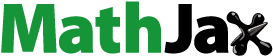
Abstract
Maize production and its yield gap of 4.3 t/ha remains a problem in Ghana in the face of increasing weather variability which further threatens yield on farmer fields. Exploring the Drought Tolerant Maize awareness and adoption rates, its determinants and potential adoption is necessary for the adoption of climate smart farming technologies. The Partial Population Exposure Model was applied to the cross-sectional data for the estimation of the rates and determinants. Awareness was determined by gender, maize farm size, number of maize farms, FBO membership and access to extension service, whereas adoption was determined by gender, maize farm size, education, farming experience, FBO membership, household size and access to extension services. Awareness rate was 69% and 86%, adoption rate was 22% and 76% and potential adoption rate was 31% and 76% in Guinea Savanna and Forest-Savanna Transition Zones, respectively. Population selection-biased estimated were statistically insignificant, indicating that the difference observed were due to lack of information on Drought Tolerant Maize. Improved agricultural technologies development and dissemination should be gender responsive, and Research and Extension services should educate farmers on the best production practices in relation to drought through periodic trainings.
1. Introduction
Maize is the largest food crop in Ghana and contributes significantly to consumer diets (Fening et al., Citation2011). Accounting for about 60% of overall cereal production, it is also the number one crop considering planted area covered (Aidoo et al., Citation2014). Maize demand has been characterized with issues of population rise, urbanization and rising poultry and fisheries subsector activities in Ghana. The threat that climate change presents to most farmers in the sub-Saharan Africa economies has been at the forefront of agriculture-related discussions. This is because agriculture in sub-Saharan Africa is mostly rain-fed and requires a determined effort (Asante and Amuakwa-Mensah, Citation2014). The fact that a number of developing countries are naturally found in the tropics means that they are prone to high temperatures which has negative implications for agricultural production (Da Cunha et al., Citation2015). The situation is made worse by the inadequacy of human and capital resources needed to deal with the negative implications (Di Falco, Citation2018). In Ghana, however, the Guinea and Sudan savanna zones have the highest potential for increasing the production and productivity of maize due to high levels of solar radiation, low temperatures at night and low occurrence of diseases. Farmers are faced with harsh realities of drought with low yields. This study aimed at establishing the level of Drought Tolerant Maize (DTM) awareness and adoption and also identifying factors that drive the awareness and adoption of DTM in the Guinea Savanna and Forest Transition Zone.
The study, however, had the following limitation;
Some farmer’s inability to provide information on some of the varieties they grow led to loss of valuable information.
The use of cross sectional data invariable did not provide the opportunity to look at trends over time.
The inability to conduct a gender responsive study to outline how DTM adoption influences men and women.
2. Literature review
Any agricultural technology that has the potential of increasing agricultural output is described as an improved technology. This is to say, it has the potential of improving on the current gains farmers make out of the existing technology. They may include seeds that guarantee high yields, chemical for nutrient enhancement (fertilizer), for weed management (herbicides/weedicides) and for insect/pest control (pesticides/insecticides), as well as machinery for farm work among others. Jain et al. (Citation2009) emphasized that based on the principle of improved inputs leading to improved outputs, improved techniques lead to increased output hence increased income.
Technology variations have been responsible for some of the major changes experienced in agriculture in recent decades, especially in developing countries. Technology adoption, however, does not occur except there is diffusion of the technology which is also referred to as awareness creation. It is worth noting that awareness and adoption are never considered to be mutually exclusive but as two concepts that work together to ensure the uptake of a technology. Awareness is a pre-requisite for adoption to occur. It is therefore all the activities that ensures that the information about an innovation reaches the target group (Diagne & Demont, Citation2007). Adoption simply may be defined as the decision and actual use of an improved technology (Diagne & Demont, Citation2007). According to Rogers et al. (Citation1983), technology awareness creation must involve a new technology, a channel for drawing attention to the technology, a time period in which it must be done and must have a social interest orientation. Feder et al. (Citation1985) defined adoption as the degree to which a new technology is used by a farmer with the aim of increasing output. Feder and Umali (Citation1993) emphasized technology as the use of a perceived or precise factor with the aim of altering the production function for a desired improved output. In another vain, Feder et al. (1985) said adoption was the extent to which a new improved technology of which a farmer has full knowledge is used in the long run. The most important issue here is the fact that the farmer has all the information that comes with the new technology including quantity required per unit area, best production practices, processing and marketing practices and the potential gains of its usage. It is obvious from all the above studies that adoption of improved agricultural technology aims at improved outputs and require a near perfect information dissemination approach to be able to achieve that. Ensuring that agricultural output increases is necessary for achieving food security in Sub Saharan Africa and this can be more effective with the introduction and usage of improved agricultural technologies (Maertens and Barrett, Citation2013). The smallholder farmer’s decision which is geared towards profit maximization can be greatly influenced by improved technology adoption which has the tendency to deepen the market component that is obtained from agricultural output of the smallholder farmer. Omiti et al. (Citation2009) advised for a concentration of farmers on the purchase of required farm inputs than the over reliance of farmers on farmer saved seeds and familiarized technologies, especially for the developing countries. Hence, a reduction in the marketing and processing related cost and reduction in the real price of food. Mishra et al. (Citation2015) emphasized the importance of technology adoption in improving efficiency in agriculture and also ensuring the reduction in food insecurity. However, they emphasized that the level of adoption in sub Saharan Africa was pacing at a very low rate, and this rate was worst in women. There is no single combination of factors that are required for technology adoption. Adoption can be influenced by many factors either singularly or collectively in clusters as may be driven by the socio-demographic characteristics of the respondents among other factors. These combined factors may be technological, economical, institutional or human.
Loevinsohn et al. (Citation2013) stated that the decision a farmer makes to adopt a new technology or not, depends on the nature of the technology itself and the surrounding situations or state of affairs. This is to say that the prevailing conditions of the farmer has an interplay with the nature of the variety itself for adoption to take place or not. Uaiene et al. (Citation2009) stated that adoption studies over the last decade had undertaken economic enquiries hinged on personal traits, inefficiencies in accessing information, risk, constraints, inputs and infrastructure availability among others, with a recent inclusion of social networks and imparting information by grouping respondents by common characteristics. These factors have been classified into various groups by different authors. Akudugu et al. (Citation2012) made a classification of economic, social and institutional determinants or factors. In a like manner, Lavison (Citation2013) cited Kebede et al. (Citation1990) saying that the determinants of adoption could be categorized into social, economic and physical factors. As much as there seems to be a consensus on the categories of these determinants, there is sharp restrictions on what constitutes each category or which factor fall under each category rigidly (Mwangi & Kariuki, Citation2015). Doss (Citation2003) touched on two important determinants of adoption of technology, namely, the characteristics of the technology and the ability of farmers to try the technology before adopting. Wandji et al. (Citation2012) in a study of farmer perceptions in relation to aquaculture technology adaptation in Cameroun concluded that farmer perceptions influenced technology adoption. Mwangi and Kariuki (Citation2015) in an in-depth study of the determinants of adoption of improved agricultural technology in developing countries emphasized that improved agricultural technologies could be an important avenue for poverty reduction in developing countries. They concluded that among a number of institutional, technological, economical and human factors, there was the need to add the perceptions farmers have about improved technology to the factors that are considered. This study explores the agro-ecological difference in relation to DTM awareness and adoption which is quite limited. It also employs the Partial Population Exposure (Diagne & Demont, Citation2007) which treats awareness as a pre-requisite for adoption instead of just a being treated as a determinant of adoption. This makes room for the determination of the factors which influence adoption; an information that would be useful for technology development and dissemination.
3. Materials and methods
3.1. Study area
This study was conducted in two agro-ecological zones, namely, Guinea Savanna (GS) and Forest/Savanna Transition (FST) as they are the most suitable for maize production across the country. Second, they were areas where major DTMA dissemination activities were undertaken. The Guinea and Forest-Savanna Transition zones of Ghana are predominantly agricultural oriented, with much focus on crop production. There are differences in the climate, soil and natural vegetation between the two agro-ecologies. The average annual rainfall is 1100 mm and 1300 mm for Guinea savanna and Forest-Savanna Transition, respectively (MoFA, Citation2018). The GS has a mono-modal rainfall regime, whereas the FST has a bi-modal rainfall regime, and these reflects in the crops they can produce and the yields they can obtain. The study was conducted in six district Nanton, Karaga, Central Gonja, North East Gonja (Guinea Savanna), Techiman North and Kintampo North (Forest Transition).
3.2. Sampling
The total sample size of 600 respondents was determined by the sample size determination table by Krejcie and Morgan (Citation1970). According to the Ministry of Food and Agriculture, the population of farm households in Northern, Savanna and Bono East Regions are 524,357, 481,360 and 352,368; hence, total of about 1,358,104 across the three regions. Considering that the MoFA estimates the 70% of these farm population are maize farmers, it translates in 950,672 maize farm households. According to sample table adopted, this population requires a sample size of 384 respondents. For a better predictive power, the sample size was increased by about 56%. After the data collection, 10 outliers were recorded and dropped; hence, results presented in this study covers about 590 respondents. Maize farmer households were targeted for this study and the time of interest is the major season in the year 2020. It is worth noting that due to changes in the rainfall pattern, this year saw one rainfall regime across the country instead of the two season down south and the one season up north. This study used the multi-stage sampling technique for selecting the sample size. Three regions (Northern, Savanna and Bono East) and six districts (Nanton, Karaga, Central Gonja, North East Gonja, Techiman North and Kintampo North) were then purposively selected based on their involvement in DTMA dissemination activities. That is to say they were regions and districts involved in farm trials and demonstration of Drought Tolerant Maize. Five communities were then purposively selected from each of the district based on their involvement in DTM dissemination activities. The study focuses on the smallholder farmer with landholdings of about five acres (two hectares); this is because beyond this threshold farmers. From purposive list of maize farmers with landholdings five acres and below in each of the communities, 20 respondents were selected using a systematic random sampling approach. In summary, 2 acro-ecologies, 3 regions, 2 districts per region, 5 communities per district and 20 respondents per community, resulting in a total sample size of 600 respondents. Quantitative data was collected using a well-structured questionnaire that will be individually administered.
4. Analytical framework
4.1. Awareness and adoption of drought Tolerant Maize (DTM)
The awareness status of the DTM for the ith farmer in the population is defined by , which has value 1 (or 0) if the farmer is (or is not) not aware of the DTM. The total number of farmers who are aware is denoted by
, and N represents the total number of farmers. Accordingly, the awareness rate (
) for the DTM is expressed as the percentage proportion of farmers who are aware and it is given by:
where represents the total number of farmers in the population who are aware of DTM.
The cultivation of a DTM by the farmer is termed as Adoption. A farmer is said to adopt if he/she cultivates at least one of the DTM varieties identified. The adoption rate () is consequently expressed as the percentage proportion of farmers who are cultivating DTM and it is given by:
where represents the number of farmers in the population who adopted a DTM.
In this study, the Average Treatment Effect (ATE) estimation procedure proposed by Diagne & Demont (Citation2007) will be used in obtaining the estimates of the determinants of population adoption rates of DTM varieties. In view of the fact that farmers may not be aware of the many DTM varieties released, this approach becomes relevant for this study. In addition to that, preceding adoption studies on the adoption subject matter have largely been on the assumption that awareness of any technology at a given time is absolutely total within the study population. The results of such estimates of the population adoption parameters are inconsistent (Geroski, Citation2000; Kabunga et al., Citation2012), and they reflect biases in nonexposure and selection (Diagne & Demont, Citation2007).
ATE parameter reflects the true population adoption rate and measures the impact of a “treatment” on a randomly selected person from the population treatment (Murphy et al., Citation2001; Wooldridge, Citation2002). In this study, the treatment will be the farmers’ awareness of the DTM varieties. Farmers who are aware will be referred to as “treated” and unaware farmers will be referred to as “untreated”. In sum, the ATE is simply the mean population adoption outcome of awareness. Stated differently, it is the mean population adoption outcome when all members of the population have knowledge of the DTN varieties (Simtowe et al., Citation2012). The ATE measures the potential demand of the varieties by the farmers under complete awareness. The gap between the population mean potential adoption and the population actual (observed) adoption measures the adoption gap, which exists because the knowledge is not completely diffused within the population (Diagne & Demont, Citation2007; Simtowe et al., Citation2012).
The average adoption rate in the subpopulation of treated (exposed) farmers is referred to as the Average Treatment Effect on the treated (ATT), commonly denoted by ATE1. The mean difference between the population adoption rate (ATE) within the exposed subpopulation (ATE1) is referred to as the Population Selection Bias (PSB; Diagne & Demont, Citation2007; Wooldridge, Citation2002).
According to the specification of Rosenbaum and Rubin (Citation1985), Simtowe et al. (Citation2012), and Wooldridge (Citation2002), let y1 be the potential adoption outcome of a farmer who is aware of DTM varieties and y0 be the potential adoption outcome when not exposed to them. The “treatment effect” for the ith farmer is the difference y1i—y0i. Subsequently, the expected population adoption impact of exposure to the new varieties is given by the mean value E(y1—y0). Diagne and Demont (Citation2007) emphasized that, the exposure to a new variety is a necessary condition for its adoption. By implication, farmers who are not exposed cannot adopt (y0 = 0). The adoption impact of the ith farmer therefore is given by y1i and the average adoption impact is given by ATE = Ey1.
To examine the impact of exposure on adoption of the DTM varieties, lets w be a binary variable that denotes awareness of the drought tolerant maize varieties where w = 1 if a farmer is aware of one or more DTM variety and w = 0, if otherwise. The rates of adoption and the factors contributing to adoption are estimated conditional on the observed covariates ((yi,wi,xi,zi), i = 1, … ., n) of a randomly selected farmer from the population, where xi is the vector of covariates determining potential adoption rates (y1) and zi is the vector of covariates that determine awareness (w1), with the possibility of xi and zi having some common factors.
The ATE methodology provides consistent estimates of true population adoption parameters by conditioning for observed awareness status. For this study, ATE will be obtained using methods that depend on the validity of the conditional independence assumptions (Diagne, Citation2012; Wooldridge, Citation2002), which states that the awareness of the improved varieties, , is independent of the potential outcomes,
(adopt) and
(not adopt), conditional on the observed set of covariates,
, that determine awareness of the improved varieties,
. This can be expressed as
.
The ATE parameters can be estimated from observed random vectors (yi, awi,xi,zi) i=1,.,n through either a pure parametric regression method in which covariates will interact with treatment status variable (heterogeneous impact) or they will be based on a two-stage estimation procedure where the conditional probability of treatment , called the propensity score, will be determined in stage one; then ATE, ATE1 and ATE0 will be determined stage two by parametric or non-parametric approaches (Diagne & Demont, Citation2007).
In addition to the conditional independence assumption, potential adoption is assumed to be independent from z, conditional on . Thus, the rate of adoption and its underlying factors can be estimated from the subsample who are aware, if the conditional independence assumption holds and that of the potential adoption is independent of vectors of determinants of awareness conditional on the vector of adoption determinants. Then the ATE (x) can be non-parametrically identified from the joint distribution of (y, z) condition on w = 1 by:
This can be consistently estimated from a random sample of yi,xi = 1, …,n drawn from only the subpopulation who are aware. The parametric estimation procedure of ATE is based on the following equation that identifies ATE(x) and which holds under the conditional independence (CI) assumption (Diagne & Demont, Citation2007):
The parametric estimation proceeds by first specifying a parametric model for the conditional expectation on the right hand side of the second equality of EquationEquation (2)(2)
(2) which involves the observed variables y, x and aw:
where g is a known (possibly nonlinear) function of the vector of covariates x and the unknown parameter vector β which is to be estimated using MLE procedures and the observations (yi,xi) from the subsample of exposed farmers only with y as the dependent variable and x the vector of explanatory variables. With an estimated parameter βˆ, the predicted values g(xi,βˆ) are computed for all the observations i in the overall sample and ATE, ATE1 and ATE0 are obtained by taking the averages of the predicted , i = 1,.,n across the full sample (for ATE) and respective subsamples (for ATE1 and ATE0):
The effects of the determinants of adoption as measured by the K marginal effects of the K-dimensional vector of covariates x at a given point x are estimated as:
where is the k-th component of
.
The ATE, ATE1, ATE0, the population adoption gap , and the population selection bias
parameters are estimated using the parametric regression-based estimators (EquationEquations 4
(4)
(4) –Equation6
(6)
(6) ).
It is essential to estimate the determinants of awareness because it provides information on the factors that are likely to influence a farmer become aware of a new technology. However, these factors could either be the same or different from the determinants of adoption.
In estimating the parametric regression based estimators, since y is a binary variable, EquationEquation 3(3)
(3) above is effectively a parametric probabilistic model; hence,
are estimated using a probit model,
. In this case, the parametric estimation of ATE reduces to a standard probit estimation restricted to the exposed sub-sample. The marginal effects in Equationequation (7)
(7)
(7) are also estimated using this ATE parametric model. Following and applying Stata add-on adoption commands, developed by Diagne and Demont (Citation2007), this paper employed appropriate estimators to consistently obtain the ATE, ATE1, ATE0, the population adoption gap, and the population selection bias.
Two empirical models of interest in relation to awareness and adoption are therefore stated as follows:
The awareness model
Following EquationEquation (1)(1)
(1) , the model for the determinants of exposure to DTM varieties (
) is explicitly expressed as:
where is the binary response variables denoting awareness;
is the standard normal cumulative density function;
is a set of covariates that represent the characteristics of the sampled households and their respective socioeconomic conditions;
represents farm-level factors;
denotes the set of institutional covariates.
The adoption model
Given that a DTM adopter refers to a farmer who cultivates DTM varieties, following Equationequation (3)(3)
(3) , the observed adoption incidence rate,
, the probit model for the determinants of adoption of the DTM varieties (
) can be explicitly expressed as:
5. Results and discussions
5.1. Summary statistics for adopters and non-adopters of drought Tolerant Maize
Table represents the summary statistics of the variables for the sampled households involved in the study.
Table 1. Summary statistics for adopters and non-adopters of drought tolerant maize production
Across location, about 82% of respondent were men with 79% and 84% of respondents being men for adopters and non-adopters, respectively, as shown in Table . From Table , the age of household heads averaged about 43 years for all respondents with adopters recording 43% and non-adopters, 43%. Across location, each farmer had approximately one farm and the mean size of the farms across location was 3.7 acres but 3.52 acres for adopters and 3.75 acres for non-adopters as indicated in Table . Average total farm size across the study location was 5.48 acres for adopters it was 6.7 acres and for non-adopters, 4.32 acres. For land tenure, across location, family lands (56%) were mostly used for cultivation. It is worth noting that 67% of non-adopters used family lands as against 36% of adopters. Years spent in formal education was used as proxy for determining the education level of the respondents, and Table shows that on average farmers had about 3.7 years of education. This fall within the range of basic level in the structure of the Ghana Education Service. So it can be concluded that on the average farmers had basic education. Though adopters had more years (6.14) than the non-adopters (4.59), the averages all fall within the basic education level. For the residential status of respondents, 73% and 82% of adopters and non-adopter, respectively, were natives and this yielded an average of 82% across the study location. Number of people in each household was captured as household size and the highest average households was observed in non-adopters (11 persons) with an average of 10 persons across location and about 8 persons for adopters. Across location, most respondents were married (91%) and they had about 16 years of farming experience across location. About 6% of these farmers belonged to a Farmer Based Organization across the study area, the highest being 16% for adopters. Access to Extension showed that 44% of adopters had access compared to 12% of non-adopters as indicated in Table . Income from Livestock production of the respondents, as indicated in Table , was ¢1362.17 across location with a difference of ¢63.88 between adopters and non-adopters in favour of non-adopters.
5.2. Definition of variables and expectations
Expect signs in logit models is a way of predicting the influence that focus variables have on the dependent variable. For this study, the emphasis is on the influence these household, farm level and institutional factors have on the awareness and adoption of Drought Tolerant Maize (DTM), and they are present in Table and explain in this section. Gender which is a dummy variable is expected to have positive effect on awareness and adoption. Men are expected to have access to improved technologies and are more likely to adopt than women (J. A. Akumbole et al., Citation2018; J. Akumbole et al., Citation2019; Hassan, Citation2018). Age is a continuous variable which could positively or negatively affect awareness and adoption of DTM. Adult farmers may have gathered so much perfected some farming practices and may not be willing to change them for new ideas. On the other hand, the young farmer who is ready to explore and more daring is likely to accept new ideas to verify the outcomes thereof. Farming Experience is also expected to have a positive effect on awareness and adoption (J. A. Akumbole et al., Citation2018; J. Akumbole et al., Citation2019; Fisher & Kandiwa, Citation2014; Hassan, Citation2018). Number of farms, Size of the Maize Farm and Total Farm Size is expected to have a positive effect on awareness and adoption. It is expected that a farmer with many farms and large farm size is more inclined to being exposed and adopting a DTM (J. A. Akumbole et al., Citation2018; J. Akumbole et al., Citation2019; Ayinde, Citation2017; Baiyegunhi et al., Citation2022; Danso-Abbeam et al., Citation2017; Kimathi et al., Citation2021). Education is expected to have a positive or negative relationship with awareness and adoption. This is to say that when a farmer has longer years in education, they are more likely to be exposed and adopt as compared to farmers who have less or no years of education (Abdi et al., Citation2018; Ajewole et al., Citation2021; J. Akumbole et al., Citation2019; Ayedun, Citation2018). Household size is expect to have positive and negative effect on awareness and adoption based on the element of family labour for farming (Abdi et al., Citation2018; J. Akumbole et al., Citation2019; Ayedun, Citation2018; Ayinde, Citation2017). Residential Status is expected to have a positive relation on awareness and adoption because it makes the farmer well known and visible for exposure and adoption (Amengor et al., Citation2017). Marital Status, Farming Experience, Membership of Farmer Based Organization and Access to Agricultural Extension Services are expected to have positive effects on awareness and adoption (J. Akumbole et al., Citation2019; Aura, Citation2016; Ayedun, Citation2018; Ayinde, Citation2017; Bello et al., Citation2021; Chandio & Jiang, Citation2018).
Table 2. Definition of variables and expected signs
5.3. Diagnostic tests for normality, heteroskedasticity and multicolinearity
The chi square value for the JB test is 2.70 and it is not significant; hence, the null hypothesis of normal distribution is not reject. This means the residuals from the regression results are normally distributed. Testing for the presence of heteroskedasticity, the white test indicated a chi square value of 43.00 and it was not significant; hence, the null hypothesis of the absence of heteroskedasticity is not rejected. It means there is no heteroskedasticity. The test for multicollinearity using the Variance Factor Inflator (VIF) indicated a mean VIF of 1.58, which is an indication of a very mild and negligible level of multicollinearity considering that 1 represent the absence of multicollinearity and 5 represent the ceiling for the acceptable range.
5.4. Determinants of awareness of drought Tolerant Maize
Table presents results of the global and agro-ecological determinants of awareness of DTM. The chi Square values in GS (35.74), FST (27.96) and Pooled (59.11) are statistically significant at 1% error level. By implication, the logit model is of good fit and the estimates obtained therefore are statistically significant and can be relied on. It was observed that gender, maize farm size, number of maize farms, FBO membership and access to extension service were statistically significant in determining the exposure of a farmer to DTM varieties in the study area. Gender is statistically significant in the GS and FST has a negative relation with awareness. This implies that a man is less likely to be exposed to DTM than a woman. That is to say being a man makes it about 13.3% and 10.1% less likely for exposure to DTM in the GS and FST, respectively. This is because, in as much as men may be the ones extension agents interact with when it comes to issues of farm technologies, women are able to source for information on trending issues than the men. They in most cases receive these kind of information but however lack the capacity to execute them mostly because of the cultural norms that makes them subjective to their husbands. This was contrary to the conclusions of Gebre et al. (Citation2021) in studying the gender differences in adoption of agricultural technologies which emphasized the inclination of exposure of improved technology towards men. Similarly, Voss et al. (Citation2021) in a study of gender inclusivity through main breeding in Africa concluded a positive relationship between gender and exposure to improved agricultural technologies. Maize Farm Size had a positive relationship with getting exposed to DTM varieties in the GS and FST, and it was statistically significant. This is to say there is a direct relationship between the size of the maize farm and getting exposed to DTM varieties. A unit increase in the farm size will increase the likelihood of getting exposed by 5.1% and 3.4% in the GS and FST, respectively. It goes without word that large size farmers are more likely to be exposed than small sized farmers. Kabunga et al. (Citation2012) and Baiyegunhi et al. (Citation2022) recorded same positive and statistically significant relationship between awareness and farm size and concluded that as a proxy for wealth, farmers with bigger land sizes were more likely to be exposed to improved varieties because they could afford the cost of technology. On the contrary, Kimathi et al. (Citation2021) found a negative relationship while studying the adoption of climate resilient potato varieties under partial population exposure and concluded that large maize farmers were more likely to put their farms to other productive use hence are not most concerned about new technologies. Number of maize farms was statistically significant with a negative effect on awareness of DTM in the GS. An increase in the number of maize farms by a unit was likely to decrease the likelihood of awareness by 28%. Farmers with smaller number of plots who are more eager to maximize them are more likely to go looking for improved methods of production. Amengor et al. (Citation2017) found results that contradicted this position an in accordance with the expectation of the relation of number of farms to exposure. Amengor et al. (Citation2017) concluded that there was positive relation between number of farms and awareness of improved varieties in a sweet potato adoption study in Ghana. Membership of a Farmer Based Organization had a positive relation with exposure and it was statistically significant. It implies that by belong to the FBO, the farmer is better placed to be exposed to DTM; hence, membership of FBO is likely to increase exposure by 14.8%. That is to say farmers are able to access information on improved method of production from more experienced farmers. S. Kumar et al. (Citation2021) concluded that FBO membership raises the likelihood of a farmer getting information of an improved technology and in this case DTM.
Table 3. Logit estimates of determinants of drought Tolerant Maize awareness by farmers
From the pooled data, access to Extension services also had a positive relation with awareness of DTM in the study area and it was statistically significant. Having access to extension services will lead to 15.8% likelihood for an increase in the exposure of the farmer to DTM. By implication having contact with extension agents and receiving information and giving feedback has the tendency of increasing the chances of the farmer in getting exposed to DTM. Ullah et al. (Citation2022), Anang and Asante (Citation2020), and S. Kumar et al. (Citation2021) similarly found a positive relationship between access to extension and awareness while studying diffusion of improved agricultural technologies. Tang et al. (Citation2013) studied estimation of awareness and perception of water scarcity among farmers in China and concluded that having access to extension service means getting the latest information on the best technologies that are at play in the industry. Again from the pooled data, household size was statistically significant and had a negative effect on exposure to DTM. This implies that the smaller the household size, the more likely it was for the household to be exposed and vice versa. A reduction in the household size by a unit was likely to increase exposure by 0.9%. Amengor et al. (Citation2017) found results that contradicted this finding saying that a larger household was more likely to increase the likelihood of exposure. It is worth noting that a smaller household means the likelihood of reaching out to find out about trending technologies is greater because they are well aware if they do not scout they may not find best practices available, hence increasing the probability of exposure to DTM.
5.5. Determinants of adoption of drought Tolerant Maize
Table presents the logit estimates for the determinants of adoption of DTM and this sections discusses these determinants. A chi square value was 179.80, which statistically significant implies the model is of good fit; hence, its estimates can be used for sound and reliable economic analysis. It was observed that Gender, Maize Farm Size, Education, Farming Experience, FBO Membership, Household Size and Access to Extension Services were statistically significant in terms of their influence on the adoption of DTM. Gender had a negative relation, and it was statistically significant in the FST. That is to say that not being women were more likely to adopt DTM more than men. That is to say that being a man the likelihood of adopting DTM is reduced by 14.7% in the FST. This was contrary to the expected sign of gender’s relation to adoption as stated in Table , which was supposed to be positive. Obayelu and Ajayi (Citation2018) found a positive relationship between gender and adoption in the study of adoption of improved technologies. Similarly, Okuthe et al. (Citation2013) studied the determinants of adoption of improved sorghum varieties and also concluded there was a positive relation between the two phenomena in question. In a more emphatic sense, Maguza-Tembo et al. (Citation2017) studied the determinants of climate smart agricultural technology adoption in Malawi and concluded that men headed households were more likely to adopt a technology, hence a positive relationship between gender and adoption. It is worth noting that in each of these instances, gender was a dummy variable which had the value of “1” for men and “0” for women. Similarly, Asante et al. (Citation2021), and Ragasa et al. (Citation2013) found similar results. In as much as these studies to a contrary stance, it is worth noting that the Ghanaian culture perceives women to be easily convinced in accepting new ways of doing things than men. The women are just constraint by access to resources to execute the new ideas they receive; hence, the position of this result is founded. Maize farm size was also found to be statistically significant and had a positive relationship with adoption of DTM in the FST. That is to say that about a unit increase in the farm size is likely to increase the probability of adoption by 8.5%. This is because large farm sizes serves as an avenue for farmers to put a portion of their land under the cultivation of the new variety without having to make much variations in what he is growing already. This provides a clear opportunity for the farmer to match the performance of the new variety against what he is growing already. Bezu et al. (Citation2014), Baiyegunhi et al. (Citation2022), and Danso-Abbeam et al. (Citation2017) made conclusions that are in sync with the result in this study where they found a positive relationship between farm size and adoption of improved agricultural technology. On the contrary, Donkoh et al. (Citation2019) found a statistically significant negative relationship between farm size and adoption which was explained to have been as a result of high cost associated with the adoption of technologies. That is to say improved technologies come with a certain increase in production cost which grows as the farm size increases hence a demotivating factor for adoption. This study however failed to underscore the overall benefit that this extra cost might bring in the form of increased profit from economies of scale. Kassie et al. (Citation2015) in agreement to this negative relation explained that when a farm land is small, there was the possibility of the farmers exploring the best methods available to maximize the proceeds from the land. Education measured in years was statistically significant with a positive relationship with adoption of DTM. That is to say a unit increase in the years of education was likely to increase the chances of adoption by 1.7%. This is because the educated are better able to articulate new technology and are more likely to understand its application than the uneducated. Obayelu and Ajayi (Citation2018) and Lavison (Citation2013) found a positive relationship between education and adoption and concluded that, as farmer’s educational level increases, the farmer’s ability to be receptive, understand and use improved technologies increases. Anang and Asante (Citation2020) concluded in a similar manner saying increasing education enhances the farmer’s ability to make informed decisions that leads to increased productivity. Education therefore has the tendency of improving the quality of human capital and the quality of decisions they make thereof. Mabe et al. (Citation2018) also studied the adoption of improved rice varieties in Volta Region, Ghana, and had similar conclusion just as it was with Ghimire et al. (Citation2015) and Rahman (Citation2021) in the study of improved variety adoptions. Azumah and Adzawla (Citation2017) also emphasized the need for at least education for a farmer to be able to understand the protocols of an improved technology which can be a good facilitator for adoption. Farming experience was statistically significant and had a negative relationship with adoption of DTM in both GS and FST. A unit increase in experience is therefore likely to decrease the probability of adoption by about 0.4%. This is to say as a farmer advances in experience, there is the possibility of the farmer adopting DTM reduces. A. Kumar et al. (Citation2020) concluded that increasing farming experience transcends into lower levels of adoption and this could be because experience farmers tend to trust their own way and methods of farming and finds it very difficult to give them up for new ones. Donkoh et al. (Citation2019) and Azumah et al. (Citation2017) also found a similar relationship between farming experience and adoption while studying adoption of improved technologies and climate smart coping strategies in Ghana, respectively. On the contrary, Obayelu and Ajayi (Citation2018) similarly said, as a farmer’s experience increases, the farmer may have less uncertainty about the technology and can also base on past experience make informed analysis about the improved technology hence increasing the probability of adoption. Danso-Abbeam et al. (Citation2017) agreed with this positive relation while studying adoption of improved and local maize varieties in Ghana. Membership of an FBO had a positive statistically significant relationship with the decision to adopt DTM in FST. Belonging to an FBO, was more likely to increase the decision to adopt a DTM by 18%. This is because the farmer will interact with other farmers to have enough information and motivation to grow DTM. Nkegbe and Shankar (Citation2014) studied the adoption of soil water conservation practices and concluded that there was a positive relationship between FBO membership and the adoption of improved technology. This is largely because belonging to an FBO is an avenue for peer learning, and this makes it possible for the less experienced farmers to tap into the knowledge of the experienced ones. In most cases too, extension services which are the agents of dissemination of improved technologies work mostly with the FBOs than individual farmers because it affords them the opportunity to reach many more people at a time. These interactions can encourage the uptake of new technologies. Danso-Abbeam et al. (Citation2017) and Mmbando and Baiyegunhi (Citation2016) concluded that due to access to good peer counsel, membership of FBO increases the probability of improved technology adoption. On the contrary, Anang and Asante (Citation2020) studied adoption of improved rice varieties and found a negative relationship with FBO membership and adoption. This could be so because some of the members with negative experience could discourage others who might have to good will of adopting. From the pooled results, household size was statistically significant and had a negative relationship with adoption of DTM. That is to say, a unit increase in the household size will lead to about 0.9% reduction in the probability of a farmer adopting DTM. Yimer et al. (Citation2019) in a study on the impact of adoption of improved wheat in Ethiopia deviates from the general expectation of small households being labour constrained hence not likely to adopt a technology. This is to say small households in an attempt to ensure their efforts on their small fields do not go wasted, will be motivated to accept improved technologies that improves their farm output. Similarly,Obayelu and Ajayi (Citation2018) found a negative relationship between household size and adoption. From the pooled results, access to extension services had a positive and statistically significant relation with adoption of DTM. This implies that the more access a farmer had to extension services, the more likely it was for the farmer to adopt DTM. A unit increase in access to extension service is likely to increase adoption by 26.1%. Danso-Abbeam et al. (Citation2017) found a similar relationship and concluded that a lot of farming information, especially with improved technologies are passed on to the farmer by extension agents. Similarly, Nkegbe and Shankar (Citation2014) emphasized this relationship saying that technical information on farm improvement is passed on to the farmer by the extension service. These studies were, however, silent and failed to acknowledge that the willingness of the farmer to apply the information received stands in the gap between reception and adoption. However, it is clear that the interactions between the two parties offers an opportunity that could help influence the farmer’s decision to adopt the said improved technologies. Ayedun (Citation2018) also studied the adoption of DTM in West Africa and concluded a positive relationship driven by the reduction in the time lag between the development and dissemination of improved technologies in agriculture. Farmers are able to receive certified farming information with reduced elements of doubt. Dinar and Yaron (Citation1992) stated that the negative effect of low level of education on adoption could see an offset with a well-established and reliable extension service.
Table 4. Logit estimates of the determinants of drought Tolerant Maize adoption by farmers
5.6. ATE estimation of structural population adoption results
As indicated in Table , adoption rate had the whole population been exposed to DTM was 32%. Farmers who were exposed to DTM had an adoption rate of 35%. The adoption rate for the exposed is higher than in the overall. Considering potential adoption among the unexposed, adoption would have been 25.4% had untreated exposed. It can therefore be simplified that potential adoption among the untreated was 25.4% as stated earlier. Had adoption been specified using the classical approach, the rate would have been 53.3%. By implication adoption rate would have been underestimated because adoption rate would have been 8% lesser. It is worth noting that 68.6% of the population were exposed to DTM. From the agro-ecology perspective as indicated in Table , overall adoption in the GS and FST was 9.7% and 74.6%, respectively. It can be inferred that adoption was very low in the GS and is largely responsible for the overall low adoption obtained across location. Adoption among the treated was 10% and 75% in the GS and FST, respectively. Potential adoption was also 9.1% and 72.9% in GS and FST, respectively. Adoption gap was also 3% and 12.7% for GS and FST, respectively.
Table 5. Adoption rates of drought Tolerant Maize
It is worth noting that, across location and within location, the population selection bias was insignificant. This implies that adoption was indeed influenced by nothing but exposure in this case. The PSB having a positive sign means that should exposure increase adoption was likely to increase.
6. Conclusions and recommendations
In conclusion, Extension Services and Farmer to Farmer were identified as the major carriers of DTM Information. Awareness of DTM was generally high and Adoption of DTM was low in GS but high in FST. Gender, maize farm size, Number of maize farms, FBO membership and access to extension service were the drivers of DTM Awareness whereas Gender, Maize farm size, Education, Farming experience, FBO membership, Household size and Access to Extension Services were the drivers of DTM Adoption. Awareness DTM was high in both GS and FST but adoption was low in the GS and high in the FST. To improve awareness of DTM, focus should be on factors such as gender, maize farm size, number of maize farms, FBO membership and access to extension service and to improve adoption of DTM, focus should be on factors such as gender, maize farm size, education, farming experience, FBO membership, household size and access to extension services.
The following recommendations are therefore made from the findings of this study.
Funding for research must include funding for dissemination activities and commodity chain interaction either via a Multi Stakeholder Platform (MSP) or an Innovation Platform (IP). This will increase awareness and access to the best varieties and technologies for maize production.
Improved agricultural technologies development and dissemination should be gender responsive to help reach a greater number of the target population through approaches that work for men and/or women.
Research and Extension services should educate farmers on the best production practices in relation to drought through periodic trainings. This will increase the knowledge of farmers on climate smart technologies hence an increased desire to adopt them.
DTM varieties should be provided to farmers in drought prone areas through a national drive to guarantee sustainable yields in periods of weather failure through a well-organized agricultural extension system.
Disclosure statement
No potential conflict of interest was reported by the author(s).
Data availability statement
Data available on request due to privacy/ethical restrictions.
https://drive.google.com/drive/folders/1ihonWWemKyFGt15NMpcUDtIhh2w5BSQq?usp=share_link
Additional information
Funding
Notes on contributors
Natson Eyram Amengor
Natson Eyram Amengor (MA Economics, Kwame Nkrumah University of Science and Technology, Kumasi-Ghana) is a Senior Research Scientist with CSIR-Crops Research Institute with interest in Economic Impact assessment, Agricultural Innovation Platform (IP) facilitation, Gender diversity, Food security, Climate change, Commodity value chains and Marketing systems. He has published articles and reports in the field of interest and engagement. He is a gender fellow under the Gender Responsive Researcher Equipped for Agricultural Transformation (GREAT) programme. He is currently a PhD Candidate at the Kwame Nkrumah University of Science and Technology, Kumasi and the Scientific Partner for Von Thunen Institute of Farm Economics in Braunschweig-Germany.
References
- Abdi, A., Faraji, A., Dehghan, F., & Khatony, A. (2018). Prevalence of self-medication practice among health sciences students in Kermanshah, Iran. BMC Pharmacology and Toxicology, 19(1), 36. https://doi.org/10.1186/s40360-018-0231-4
- Adoption of Agricultural Innovations in Developing Countries: A Survey Gershon Feder ([email protected]), Richard Just and David Zilberman ([email protected]. (1985). Economic development and cultural change, 33(2). 255–19.
- Aidoo, R., Mensah, J. O. B., Omono, F., & Abankwah, V. (2014). Factors determining the use of certified maize seeds by farmers in Ejura-Sekyedumasi Municipality in Ghana. World Journal of Agricultural Sciences, 2(5), 084–090.
- Ajewole, O. O., Ayinde, O. E., Tahirou, A., Miranda, M. J., & Olaoye, I. J. (2021). Adoption of stress Tolerant Maize varieties. Does Gender Matter?.
- Akudugu, M. A., Guo, E., & Dadzie, S. K. (2012). Adoption of modern agricultural production technologies by farm households in Ghana: What factors influence their decisions. International Institute for Science, Technology and Education, 2(3), 1–14.
- Akumbole, J., Adam, H., & Zakaria, H. (2019). Effects of adoption of improved maize technology on yield among smallholder maize farmers in the Bawku West District of Upper East Region of Ghana. International Journal of Agricultural Science, Research and Technology in Extension and Education Systems, 9(1), 19–34. https://ijasrt.shoushtar.iau.ir/article_665305.html
- Akumbole, J. A., Zakaria, H., & Adam, H. (2018). Determinants of adoption of improved maize technology among smallholder maize farmers in the Bawku West District of the Upper East Region of Ghana. development, 3, 6. https://doi.org/10.22377/aextj.v2i3.106
- Amengor, N., Owusu-Asante, B., Adofo, K., Acheampong, P., Nsiah-Frimpong, B., Nimo-Wiredu, A., and Baafi, E. (2017). Adoption of improved sweetpotato varieties in Ghana. https://doi.org/10.9734/AJAEES/2018/39874
- Anang, B. T., & Asante, B. O. (2020). Farm household access to agricultural services in northern Ghana. Heliyon, 6(11), e05517. https://doi.org/10.1016/j.heliyon.2020.e05517
- Asante, F. A., & Amuakwa-Mensah, F. (2014). Climate change and variability in Ghana: Stocktaking. Climate, 3(1), 78–101. https://doi.org/10.3390/cli3010078
- Asante, B. O., Koomson, I., Villano, R. A., & Wiredu, A. N. (2021). Adoption of integrated crop-livestock management practices (ICLMPs) among men and women smallholder farmers in Ghana. Gender, Technology and Development, 25(2), 163–192. https://doi.org/10.1080/09718524.2021.1911021
- Aura, S. (2016). Determinants of the adoption of integrated soil fertility management technologies in Mbale division, Kenya. African Journal of Food, Agriculture, Nutrition and Development, 16(1), 10697–10710. https://doi.org/10.18697/ajfand.73.15735
- Ayedun, B. (2018). Drought Tolerant Maize adoption and its determinants in West Africa. Acta Scientific Nutritional Health, 2(1), 21–30. https://www.actascientific.com/ASNH/pdf/ASNH-02-0045.pdf
- Ayinde, O. (2017). Risk analysis in innovation system: A case study of production of vitamin A cassava variety among farmers in Oyo State, Nigeria. Gaziosmanpașa Üniversitesi Ziraat Fakültesi Dergisi, 34(3), 261–268. https://doi.org/10.13002/JAFAG4190
- Azumah, S. B., & Adzawla, W. (2017). Effect of urea deep placement technology adoption on the production frontier: Evidence from irrigation rice farmers in the Northern Region of Ghana. International Journal of Biological, Biomolecular, Agricultural, Food and Biotechnological Engineering, 11(4), 288–294. https://doi.org/10.5281/zenodo.1130019
- Azumah, S. B., Donkoh, S. A., & Ansah, I. G. K. (2017). Contract farming and the adoption of climate change coping and adaptation strategies in the northern region of Ghana. Environment, Development and Sustainability, 19(6), 2275–2295. https://doi.org/10.1007/s10668-016-9854-z
- Baiyegunhi, L., Akinbosoye, F., & Bello, L. (2022). Welfare impact of improved maize varieties adoption and crop diversification practices among smallholder maize farmers in Ogun State, Nigeria. Heliyon, 8(5), e09338. https://doi.org/10.1016/j.heliyon.2022.e09338
- Bello, L. O., Baiyegunhi, L. J., & Danso-Abbeam, G. (2021). Productivity impact of improved rice varieties’ adoption: Case of smallholder rice farmers in Nigeria. Economics of Innovation and New Technology, 30(7), 750–766. https://doi.org/10.1080/10438599.2020.1776488
- Bezu, S., Kassie, G. T., Shiferaw, B., & Ricker-Gilbert, J. (2014). Impact of improved maize adoption on welfare of farm households in Malawi: A panel data analysis. World Development, 59, 120–131. https://doi.org/10.1016/j.worlddev.2014.01.023
- Chandio, A. A., & Jiang, Y. (2018). Factors influencing the adoption of improved wheat varieties by rural households in Sindh, Pakistan. AIMS Agriculture and Food, 3(3), 216–228. https://doi.org/10.3934/agrfood.2018.3.216
- Da Cunha, D. A., Coelho, A. B., & Féres, J. G. (2015). Irrigation as an adaptive strategy to climate change: An economic perspective on Brazilian agriculture. Environment and Development Economics, 20(1), 57–79. https://doi.org/10.1017/S1355770X14000102
- Danso-Abbeam, G., Bosiako, J. A., Ehiakpor, D. S., Mabe, F. N., & Aye, G. (2017). Adoption of improved maize variety among farm households in the northern region of Ghana. Cogent Economics & Finance, 5(1), 1416896. https://doi.org/10.1080/23322039.2017.1416896
- Diagne, A. (2012). Adoption: A new Stata routine for estimating consistently population technological adoption parameters. Paper presented at the SAN12 Stata Conference. https://econpapers.repec.org/RePEc:boc:scon12:17
- Diagne, A., & Demont, M. (2007). Taking a new look at empirical models of adoption: Average treatment effect estimation of adoption rates and their determinants. Agricultural Economics, 37(2‐3), 201–210. https://doi.org/10.1111/j.1574-0862.2007.00266.x
- DiFalco, S., & Sharma, S. (2018). Investing in climate change adaptation: Motivations and green incentives in the Fiji Islands. Ecological Economics, 154, 394–408. https://doi.org/10.1016/j.ecolecon.2018.08.015
- Dinar, A., & Yaron, D. (1992). Adoption and abandonment of irrigation technologies. Agricultural Economics, 6(4), 315–332. https://doi.org/10.1111/j.1574-0862.1992.tb00191.x
- Donkoh, S. A., Azumah, S. B., & Awuni, J. A. (2019). Adoption of improved agricultural technologies among rice farmers in Ghana: A multivariate probit approach. Ghana Journal of Development Studies, 16(1), 46–67. https://doi.org/10.4314/gjds.v16i1.3
- Doss, C. R. (2003). Understanding farm level technology adoption: Lessons learned from CIMMYT's micro surveys in Eastern Africa. CIMMYT.
- Feder, G., & Umali, D. L. (1993). The adoption of agricultural innovations: A review. Technological Forecasting and Social Change, 43(3–4), 215–239.
- Fening, J. O., Ewusi- Mensah, N., & Safo, E. Y. (2011). Short-term effects of Cattle Manure Compost and NPK Application on Maize Grain Yield and Soil Chemical and Physical Properties. Agricultural Science Research Journal, 1(3), 69–83.
- Fisher, M., & Kandiwa, V. (2014). Can agricultural input subsidies reduce the gender gap in modern maize adoption? Evidence from Malawi. Food Policy, 45, 101–111. https://doi.org/10.1016/j.foodpol.2014.01.007
- Gebre, G. G., Mawia, H., Makumbi, D., & Rahut, D. B. (2021). The impact of adopting stress‐tolerant maize on maize yield, maize income, and food security in Tanzania. Food and Energy Security, 10(4), e313. https://doi.org/10.1002/fes3.313
- Geroski, P. A. (2000). Models of technology diffusion. Research Policy, 29(4), 603–625. https://doi.org/10.1016/S0048-7333(99)00092-X
- Ghimire, R., Wen-Chi, H., & Shrestha, R. B. (2015). Factors affecting adoption of improved rice varieties among rural farm households in Central Nepal. Rice Science, 22(1), 35–43. https://doi.org/10.1016/j.rsci.2015.05.006
- Hassan, W. (2018). Determinants of adoption of improved Maize Varieties In Zabzugu Tatale Area In The Northern Region Of Ghana: A case study of obatanpa. https://www.researchgate.net/publication/330839003_Determinants_of_Adoption_of_Improved_Maize_Varieties_in_Zabzugu-Tatale_Districts_in_the_Northern_Region_of_Ghana_A_Case_Study_of_Obaatanpa_Variety
- Jain, R., Arora, A., & Raju, S. S. (2009). A novel adoption index of selected agricultural technologies: Linkages with infrastructure and productivity. Agricultural Economics Research Review, 22(347–2016–16726), 109–120.
- Kabunga, N. S., Dubois, T., & Qaim, M. (2012). Heterogeneous information exposure and technology adoption: The case of tissue culture bananas in Kenya. Agricultural Economics, 43(5), 473–486. https://doi.org/10.1111/j.1574-0862.2012.00597.x
- Kassie, M., Stage, J., Teklewold, H., & Erenstein, O. (2015). Gendered food security in rural Malawi: Why is women’s food security status lower? Food Security, 7(6), 1299–1320. https://doi.org/10.1007/s12571-015-0517-y
- Kebede, Y., Gunjal, K., & Coffin, G. (1990). Adoption of new technologies in Ethiopian agriculture: The case of Tegulet-Bulga District, Shoa Province. Agricultural Economics: The Journal of the International Association of Agricultural Economists, 4(968–2016–75386), 27–43.
- Kimathi, S. M., Ayuya, O. I., Mutai, B., & Yildiz, F. (2021). Adoption of climate-resilient potato varieties under partial population exposure and its determinants: Case of smallholder farmers in Meru County, Kenya. Cogent Food & Agriculture, 7(1), 1860185. https://doi.org/10.1080/23311932.2020.1860185
- Krejcie, R. V., & Morgan, D. W. (1970). Determining sample size for research activities. Educational and Psychological Measurement, 30(3), 607–610. https://doi.org/10.1177/001316447003000308
- Kumar, S., Raut, R. D., Nayal, K., Kraus, S., Yadav, V. S., & Narkhede, B. E. (2021). To identify industry 4.0 and circular economy adoption barriers in the agriculture supply chain by using ISM-ANP. Journal of Cleaner Production, 293, 126023. https://doi.org/10.1016/j.jclepro.2021.126023
- Kumar, A., Takeshima, H., Thapa, G., Adhikari, N., Saroj, S., Karkee, M., & Joshi, P. (2020). Adoption and diffusion of improved technologies and production practices in agriculture: Insights from a donor-led intervention in Nepal. Land Use Policy, 95, 104621. https://doi.org/10.1016/j.landusepol.2020.104621
- Lavison, R. K. (2013). Factors influencing the adoption of organic fertilizers in vegetable production in Accra. University of Ghana.
- Loevinsohn, M., Sumberg, J., Diagne, A., & Whitfield, S. (2013). Under what circumstances and conditions does adoption of technology result in increased agricultural productivity? A Systematic Review.
- Mabe, F., Ehiakpor, D., Adam, B., & Dumasi, D. (2018). Determinants of adoption of improved rice varieties: Effects on output in Volta region. http://www.udsspace.uds.edu.gh/handle/123456789/2462
- Maertens, A., & Barrett, C. B. (2013). Measuring social networks' effects on agricultural technology adoption. American Journal of Agricultural Economics, 95(2), 353–359.
- Maguza-Tembo, F., Edriss, A.-K., & Mangisoni, J. (2017). Determinants of climate smart agriculture technology adoption in the drought prone districts of Malawi using a multivariate probit analysis. Asian Journal of Agricultural Extension, Economics & Sociology, 16(3), 1–12. https://doi.org/10.9734/AJAEES/2017/32489
- MINISTRY OF FOOD AND AGRICULTURE (MoFA) (2018). Agriculture in Ghana. Facts and Figures 2017. Statistics, Research and Information Directorate (SRID) of MoFA, October . www.mofa.gov.gh
- Mishra, K., Abdoul, S. G., Miranda, M. J., & Diiro, G. M. (2015). Gender and Dynamics of Technology Adoption. Evidence from Uganda.
- Mmbando, F. E., & Baiyegunhi, L. J. (2016). Socio-economic and institutional factors influencing adoption of improved maize varieties in Hai District, Tanzania. Journal of Human Ecology, 53(1), 49–56. https://doi.org/10.1080/09709274.2016.11906955
- Murphy, S. A., van der Laan, M. J., Robins, J. M., & Group, C. P. P. R. (2001). Marginal mean models for dynamic regimes. Journal of the American Statistical Association, 96(456), 1410–1423. https://doi.org/10.1198/016214501753382327
- Mwangi, M., & Kariuki, S. (2015). Factors determining adoption of new agricultural technology by smallholder farmers in developing countries. Journal of Economics and Sustainable Development, 6(5).
- Nkegbe, P. K., & Shankar, B. (2014). Adoption intensity of soil and water conservation practices by smallholders: Evidence from Northern Ghana. Bio-based and Applied Economics Journal, 3(1050–2016–85757), 159–174. https://doi.org/10.13128/BAE-13246
- Obayelu, A. E., & Ajayi, D. O. (2018). Economic impact and determinants of adoption of improved maize production technologies. Journal of Agricultural Sciences, Belgrade, 63(2), 217–228. https://doi.org/10.2298/JAS1802217O
- Okuthe, I. K., Ngesa, F., & Ochola, W. (2013). The socio-economic determinants of the adoption of improved sorghum varieties and technologies by smallholder farmers: Evidence from South Western Kenya. International Journal of Humanities and Social Science, 3(18), 280–292. http://www.ijhssnet.com/journals/Vol_3_No_18_October_2013/29.pdf
- Omiti, J. M., Otieno, D. J., Nyanamba, T. O., & McCullough, E. B. (2009). Factors influencing the intensity of market participation by smallholder farmers: A case study of rural and peri-urban areas of Kenya. African Journal of Agricultural and Resource Economics, 3(311–2016–5509), 57–82.
- Ragasa, C., Berhane, G., Tadesse, F., & Taffesse, A. S. (2013). Gender differences in access to extension services and agricultural productivity. The Journal of Agricultural Education and Extension, 19(5), 437–468. https://doi.org/10.1080/1389224X.2013.817343
- Rahman, M. (2021). Determinants of integrated pest management (IPM) practices dis-adoption in vegetables production in Bangladesh. International Journal of Tropical Insect Science, 41(1), 487–494. https://doi.org/10.1007/s42690-020-00231-7
- Rogers, E. M., & Williams, D. (1983). Diffusion of Innovations. The Free Press.
- Rosenbaum, P. R., & Rubin, D. B. (1985). Constructing a control group using multivariate matched sampling methods that incorporate the propensity score. The American Statistician, 39(1), 33–38. https://doi.org/10.2307/2683903
- Simtowe, F., Muange, E., Munyua, B., & Diagne, A. (2012). Technology awareness and adoption: The case of improved pigeon pea varieties in Kenya. Paper presented at the Selected Paper prepared for presentation at the International Association of Agricultural Economists (IAAE) Triennial Conference, Foz do Iguaçu, Brazil. https://econpapers.repec.org/RePEc:ags:iaae12:126760
- Tang, J., Folmer, H., & Xue, J. (2013). Estimation of awareness and perception of water scarcity among farmers in the Guanzhong Plain, China, by means of a structural equation model. Journal of Environmental Management, 126, 55–62. https://doi.org/10.1016/j.jenvman.2013.03.051
- Uaiene, R. N., Arndt, C., & Masters, W. (2009) Determinants of agricultural technology adoption in Mozambique. Discussion papers(67E).
- Ullah, A., Saqib, S. E., & Kächele, H. (2022). Determinants of farmers’ awareness and adoption of extension recommended wheat varieties in the rainfed areas of Pakistan. Sustainability, 14(6), 3194. https://doi.org/10.3390/su14063194
- Voss, R. C., Jansen, T., Mané, B., & Shennan, C. (2021). Encouraging technology adoption using ICTs and farm trials in Senegal: Lessons for gender equity and scaled impact. World Development, 146, 105620. https://doi.org/10.1016/j.worlddev.2021.105620
- Wandji, D. N., Pouomogne, V., Binam, J. N., & Nouaga, R. Y. (2012). Farmer’s perception and adoption of New Aquaculture technologies in the Western Highlands of Cameroon. Tropicultura, 30(3), 180–184.
- Wooldridge, J. M. (2002). Inverse probability weighted M-estimators for sample selection, attrition, and stratification. Portuguese Economic Journal, 1(2), 117–139. https://doi.org/10.1007/s10258-002-0008-x
- Yimer, F., Abay, K., & Degu, T. (2019). Evaluation of modern agricultural technologies adoption and impact of adoption on productivity. FARA Res. Rep, 4(3), 1–26. https://library.faraafrica.org/mp-files/evaluation-of-modern-agricultural-technologies-adoption-and-impact-of-adoption-download.pdf/