j.njas.2016.05.006
- Fernandez, M. E. (1988, April 11-12). Methodologies for participatory technology transformation. Workshop “Operational approaches for participative technology development in sustainable agriculture”, ILEIA (pp. 15–17). Leusden. Also in ILEIA Newsletter, 4(3) 1988, . 20p. ODI Loc. 1608.
- Geremew, G., Asfaw, A., Taye, T., Tesfaye, T., Ketema1, B., & Michael, S. (2004). Development of sorghum varieties and hybrids for dryland areas of Ethiopia. Uganda Journal of Agricultural Sciences, 9, 594–605.
- International Crop Research Institute for the Semi- arid Tropics (ICRISAT). (1991). Annual report for year 1990. The cereals program (semi-formal publication). Patancheru, A.P.
- Kleene, P. (1984). Experimental approaches in southern Mali. In P. Matlon, R. Cantrell, D. King, & M. Benoit-Cattin Eds., Coming Full Circle (pp. 131–138). Ottawa: IDRC. GDI Loc, 1801.
- Kojo, A. (1956). Analytical abstracts on farmer participatory research. Overseas Development Institute Agricultural Administration Unit Occasional Paper ODI, Agriculture Research projects Regent’s College, Inner Circle (pp. 10 85–138). Regent’s Park, London.
- Matlon, P. (1984). Technology evaluation: Five case studies from West Africa. In P. Matlon, R. Cantrell, D. King, & M. Benoit-Cattin Eds., Coming full circle (pp. 95–118). Ottawa: IDRC. GDI Loc. 1801.
- Ogwal-Kasimiro, O., Wakulira, J., Kiyini, M., Mwebaze, R., & Yiga, D. (2012, September 24–28). Dissemination of agricultural technologies: A new approach for Uganda. Paper presented at Third RUFORUM Biennial Meeting. Entebbe, Uganda. doi:10.1094/PDIS-11-11-0999-PDN
- Russell. (1997). Pair wise ranking made easy, In PLA notes No 28, Methodological complimentary’ (pp. 25–27). London: International Institute of Environmental and Development (IIED.
- Sanghi, N. K. (1987, July 26-31). Participation of farmers as co-research worker: Some case studies on dryland agriculture. Workshop on “Farmers and agricultural research: Complementary methods” (pp. 28). IDS, University of Sussex. GDI Loc. 1607.
- Siriwardena, S. A. L. (1988, April 11-12). Problems of application of new input technology where there is no farmer participation, some experiences in the Mahaweli settlement scheme, Sri Lanka. Workshop “Operational approaches for participative technology development in sustainable agriculture”. Leusden: ILEIA. 13 GDI Loc. 1608. doi:10.3168/jds.S0022-0302(88)79586-7
- Sollows, J. (2012). On-farm research: Some thoughts’ (pp. 5). Ubon, Thailand: CUSO. GDI Loc. 1696.
- SPSS. (2007). SPSS user’s guide, released V-16 editions’. Cary, North Carolina: Author.
- Woreda Office of Agricultural Development (WoA). (2015). Basic geographical information of Abergele Woreda: A working manual. Prepared by regional advisory experts. Bahir Dar, Ethiopia.
- Yadav, D. B., Kamboj, B. K., & Garg, R. B. (2004). Increasing the productivity and profitability of sunflower through front line demonstrations in irrigated agro-ecosystem of eastern Haryana’. Haryana Journal of Agronomy, 20(1–2), 3335.