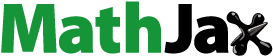
Abstract
One way of fostering smallholder farmers’ agricultural productivity is through encouraging agricultural technology adoptions. However, agricultural technology adoption remains very low in Ethiopia. As a consequence, many studies have been researched why agricultural technology adoption remained low. Nonetheless, a handful information is available on aggregate factors influencing agricultural technology adoption in Ethiopia. Therefore, very little is known about the common factors affecting agricultural technology adoption in Ethiopia. Hence, this meta-analysis was aimed to quantify the determinants of agricultural technology adoption from adoption literature. Based on a comprehensive and systematic search strategy, and inclusion and exclusion criterion, 12 studies conducted between 2010 and 2018 were included in this meta-analysis. Random effect model was used to identify the determinants of agricultural technology adoption in Ethiopia. Results of random effect model confirmed that age of the household head, education level, farm size, livestock holding, access to extension services, access to credit services, cooperative membership and distance from the market were significantly associated with agricultural technology adoption. Therefore, policy makers and other stakeholders should focus on common variables found to affect agricultural technology adoption in designing policies that encourage agricultural technology adoption in Ethiopia.
PUBLIC INTEREST STATEMENT
In developing countries, agricultural technology adoption remains a focal government policy to improve production and productivity in ensuring food security and eradicating poverty. Adoption of multiple agricultural technologies as recommended by experts has a crucial importance to achieve a desired outcomes if complemented with good farming practices. In Ethiopia, it has been argued that different socio-economic, institutional, and marketing factors are critical factors affecting adoption of agricultural technologies such as improved seed, fertilizer, and soil and water conservation practices. However, there is a scanty of researches that show common factors affecting adoption of agricultural technology at the country level. Hence, in the current paper, attempts were made to identify the factors affecting agricultural technology adoption. This helps policy makers to focus on the common factors affecting adoption of agricultural technology.
1. Introduction
Ethiopia is among the poorest countries in the world, highly drought-prone and has an agricultural sector that accounts for 85% of employment (Dercon et al., Citation2012). Despite the efforts made in the recent years, and fastest economic growth recorded over the last decade, food production and distribution is still a major challenge in the country. Hence, improving living conditions and food security of smallholder farmers through increasing agricultural growth remains the main objective of decision makers in the country (Admassie and Ayele, Citation2010). Many studies suggested that the way forward is fostering smallholders’ productivity, which mainly depends on the adoption of suitable new or improved agricultural technologies (Admassie and Ayele, Citation2010; Tura et al., Citation2010).
Ethiopia’s population is expected to grow to 160 million by 2050 (Josephson et al., Citation2014). Farm sizes have been rapidly declining, increasing the need for agricultural intensification (McDermott et al., Citation2010). Therefore, increasing the productivity of smallholder farmers through improved technology has become a policy priority for the government of Ethiopia (Abebaw & Haile, Citation2013). Agricultural technology adoption is assumed to improve the welfare of adopters through higher crop yields, reduced per unit cost of production which leads to higher own personal consumption and disposable income (Zeng et al., Citation2017). However, agricultural technology adoption remains very low in Ethiopia. For example, in 2014/15 cropping season, adoption rate of fertilizers, improved seed, pesticides and irrigation were 55.06%, 8.55%, 22.32% and 6.15%, respectively (Shita et al., Citation2018). These indicate that a lot of works need to be done to make sure that farmers perceive agricultural technologies as important contributor of agricultural productivity and ensure better adoption at the farm level.
Several studies have been conducted on the determinants of agricultural technology adoption in Ethiopia as shown by the increasing number of publications (for example, Admassie and Ayele, Citation2010; Aweke, Citation2013; Kassa et al., Citation2014; Ketema et al., Citation2016). Their findings revealed that different factors such as; demographic, institutional, socio-economic characteristics hindered adoption of improved and/or new agricultural technologies in Ethiopia. As a result, it had not been possible to benefit from existing/new agricultural technologies which would have been contributed to the achievement of national/regional goals such as eradicating poverty, ensuring food security, and overall economic development.
A study conducted by Tura et al. (Citation2010) showed that agricultural technology adoption decision of the farmers is influenced by land ownership, credit access, distance to the nearest market, livestock holding. The authors also indicated that agricultural technology adoption has significant and positive effect on farmers’ income. Another study conducted by Masresha et al. (Citation2017) on adoption of improved white haricot beans revealed that the decision to adopt white haricot beans variety was influenced by various factors. The study found that frequency of extension visits, land size allocated to haricot beans, agricultural income, price perception, training obtained, and perception on fertility enhancement benefit of the crop positively affected farmers’ decision to adopt improved variety, and distant to market, ownership of haricot beans farm land (tenure) and nutritional perception of the crop affected negatively.
Recent studies are also very much focused on the determinants of agricultural technology adoption and intensity of adoption, the extent and continued use of adopted technologies, (Aweke, Citation2013; Kassa et al., Citation2014; Masresha et al., Citation2017; Milkias & Abdulahi, Citation2018; Zeweld et al., Citation2017). These studies have underscored that identifying the determinants of agricultural technologies adoption has a vital importance for policy makers to focus on factors affecting adoption decision of farmers. It also helps government and any other bodies interested at increasing agricultural productivity, raising income, eradicating poverty and food insecurity through increased agricultural technologies adoption to design and implement their respective policy or interventions.
Although the findings of these studies indicated the determinants of agricultural technology adoption, their practical applicability at the national level is limited due to heterogeneity in the studies. This calls for a study that aggregates the findings of different studies conducted in different parts of the country. However, there is a scanty of studies conducted on meta-analysis or systematic review, which could have elaborated the determinants of agricultural technology adoption at the country level. Therefore, this meta-analysis aimed to fill this knowledge gaps by identifying factors that affects the adoption of agricultural technologies in Ethiopia. This is much better than individual studies in informing policy makers which factors are more important in agricultural technology adoption (Thomas et al., Citation2012).
The objectives of this meta-analysis were to identify the major drivers (determinants) of agricultural technology adoption in Ethiopia.
2. Data and methods
2.1. Literature search strategy
Original studies for inclusion in this meta-analysis were identified through keyword searches in relevant literature databanks. Studies were searched in the Google, Google Scholar, Research gate, and Haramaya University library search. Different key words such as adoption, agricultural technology, and Ethiopia were combined using Boolean operators to download relevant studies. Studies that have been published since 2000 were searched in the English language. However, the final studies included in the meta-analysis were published after 2010. Many studies were excluded based on the criterion explained in 2.2 in detail.
Most of the publications obtained were articles in peer-reviewed journals. Book chapters and grey literature such as conference papers, working papers, and reports in institutional series were also obtained. Some of the publications were peer-reviewed, while many were not. Some of the working papers and reports are published by research institutes or government organizations while others are NGOs publications. In this paper, the samples were not limited to peer-reviewed studies.
2.2. Selection of individual studies
After related literature have been searched, the next step was screening to identify studies which should be used in meta-analysis (). The following inclusion and exclusion criteria were used. First, study titles were checked for suitability for this meta-analysis. Accordingly, of 56 studies obtained through literature search, 10 studies were discarded as they had been conducted in other countries. Secondly, abstracts of 46 studies were critically reviewed. Studies which have analyzed other technology adoption (non-agricultural technology) have been discarded. As a result, 20 studies were excluded from full text assess for eligibility. Thirdly, 26 studies were read for full text eligibility. Based on the availability of important variables, 14 more studies were discarded. Finally, 12 studies, which have passed the inclusion and exclusion criterion were selected and used in this meta-analysis (). A complete list of the studies included in this meta-analysis is provided in the Appendix.
Table 1. Description of studies included in meta-analysis
2.3. Method of data analysis
In literature, there are different models to conduct meta-analysis. The two dominant models widely used are fixed effect model and random effect model. The key difference between fixed effect model and random effect model is that fixed-effect model which is used when the researcher believes that all the studies included in the analysis are functionally identical (Borenstein et al., Citation2009). However, when data are collected from a set of studies that had been done independently, the underlying assumption of fixed-effect model does not hold. In this case, the random-effect model is more appropriate. Several previous studies have praised importance of the random effect model in estimating the mean effect of different studies on a particular topic (see; Brockwell & Gordon, Citation2001). In this paper, the random effect model was used to synthesize the mean effects of the effect sizes.
According to Borenstein et al. (Citation2009), the mean effect was computed as:
Where k is the number of studies, is the weight related to the study i and
is the effect size collected from the study i.
The weight is calculated as:
Where is the within-study variance for study i (
) plus the between-study variance (
).
can be estimated as:
Where;
Degrees of freedom (df)
2.4. Variables hypothesized to affect agricultural technology adoption
Outcome variable: the outcome variable of the current paper is farmers’ decision to adopt or not a particular agricultural technology. Types of agricultural technology studied are portrayed in .
Independent variables: original studies included in this meta-analysis have included different demographic, socioeconomic, farm characteristics, institutional factors as determinants of agricultural technology adoption ().
Table 2. Mean effects of the determinants of agricultural technology adoption, random effect model results
3. Results and discussion
It is observed that majority of the technology adoption studies had examined factors affecting improved seed variety. Ten of the twelve studies included in this meta-analysis were conducted on the determinants of improved seed variety (). A few studies have examined determinants of agricultural technology package (combination of seed, fertilizer, herbicides). Three studies had investigated the determinants of multiple agricultural technology adoption. Overall, the percentage of agricultural technology adoption ranges from 40 to 83%. Both the lowest and highest percentage of adoption were recorded in Oromia region.
The distribution of the studies included in this meta-analysis is also portrayed in . It indicates that 8, 2, and 1 studies were conducted in Oromia, Tigray and Ahmara regions, respectively. One study had covered four major regions of Ethiopia (Oromia, SNNPR,Footnote1 Ahmara and Tigray).
3.1. Analyzing the mean effects
Many explanatory variables were used in the original studies. Out of the variables used in the original studies, about 10 variables were commonly used in most studies. presents variables used in this meta-analysis, estimated mean effects with their standard errors. Moreover, number of studies employed in each variable is also presented.
Except the two variables, namely, gender and family size, the other mean effects of all the variables included in the model were found to be significant at 1% probability level. Accordingly, age of the household head, education level, farm size, livestock holding, access to extension services, access to credit services, cooperative membership and distance from the market significantly and positively influenced agricultural technology adoption. Each of the significant variables are explained as follows:
Out of the 12 studies included in the meta-analysis, ten of them had included age of the household head as an independent variable in analyzing the determinants of agricultural technology adoption. The mean effect of age of the household head was found to have a positive influence on farmers’ decision to adopt new/improved agricultural technology. This is consistent with a previous study of Admassie and Ayele (Citation2010) who have found positive association between age of the household had and agricultural technology adoption. A plausible explanation for this is that older households might have better awareness about the benefit of new agricultural technologies from their life experiences. In addition, older households may have also larger farm size and better endowed with different assets which helps them to adopt more agricultural technology.
Education level of the household head was used in eleven studies included in the meta-analysis. The mean effect of education indicates that education level of the household head has a positive association with farmers’ decision to adopt agricultural technology. Results of original studies included in the meta-analysis have also confirmed that education level of the household head has a significant and positive effect on farmers’ agricultural technology adoption decision (Hagos & Zemedu, Citation2015; Tura et al., Citation2010).
Farm size was included in eleven of the original studies included in this meta-analysis. Its mean effect has a significant and positive effect on farmers’ decision to adopt agricultural technology. Likewise, several previous studies have also confirmed the positive effect of farm size on farmer’s decision to adopt agricultural technology (see; Abebe & Bekele, Citation2015; Hasen, Citation2015; Tura et al., Citation2010).
Likewise, livestock holding is also one of the independent variables included in ten of the studies included in this meta-analysis. The estimated mean effect of the livestock holding shows that it has a significant effect on farmers’ decision to adopt agricultural technology. The findings of some studies included in this meta-analysis also confirmed that livestock holding has a significant effect on farmer’s decision to adopt agricultural technology (Hasen, Citation2015: Kassa et al., Citation2014; Ketema et al., Citation2016).
Access to extension services was included in eight of the original studies included in this meta-analysis. Its mean effect has a significant and positive effect on farmers’ decision to adopt agricultural technology. Several previous studies have also found similar effects of access to extension services on the farmer’s decision to adopt agricultural technology (Admassie and Ayele, Citation2010; Ketema et al., Citation2016).
Access to credit is also one of the independent variables included in eleven of the original studies included in this meta-analysis. The estimated mean effect of credit access showed that it has a positive and significant effect on farmers’ decision to adopt agricultural technology. The findings of some studies included in this meta-analysis also confirmed that credit access has a positive effect on the farmer’s decision to adopt agricultural technology (Hagos & Zemedu, Citation2015; Tura et al., Citation2010).
Distance from the market is also one of the independent variables included in ten of the original studies included in this meta-analysis. In the paper, distance from the market was found to have a significant and positive effect on the farmers’ decision to adopt agricultural technology. Contrast to this, original studies result indicated that distance from the market has significant and negative effect on the farmers’ decision to adopt agricultural technology (Admassie and Ayele, Citation2010; Hagos & Zemedu, Citation2015). A plausible explanation for this contradicting result would be those households far from the market (town in most cases) have larger farm sizes and able to adopt different technologies.
Membership to cooperatives was also included in eight of the original studies included in this meta-analysis. Its mean effect has a significant and positive effect on the farmers’ decision to adopt agricultural technology. Similarly, previous studies have confirmed that membership to cooperatives has a significant effect on farmer’s decision to adopt agricultural technology (Aweke, Citation2013; Ketema et al., Citation2016).
4. Conclusion and policy implications
Several empirical studies have been conducted on the determinants of agricultural technology adoption in Ethiopia over the last four decades. As a consequence, it is evident that different factors such as demographic, institutional, and socio-economic factors have a crucial importance in adoption of improved and/or new agricultural technologies. Nonetheless, a scanty of evidence is available as to which factors are common in affecting different agricultural technology adoption at the country level.
The purpose of this meta-analysis was to quantify factors affecting agricultural technology adoption, and identify the underlying factors affecting agricultural technology adoption at the country level over the years 2010 to 2018. Twelve original studies were included for analysis. The result of the random effect model shows that age of the household head, education level, farm size, livestock holding, access to extension services, access to credit services, cooperative membership and distance from the market significantly and positively influenced agricultural technology adoption.
Therefore, policy makers and other stakeholders should focus on common variables found to affect agricultural technology adoption to realize that a new/improved practice would be adopted. This helps smallholder farmers increase their productivity and production, and helps ensuring food security.
Ethical approval
Not applicable.
Acknowledgements
The author would like to thank two anonymous reviewers and editors for their excellent insights.
Disclosure statement
The author declared no conflict of interest.
Additional information
Funding
Notes on contributors
Bekele W. Feyisa
Bekele Wegi Feyisa is an Agricultural Economist. He is a lecturer at the School of Agricultural Economics and Agribusiness, Haramaya University, Ethiopia. He obtained his MSc and BSc degrees in Agricultural Economics from Haramaya University and Addis Ababa University, respectively. Currently, he is a PhD scholar (Agricultural Economics) at Haramaya University. He also works as a PhD research fellow at The Ohio State University Global One Health. His research areas include value chain, technology adoption, food safety, food and nutrition security, international trade and impact evaluation.
Notes
1. SNNPR is the Southern Nation, Nationalities and peoples’ Region.
References
- Abebaw, D., & Haile, M. G. (2013). The impact of cooperatives on agricultural technology adoption: Empirical evidence from Ethiopia. Food Policy, 38, 82–10. https://doi.org/10.1016/j.foodpol.2012.10.003
- Abebe, Y., & Bekele, A. (2015). Analysis of adoption spell of improved common bean varieties in the central rift valley of Ethiopia: A duration model approach. Journal of Agricultural Economics and Development, 4(3), 037–043.
- Admassie, A., & Ayele, G. (2010, April). Adoption of improved technology in Ethiopia. Ethiopian Journal of Economics, 19(1), 155-179.
- Aweke, M. 2013. Factors influencing adoption of improved Maize varieties: The case of Goro-Gutu woreda of eastern Hararge, Ethiopia [MSc thesis]. Haramaya University
- Borenstein, M., Hedges, L. V., Higgins, J. P. T., & Rothstein, H. R. (2009). Introduction to meta-analysis. John Wiley and Sons, Ltd.
- Brockwell, S. E., & Gordon, I. R. (2001). A comparison of statistical methods for meta-analysis. Statistics in Medicine, 20(6), 825–840. https://doi.org/10.1002/sim.650
- Dercon, S., Hoddinott, J., & Woldehanna, T. (2012). Growth and chronic poverty: Evidence from rural communities in Ethiopia. Journal of Development Studies, 48(2), 238–253. https://doi.org/10.1080/00220388.2011.625410
- Hagos, A., & Zemedu, L. (2015). Determinants of improved rice varieties adoption in Fogera district of Ethiopia. Science. Science, Technology and Arts Research Journal, 4(1), 221–228. https://doi.org/10.4314/star.v4i1.35
- Hasen, M. (2015). Adoption of multiple agricultural technologies in maize production of the Central Rift Valley of Ethiopia. Studies in Agricultural Economics, 117(3), 162–168. https://doi.org/10.7896/j.1521
- Josephson, A. L., Ricker-Gilbert, J., & Florax, G. M. (2014). How does population density influence agricultural intensification and productivity? Evidence from Ethiopia. Food Policy, 48, 142–152. https://doi.org/10.1016/j.foodpol.2014.03.004
- Kassa, B., Kassa, B., & Aregawi, K. (2014). Adoption and impact of agricultural technologies on farm income: Evidence from southern Tigray, northern Ethiopia. International Journal of Food and Agricultural Economics, 2(4), 91–106.
- Ketema, M., Kebede, D., Dechassa, N., & Hundessa, F. (2016). Determinants of adoption of potato production technology package by smallholder farmers: Evidences from eastern Ethiopia. Review of Agricultural and Applied Economics, 19(2), 61–68. https://doi.org/10.15414/raae.2016.19.02.61-68
- Masresha, D., Legesse, B., Haji, J., & Zemedu, L. (2017). Determinants of the adoption of improved white haricot beans in east Shewa zone, south-eastern Ethiopia. Journal of Development and Agricultural Economics, 9(12), 355–372. https://doi.org/10.5897/JDAE2017.0860
- McDermott, J. J., Staal, S. J., Freeman, H. A., Herrero, M., & Van de Steeg, J. A. (2010). Sustaining intensification of smallholder livestock systems in the tropics. Livestock Science, 130(1–3), 95–109. https://doi.org/10.1016/j.livsci.2010.02.014
- Milkias, D., & Abdulahi, A. (2018). Determinants of agricultural technology adoption: The case of improved highland maize varieties in Toke Kutaye district, Oromia Regional State, Ethiopia. Journal of Investment and Management, 7(4), 125–132. https://doi.org/10.11648/j.jim.20180704.13
- Shita, A., Kumar, N., & Singh, S. (2018). Agricultural technology adoption and its determinants in Ethiopia: A reviewed paper. Asia Pacific Journal of Research, 1(55), 99–104.
- Thomas, J., Graziosi, S., Higgins, S., Coe, R., Torgerson, C., & Newman, M. 2012. Teaching meta-analysis using MetaLight. Research Note.
- Tura, M., Aredo, D., Tsegaye, W., La Rovere, R., Tesfahun, G., Mwangi, W., & Mwabu, G. (2010). Adoption and continued use of improved maize seeds: Case study of central Ethiopia. African Journal of Agricultural Research, 5(17), 2350–2358.
- Zeng, D., Jeffrey Alwang, G. W., Norton, B. S., Jaleta, M., & Yirga, C. (2017). Agricultural technology adoption and child nutrition enhancement: Improved maize varieties in rural Ethiopia. Agricultural Economics, 48(5), 573–586. https://doi.org/10.1111/agec.12358
- Zeweld, W., Hagos, A., & Hailu, G. (2017). Adoption of irrigation and its consequences on household income in the northern Ethiopia. Ethiopian Journal of Environmental Studies and Management, 10(5), 654–667. https://dx.doi.org/10.4314/ejesm.v10i5.9
Appendix
A complete List of original studies included in the analysis
Milkias, D., & Abdulahi, A. (2018). Determinants of agricultural technology adoption: the case of improved highland maize varieties in Toke Kutaye District, Oromia Regional State, Ethiopia. Journal of Investment and Management, 7(4), 125–132.
Admassie, A., & Ayele, G. (2010). Adoption of improved technology in Ethiopia. Ethiopian Journal of Economics, 19(1), 155–179.
Ketema, M., Kebede, D., Dechassa, N., & Hundessa, F. (2016). Determinants of adoption of potato production technology package by smallholder farmers: evidences from Eastern Ethiopia. Review of Agricultural and Applied Economics (RAAE), 19(395–2016-24364), 61–68.
Zeweld, W., Hidgot, A., & Hailu, G. (2017). Adoption Of Irrigation And Its Consequences On Household Income In The Northern Ethiopia. Ethiopian Journal of Environmental Studies and Management, 10(5), 654–667.
Hailu, B. K., Abrha, B. K., & Weldegiorgis, K. A. (2014). Adoption and impact of agricultural technologies on farm income: Evidence from Southern Tigray, Northern Ethiopia. International Journal of Food and Agricultural Economics (IJFAEC), 2(1128–2016-92058), 91–106.
Masresha, D., Legesse, B., Haji, J., & Zemedu, L. (2017). Determinants of the adoption of improved white haricot beans in East Shewa Zone, South-Eastern Ethiopia. Journal of Development and Agricultural Economics, 9(12), 355–372.
Tura, M., Aredo, D., Tsegaye, T., La Rovere, R., Kassie, G. T., Mwangi, W. M., & Mwabu, G. (2010). Adoption and continued use of improved maize seeds: Case study of Central Ethiopia. African Journal of Agricultural Research.
Ahmed, M. H. (2015). Adoption of multiple agricultural technologies in maize production of the Central Rift Valley of Ethiopia. Studies in Agricultural Economics, 117(1316–2016-102848), 162–168.
Ahmed, M. H. (2015). Adoption of multiple agricultural technologies in maize production of the Central Rift Valley of Ethiopia. Studies in Agricultural Economics, 117(1316–2016-102848), 162–168.
Abebe, Y., & Bekele, A. (2015). Analysis of adoption spell of improved common bean varieties in the central rift valley of Ethiopia: A duration model approach. Journal of Agricultural Economics and Development, 4(3), 037–043.
Hagos, A., & Zemedu, L. (2015). Determinants of improved rice varieties adoption in Fogera district of Ethiopia. Science, Technology and Arts Research Journal, 4(1), 221–228.
Aweke, M. (2013). Factors influencing adoption of improved maize varieties: the case of Goro-Gutu Woreda of Eastern Hararghe, Ethiopia (MSc Thesis, Haramaya University, Haramaya, Ethiopia).