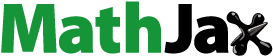
Abstract
Information communication technology is energetic for rural transformation and plays a great role in agricultural development. However, there are factors affecting farmers’ use of information. This study focused on determinants of various agricultural information sources. Household survey from 165 respondents and multi-stage sampling techniques were used. Multivariate probit model regression output shows that social participation and distance are significantly related with the use of all information sources at different significance levels. Age, extension contact, education, sex, credit access, land size, non/off-farm income and farm income are significant with the use of different information sources at different significance levels. The Wald test (χ2 (56) = 101.39, p = 0.000) is significant, which indicates that MVP model fits the data. LR (χ2 (6) = 232.942, Prob > χ2 = 0.0000) indicates the goodness of fit of the model. For more use of agriculture-related information, Ministry of Agriculture and NGOs need to intervene in rural agricultural programs.
1. Introduction
Information and communication technologies (ICTs) provide much opportunity to transfer knowledge and information (Tadesse et al., Citation2017). The rapid evolution and uptake of digital technologies hold great promise for agriculture in developing countries including Ethiopia, and it is associated with motivation, digital literacy and attitude changes toward information and communication technologies (Baumuller, Citation2013; Soomro et al., Citation2018). Ethiopian’s agriculture is based on rain-fed agriculture and exposed to climate change (Andersson et al., Citation2011). information provision is the central for advanced agriculture. So that information from different source is needed to boost farmers’ livelihood (Eakin et al., Citation2018; Yaseen et al., Citation2016). However, there are factors affecting farmers’ use of information (Kumar & Chander, Citation2011).
Information is essential in agronomic practices and animal-husbandry-related issues (Naveed & Anwar, Citation2013). It is vital for rural development, better farm productivity and enhances farm productivity (Naruka et al., Citation2017; Ommani & Chizari, Citation2008; Pezeshki-Rad & Zamani, Citation2005). Moreover, information regarding agricultural extension services and dissemination of meteorology information advances rural livelihoods (Baumuller, Citation2013). Because of these reasons, modern information communication technology plays a countless role in delivering information to farmers (Mittal & Mehar, Citation2016).
Extension agents are another source of information, educating and training farmers to change their attitude (Prokopy et al., Citation2015), whereas education, gender, age, knowledge and skill affect extension agents (Jayaratne, Citation2014; Olorunfemi et al., Citation2020; Zikhali, Citation2016). Adequate information and timely training on climate change, the effect and initiatives to combat is a critical factor of the functional competency of extension agents (Gombe et al., Citation2015; Olorunfemi et al., Citation2020; Ragasa et al., Citation2016). This leads to slow rural development, and the rural farmers cannot make proper contribution to the national development of the country (Asenso-Okyere & Mekonnen, Citation2012).
Farmers’ access to different information sources can improve agricultural productivity, practices and their livelihoods (Asenso-Okyere & Mekonnen, Citation2012). State government and agricultural agencies are also the sources of information (Adio et al., Citation2016). Mobile phones have its own impact on farmers’ marketing decisions and prices in rural Ethiopia (Tadesse & Bahiigwa, Citation2015). SMS is one of the ways to share agricultural information for rural farmers and extension officers in Ethiopia (Tegegne & Alemu, Citation2019). However, there are different constraints that affect rural farmers’ participation in the usage of agricultural information and make them unable to produce more. Thus, farmers’ participation in extension planning is very weak. Moreover, the small size of farms, lack of organizational unity among farmers and instability of national agricultural policies have played a part in undermining the helpfulness of the agricultural information delivery. To this end, this study tries to fill the gap in farmers’ usage of agriculture-related information in the study area.
2. Study area and methodology
The study was conducted in Hadiya Zone in Mirab Badawacho woreda. The capital town of Mirab Badawacho is Danema. It is 352 km far from southwest of Addis Ababa and 127 km from the regional capital, Hawassa. The total population of woreda is 83,439. Geographically, the absolute location of the district is between 07°69ʹ00″N to 07°91ʹ91″N latitude and 37°95ʹ00″E to 38°10ʹ00″E longitude. Mirab Badawacho woreda is bordered on the north and northeast by Kambata Tambaro Zone, on the east by Misrak Badawacho, on the south by Wolaita Zone and on the northwest by Kachabira. Agriculture is the main source of income such as Teff, Maize, Haricot beans, Enset and Banana. Coffee and chat are also important cash crops in the study area.
Both qualitative data and quantitative data types were used. Data source such as primary data source was used. For this study, semi-structured questionnaire and key informant interview schedule were used as tools of data collection.
Multi-stage sampling techniques were used for this study. In the first stage, Mirab Badawacho was selected purposively based on its relative potential in agricultural production. In the second stage, three kebeles were randomly selected from 22 kebeles. In the third stage, 165 sample units were selected by using simple random sampling technique. Yamane (Citation1967) formula was applied to determine the sample size.
The formula:
N = total population, n = sample unit, and e = error term.
Data were analyzed by using STATA software. Both descriptive and econometric statistics were used. Moreover, econometric model was employed to analyze the determinants of various agricultural information sources.
For this study, multivariate probit model was used. It is a generalization of the probit model used to estimate several correlated binary outcomes jointly. It is one form of a correlated binary response regression model that simultaneously estimates the influence of independent variables on more than one dependent variables. It allows for the error terms to be freely correlated. Empirically, the model can be specified as follows:
Yi1 = X * im1β1 + εi1
Yi2 = X * im2β2 + εi2
Yi3 = X * im3β1 + εi3
Yi4 = X * im4β1 + εi4
Yi where, i = farmer id, Yi1 = 1, if farmer uses extension agents (0 otherwise), otherwise, Yi2 = 1, if farmer uses modern information and communication technology (0 otherwise), Yi3 = 1, if farmer uses social network (0 otherwise), and Yi4 = 1, if farmer uses traditional media (0 otherwise). X′i = vector of factors affecting farmers decision to participate in various agriculture-related information, βm = vector of unknown parameters (m = 1, 2, 3, 4), and ε is the error term.
3. Results and discussion
The total households in the study area were 873 (). The result of this study shows that the mean age of farmers in the study area was 46.30 (Table ). This implies that farmers in the study area were productive and strive to utilize information for their farm activity. The mean of family size in the study area was 6.41 (Table ). Mean land size and farming experience in the study area were 0.85 ha and 8.84 years, respectively (Table ). The mean of farming experience in the study area was 8.84 (Table ). The mean distance from kebele extension office was 2.28 km, and the mean farm income and off/non-farm income were 9612.73ETB and 5286.15ETB, respectively (Table ).
Table 1. Study kebeles, total population, and number of sample respondents
Table 2. Descriptive statistics for continuous variables
Sex affects the farmer’s use of information. In this study, 93.33% of farmers were male and 6.67% were female (Table ). The marital status of household was single (1.82%), married (91.52%), divorced (2.42%) and widowed (4.24%; Table ). In the study area, 84.85% read and write and only 15.5% farmers did not have any formal education (Table ). Overall, 64.85% of farmers participated in Iddir and Equb in Ethiopia and there were only 35.15% of farmers who did not participate in any institutions (Table ). From total respondents, 63.03% of farmers had an access to credit and 36.97% of farmers had no access to credit (Table ). In the study area, 44.85% of farmers strongly agree and 6.67% of farmers strongly disagreed on current extension technology (Table ). There were 32.73% of farmers in the study area who had a contact with extension agent every week and 0.61% had a contact with extension agent once in a year (Table ).
Table 3. Descriptive statistics for categorical variables
3.1. Determinants of framers’ use of information for sustainable agricultural development
The Wald test (χ2 (56) = 101.39, p = 0.000) is significant, which indicates that the model is jointly significant and MVP model fits the data (Table ). LR (χ2 (6) = 232.942, Prob >χ2 = 0.0000) indicates that the independence between the source of information utilization for extension services is significant (Table ).
Table 4. Joint correlations for the estimated coefficients across the equations in the models
Table 5. Multivariate probit model result
Access to agricultural information services is biased, and the four information sources are interdependent decisions. Using agricultural information source among rural households is reflected in the estimated correlation matrix. The correlation between accessing information through extension agents and modern information and communication technology is negative and statistically significant. There is a competitive relationship between extension agents and modern information and communication technology (Table ).
The correlation between accessing information through extension agents and social networks is positive and statistically significant. There is a mutual relationship between accessing information through extension agents and social networks (Table ). The correlation between accessing information through extension agents and traditional media is positive and statistically significant (Table ). There is a mutual relationship between accessing information through extension agents and social networks. The correlation between accessing information through modern information and communication technology and social networks is positive and statistically significant. There is a mutual relationship between accessing information through modern information and communication technology and social networks (Table ). The correlation between modern information and communication technology and traditional media is significant and negatively interdependent. There is a competitive relationship between modern information and communication technology and traditional media, while correlation between social network and traditional media is significant and positively associated, indicating a mutual relationship between modern information and communication technology and traditional media (Table ).
The result in Table shows that some of the explanatory variables were significant to affect farmer’s decision of accessing agricultural information from particular information sources. The result reveals that information source is influenced by the same or different factors.
Multivariate probit (SML, # draws = 5) Number of obs = 165
Log likelihood = −194.2328 Wald chi2 (56) = 101.39
Prob > chi2 = 0.0002
For farmers’ use of agricultural information from extension agent, sex, social participation, distance and credit utilization variables are significant at 10% level of significance. Sex has a negative association with extension agents. This implies that male households have better chance to communicate with extension agents. The result is consistent with the study by Mittal and Mehar (Citation2016) which proves that women households were influenced by different factors. The coefficient for social participation is positive, indicating that farmer who had participated in different social organizations was more likely to communicate with extension agents. A study by Xu et al. (Citation2021) revealed that the norms in organization influence the process of farmers’ participation in integrated pest management. The coefficient for distance is negative; this indicates that farmer who is far from the extension service area is less likely to pursue extension services. This finding is consistent with that of Gido et al. (Citation2015). The coefficient for credit utilization is positive and this shows that access to credit influences farmers to use different sources for agricultural information. A study by Tadesse et al. (Citation2017) stated that credit had positive association and significant influence on agricultural activity.
In farmers’ utilization of modern communication technology information source equation, age, social participation and distance variables are significant. Age of households has a negative relation with likelihood of access to agricultural information through modern information and communication technology at 10%. This reflects that aged farmers most of the times come up with their previous knowledge and they are not eager to adopt new and updated evidence. The finding is consistent with the result of Tadesse et al. (Citation2017), Tadesse and Bahiigwa (Citation2015) and Daniso et al. (Citation2020). Their findings stated that technology is usually adopted faster by younger people in Ethiopia. The coefficient for social participation is negative, which indicates that distance influences farmers’ use of information. Hasan et al. (Citation2013) stated that distance to extension services is unlikely to have a direct effect on agricultural productivity. The coefficient for distance is positive, which indicates that distance does not have a significant effect. A study by Lee and Bellemare (Citation2013) confirmed that mobile phone is used to communicate and ask the market price for their production.
In farmers’ use of information from social network (farmers’ group, relatives and village leader) equation, land, social participation, distance, credit utilization and non/off-farm income variables are significant. The coefficient for land is positive, indicating that farmers who had more unit of land used information from farmer groups, relatives and village leader. The coefficient for social participation is positive, showing that farmers who had participated in different organizations had access to more information. Farmers who had participated more in social network groups have more free time and they also need to expand their farmer networks (Aonngernthayakorn & Pongquan, Citation2017). The coefficient for distance is negative which implies that distance from village or nearby community affects farmers’ access to information from relatives. This study is consistent with the study by Gido et al. (Citation2015) who found that distance negatively influenced the likelihood of a higher demand for extension services. Credit utilization has positive association with social networks; this denotes that farmers who had an access to credit/loan produce more. A study by Hussain and Taqi (Citation2014) confirmed that long-term loans have positive impact on productivity. Income from off/non-farm has a negative relation with social networks at 5%. The result implies that a household who gets income from off/non-farm does not use social networks to access agricultural information. A study by Gido et al. (Citation2015) confirmed that farmers who are involved in off-farm activities do not communicate with social network for agriculture-based activities.
In farmers’ use of traditional media source equation, education, social participation, distance, credit utilization, farm income and frequency of extension contact variables are significant. Education has a positive relationship with traditional media and is significant at 5% level; this implies that educated farmers use traditional media to access agricultural information. The result is aligned by Katengeza (Citation2012). The coefficient for social participation is positive which implies that farmers who had participated in different social organizations utilized information from traditional media (such as radio and television). This finding is consistent with that of Mittal et al. (Citation2010) and Aker (Citation2011). The coefficient for distance is negative, indicating that distance affects farmers’ utilization of traditional media. Tadesse and Bahiigwa (Citation2015) found that farmers who are closer to markets had better access to information in Ethiopia. Credit utilization has a positive relationship with traditional media; this shows that farmers who had an access to credit are willing to use agricultural information from traditional media source. The result from the study by Ahmad et al. (Citation2018) confirmed that credit is essential for facilitating the flow of agricultural inputs. Farm income is negatively associated with traditional media, indicating that farmers do not use traditional media sources. A study by Mittal and Mehar (Citation2016) confirmed that farmers are highly interested in other media sources for farm risk management. The coefficient for frequency of extension contact is negative. The negative and significant result shows that farmers are not willing to contact extension agents for traditional media (television, radio and newspaper) to get useful information. The result is in line with that of Mittal et al. (Citation2010) and Aker (Citation2011). On the contrary, Mtega (Citation2012) found out that extension contact had positive effect on usage of information among rural communities in Tanzania.
4. Conclusion and recommendations
Information about agriculture is obtained from different sources. In this paper, the sources of agricultural information are extension agent, modern information and communication technology, social network and traditional media. However, the farmers’ use of information was determined by different factors. This study focused on determinants of farmers’ decision level to use different agricultural information for sustainable agricultural development. To help farmers to be accessible for agriculture-based information, the study aimed at suggesting recommendations. Considering the contribution of information in agriculture, the government of Ethiopia should emphasize on the agriculture-based information.
Distance is negatively affecting rural farmers’ utilization of agricultural information from extension agent, social network and traditional media. Therefore, the government of the country should intervene through increasing number of extension workers, creating opportunity of farmers to farmers’ linkage and fulfill information technology infrastructures. Social participation is positively associated with the information source selection of rural farmers, particularly extension agent, social network and traditional media. Local government needs to promote social participation such as Iddir and Equb to disseminate agricultural information for rural mass peoples. However, social participation is negatively associated with information access with modern communication technology. Therefore, enhancing farmers’ use of information and creating awareness about all information source utilization are needed. Credit access is positively related with the information utilization from extension agent, social network and traditional media. Credit user farmers strived to get new technology and new information. Due to this, local government of Ethiopia should create credit access opportunity from different microfinance institutions for rural farmers.
An education influences farmers to use new innovative technology, and it is positively associated with traditional media. Therefore, the government of Ethiopia particularly Ministry of ICT and Ministry of Innovation Technology should take an initiative to increase adult literacy education program in rural areas. Farm income and non/off-farm income are negatively associated with farmers utilization of information from both traditional media and social network. The local government needs to create awareness among farmers regarding the importance of using information for their farm activities. Land is an important resource, and it is positively associated with information utilization by rural farmers from social network. Creating awareness in significance of social network can enhance farmers’ productivity; therefore, the stakeholders such as extension workers, community leader, and woreda agriculture and natural resource office should create awareness among rural farmers.
Acknowledgements
The author wishes to thank Wolaita Sodo University for inspiring the researcher in doing this research work.
Disclosure statement
No potential conflict of interest was reported by the author(s).
Additional information
Funding
Notes on contributors
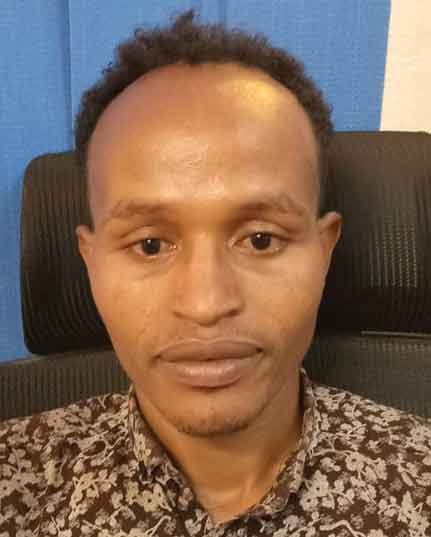
Berhanu Daniso
Berhanu Daniso was born on October 1993 in Mirab Badawacho Woreda, Hadiya Zone, Southern Nations, Nationalities, and Peoples' Region of Ethiopia. He joined Jimma University in 2012 and graduated with Bachelor of Science Degree in Rural Development and Agricultural Extension in July 2014. After his graduation, he was employed at Mirab Badawacho Woreda Agricultural Development Office at Extension agent and coordinator position in 2015 and served for 2 years until he began postgraduate study at Jimma University. He graduated with his M.Sc. degree in Agricultural Communication and Innovation in 2018. He has been employed at lecturing position at Wolaita Sodo Agricultural Technical Vocational Education and Training Colleges for 8 months. Currently, he is a lecturer at Wolaita Sodo University. Development, rural innovation, gender, climate change, communication for innovation and sustainable livelihood analysis are the author’s interest areas of research.
References
- Adio, E. O., Abu, Y., Yusuf, S. K., & Nansoh, S. (2016). Use of agricultural information sources and services by farmers for improve productivity in Kwara State. Library Philosophy and Practice (e-journal), 1456, 1–10. http://digitalcommons.unl.edu/libphilprac/1456
- Ahmad, D., Chani, M. I., & Afzal, M. (2018). Impact of formal credit on agricultural output: Empirical evidence from Pakistan. Sarhad Journal of Agriculture, 34(3). https://doi.org/10.17582/journal.sja/2018/34.3.640.648
- Aker, J. C. (2011). Dial “A” for agriculture: A review of information and communication technologies for agricultural extension in developing countries. Agricultural Economics, 42(6), 631–647. https://doi.org/10.1111/j.1574-0862.2011.00545.x
- Andersson, C., Mekonnen, A., & Stage, J. (2011). Impacts of the productive safety net program in Ethiopia on livestock and tree holdings of rural households. Journal of Development Economics, 94(1), 119–126. https://doi.org/10.1016/j.jdeveco.2009.12.002
- Aonngernthayakorn, K., & Pongquan, S. (2017). Determinants of rice farmers’ utilization of agricultural information in Central Thailand. Journal of Agricultural & Food Information, 18(1), 25–43. https://doi.org/10.1080/10496505.2016.1247001
- Asenso-Okyere, K., & Mekonnen, D. A. (2012). The importance of ICTs in the provision of information for improving agricultural productivity and rural incomes in Africa. African Human Development Report. UNDP Sponsored research Series.
- Baumuller, H. (2013). Mobile technology trends and their potential for agricultural development (ZEF Working Paper 123).
- Daniso, B., Muche, M., Fikadu, B., Melaku, E., Lemma, T., & Yildiz, F. (2020). Assessment of rural households’ mobile phone usage status for rural innovation services in Gomma Woreda, Southwest Ethiopia. Cogent Food & Agriculture, 6(1), 1728083. https://doi.org/10.1080/23311932.2020.1728083
- Eakin, H., Muñoz-Erickson, T. A., & Lemos, M. C. (2018). Critical lines of action for vulnerability and resilience research and practice: Lessons from the 2017 hurricane season. Journal of Extreme Events, 5(2n03), 1850015. https://doi.org/10.1142/S234573761850015X
- Gido, E. O., Sibiko, K. W., Ayuya, O. I., & Mwangi, J. K. (2015). Demand for agricultural extension services among small-scale maize farmers: Micro-level evidence from Kenya. The Journal of Agricultural Education and Extension, 21(2), 177–192. https://doi.org/10.1080/1389224X.2013.872045
- Gombe, S., Suandi, T., Ismail, I. A., & Omar, Z. (2015). Extension worker competencies needed for effective management of self-help groups (SHG) in Gombe State. International Journal of Education and Training, 1(1), 1–7.
- Hasan, M. F., Imai, K. S., & Sato, T. (2013). Impacts of agricultural extension on crop productivity, poverty and vulnerability: Evidence from Uganda. RIEB Discussion Papers, Kobe University.
- Hussain, A., & Taqi, M. (2014). Impact of agricultural credit on agricultural productivity in Pakistan: An empirical analysis. International Journal of Advanced Research in Management and Social Sciences, 3(4), 125–139.
- Jayaratne, K. S. U. (2014). Identification of current proficiency level of Extension competencies and the competencies needed for Extension agents to be successful in the 21st century. Journal of Human Sciences and Extension, 2(1), 5. https://scholarsjunction.msstate.edu/jhse/
- Katengeza, S. (2012). ICT-based market information services, operational environment and performance: The case of Malawi agricultural commodity exchange and food and nutrition security joint task force. http://www.ndr.mw:8080/xmlui/handle/123456789/1249
- Kumar, S. S., & Chander, M. (2011). Perceived information needs of dairy farmers in accessing ICT-enabled village information centres. Journal of Dairying, Foods & Home Sciences, 30(4), 239–241.
- Lee, K. H., & Bellemare, M. F. (2013). Look who’s talking: The impacts of the intrahousehold allocation of mobile phones on agricultural prices. The Journal of Development Studies, 49(5), 624–640. https://doi.org/10.1080/00220388.2012.740014
- Mittal, S., Gandhi, S., & Tripathi, G. (2010). Socio-economic impact of mobile phones on Indian agriculture (No. 246). Working paper.
- Mittal, S., & Mehar, M. (2016). Socio-economic factors affecting adoption of modern information and communication technology by farmers in India: Analysis using multivariate probit model. The Journal of Agricultural Education and Extension, 22(2), 199–212. https://doi.org/10.1080/1389224X.2014.997255
- Mtega, W. P. (2012). Access to and usage of information among rural communities: A case study of Kilosa District Morogoro Region in Tanzania. Partnership: The Canadian Journal of Library and Information Practice and Research, 7(1). https://doi.org/10.21083/partnership.v7i1.1646
- Naruka, P. S., Verma, S., Sarangdevot, S. S., Pachauri, C. P., Kerketta, S., & Singh, J. (2017). A study on role of WhatsApp in agriculture value chains. Asian Journal of Agricultural Extension, Economics & Sociology, 20(1), 1–11. https://doi.org/10.9734/AJAEES/2017/36498
- Naveed, M. A., & Anwar, M. A. (2013). Agricultural information needs of Pakistani farmers. Malaysian Journal of Library & Information Science, 18(3), 13–23.
- Olorunfemi, T. O., Olorunfemi, O. D., & Oladele, O. I. (2020). Determinants of the involvement of extension agents in disseminating climate smart agricultural initiatives: Implication for scaling up. Journal of the Saudi Society of Agricultural Sciences, 19(4), 285–292. https://doi.org/10.1016/j.jssas.2019.03.003
- Ommani, A. R., & Chizari, M. (2008). Information dissemination system (IDS) based E-learning in agricultural of Iran (perception of Iranian extension agents). International Journal of Human and Social Sciences, 2(3), 129–133.
- Pezeshki-Rad, G., & Zamani, N. (2005). Information-seeking behaviour of Iranian extension managers and specialists. Information Research: An International Electronic Journal, 10(3), n3.
- Prokopy, L. S., Carlton, J. S., Arbuckle, J. G., Haigh, T., Lemos, M. C., Mase, A. S., Power, R., Dunn, M., Andresen, J., Angel, J., Hart, C., & Power, R. (2015). Extension′ s role in disseminating information about climate change to agricultural stakeholders in the United States. Climatic Change, 130(2), 261–272. https://doi.org/10.1007/s10584-015-1339-9
- Ragasa, C., Ulimwengu, J., Randriamamonjy, J., & Badibanga, T. (2016). Factors affecting performance of agricultural extension: Evidence from Democratic Republic of Congo. The Journal of Agricultural Education and Extension, 22(2), 113–143. https://doi.org/10.1080/1389224X.2015.1026363
- Soomro, K. A., Kale, U., Curtis, R., Akcaoglu, M., & Bernstein, M. (2018). Development of an instrument to measure Faculty’s information and communication technology access (FICTA). Education and Information Technologies, 23(1), 253–269. https://doi.org/10.1007/s10639-017-9599-9
- Tadesse, G., & Bahiigwa, G. (2015). Mobile phones and farmers’ marketing decisions in Ethiopia. World Development, 68, 296–307. https://doi.org/10.1016/j.worlddev.2014.12.010
- Tadesse, M., Kifle, M., & Ndemo, E. (2017). Factors affecting the use of mobile phone by smallholder farmers in vegetable marketing: the case of Fogera district of South Gondar Zone, Amhara Regional State, Ethiopia (Doctoral dissertation, Haramaya university).
- Tegegne, A. K., & Alemu, T. A. (2019). SMS-based agricultural information system for rural farmers in Ethiopia. Journal of Usability Studies, 15(1).
- Xu, G., Sarkar, A., & Qian, L. (2021). Does organizational participation affect farmers’ behavior in adopting the joint mechanism of pest and disease control? A study of Meixian County, Shaanxi Province. Pest Management Science, 77(3), 1428–1443. https://doi.org/10.1002/ps.6161
- Yamane, T. (1967). Statistics: An introductory analysis. Harper and Row.
- Yaseen, M., Xu, S., Yu, W., & Hassan, S. (2016). Farmers’ access to agricultural information sources: Evidences from rural Pakistan. Journal of Agricultural Chemistry and Environment, 5(1), 12–19. https://doi.org/10.4236/jacen.2016.51B003
- Zikhali, Z. M., 2016. Meeting the extension needs of smallholder farmers: the climate information gap in the public agricultural extension and advisory services in Limpopo, South Africa ( Doctoral dissertation).