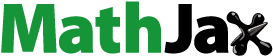
Abstract
Cereals are important in meeting the security needs of households and also as an industrial crop for the poultry and brewery industries. In recent times, climate change has been one of the great constraints to crop production and productivity including cereals. Among the various adaptation strategies is the choice and diversification of crops. Following this, there have been claims supported by various scientific disciplines to the effect that millet and sorghum are climate-resilient relative to other cereals. This study uses real production data to investigate whether indeed they are climate-resilient. The study used the Autoregressive Distributed Lag (ARDL) cointegration approach. The study employs time series data on sorghum and millet production spanning the period of 1970 to 2018 obtained from the Ministry of Food and Agriculture (MoFA). Further, rainfall and temperature data are obtained from the World Bank climate portal, and carbon dioxide (CO2) emission is obtained from Index Mundi, all spanning from the period 1970 to 2018. The results of the analysis confirm the claim that sorghum and millets are indeed climate-resilient and do not respond to changes in climatic parameters. Ljung Box test and the ARCH-LM reveals that the residuals in the models fitted are free from conditional heteroscedasticity as well as serial autocorrelation. The study joins the call for the promotion of these crops in the country for their resilience to climate, whiles continuous effort is made to make the other crops more climate-resilient.
1. Introduction
The production of cereals feeds billions of households worldwide. Projections made by the OECD estimated that cereal consumption will reach 2818 MT by 2025 with an increasing rate of 14% per annum (OECD (Organization for Economic Co-operation and Development)-FAO (Food and Agriculture Organization), Citation2016). More also, cereal yield in the continent is argued to be lower relative to the world average Macauley (Citation2015). In Ghana and many other parts of Africa, rice, maize, millet, and sorghum are essential cereals cultivated, and they contribute significantly to the Agricultural GDP of the country. These cereals also serve as staple food crops and are of great socio-economic importance.
In Ghana, cereal output for 2018 was projected at 3.4 million tons which is 9% higher than the 2017 cereal output projection, however, despite the higher projection in 2018 cereal production output, the 1.4 million tons of cereals import was required to augment domestic consumption for 2018/19 production years (FAO, IFAD, UNICEF, WFP and WHO, Citation2019). However, the outputs of these cereals in recent times have been negatively affected by climate change. This has been a great concern to many researchers as agricultural production in many ways is dependent on the weather especially in developing countries (Ben Zaied & Ben Cheikh, Citation2015). The relationship between agricultural production and climate change has been reported by empirical studies (Blanc, Citation2012; Chandio, Akram et al., Citation2022; Chandio, Gokmenoglu et al., Citation2022; Chandio, Jiang, Akram et al., Citation2021; Gbetibouo & Hassan, Citation2005; Lippert et al., Citation2009).
Although variations in climate change impacts exist globally, the adverse effect is particularly devastating in the global south countries, especially in terms of food insecurity (Chandio, Jiang, Amin et al., Citation2021). This can be linked to a myriad of factors such low adaptive capacity of farmers, variability in the start and end of the rainy seasons, variations in precipitation, low technological advancement in detecting and predicting weather conditions, variation in precipitation, and the over-dependence on natural resources (Kurukulasuriya & Rosenthal, Citation2013). In low-income countries, climate change impacts are more severe on the agricultural sector as compared with other sectors of the economy, and the quantum of such impact is expected to either intensity or at least, remain constant (McCarthy et al., Citation2001; Tol, Citation2002). Ghana as a country cannot be exempted from the negative impacts of climate change on agriculture. This invariably will have deleterious effects on the Ghanaian economy since it is agrarian. Ghana’s economy is very dependent on highly climate-sensitive sectors like forestry, hydropower, and agriculture. The agriculture sector grew by 4.8% in 2018, which was lower than the 6.1% growth rate in 2017. Agriculture’s contribution to national GDP fell from 21.1% in 2017 to 19.7% in 2018 (GSS (Ghana Statistical Service), Citation2019).
At the Paris conference of the members of the UN Framework Convention on Climate Change (UNFCCC) in 2015, adaptation to climate change was the main outcome with over 90% of the participating countries including agriculture in their implementation plan (Lipper & Zilberman, Citation2018). Various adaptation strategies have been proposed or implemented. Based on the literature, Abou et al. (Citation2021), outlined 3 different categorizations of adaption strategies. These various categorizations of adaptation strategies vary across farming systems, varieties, and locations (Hassan & Nhemachena, Citation2008). Among the adaptation strategies, are conservation agriculture, change in the topography of the land, change in farming systems, change in the timing of farm operations, use of different crop varieties, diversification of crop production, crop and livestock integration, intensifying the use of agricultural inputs, drought, and climate-resilient crops, resorting to early maturing varieties of crops, supplementary irrigation, protecting against soil erosion, tree planting, temporal or permanent migration, etc. (Akinnagbe & Irohibe, Citation2014; Asare-Nuamah et al., Citation2021; Belay et al., Citation2017; Fagariba et al., Citation2018; FAO & UNDP, Citation2020; Mushore et al., Citation2021; Sims et al., Citation2017). The integration of these adaptation strategies and mitigation with the sole aim of improving food security is what is referred to as Climate Smart Agriculture (CSA; Asfaw & Branca, Citation2018). Furthermore, the adoption of these adaptation strategies is faced with challenges, especially in less developing countries like Ghana. These challenges are inherent in the individual, societal and institutional factors (Antwi-Agyei & Nyantakyi-Frimpong, Citation2021; Mccarl et al., Citation2016). Within the context of Ghana, this climate change adaptive capacity is much lower in the northern regions of Ghana (CARE, Citation2013).
Under the adaptation strategies that border on crop diversification, the chosen crops are expected to be climate-resilient. Whiles cassava and sweet potato are labeled as climate-resilient among roots and tubers (Githunguri & Njiru, Citation2021), millet and sorghum are the most climate-resilient cereals (Dhankher & Foyer, Citation2018; Nciizah et al., Citation2021). These conclusions are as a result of rigorous scientific studies in agronomy. It has been found that these crops relative to other crops in the same category are more productive when subjected to high temperatures and drought. For this study, which focuses on cereals, millet and sorghum are found to exhibit these drought-tolerant characteristics compared with maize, rice, and soybean, hence their designation as climate resilience cereals (Armah et al., Citation2011; Nciizah et al., Citation2021).
The designation of millet and sorghum as climate resilience with the mountain of scientific and agronomic evidence has never been in doubt (Armah et al., Citation2011; Dhankher & Foyer, Citation2018; Nciizah et al., Citation2021; Wang et al., Citation2018). This designation is further strengthened by the fact that these crops are more indigenous, more nutritious, more pest-resistant, have low input requirements, and can be stored for a longer period compare with other cereals (Chazovachii et al., Citation2010; Mukarumbwa & Mushunje, Citation2010; Mutasa, Citation2011; Saleh et al., Citation2013).
The objective of this study is to investigate whether production data for these cereals supports the fact they are climate-resilient. Despite the scientific support for these crops as climate-resilient, this study attempts to subject it to data with climatic variables and outputs of millet and sorghum from 1970 to 2018 in the Ghanaian context. The results of this study will strengthen or otherwise the claim that millet and sorghum have been climate-resilient crops from the agronomic point of view.
2. Millet and sorghum resilience to climate change
Millet and sorghum are considered by many agronomists as important climate-resilient crops. This assertion often stems from the fact that these crops relative to other cereals (maize, rice, soybean, etc.) are productive in hot areas owing to their drought-tolerant characteristics (Armah et al., Citation2011; Nciizah et al., Citation2021). In addition to the drought tolerance of these crops, Mutasa (Citation2011), added that they are indigenous to African people and more importantly have more nutritional benefits than rice and maize. Furthermore, the ability of these crops to be stored for a relatively long time without perishing adds to the argument in favor of them being climate-resilient (Mukarumbwa & Mushunje, Citation2010). They have also been proven to be high temperature tolerant making them climate-resilient crops for arid and semiarid areas where rainfall is low (Dhankher & Foyer, Citation2018). In addition to the above, Chazovachii et al. (Citation2010) found that millet and sorghum compared to other cereals are resistant to pests and diseases in both pre-harvest and post-harvest storage. They also have the lowest input requirements and relatively early maturing (Saleh et al., Citation2013). Another important aspect of these crops is that their production and consumption are not only “good for the individual (nutritious and healthy) and good for the farmer (climate-resilient)” but “good for the planet (environmentally sustainable)” (Kane-Potaka et al., Citation2021). Aside from individual empirical studies, Wang et al. (Citation2018) made a global review of literature on the effects of climate change on cereal crop yields. On millet and sorghum, they concluded they are climate tolerant, and environmentally sustainable because they emit fewer greenhouse gases coupled with the fact that they require little or no input of fertilizer compared with other cereal crops.
Millet and sorghum have also been proven to reduce food insecurity which has an extricable link to climate change (Burke & Lobell, Citation2010). In a study across Africa, Taylor (Citation2003) declared sorghum and millet as the most important cereal to achieve food security in Africa. Further details of this assertion is expanded by Muzerengi and Tirivangasi (Citation2019), who stated that the basis for this relationship lies in the fact that millet and sorghum can be stored for over 5 years making them readily available. They posited further that a small amount of these crops are needed when preparing meals, and they can give more energy and satisfy hunger much better.
Especially in the context of Ghana, we have not found a study that seeks to establish millet and sorghum as climate-resilient crops with time-series data. Those that have made attempts normally aggregate all cereals for the analysis, and have mostly concluded that cereals are not climate resistant. An example is the work of Amponsah et al. (Citation2015), who found a negative long-run and short-run relationship between one of the climatic variables (Carbon dioxide concentration—CO2) and cereal yield. Even though they employed the same estimation techniques as this study (the Auto-Regressive Distributed Lag (ARDL) cointegration approach), they measured a single climatic variable against an aggregation of cereals. Lesk et al. (Citation2016) also found a negative relationship between cereals and extreme weather disasters (droughts and extreme heat) which are found to be direct consequences of climate change. With combined cereal data, Ben Zaied and Ben Cheikh (Citation2015), as well as Casemir and Diaw (Citation2018), found a negative relation between annual temperature and cereal production.
For studies that segregated cereals, most of them relied on simulated predictive or historical data for the analysis. Using predictive data and models, Mohamed et al. (Citation2002), Roudier et al. (Citation2011), and Blanc (Citation2012) found millet production to decrease under all climatic scenarios in Niger, West Africa, and SSA, respectively. Blanc (Citation2012) however, was quick to add that this finding should be used with caution as “ … … predictions of the impact of climate change are beset by uncertainty.” In a study in Ghana and Senegal, MacCarthy et al. (Citation2021) found a lower climate change impact on millet and sorghum relative to other cereals. Like the previous studies, it also employed simulated predicted data for the predictive analysis. Sultan et al. (Citation2013) simulated sorghum and millet data subject to 35 possible future climate conditions in Senegal, Mali, Burkina Faso, and Niger, and found that in almost all the scenarios, these crops will be negatively affected if temperatures rise by +2°C. Furthermore, Sultan et al. (Citation2019) employed historical climate simulations for the 2000–2009 decade and concluded that for millet and sorghum, climate change caused a reduction of 10–20% and 5–15%, respectively. They further monetized the crop loss as a result to be 2.33–4.02 billion USD for millet and 0.73–2.17 billion USD for sorghum.
Among studies that segregated the cereals, the following used real data as opposed to simulated and predictive data. Amikuzino and Donkoh (Citation2012) established a long-run relationship between rainfall and selected crops in various agroecological zones in northern Ghana. The relationship was not established for millet and sorghum at some locations, which the authors attributed to their drought tolerance. Compared with this study, the scope is narrow, and as such only one climatic variable is used as an approximation to climate variability. Similar to this study is the work of Baffour-Ata et al. (Citation2021), except for the estimation technique and the inclusion of a second climatic variable (temperature). They found variations in temperature and rainfall not to have any influence on variations of millet and rice. This finding was attributed to the fact that the study did not control for several factors that could equally be responsible for the variations in these food crops. Jena and Kalli (Citation2018) in the Indian context found increasing temperatures to be responsible for the loss of nearly 16% to 23% of millet output in the period 1992-to 2013. The yield of individual food crops which include millet is found to be influenced by climatic variables in Nepal under summer and winter conditions (Aryal et al., Citation2018; Maharjan & Joshi, Citation2013). Larsson (Citation1996) found a relationship between rainfall and sorghum yield but not with millet and sesame in Sudan. He applied correlation analysis which rarely justifies a relationship, and as such depended on only one climatic variable (rainfall).
3. Materials and methods
3.1. Data types and sources
Time series data on millet and sorghum production, spanning from the period 1970 to 2018 were obtained from the MoFA. Further, data on the temperature and rainfall for the period 1970 to 2018 were obtained from the World Bank climate portal. Also, CO2 data were obtained from the Index Mundi.
3.2. Methodology
3.2.1. Trend analysis
Trend analysis shows the rise or decline of the series over time. Various trends viz the log-linear trend, linear and quadratic trends were estimated in this study. The three different models were estimated to examine which of the trends best characterizes the series.
A time trend in a time series is a linear function of time t. The model is given by;
Where is the actual value at time
is the error term and,
, are the regression coefficients to be estimated. A linear trend indicates that; the series is either increasing or decreasing at a steady rate.
If the production of sorghum or millet exhibits a linear trend, the empirical specification will be as follows;
Empirically, if a climate variable (CO2 emission, temperature, rainfall) exhibits a linear trend, then the empirical specification will be given as;
The model is given as follows if the series exhibit a quadratic trend;
Where is the actual value for time
is given as the error term and,
,
represents the regression coefficients to be estimated. A quadratic trend in a series means that it lowers and increases at a non-constant pace.
Similarly, if a cereal production variable (millet and sorghum) exhibits a quadratic, the empirical specification will be as follows;
Empirically, if a climate variable (CO2 emission, temperature, rainfall) exhibits a quadratic trend, the empirical specification will be as follows;
Log-linear representation of the model is presented as;
Where is the actual value for time
is given as the error term and,
,
represents the regression coefficients of the real values at any time.
Empirically, if a cereal production variable (millet and sorghum production) exhibits a log-linear trend, the empirical specification will be as follows;
Similarly, if a climate variable (CO2 emission, temperature, rainfall) exhibits a log-linear trend, the empirical specification will be as follows;
3.2.2. Unit root test
The study employed the Augmented Dickey–Fuller Test (ADF) test and the Kwiatkowski-Phillips-Schmidt-Shin (KPSS) Test to examine the unit root properties of the series
3.2.3. Cointegration analysis
The Cointegration concept came to being through works by Granger (Citation1981), Engle and Granger (Citation1987), and Johansen (Citation1988) as well as Johansen and Juselius (Citation1990) after many researchers provided analytical results, showing the dangers inherent in regressions between non-stationary time series variables. Most often, co-integration is applied to situations where the time series variables in their levels exhibits a unit root. The presence of cointegration indicates that a group of time series variables has a long-run equilibrium connection, indicating that the variables advance in lockstep through time and are driven by a common stochastic trend. A set of variables might have a linear or non-linear cointegration relationship.
In instances when a variable is integrated into distinct orders, cointegration analysis, as defined by Granger (Citation1981) and Engle and Granger (Citation1987), is not applicable. Regardless of whether the primary variable is being integrated of order zero I (0), integrated of order one I (1), or a mix of the two, the Autoregressive Distributed Lag (ARDL) is utilized to determine a dynamic long-run relationship between series of other orders of integration (Pesaran & Shin, Citation1999; Pesaran et al., Citation2001). The spontaneous and the long-run relationship of the variables under consideration are gotten from the parametrized results. As a result, the ARDL approach to estimating cointegration gives more realistic and efficient results. The Equilibrium Correction Model (ECM) is the reparametrized version of the ARDL method of cointegration if at least one cointegration vector is identified. Because the ARDL model is a dynamic single model equation with a comparable form to the Error Correction Model (ECM), its reparameterization is possible (Chandio, Jiang, Akram et al., Citation2021; Nkoro & Uko, Citation2016).
The ARDL model is specified below:
assume the lag order
is equal for all variables. The variables
are then allowed to be only integrated of order zero, I (0), and integrated purely of order one, I (1), or a combination of different orders of integrations.
The optimal lag orders and
can be obtained by minimizing a particular model selection criterion, e.g., AIC, BIC, HQIC.
Reparameterization in the condition of Error correction is given:
with the speed of adjustment coefficient
4. Results and discussion
4.1. Summary statistics of data
As indicated in , the average production of millet is 149,898 Mt with a minimum and a maximum production value being 74,500 MT and 245,550 MT. Furthermore, the average annual production of sorghum is 233,673 MT with a minimum and maximum production being 106,000 MT and 387,400 MT, respectively. Sorghum has the highest production average over the study period followed by millet. The higher production average of sorghum can be inferred from the fact that there is a growing demand for sorghum both for domestic consumption and demand by beverage industries.
Table 1. Descriptive statistics of cereal production
The production of sorghum has the highest variability followed by millet production with S.D. values of 35,442.6 and 81,115.
The skewness of the series shows that the series is positively skewed with a skewness value of millet and sorghum being 0.12 and 0.079, respectively. This indicates the production of millet and sorghum are leptokurtic.
In this section, the descriptive statistics of climatic variables are explored. CO2 emissions during the entire time averaged 0.344 tons per capita, with a minimum of 0.210 tons per capita and a maximum of 0.680 tons per capita, as shown in . In addition, the average annual rainfall for the entire study period is 95.546 mm, with the lowest and maximum rainfall of 68.863 mm and 114.09 mm, respectively, during the entire period. Throughout the study period, the average yearly temperature was 27.490°C, with minimum and highest values of 26.49°C and 28.24°C, respectively. Rainfall has the most variability of all the climatic variables studied, followed by temperature, and finally CO2 emissions. With a skewness value of 1.5, the CO2 emission for the study period indicates that it is positively skewed whiles rainfall and temperature have values of −0.523 and −0.486 relatively, indicating that they are negatively skewed.
Table 2. Preliminary analysis of climate variable
The CO2 and rainfall are Leptokurtic, having Excess Kurtosis values of 1.715 and 0.395, respectively. Temperature, on the other hand, has an excess kurtosis score of −0.085, indicating that the series is platykurtic.
4.2. Trend analysis of cereal production
The findings of the cereal production trend analysis show that the log-linear trend best fits all cereals (millet and sorghum) since the AIC and BIC values were the lowest. As shown in . This shows that the trend fitted accounts for a larger proportion of variations in the series.
Table 3. Trend analysis of cereal production
Millet production trends are seen to be fluctuating throughout the study period, recording a minimum in 1990 and a peak in 2010 (see, ). The fluctuating trend in millet production can be attributed to the erratic nature of rainfall since most millet production systems in Ghana are mostly rain-fed in nature.
The trend of sorghum production is indicated in . There is generally a fluctuating trend in the production series of sorghum throughout the study period with an increasing spike after 1990. The rising spikes in sorghum production can be ascribed to its increasing demand as an industrial crop by brewery industries. This fluctuating trend in the production of millet and sorghum is confirmed in studies by Baffour-Ata et al. (Citation2021), Macauley (Citation2015), and Luguterah et al. (Citation2013).
Figure 2. Trend of sorghum from 1970 to 2018.
The estimated parameters of the best fit trend of the cereals are presented in .
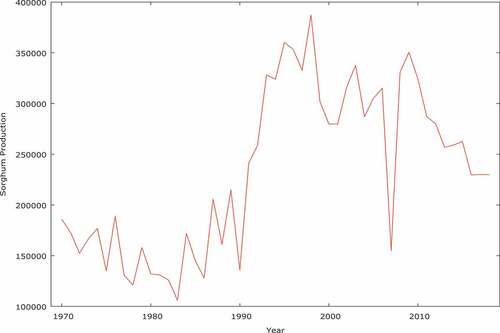
Table 4. Estimated parameters for the best-fit trend of cereal production
4.3. Trend analysis of climate variables
The trend analysis of climatic variables employed in this study is illustrated in . The best trend of CO2 emission is the log-linear trend because it has the lowest value of BIC. The log-linear trend best characterizes the rainfall and temperature series because it has the lowest values of AIC and BIC.
Table 5. Trend analysis of climate variables
CO2 emission trends indicate a fluctuating but consistent trend up to the 1990s, then increases for the remainder of the time as indicated in . The increasing trend of CO2 can be attributed to the increase in anthropogenic activities such as extraction and burning of fossil fuels, deforestation, and unsustainable agricultural practices.
As indicated in , rainfall exhibits a fluctuating trend in the series for the entire period with the lowest rainfall occurring in 1984. This finding is found to be consistent with that of Asante and Amuakwa-Mensah (Citation2015), who found that rainfall in Ghana has exhibited an inconsistent and erratic pattern over the years.
Temperature generally exhibits an increasing trend throughout the study period with periodically fluctuating trends in some years as indicated in . This finding is in line with the findings of Asante and Amuakwa-Mensah (Citation2015), who found that temperature trends in Ghana are generally variable.
The trend established in this finding of the three climatic variables (CO2 emission, rainfall, and temperature) has been confirmed by Baffour-Ata et al. (Citation2021) and Asamoah and Ansah-Mensah (Citation2020), who also found that rainfall is erratic and temperatures continue to increase over time. Aside from the manifestation of this trend in time series data, Antwi-Agyei et al. (Citation2014) found that over 80% of farmers in Ghana have perceived that, temperatures are on the rise and rainfall has become more unpredictable. A trend analysis of rainfall and temperature in neighboring Togo, which bears similar climate characteristics to Ghana also confirms the results of this study, using the Mann–Kendall Test (Gadedjisso-Tossou et al., Citation2021). In the case of CO2, the work of Owusu and Asumadu-Sarkodie (Citation2017) established that it has been on the increase as found in this study.
The study calculates the parameters for the optimal trend of the climate variables after establishing the graphical trend, as indicated in .
Table 6. Estimated parameters for the best trend of climate variables
4.4. Unit root test
The null hypothesis of the KPSS test is that the series is stationary, while the alternative hypothesis is that it is not stationary. When doing a KPSS test, the decision rule is to reject the null hypothesis if the test statistic is greater than the critical value. The null hypothesis is not rejected if the critical value is less than the critical value.
PSS test was conducted to check the results of the ADF test of the undifferentiated series. For all the series, the KPSS test was fitted for constant as well as constant and trend. The results of the KPSS test as indicated in reveals a significant test statistic that is greater than the critical value at 5% significance level for all the series except that of rainfall for the model fitted with constant as well as constant and time trend. This indicates that the null hypothesis that the series for each of the variables is stationary is rejected for all except rainfall. Rainfall, therefore, is the only variable among the series that is integrated of order zero, I (0).
Table 7. KPSS test of the series in levels
The unit-roots properties of the series were further examined after first differencing since some of the series are not integrated of order zero, I (0). The results of the KPSS test of the first differentiated series as indicated in reveals a significant test lower than the critical value for both the model fitted with constant and the model fitted with constant and time. This indicates that the CO2, temperature, millet production, and sorghum production, are integrated of order one, I (1).
Table 8. KPSS test of the first difference of the series
4.5. Auto-Regressive Distributed Lag (ARDL) cointegration
Haven examined the stationarity property of the series, the study proceeded to conduct the Autoregressive Distributed Lag cointegration test. The ARDL cointegration approach is employed in the study because some of the variables employed in the study are integrated of order zero, I (0) and hence the traditional Johansen and Juselius cointegration approaches are not applicable in this situation. The dynamic link relationship between cereal production series and climate variables is investigated using the Bounds test of Cointegration. If the F-computed value is larger than the upper bound of 5%, the null hypothesis is rejected. However, if the F-calculated value is less than the upper bound critical value at the 5% significance level, we are unable to reject the null hypothesis.
The results presented in indicate no Cointegration between any of the cereal production series and the climatic variables. In addition, the F-statistics calculated in modeling the association between millet production and climatic variables is 2.781, which is lower than the upper bounds at 5% and 1% significant levels. This designates that there exists no dynamic relationship between the climatic variables and millet production. Also, the F-calculated value obtained in examining the dynamic relationship between the sorghum production and the climatic variables is 0.625, which is found to be lower than the upper bound values at 10%, 5%, and 1% significance levels. This means the production of sorghum and the climatic variables are not cointegrated, given an indication that the null hypothesis that there is no long-run dynamic relationship between the production of millet and sorghum is not rejected, and hence there is no cointegration between the production of these cereals and climatic variables during the period under study.
Table 9. ARDL cointegration test between cereals production and climate variables
The finding is found to be partially consistent with Blanc (Citation2012), Houngbedji and Diaw (Citation2018), and Ngoma (Citation2008) who reported that there is no long-run dynamic relationship between climate change variables and cereals production as a whole. However, the finding is contrary to that of Amponsah et al. (Citation2015) and Ben Zaied and Ben Cheikh (Citation2015) who concluded that annual temperature increase results in a decrease in the production of cereals in general, whiles annual rainfall has a positive impact on the production of cereals.
4.6. Model diagnostic test
Having conducted the cointegration test, the study proceeds to conduct the model diagnostic test. The results in reveal that all the residuals in the model investigating the relationship between the cereals productions and the climatic variables in the study show that they are free from serial correlation and heteroscedasticity as the p-values for each of the models were insignificant. This means that the resultant residuals from the models are free from autocorrelation and that the error term is declared homoscedastic. This indicates that the error terms in the model can be considered white noise.
Table 10. Ljung-box test and ARCH-LM test for models millet and sorghum production and climate variables
5. Conclusion and recommendation
Crop diversification is a climate change adaptation strategy, and millet and sorghum have been promoted as climate-resilient, the study sets out to investigate this claim with time-series data. The results of the trend analysis for both the crops and the climatic variables exhibit trends not uniquely different from other studies. Though erratic, both millet and sorghum exhibited an increasing trend. Whiles the erratic nature is attributable to erratic rainfall patterns, the increasing trend is attributable to the increasing industrial demand for these cereals. On the climatic variables, CO2 emissions consistently increased from the 1990s which is attributable to increases in anthropogenic activities such as mineral extraction, burning fossil fuels, deforestation, and unsustainable agricultural practices. Rainfall over the period exhibited a fluctuating trend whiles temperature has been increasing consistently.
The ARDL estimation concludes that the production of millet and sorghum in Ghana is unhindered by the effects of climate change, confirming the claim that they are climate-resilient. This finding goes to emphasize the fact that the climate resilience status of millet and sorghum is not only based on their agronomic properties but production data.
The study, therefore, recommends that efforts by the government and the citizenry should be geared towards maintaining and reducing the amount of CO2 which can lead to decreasing the rising temperatures. The erratic nature of the rain is a signal to the country to reduce its dependence on rain-fed agriculture by establishing irrigation dams for sustaining agricultural production. Further, the MoFA and the national buffer stock should give more attention to the production of millet and sorghum as climate-resilient crops in the short run. However, in the long run, whiles still promoting these crops, there should be efforts in scientific research to make the other cereals more climate-resilient.
Disclosure statement
No potential conflict of interest was reported by the author(s).
Additional information
Funding
Notes on contributors
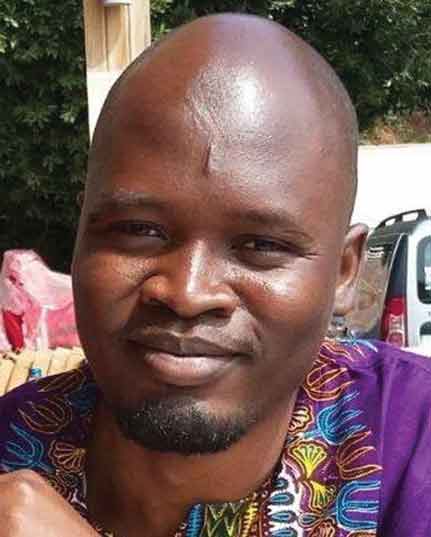
Abdul-Basit Tampuli Abukari
Mr. Karim Abdullah is a Senior Research Assistant in the department of Food Security and Climate Change, University for Development Studies, Ghana. He has interests in agricultural economics, food security, climate change and climate change modelling.
Dr. Abdul-Basit Tampuli Abukari is a Lecturer at the Department of Agricultural and Food Economics of the Faculty of Agricultural, Food and Consumer Sciences, University for Development Studies, Ghana. His interests are in agricultural economics, climate change and agricultural extension.
Dr. Abdul-Malik Abdulai is a Senior Lecturer and the Head of Department of the Department of Applied Economics, University for Development Studies, Ghana.
References
- Abou, S., Ali, M., Wakponou, A., & Sambo, A. (2021). Sorghum farmers’ climate change adaptation strategies in the semiarid region of Cameroon. In N. Oguge, D. Ayal, & L. Adeleke, I. da Silva (Eds.), African handbook of climate change adaptation (pp. 147–19). Springer. https://doi.org/10.1007/978-3-030-45106-6_41
- Akinnagbe, O. M., & Irohibe, I. J. (2014). Agricultural adaptation strategies to climate change impacts in Africa: A review. Bangladesh Journal of Agricultural Research, 39(3), 407–418. https://doi.org/10.3329/bjar.v39i3.21984
- Amikuzino, J., & Donkoh, S. A. (2012). Climate variability and yields of major staple food crops in Northern Ghana. African Crop Science Journal, 20 (2) , 349–360.
- Amponsah, L., Kofi Hoggar, G., & Yeboah Asuamah, S. (2015). Climate change and agriculture: Modelling the impact of carbon dioxide emission on cereal yield in Ghana. Published In: Agriculture and Food Sciences Research, 2(2), 32–38. https://mpra.ub.uni-muenchen.de/id/eprint/68051
- Antwi-Agyei, P., Stringer, L. C., & Dougill, A. J. (2014). Livelihood adaptations to climate variability: Insights from farming households in Ghana. Regional Environmental Change, 14(4), 1615–1626. https://doi.org/10.1007/s10113-014-0597-9
- Antwi-Agyei, P., & Nyantakyi-Frimpong, H. (2021). Evidence of climate change coping and adaptation practices by smallholder farmers in northern Ghana. Sustainability, 13(3), 1308. https://doi.org/10.3390/su13031308
- Armah, F. A., Odoi, J. O., Yengoh, G. T., Obiri, S., Yawson, D. O., & Afrifa, E. K. (2011). Food security and climate change in drought-sensitive savanna zones of Ghana. Mitigation and Adaptation Strategies for Global Change, 16(3), 291–306. https://doi.org/10.1007/s11027-010-9263-9
- Aryal, M., Regmi, P., Thapa, R., Pande, K., & Pant, K. (2018). Impact of climate variables to major food crops’ yield in midhills of western development region, Nepal. Journal of Agriculture and Environment, 17, 65–72. https://doi.org/10.3126/aej.v17i0.19862
- Asamoah, Y., & Ansah-Mensah, K. (2020). Temporal description of annual temperature and rainfall in the Bawku Area of Ghana. Advances in Meteorology, 2020, 1–18. https://doi.org/10.1155/2020/3402178
- Asante, F. A., & Amuakwa-Mensah, F. (2015). Climate change and variability in Ghana: Stocktaking. Climate, 3(1), 78–99. https://doi.org/10.3390/cli3010078gt
- Asare-Nuamah, P., Mandaza, M. S., & Amungwa, A. F. (2021). Adaptation strategies and farmer-led agricultural innovations to climate change in mbire district of Zimbabwe. International Journal of Rural Management, 097300522199991. https://doi.org/10.1177/0973005221999913
- Asfaw, S., & Branca, G. (2018). Introduction and overview. In L. Lipper, N. McCarthy, D. Zilberman, S. Asfaw, & G. Branca (Eds.), Climate smart agriculture. natural resource management and policy (Vol. 52, pp. 3–12). Springer. https://doi.org/10.1007/978-3-319-61194-5_1.
- Baffour-Ata, F., Antwi-Agyei, P., Nkiaka, E., Dougill, A. J., Anning, A. K., & Kwakye, S. O. (2021). Effect of climate variability on yields of selected staple food crops in northern Ghana. Journal of Agriculture and Food Research, 6, 100205. https://doi.org/10.1016/j.jafr.2021.100205
- Belay, A., Recha, J. W., Woldeamanuel, T., & Morton, J. F. (2017). Smallholder farmers’ adaptation to climate change and determinants of their adaptation decisions in the Central Rift Valley of Ethiopia. Agriculture & Food Security, 6(1), 1–13. https://doi.org/10.1186/s40066-017-0100-1
- Ben Zaied, Y., & Ben Cheikh, N. (2015). Long-run versus short-run analysis of climate change impacts on agricultural crops. Environmental Modeling and Assessment, 20(3), 259–271. https://doi.org/10.1007/s10666-014-9432-4
- Blanc, E. (2012). The impact of climate change on crop yields in Sub-Saharan Africa. American Journal of Climate Change, 1(1), 1–13. http://dx.doi.org/10.4236/ajcc.2012.11001
- Burke, M., & Lobell, D. (2010). Climate effects on food security: An overview. In D. David Lobell & M. Burke (Eds.), Climate change and food security: Adapting agriculture to a warmer world (Advances in global change research, 37) (pp. 13–30).
- CARE. (2013). Climate change vulnerability and adaptive capacity in Northern Ghana.
- Casemir, B. H., & Diaw, A. (2018). Analysis of climate change effect on agricultural production in Benin. Asian Journal of Agricultural Extension, Economics & Sociology, 241, 1–12. https://doi.org/10.9734/AJAEES/2018/40522
- Chandio, A. A., Jiang, Y., Akram, W., Adeel, S., Irfan, M., & Jan, I. (2021). Addressing the effect of climate change in the framework of financial and technological development on cereal production in Pakistan. Journal of Cleaner Production, 288, 125637. https://doi.org/10.1016/j.jclepro.2020.125637
- Chandio, A. A., Jiang, Y., Amin, A., Ahmad, M., Akram, W., & Ahmad, F. (2021). Climate change and food security of South Asia: Fresh evidence from a policy perspective using novel empirical analysis. Journal of Environmental Planning and Management, 1–22. https://doi.org/10.1080/09640568.2021.1980378
- Chandio, A. A., Akram, W., Bashir, U., Ahmad, F., Adeel, S., & Jiang, Y. (2022). Sustainable maize production and climatic change in Nepal: Robust role of climatic and non-climatic factors in the long-run and short-run. Environment, Development and Sustainability, 1–31. https://doi.org/10.1007/s10668-022-02111-1
- Chandio, A. A., Gokmenoglu, K. K., Ahmad, M., & Jiang, Y. (2022). Towards sustainable rice production in Asia: The role of climatic factors. Earth Systems and Environment, 6(1), 1–14. https://doi.org/10.1007/s41748-021-00210-z
- Chazovachii, B., Chigwenyu, A., & Mushuku, A. (2010). Adaptation of climate resilient rural livelihoods through growing of small grains in Munyaradzi communal area. Gutu District. Afr. J. Agric. Res, 7, 1335–1345. https://doi.org/10.5897/AJAR10.921
- Dhankher, O. P., & Foyer, C. H. (2018). Climate resilient crops for improving global food security and safety. Plant Cell and Environment, 41(5), 877–884. https://doi.org/10.1111/pce.13207
- Engle, R. F., & Granger, C. W. J. (1987). Co-Integration and error correction: representation, estimation, and testing. Econometrica, 55(2), 251–276. https://doi.org/10.2307/1913236
- Fagariba, C. J., Song, S., & Soule Baoro, S. K. G. (2018). Climate change adaptation strategies and constraints in Northern Ghana: Evidence of farmers in Sissala West District. Sustainability, 10(5), 1484. https://doi.org/10.3390/su10051484
- FAO & UNDP. (2020). Conservation agriculture for climate change adaptation in Zambia: A cost-benefit analysis.
- FAO, IFAD, UNICEF, WFP and WHO. (2019). The state of food security and nutrition in the world 2019. Safeguarding against economic slowdowns and downturns. FAO.Licence: CC BY-NC-SA 3.0 IGO.
- Gadedjisso-Tossou, A., Adjegan, K. I., & Kablan, A. K. M. (2021). Rainfall and temperature trend analysis by Mann–Kendall test and significance for rainfed cereal yields in Northern Togo. Sci, 3(1), 17. https://doi.org/10.3390/sci3010017
- Gbetibouo, G. A., & Hassan, R. M. (2005). Measuring the economic impact of climate change on major South African field crops: A Ricardian approach. Global and Planetary Change, 47(2–4), 143–152. https://doi.org/10.1016/j.gloplacha.2004.10.009
- Githunguri, C. M., & Njiru, E. N. (2021). Role of cassava and sweetpotato in mitigating drought in semi-arid Makueni County in Kenya. In W. Leal Filho, N. Oguge, D. Ayal, L. Adeleke, & I. da Silva (Eds.), African handbook of climate change adaptation, 241–259. Springer. https://doi.org/10.1007/978-3-030-45106-6_11
- Granger, C. W. J. (1981). Some properties of time series data and their use in econometric model specification. Journal of Econometrics, 16(1), 121–130. https://doi.org/10.1016/0304-4076(81)90079-8
- GSS (Ghana Statistical Service) (2019). Statistics for development and progress annual gross domestic product September 2015 Edition. September, 3.
- Hassan, R. M., & Nhemachena, C. (2008). Determinants of African farmers’ strategies for adapting to climate change: Multinomial choice analysis. African Journal of Agricultural and Resource Economics, 2(311–2016–5521), 83–104. http://dx.doi.org/10.22004/ag.econ.56969
- Houngbedji, C., & Diaw, A. (2018). Analysis of climate change effect on agricultural production in Benin. Asian Journal of Agricultural Extension, Economics & Sociology, 24(4), 1–12. https://doi.org/10.9734/AJAEES/2018/40522
- Jena, P. R., & Kalli, R. (2018). Measuring the impact of climate variability on rice and finger millet: Empirical evidence from a drought prone region of India (No. 2058-2018-5278). 2018 International Association of Agricultural Economists (IAAE) July 28-August 2, 2018, Vancouver, British Columbia. http://dx.doi.org/10.22004/ag.econ.275970
- Johansen, S. (1988). Statistical analysis of cointegration vectors. Journal of Economic Dynamics and Control, 12(2–3), 231–254. https://doi.org/10.1016/0165-1889(88)90041-3
- Johansen, S., & Juselius, K. (1990). Maximum likelihood estimation and inference on cointegration — With applications to the demand for money. Oxford Bulletin of Economics and Statistics, 52(2), 169–210. https://doi.org/10.1111/j.1468-0084.1990.mp52002003.x
- Kane-Potaka, J., Anitha, S., Tsusaka, T., Botha, R., Budumuru, M., Upadhyay, S., Jalagam, A. K., Hunasgi, R., Jalagam, A. K., Nedumaran, S., & Kumar, P. (2021). Assessing millets and sorghum consumption behavior in urban India: A large-scale survey. Frontiers in Sustainable Food Systems, 5, 260. https://doi.org/10.3389/fsufs.2021.680777
- Kurukulasuriya, P., & Rosenthal, S. (2013). Climate change and agriculture: A review of impacts and adaptations. Environment department papers; no. 91. Climate change series. World Bank. © World Bank. http://hdl.handle.net/10986/16616, License: CC BY 3.0 IGO
- Larsson, H. (1996). Relationships between rainfall and sorghum, millet and sesame in the Kassala Province, Eastern Sudan. Journal of Arid Environments, 32(2), 211–223. https://doi.org/10.1006/jare.1996.0018
- Lesk, C., Rowhani, P., & Ramankutty, N. (2016). Influence of extreme weather disasters on global crop production. Nature, 529(7584), 84–87. https://doi.org/10.1038/nature16467
- Lipper, L., & Zilberman, D. (2018). A short history of the evolution of the climate smart agriculture approach and its links to climate change and sustainable agriculture debates. In L. Lipper, N. McCarthy, D. Zilberman, S. Asfaw, & G. Branca (Eds.), Climate smart agriculture. natural resource management and policy (Vol. 52, pp. 13–30). Springer. https://doi.org/10.1007/978-3-319-61194-5_2.
- Lippert, C., Krimly, T., & Aurbacher, J. (2009). A Ricardian analysis of the impact of climate change on agriculture in Germany. Climatic Change, 97(3), 593–610. https://doi.org/10.1007/s10584-009-9652-9
- Luguterah, A., Nasiru, S., & Anzagra, L. (2013). Dynamic relationship between production growth rates of three major cereals in Ghana. Mathematical Theory and Modeling, 3(8), 68–75.
- Macauley, H. (2015). Background paper: Cereal crops: Rice, maize, millet, sorghum, wheat. African Development Bank.
- MacCarthy, D. S., Adam, M., Freduah, B. S., Fosu-Mensah, B. Y., Ampim, P. A. Y., Ly, M., Traore, P. S., & Adiku, S. G. K. (2021). Climate change impact and variability on cereal productivity among smallholder farmers under future production systems in West Africa. Sustainability, 13(9), 5191. http://dx.doi.org/10.3390/su13095191
- Maharjan, K. L., & Joshi, N. P. (2013). Effect of climate variables on yield of major food-crops in Nepal: A time-series analysis. In K. L. Maharjan, N. P. Joshi (eds.), Climate change, agriculture and rural livelihoods in developing countries (pp. 127–137). Springer. https://doi.org/10.1007/978-4-431-54343-5_9
- Mccarl, B., Thayer, A., & Jones, J. (2016). The challenge of climate change adaptation for agriculture: An economically oriented review. Journal of Agricultural and Applied Economics, 48(4), 321–344. https://doi.org/10.1017/aae.2016.27
- McCarthy, J. J., Canziani, O. F., Leary, N. A., Dokken, D. J., & White, K. S. (Eds.). (2001). Climate change 2001: Impacts, adaptation, and vulnerability: Contribution of Working Group II to the third assessment report of the Intergovernmental panel on climate change (Vol. 2). Cambridge University Press.
- Mohamed, A. B., Van Duivenbooden, N., & Abdoussallam, S. (2002). Impact of climate change on agricultural production in the Sahel–Part 1. Methodological approach and case study for millet in Niger. Climatic Change, 54(3), 327–348. https://doi.org/10.1023/A:1016189605188
- Mukarumbwa, P., & Mushunje, A. (2010). Potential of Sorghum and finger millet to enhance household food security in Zimbabwe’s semi-arid regions: A review. A Review, No 96430, 2010 AAAE Third Conference/AEASA 48th Conference, September 19–23, 2010, Cape Town, South Africa, African Association of Agricultural Economists (AAAE). http://dx.doi.org/10.22004/ag.econ.96430
- Mushore, T. D., Mhizha, T., Manjowe, M., Mashawi, L., Matandirotya, E., Mashonjowa, E., Mutasa, C., Gwenzi, J., & Mushambi, G. T. (2021). Climate change adaptation and mitigation strategies for small holder farmers: A case of Nyanga District in Zimbabwe. Front. Clim, 3, 676495. https://doi.org/10.3389/fclim.2021.676495
- Mutasa, M. (2011). Climate change vulnerability and adaptation in failing states: Zimbabwe’s Drought struggle: Paper prepared for the initiative on climate adaptation research and understanding through the social sciences (ICARUS-2) meeting at the University of Michigan (5–8 May 2011) themed, “Vulnerability and adaptation: Marginal peoples and environments initiative on climate adaptation research and understanding through the social sciences (ICARUS-2) 5-8 May, 2011 University of Michigan.”
- Muzerengi, T., & Tirivangasi, H. M. (2019). Small grain production as an adaptive strategy to climate change in Mangwe District. Matabeleland South in Zimbabwe. Jamba (Potchefstroom, South Africa), 11(1), 652. https://doi.org/10.4102/jamba.v11i1.652
- Nciizah, T., Nciizah, E., Mubekaphi, C., & Nciizah, A. D. (2021). Role of small grains in adapting to climate change: Zvishavane District, Zimbabwe. In W. Leal Filho, N. Oguge, D. Ayal, L. Adeleke, & I. da Silva (Eds.), African handbook of climate change adaptation, 581–599. Springer. https://doi.org/10.1007/978-3-030-45106-6_254
- Ngoma, J. (2008). Effects of climate change on maize production in Zambia. In Department of water and environmental studies, 1–74. Linköping University, Campus Norrköping, SE-601 74.
- Nkoro, E., & Uko, A. K. (2016). Autoregressive distributed lag (ARDL) cointegration technique: Application and interpretation. Journal of Statistical and Econometric Methods, 5 (4), 63–91. SCIENPRESS Ltd.
- OECD (Organization for Economic Co-operation and Development)-FAO (Food and Agriculture Organization) (2016). OECD-FAO agricultural outlook 2016–2025, OECD Publishing. http://dx.doi.org/10.1787/agr_outlook-2016-en
- Owusu, P., & Asumadu-Sarkodie, S. (2017). Is there a causal effect between agricultural production and carbon dioxide emissions in Ghana? Environmental Engineering Research, 22(1), 40–54. https://doi.org/10.4491/eer.2016.092
- Pesaran, M., & Shin, Y. (1999). An autoregressive distributed-lag modelling approach to cointegration analysis. In S. Strøm (Ed.), Econometrics and economic theory in the 20th century: The Ragnar Frisch centennial symposium (Econometric Society Monographs), 371–413. Cambridge University Press. https://doi.org/10.1017/CCOL521633230.011
- Pesaran, M. H., Shin, Y., & Smith, R. J. (2001). Bounds testing approaches to the analysis of level relationships. Journal of Applied Econometrics, 16(3), 289–326. https://doi.org/10.1002/jae.616
- Roudier, P., Sultan, B., Quirion, P., & Berg, A. (2011). The impact of future climate change on West African crop yields: What does the recent literature say? Global Environmental Change, 21(3), 1073–1083. https://doi.org/10.1016/j.gloenvcha.2011.04.007
- Saleh, S. M. A., Zhang, Q., Chen, J., & Shen, Q. (2013). Millet grains: Nutritional quality, processing, and potential health benefits. Comprehensive Reviews in Food Science and Food Safety, 12(3), 281–295. https://doi.org/10.1111/1541-4337.12012
- Sims, R., Flammini, A., Santos, N., Dias Pereira, L., Carita, A., Bracco, S., & Oze, D. (2017). Adoption of climate technologies in the agrifood sector FAO 80 Methodology. FAO Investment Centre. Directions in Investment (FAO) eng no. 12.
- Sultan, B., Roudier, P., Quirion, P., Alhassane, A., Muller, B., Dingkuhn, M., … Baron, C. (2013). Assessing climate change impacts on sorghum and millet yields in the Sudanian and Sahelian savannas of West Africa. Environmental Research Letters, 8(1), 014040. https://doi.org/10.1088/1748-9326/8/1/014040
- Sultan, B., Defrance, D., & Iizumi, T. (2019). Evidence of crop production losses in West Africa due to historical global warming in two crop models. Scientific Reports, 9(1), 1–15. https://doi.org/10.1038/s41598-019-49167-0
- Taylor, J. R. N. (2003, April). Overview: Importance of sorghum in Africa. Afripro: Workshop on the Proteins of Sorghum and Millets: Enhancing Nutritional and Functional Properties for Africa, Pretoria, 2(4), 1–21.
- Tol, R. S. (2002). Estimates of the damage costs of climate change. Part 1: Benchmark estimates. Environmental and Resource Economics, 21(1), 47–73. https://doi.org/10.1023/A:1014500930521
- Wang, J., Vanga, S. K., Saxena, R., Orsat, V., & Raghavan, V. (2018). Effect of climate change on the yield of cereal crops: A review. Climate, 6(2), 41. https://doi.org/10.3390/cli6020041