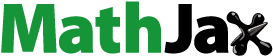
Abstract
Climate change poses a severe threat to the agriculture sector of low-income economies. To mitigate the influence of the intensifying deforestation and climate change on the environment and the livelihoods of many societies, it is crucial to illustrate the association between environmental pollution, degradation, and crop production. Somalia is facing massive deforestation. The key drivers of land degradation in Somalia are the dependence on traditional biomass energy—charcoal and firewood—, and unsustainable agriculture cultivation methods. In this regard, this study assesses the role of CO2 emissions, agriculture methane emissions, and environmental degradation on crop production in Somalia from 1990 to 2018. The long-run Cointegration and causality of the variables were assessed using an ARDL bound test and Granger causality, respectively. The study found strong empirical evidence that CO2 and agricultural methane emissions enhance crop production in the long run in Somalia. On the contrary, environmental degradation has a long-run adverse impact on crop productivity. The long-run results of the study are robust for various econometric methods. Besides, bidirectional causality between CO2 emissions and agricultural output, and Agricultural methane emissions and crop production were established. Nevertheless, the study suggests policy reforms and investments in agriculture research, agricultural input markets, seed and fertilizers systems, and policies that enhance farmers’ and pastoralists’ awareness of environmental quality.
1. Introduction
Climate change is posing a serious threat to sustainable global food security. The changes in temperature and rainfall patterns adversely influence crop production in many developing countries, which are dependent on agricultural production (Warsame et al., Citation2022b; Demirhan, Citation2020; Mohamed et al., Citation2022; Ramzan et al., Citation2022). The primary driver of climate change is the increase in carbon dioxide concentration and other greenhouse gases (GHGs) in the atmosphere, including forest loss, combustion of fossil fuels, and other non-renewable forms of energy in a variety of industrial processes (Akella et al., Citation2009; Bekun, Citation2022; Pickson et al., Citation2020). The rapid world population growth puts pressure on forestry to satisfy its increasing food demand (Tan et al., Citation2022). Farmers are using increasing quantities of fertilizers and pesticides, which caused a 60% rise in agricultural emissions (CH4) over the past four decades (Hofstra & Vermeulen, Citation2016; Tan et al., Citation2022). Consequently, it significantly contributed to environmental pollution and degradation that can be attributed to the stagnating agricultural productivity in many emerging economies (Warsame, Sheik-Ali, Barre et al., Citation2022c).
Agricultural production plays a significant role in maintaining food security and long-run economic growth in developed and developing countries (Yurtkuran, Citation2021). However, to satisfy the growing demand for agricultural products, the expansion of farming activities has resulted in a significant quantity of methane (CH4) and nitrous oxide (N2O) emissions into the atmosphere (Appiah et al., Citation2018). In the past, agricultural practices of subsistence farming were based on the utilization of manpower and animals (Dagar et al., Citation2020; Rehman et al., Citation2021). Currently, the agriculture sector relies on fossil fuels, coal, and oil for its production. These non-renewable energy sources increase the sector’s emissions, although nitrogen-rich fertilizer applications on the farm fields adversely contribute unintentionally (Yurtkuran, Citation2021). The emissions from agricultural activities account for 14–30% of global GHGs emissions (Balsalobre-Lorente et al., Citation2019). Furthermore, a diversity of human activities, including the conversion of pastures into arable land for agricultural use, overgrazing and deforestation activities, have also added to the accumulation of worldwide GHGs emissions (Rehman et al., Citation2021; Yurtkuran, Citation2021). This has raised concern for a cleaner environment as well as the achievement of sustainable agriculture production. The empirical evidence demonstrates that agricultural value-added and economic growth are the main drivers of carbon emissions due to unsustainable agricultural practices (Adedoyin et al., Citation2021; Yurtkuran, Citation2021). Rehman et al. (Citation2021) observed that an increase in crop production threatens the climatic quality by intensifying CO2 emissions. Nevertheless, the relentless increase in GHGs emissions, such as agriculture methane, CO2 emissions, and environmental degradation, lead to an increase in temperature, variations in rainfall patterns, and adversely impacting water and land resources. In addition, GHGs emissions negatively influence health and agriculture productivity (Yurtkuran, Citation2021).
Ample studies have used various cointegration methods to investigate the short and long-run impacts of climate change on crop production in different regions (Emam et al., Citation2015; Le, Citation2016; Wang, Citation2022). For instance, Ahsan et al. (Citation2020) evaluated the impacts of CO2 emissions on cereal crop output in Pakistan from 1971 to 2014. They revealed that CO2 emissions have a constructive role in enhancing cereal crop output. A bidirectional relationship between CO2 emissions and cereal crop production has also been established. Similarly, Chandio et al. (Citation2020b) investigated the impact of climate change on agricultural production in China over the period 1982–2014 using the Johansen cointegration method and the ARDL bounds test. They revealed that CO2 emissions stimulate agricultural production in the long-run and short-run. On the contrary, Ramzan et al. (Citation2022) concluded that CO2 emissions put a strain on agricultural output in Pakistan. In the same vein, Warsame et al. (Citation2021) found that CO2 emissions are inconsequential in the long run but hamper crop production in the short run in Somalia.
Other studies attributed the decline of agricultural production to the increase in industrial air pollution and overuse of fertilizers. Wei and Wang (Citation2021) investigated the impact of industrial air pollution on crop yield. The study implemented stochastic frontier analysis using data collected from a field survey in the case of corn. The findings indicated that industrial air pollution inhibits corn production. Unlike these studies, Janjua et al. (Citation2014) and Chandio et al. (Citation2020a)—using an ARDL bound test—reported climate change is inconsequential both in the short and long-run in Pakistan. The heterogeneity in the climate change-agriculture production nexus results could be attributed to the discrepancy in the methods applied, data employed, and the measurement of agriculture production. Although the literature extensively covered how environmental deterioration affects agricultural productivity, several studies concentrated on the path to sustainable development. It is observed that using green energy has a detrimental influence on environmental risks while also providing assistance in reducing environmental hazards (Zandi & Haseeb, Citation2019). Bekun (Citation2022) points out that as the usage of non-renewable energy sources is a crucial driver of economic growth in emerging nations, these countries should adopt renewable energy, which supports sustainable economic growth.
Deforestation, on the other hand, is one of the most critical aspects of environmental degradation that threatens the attainment of sustainable agriculture (Badapalli et al., Citation2022; Kumar et al., Citation2022). Forests contribute to the provision of food, prevent global warming and soil erosion, reduce pollutants and improve the water cycle (Tan et al., Citation2022). According to Hermans and McLeman (Citation2021), climate change and land degradation put increasing pressure on rural livelihoods in low-income nations. Land degradation is a major contributor to droughts and floods and has adverse effects on resource-dependent rural people leading to job losses and migration out of afflicted regions. It is argued that unsustainable farming cultivation methods hamper the ecosystem and environmental quality (Olanipekun et al., Citation2019). Crop production decline is linked to greater levels of land degradation (Sonneveld et al., Citation2016). However, certain exceptions exist, particularly in places where fertilizer is not applied. Honfoga and Parrales (Citation2018) diagnosed the link between differential soil degradation over time and fertilizer use in Benin’s cotton production. It was discovered that current fertilizer usage strategies in export-oriented cotton production systems ignore geographical disparities in soil fertility status. According to the F.A.O (Citation2018), agriculture was the leading cause of deforestation, with natural habitats, particularly forest land and natural peat lands, being converted to agricultural land, undermining the agriculture production itself. Several empirical studies have backed this argument. For instance, Mohamed and Nageye (Citation2020) found that agriculture production decreases as a result of land degradation and climate change in Somalia. Likewise, Tan et al. (Citation2022) investigated how biodiversity loss, deforestation, and agricultural emissions affect agricultural production, as well as cereal and vegetable production, using a panel of 35 European countries. The findings from the Driscoll and Kraay estimator show that biodiversity loss undermines agricultural, cereal, and vegetable output, but an increase in forest area is favourable for cereal and vegetable productivity. Agricultural emissions, on the other hand, have a negative impact on cereal output but a favourable impact on vegetable production.
Studies in Sub-Saharan African countries show the significant impact of degradation on crop output (Bindraban et al., Citation2012). Lal and Singh (Citation1998) argue that per capita arable land area is decreasing in heavily populated East African regions. Furthermore, soil erosion reduces crop yields by influencing soil organic carbon, nitrogen, phosphorous, potassium levels, and soil pH. Agricultural methane and nitrous oxide emissions, which are powerful GHGs, produced 5.3 billion tons of CO2 eq in 2018, up 14% since 2000 (F.A.O, Citation2018). In the Horn of Africa region, severe environmental degradation of forest regions and climate change significantly impacted the livestock and agriculture subsectors in recent decades (F.A.O, Citation2018). Almost all of Somalia’s floodplain forests had been destroyed for irrigated agriculture cultivation by the late 1980s. Forest cover accounted for barely 10% of the country’s land area in 2014, down from 62% in 1980. Since 1990, Somalia’s forests have lost an average of about 1% every year. The major cause of large-scale deforestation has been the unsustainable harvesting of acacia trees for charcoal production, where its annual charcoal exports peaked at $56 million in 2011. Somalia has been encountering recurrent droughts and changes in rainfall patterns, which have significantly harmed grain production for the past two decades. Moreover, the aggregate effects of several failed rainy seasons have reduced crop production, which led to a severe humanitarian situation that turned into a famine in 2011, 2017, and 2022 for many parts of Somalia (F.A.O, Citation2018; Warsame, Sheik-Ali, Barre et al., Citation2022c).
Environmental degradation—measured for deforestation—was stable in Somalia from 1990 to 2001 as depicted in Figure . It started to increase in 2001, peaking in 2005 mainly due to the growing charcoal export market, which was mainly driven by extensive deforestation. It showed oscillations from 2006 to 2013, while it remained constant from 2014 and onward. On the other hand, agricultural CH4 emissions—emissions from animals, animal waste, rice production, and agricultural waste burning—cause a rise in the earth’s surface temperature. It has shown an increasing trend from 1991 to 2005 in Somalia, as shown in Figure . It then decreased until 2008, before it began to rise again in 2009. In summing up, environmental degradation and pollution in Somalia are extremely volatile that could adversely affect agriculture production in the country.
Figure 1. Environmental pollution and degradation in Somalia. Data source: World bank, (Citation2022).
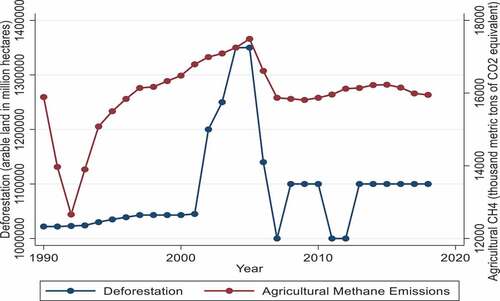
For sustainable development strategies that aim to reduce environmental degradation and improve food security, it is essential to understand the factors that influence crop output. Determining the mechanisms driving environmental degradation, CO2 emissions, and farm methane emissions is crucial since rising CO2 emissions and deforestation contribute to the diminishing agricultural output in Somalia. Several studies have been implemented on climate change—agricultural production nexus in Somalia (Mohamed & Nageye, Citation2020; Warsame et al., Citation2021). For instance, Mohamed and Nageye (Citation2020) examined the impact of land degradation on crop production, whereas (Warsame et al., Citation2021) assessed the role of CO2 emissions on crop production. Apart from the fact that these studies concluded blended findings, however, they did not consider the effect of agriculture methane emissions on crop production in Somalia, which has been very significant for the past three decades. Agriculture methane emissions represent 85.1 % of the total emissions in Somalia (World Bank, Citation2022). Against this backdrop, this study ascertains the impact of agriculture methane emissions on crop production in Somalia using a times series data spanning 1990–2018. Nevertheless, this study contributes to the literature in several ways. First, this undertaking is the first of its kind to examine the impact of agricultural methane emissions on crop production in Somalia. Second, unlike previous studies, this study incorporates environmental degradation, CO2 emissions, and agriculture methane emissions in a single model to determine their coefficient elasticities on agriculture production which is crucial for the policymakers to discover which environmental pollution indicator is harmful to agriculture production and which is helpful. Third, several econometric methods are utilized to find out robust results, such as the ARDL bound test, Granger causality, and Fully Modified Ordinary Least Square (FMOLS). The ARDL bound test precisely estimates the short- and long-run cointegration between the interested parameters, and it is also good at estimating small sample sizes (Pesaran et al., Citation2001). FMOLS is used as robust for the bound test result of the long-run coefficients. It also produces unbiased results by addressing endogeneity issues and serial correlation (Pedroni, Citation2000). Further, unlike the bound test and FMOLS, the granger causality determines the causal path of the sampled variables.
The rest of the paper is organized as follows. Section 2 presents the data and methodologies of the study. Section 3 demonstrates the empirical results of the study as well as discussion of the findings. The final section concludes the study and presents the policy implications of the findings.
2. Data and methods
This study emphasizes the connotation of environmental degradation, CO2, agricultural methane emissions, and crop production in Somalia. Somalia is considered one of the most vulnerable countries to climate change in the world (Wheeler, Citation2011). Climate consequences became recurrent and undermine both livelihoods and agricultural production in the country. This undertaking employs annual time series data stretching from 1990 to 2018 to determine the effects of CO2 emissions, agriculture methane, environmental degradation, labor, and land under cereal cultivation on crop production in Somalia. The data was extracted from different sources—the Organization of Islamic Cooperation (OIC) and the World Bank. The dependent variable is the crop production index, whereas the independent variables are environmental degradation, CO2, and agriculture methane emissions. To find out robust results, we also incorporated labor and land under cereal cultivation as control variables. Deforestation (arable land in a million hectares) is taken as a proxy for environmental degradation (Warsame & Sarkodie, Citation2021), while CO2 emissions are measured in kilo tons (Agboola & Bekun, Citation2019). Moreover, agriculture methane emission is measured in of thousands of metric tons of total CO2 emissions equivalent (Ahmed et al., Citation2021). Population growth is taken as a proxy for labor (Warsame et al., Citation2022a). Finally, land under cereal cultivation is measured in land under cereal production in a million hectares (Pickson et al., Citation2020). Notably, we converted all the variables into a natural logarithm to interpret the results as a percentage. More importantly, it reduces heteroskedasticity and serial correlation. Figure below presents the trends of the sampled variables.
==We utilized the ARDL bound test to examine the long- and short-run relationship between the variables under consideration (Pesaran et al., Citation2001). The bound test is employed on the premise that it provides efficient and robust estimates in a small sample size compared to other traditional Cointegration methods, such as Engle and Granger, and Johansen and Juselius Cointegration methods. The bound test also simultaneously estimates and presents the short and long-run relationship of the model and error correction terms (ECT), which shows the speed of adjustment of the model. Moreover, the ARDL bound test is good at estimating variables integrated at the level I (0), the first difference I (1), or the combination of both. To evaluate the role of CO2 emissions, agriculture methane, environmental degradation, labor, and land under cereal cultivation in crop production, we specify the following ARDL bound test model—by following the previous studies of Mohamed and Nageye (Citation2020), Ahmed et al. (Citation2021), and Ramzan et al. (Citation2022).
Where ,
,
,
,
, and
stand for the natural logarithm of crop production, carbon dioxide emissions, agriculture methane emissions, environmental degradation, labor, and land under cereal cultivation, respectively.
represents the disturbance term.
and
indicate the intercept and short-run coefficients, respectively.
represents the long-run coefficient parameters.
are the lag lengths of dependent and explanatory variables, respectively.
indicate the difference operators.
is the speed of adjustment.
First, we examine the presence of long-run cointegration among the variables using ordinary least squares (OLS). To conclude whether there is a cointegration or not, we compare the F-bound test with bound critical values. If the F-bound statistics fall above the upper bound critical value, we conclude that the variables are cointegrated in the long run. If it falls below the lower bound critical value, we conclude that the variables are not cointegrated in the long run. If the F-bound statistics fall between the two critical values, we conclude that it is inconclusive.
3. Empirical results and discussions
3.1. Descriptive statistics
Table presents the summary statistics and correlation of the scrutinized variables of the study. All the variables are negatively skewed except crop production and environmental degradation. Likewise, some variables—crop production, CO2 emission, and land under cereal cultivation—are identically and independently distributed, whereas the rest of the variables are not. In contrast, correlations of the interesting variables are also presented in Table . All variables are positively correlated with crop production. In the same vein, it was observed that all of the variables have a positive correlation with environmental degradation, except CO2 emissions. Unlike environmental degradation, CO2 emissions have negative correlation with all other variables except crop production, which is a positive relationship.
Table 1. Descriptive statistics
3.2. Unit root test
Time series data are often trending, which violates the assumption of stationary. Hence, we examine the unit root of the interested variables to circumvent spurious results using Augmented Dickey-Fuller (ADF) and Philips Perron (PP) tests. The unit root results are exhibited in Table . Crop production, agriculture methane emissions, and land under cereal cultivation have shown mixed order of integration at level I (0) and the first difference I (1). In contrast, other variables are stationary at the first difference I (1). Hence, the ARDL method is suitable for the character of our data. Next, we proceed to estimate the presence of long-run Cointegration and their coefficient elasticity.
Table 2. Result of the ADF and PP unit root tests
We examine the existence of long-run Cointegration among crop production and the explanatory variables—CO2 emissions, environmental degradation, agriculture methane, labor, and land under cereal cultivation—using the bound test. The bound test result is reported in Table . The F-bound test statistics (17.3) is greater than the upper bound critical value (6.32) at a 1% significance level. This sheds light on the presence of long-run movement together between crop production and the scrutinized independent variables.
Table 3. F-bounds cointegration tests
The long- and short-run results are presented in Tables , respectively. It was established that all the explanatory variables are statistically significant in the long run except land under cereal cultivation, which is statistically insignificant. CO2 and agriculture methane emissions significantly enhance crop production in the long run in Somalia. On the contrary, environmental degradation significantly undermines crop production in the long run. Interpretively, a 1% increase in CO2 and agriculture methane emissions result in crop production increasing by about 0.56% and 1.95%, respectively, in the long run. Moreover, environmental degradation hampers agriculture production by about 0.25% in the long run if it is increased by 1%. Besides, labor—which is used as a control variable—has produced a significant and negative result, whereas land under cereal cultivation is not different from zero amid its insignificance. A 1% increase in labor inhibits agriculture production by about 0.088% in the long run.
Table 4. Long-run coefficient elasticities
Table 5. Short-run dynamic effect and diagnostic test
A striking result of our study is that environmental pollution and degradation have blended effects on crop production in Somalia in the long run.
On the other hand, short-run dynamic effects are estimated and presented in Table . Environmental degradation is statistically insignificant in the short run. In the short run, CO2 emissions have the same effect as in the long run. It significantly stimulates crop production in the short run. A 1% increase in CO2 emissions significantly increases crop production by about 0.98% in the short run. In the same vein, agriculture methane emissions enhance crop production by about 1.1% in the short run if it is increased by 1%. Notably, agriculture methane emissions contribute substantially to the rise of crop production amid its high coefficient elasticity both in the long and short run. In addition, labor impedes crop production in the short run by about 0.12% if labor is increased by 1%. Land under cereal cultivation significantly improves crop production in the short run. A 1% increase in land under cereal cultivation results in crop production increasing by 0.149% in the short run. More importantly, the error correction term (ECT), presented in Table , revealed that a long-run crop production convergence occurs in the short run. Any shock disequilibrium that occurs in crop production in the short run is adjusted 82% by the scrutinized independent variables in the long run.
Furthermore, the diagnostic tests and model stability of the study are also reported in Table . Several diagnostic tests were performed, such as; serial correlation (Breusch-Godfrey test), heteroskedasticity (Breusch-Pagan test), model misspecification (Ramsey reset test), and normality test. No diagnostic test has been detected, and the model of the study is free from all the diagnostic problems. Likewise, the model of the study is also stable, as depicted in Figures . In addition, the goodness fit of the model is suitable as presented by the Adjusted R-squared, where 95% of the variation in crop production is explained by the scrutinized explanatory variables—CO2, agriculture methane emissions, environmental degradation, labor, and land under cereal cultivation.
Robustness of the ARDL long-run results of the study was performed using fully modified ordinary least squares (FMOLS). Its result presented in Table indicated that CO2 emissions, agriculture methane emissions, and land under cereal cultivation significantly enhance crop production in Somalia in the long run. On the contrary, environmental degradation and labor undermine crop production in the long run, even though they are statistically insignificant. Overall, the result of the FMOLS has robustly verified the long-run results of the ARDL.
Table 6. Method: Fully modified ordinary least squares (FMOLS)
3.3. Granger causality
After analyzing the long-run Cointegration between crop production and its determinants, we subsequently examine the Granger causality among the interested variables. Granger causality result is reported in Table . It revealed the presence of bidirectional causality between CO2 emissions and crop production. This implies that both variables affect each other. Likewise, there is bidirectional causality between agriculture methane emissions and crop production. This result is in line with the previous study by Sarkodie and Owusu (Citation2017) and Rehman et al. (Citation2020), who found the same results. Notably, agriculture methane and CO2 emissions cause each other, hence, confirming the presence of bidirectional causality between them. On the contrary, environmental degradation and crop production do not cause each other. Regarding this, environmental pollutions cause crop production, whereas environmental degradation does not. Moreover, labor and crop production share bidirectional causality, whereas a unidirectional causality is established from land under cereal cultivation to crop production. Bidirectional causality is observed between labor and CO2 emissions. Labor—which is measured for population growth—is a key determinant for both environmental pollution and crop production in Somalia. Population growth exerts the increase of anthropogenic emissions, which affect agriculture production. Finally, land under cereal cultivation granger causes crop production. This could be explained by the fact that an increase in agricultural land tends to raise agricultural production due to the availability of land. Moreover, this finding corroborates some previous studies (see, Ben Jebli & Ben Youssef, Citation2018; Warsame et al., Citation2021).
Table 7. Pairwise granger causality tests
3.4. Discussion of the result
The empirical results of the study have detected that environmental pollution—CO2 and agriculture methane emissions—significantly contribute to crop production increase in the long run, whereas environmental degradation hampers it. Moreover, environmental pollution indicators and crop production are also observed to granger cause each other, whereas environmental degradation and crop production do not.
Environmental pollutions result from burning fossil fuels, using agricultural fertilizers, and burning trees for coal consumption, whereas environmental degradation is derived from degrading the trees. Hence, the latter has direct adverse effects on the quality of the soil, ecosystem, and exploitation of natural resources. Consequently, agriculture production decreases amid the fertility loss of the land. However, this justifies our negative finding of environmental degradation in agriculture production. It also corroborates the previous study of Mohamed and Nageye (Citation2020), who concluded that environmental degradation hampers agriculture production in Somalia. It is also supported by the recent study of Tan et al. (Citation2022), who reported that environmental degradation—in the form of biodiversity loss and deforestation—undermines agriculture production in 35 European countries.
On the other extreme, environmental pollution indicators—CO2 and agriculture methane emissions—significantly enhance crop production in Somalia. The world population is predicted to reach 9.7 billion in 2050 (Arora, Citation2019). Therefore, the increasing consumption of fertilizers and unsustainable agriculture production to meet the growing food demand contributes the increasing greenhouse gases (GHG), such as CO2 and agriculture methane emissions. They increase agriculture production at the cost of society in the long-run, such as by causing modifications in rainfall patterns and climate change. Doubling CO2 concentrations will result in a 2–3% increase in temperature. But agriculture yields will increase with CO2 enrichment (Kimball & Idso, Citation1983). Nevertheless, this justifies our finding that environmental pollution has a constructive role in stimulating agriculture production in the long run in Somalia. Our result is supported by the previous examination of Wang (Citation2022) who revealed that environmental pollution—specifically CO2 emissions—enhance agriculture economic growth in China in the long-run. Ramzan et al. (Citation2022) arrived at the same conclusion that environmental pollution increases agriculture production in the long run in Pakistan. Similar findings of the positive effect of CO2 emissions on agriculture production were observed in Pakistan and China by Chandio et al. (Citation2020) and Ahsan et al. (Citation2020), respectively.
Further, population growth, which is used as a proxy for labor, is determined to have an inhibiting effect on crop production in Somalia in the long run. This result corroborates similar studies conducted in Somalia (Warsame et al., Citation2021; Warsame, Sheik-Ali, Mohamed et al., Citation2022c). They found that agricultural labor undermines crop production and maize production in Somalia in the long run. Similarly, Warsame et al. (Citation2022a) reported that agricultural labor impedes livestock production in Somalia in the long run. The Somali population is growing at a higher rate, whereas domestic food production is stagnant. Hence, this puts pressure on the scarce food available. In contrast, land under cereal cultivation is inconsequential in the long run; even though it has positive coefficient elasticity. This finding is in line with the previous results of Ramzan et al. (Citation2022) who documented that agricultural land is insignificant both in the short- and long-run in Pakistan.
4. Conclusion and policy recommendation
Climate change is a global concern and an imminent threat in the 21st century amid its adverse consequences on health, agriculture production, and food security. To account for the effects of climate change on agriculture production, several studies have employed various measurements of climate change, including rainfall, temperature, greenhouse gases (GHG), and environmental degradation. In this regard, this study assessed the role of CO2 emissions, agriculture methane, and environmental degradation—along with labor and land under cereal cultivation—on crop production in Somalia over the period 1990 - 2018. The ARDL bound test and Granger causality were used to assess the long-run relationship and causality of the variables respectively.
The empirical results indicated that CO2 and agriculture methane emissions significantly enhance crop production in the long run in Somalia. On the contrary, environmental degradation significantly undermines crop production in the long run. Interpretively, a 1% increase in CO2 and agriculture methane emissions leadto crop production increase by about 0.56% and 1.95%, respectively, in the long run. Environmental degradation hampers crop production by about 0.25% in the long run if it is increased by 1%. Furthermore, labor—which is used as a control variable—has produced a significant and negative result, whereas land under cereal cultivation is not different from zero amid its insignificant in the long run. A 1% increase in labor inhibits crop production by about 0.088% in the long run. The FMOLS verifies the long-run results of the ARDL. Besides, the granger causality revealed the presence of bidirectional causality between CO2 emissions and crop production. Likewise, there is bidirectional causality between agriculture methane emissions and crop production. Notably, agriculture methane and CO2 emissions cause each other, confirming the bidirectional causality between them. On the contrary, environmental degradation and crop production do not cause each other. Regarding this, environmental pollutions cause crop production whereas environmental degradation does not. Moreover, labor and crop production share bidirectional causality, whereas a unidirectional causality is established from land under cereal cultivation to crop production. Bidirectional causality is observed between labor and CO2 emissions. Finally, land under cereal cultivation granger causes crop production.
In light of policy implications, we suggest the following policy recommendations. First, as per our findings, agriculture production is strongly linked to CO2, agriculture methane emissions, and deforestation in Somalia. Somalia is one of the most vulnerable countries to climate variability in the world, hence, hampering the agriculture sector. Hence, to confirm sustainable development goals (SDGs 2, 4, and 12) that emphasize sustainable agriculture production, policies towards deforestation reduction should be implemented, such as mitigating the dependence on traditional biomass energy and the unsustainable agriculture production by investing in clean energy and introducing sustainable agriculture cultivation methods. Since energy is the engine of growth and agriculture productivity, clean energy reduces environmental pollution and degradation without compromising sustainable agriculture production. Further, increasing investments in strengthening agriculture production against diseases and pests should also be implemented. Second, even though environmental pollutions have favorable effects on crop production in Somalia, it will adversely impact the environment and agriculture in the long run. Hence, the study suggests policy reforms and investments in agriculture research, agricultural input markets, seed and fertilizers systems, and policies that enhance farmers’ awareness of environmental quality. These will not only help reduce environmental pollution but also confirm sustainable agriculture production. Third, the study proposes that improved and resistant crops to climate change should be developed and introduced to cope with the adverse effects of climate change.
One of the limitations of the study is that it focused on the impact of environmental pollution and degradation on crop production. Nevertheless, different types of crops react differently to land degradation and emissions. Hence, future studies should examine the influence of degradation and emissions on disaggregated food crops.
Credit author statement
Abdimalik Ali Warsame: Conceptualization, methodology, data collection, and analysis. Reviewing and Editing.
Abdikafi Hassan Abdi: Writing the introduction and literature review.
Disclosure statement
No potential conflict of interest was reported by the author(s).
Data availability statement
The datasets used and/or analyzed during the current study are available from the following link:
https://data.worldbank.org/indicator/AG.PRD.CROP.XD?locations=SO
https://www.sesric.org/query.php
https://climateknowledgeportal.worldbank.org/download-data
Compliance with ethical standards
Additional information
Funding
References
- Adedoyin, F. F., Bein, M. A., Gyamfi, B. A., & Bekun, F. V. (2021). Does agricultural development induce environmental pollution in E7? A myth or reality. Environmental Science and Pollution Research, 28(31), 41869–16. https://doi.org/10.1007/s11356-021-13586-2
- Agboola, M. O., & Bekun, F. V. (2019). Does agricultural value added induce environmental degradation? Empirical evidence from an agrarian country. Environmental Science and Pollution Research, 26(27), 27660–27676. https://doi.org/10.1007/s11356-019-05943-z
- Ahmed, M., Aziz, N., Tan, Z., Yang, S., Raza, K., & Kong, R. (2021). Green growth of cereal food production under the constraints of agricultural carbon emissions : A new insights from ARDL and VECM models. Sustainable Energy Technologies and Assessments, 47(July), 101452. https://doi.org/10.1016/j.seta.2021.101452
- Ahsan, F., Chandio, A. A., & Fang, W. (2020). Climate change impacts on cereal crops production in Pakistan: Evidence from cointegration analysis. International Journal of Climate Change Strategies and Management, 12(2), 257–269. https://doi.org/10.1108/IJCCSM-04-2019-0020
- Akella, A. K., Saini, R. P., & Sharma, M. P. (2009). Social, economical and environmental impacts of renewable energy systems. Renewable Energy, 34(2), 390–396. https://doi.org/10.1016/j.renene.2008.05.002
- Appiah, K., Du, J., & Poku, J. (2018). Causal relationship between agricultural production and carbon dioxide emissions in selected emerging economies. Environmental Science and Pollution Research, 25(25), 24764–24777. https://doi.org/10.1007/s11356-018-2523-z
- Arora, N. K. (2019). Impact of climate change on agriculture production and its sustainable solutions. Environmental Sustainability, 2(2), 95–96. https://doi.org/10.1007/s42398-019-00078-w
- Badapalli, P. K., Kottala, R. B., Madiga, R., & Golla, V. (2022). An integrated approach for the assessment and monitoring of land degradation and desertification in semi-arid regions using physico-chemical and geospatial modeling techniques. Environmental Science and Pollution Research. https://doi.org/10.1007/s11356-022-23002-y
- Balsalobre-Lorente, D., Driha, O. M., Bekun, F. V., & Osundina, O. A. (2019). Do agricultural activities induce carbon emissions? The BRICS experience. Environmental Science and Pollution Research, 26(24), 25218–25234. https://doi.org/10.1007/s11356-019-05737-3
- Bekun, F. V. (2022). Mitigating emissions in India: Accounting for the role of real income, renewable energy consumption and investment in energy. International Journal of Energy Economics and Policy, 12(1), 188–192. https://doi.org/10.32479/ijeep.12652
- Ben Jebli, M., & Ben Youssef, S. (2018). Investigating the interdependence between non-hydroelectric renewable energy, agricultural value added, and arable land use in Argentina. Environmental Modeling and Assessment, 24(5), 533–546. https://mpra.ub.uni-muenchen.de/77513/
- Bindraban, P. S., van der Velde, M., Ye, L., Van den Berg, M., Materechera, S., Kiba, D. I., Van Lynden, G., Ragnarsdóttir, K. V., Jongschaap, R., Hoogmoed, M., Hoogmoed, W., van Beek, C., & van Lynden, G. (2012). Assessing the impact of soil degradation on food production. Current Opinion in Environmental Sustainability, 4(5), 478–488. https://doi.org/10.1016/j.cosust.2012.09.015
- Chandio, A. A., Jiang, Y., Rauf, A., Ahmad, F., Amin, W., & Shehzad, K. (2020a). Assessment of formal credit and climate change impact on agricultural production in Pakistan: A time series ARDL modeling approach. Sustainability, 12(13), 5241. https://doi.org/10.3390/su12135241
- Chandio, A. A., Jiang, Y., Rehman, A., & Rauf, A. (2020). Short and long-run impacts of climate change on agriculture: An empirical evidence from China. International Journal of Climate Change Strategies and Management, 12(2), 201–221. https://doi.org/10.1108/IJCCSM-05-2019-0026
- Chandio, A. A., Jiang, Y., Rehman, A., & Rauf, A. (2020b). Short and long-run impacts of climate change on agriculture: An empirical evidence from China. International Journal of Climate Change Strategies and Management, 12(2), 201–221. https://doi.org/10.1108/IJCCSM-05-2019-0026
- Dagar, V., Bansal, E., Murshed, M., Mishra, V., Alvarado, R., Kumar, A., & Anser, M. K. (2020). Stochastic frontier analysis to measure technical efficiency: Evidence from skilled and unskilled agricultural labor in India. International Journal of Agricultural and Statistical Sciences, 16(2), 647–657. https://connectjournals.com/file_full_text/3265400H_647-657a.pdf
- Demirhan, H. (2020). Impact of increasing temperature anomalies and carbon dioxide emissions on wheat production. Science of the Total Environment, 741, 139616. https://doi.org/10.1016/j.scitotenv.2020.139616
- Emam, A. R., Kappas, M., & Hosseini, S. Z. (2015). Assessing the impact of climate change on water resources, crop production and land degradation in a semi-arid river basin. Hydrology Research, 46(6), 854–870. https://doi.org/10.2166/nh.2015.143
- F.A.O. (2018). Rebuilding Resilient and Sustainable Agriculture in Somalia. Food and Agriculture Organization of the United Nations. https://www.fao.org/publications/card/en/c/I9060EN/
- Hermans, K., & McLeman, R. (2021). Climate change, drought, land degradation and migration: Exploring the linkages. Current Opinion in Environmental Sustainability, 50, 236–244. https://doi.org/10.1016/j.cosust.2021.04.013
- Hofstra, N., & Vermeulen, L. C. (2016). Impacts of population growth, urbanisation and sanitation changes on global human Cryptosporidium emissions to surface water. International Journal of Hygiene and Environmental Health, 219(7), 599–605. https://doi.org/10.1016/j.ijheh.2016.06.005
- Honfoga, B. G., & Parrales, I. G. (2018). Diagnosing soil degradation and fertilizer use relationship for sustainable cotton production in Benin. Cogent Environmental Science, 4(1), 1422366. https://doi.org/10.1080/23311843.2017.1422366
- Janjua, P. Z., Samad, G., & Khan, N. (2014). Climate change and wheat production in Pakistan: An autoregressive distributed lag approach. NJAS-Wageningen Journal of Life Sciences, 68(1), 13–19. https://doi.org/10.1016/j.njas.2013.11.002
- Kimball, B. A., & Idso, S. B. (1983). Increasing atmospheric CO2: Effects on crop yield, water use and climate. Agricultural Water Management, 7(1–3), 55–72. https://doi.org/10.1016/0378-3774(83)90075-6
- Kumar, B. P., Babu, K. R., Anusha, B. N., & Rajasekhar, M. (2022). Geo-environmental monitoring and assessment of land degradation and desertification in the semi-arid regions using Landsat 8 OLI/TIRS, LST, and NDVI approach. Environmental Challenges, 8(February), 100578. https://doi.org/10.1016/j.envc.2022.100578
- Lal, R., & Singh, B. R. (1998). Effects of soil degradation on crop productivity in East Africa. Journal of Sustainable Agriculture, 13(1), 15–36. https://doi.org/10.1300/J064v13n01_04
- Le, T. T. (2016). Effects of climate change on rice yield and rice market in Vietnam. Journal of Agricultural and Applied Economics, 48(4), 366–382. https://doi.org/10.1017/aae.2016.21
- Mohamed, J., Abdi, D., Abdimalik, A., Warsame, A., & Bakri, M. (2022). Two phases of long term shift in extreme precipitation in Somalia. Meteorology and Atmospheric Physics, 134(3). https://doi.org/10.1007/s00703-022-00896-4
- Mohamed, A. A., & Nageye, A. I. (2020). Measuring the effect of land degradation and environmental changes on agricultural production in Somalia with two structural breaks. Management of Environmental Quality: An International Journal, 32(2), 160–174. https://doi.org/10.1108/MEQ-02-2020-0032
- Olanipekun, I. O., Olasehinde-Williams, G. O., & Alao, R. O. (2019). Agriculture and environmental degradation in Africa: The role of income. Science of the Total Environment, 692, 60–67. https://doi.org/10.1016/j.scitotenv.2019.07.129
- Pedroni, P. (2000). Fully modified Ols for heterogeneous cointegrated panels. In nonstationary panels, panels cointegration, and dynamic panels. Nonstationary Panels, Panel Cointegration and Dynamic Panels, 15, 93–130. https://pdfs.semanticscholar.org/b6b4/fe66e3344b173e4cd91c9ec768296c2e4fbf.pdf
- Pesaran, M. H., Shin, Y., & Smith, R. J. (2001). Bounds testing approaches to the analysis of level relationships. Journal of Applied Econometrics, 16(3), 289–326. https://doi.org/10.1002/jae.616
- Pickson, R. B., He, G., Ntiamoah, E. B., & Li, C. (2020). Cereal production in the presence of climate change in China. Environmental Science and Pollution Research, 27(36), 45802–45813. https://doi.org/10.1007/s11356-020-10430-x
- Ramzan, M., Iqbal, H. A., Usman, M., & Ozturk, I. (2022, January 1–20). Environmental pollution and agricultural productivity in Pakistan: New insights from ARDL and wavelet coherence approaches. Environmental Science and Pollution Research, 29(19), 28749–28768. https://doi.org/10.1007/s11356-021-17850–17853.
- Rehman, A., Ma, H., & Ozturk, I. (2020). Decoupling the climatic and carbon dioxide emission influence to maize crop production in Pakistan. Air Quality, Atmosphere and Health, 13(6), 695–707. https://doi.org/10.1007/s11869-020-00825-7
- Rehman, A., Ulucak, R., Murshed, M., Ma, H., & Işık, C. (2021). Carbonization and atmospheric pollution in China: The asymmetric impacts of forests, livestock production, and economic progress on CO2 emissions. Journal of Environmental Management, 294, 113059. https://doi.org/10.1016/j.jenvman.2021.113059
- Sarkodie, S. A., & Owusu, P. A. (2017). The relationship between carbon dioxide, crop and food production index in Ghana: By estimating the long-run elasticities and variance decomposition. Environmental Engineering Research, 22(2), 193–202. https://doi.org/10.4491/eer.2016.135
- Sonneveld, B. G. J. S., Keyzer, M. A., & Ndiaye, D. (2016). Quantifying the impact of land degradation on crop production: The case of Senegal. Solid Earth, 7(1), 93–103. https://doi.org/10.5194/se-7-93-2016
- Tan, D., Adedoyin, F. F., Alvarado, R., Ramzan, M., Kayesh, M. S., & Shah, M. I. (2022). The effects of environmental degradation on agriculture: Evidence from European countries. Gondwana Research, 106(June), 92–104. https://doi.org/10.1016/j.gr.2021.12.009
- Wang, H. (2022). Role of environmental degradation and energy use for agricultural economic growth: Sustainable implications based on ARDL estimation. Environmental Technology and Innovation, 25, 102028. https://doi.org/10.1016/j.eti.2021.102028
- Warsame, A. A., & Sarkodie, S. A. (2021). Asymmetric impact of energy utilization and economic development on environmental degradation in Somalia. Environmental Science and Pollution Research, 29(16), 23361–23373. https://doi.org/10.1007/s11356
- Warsame, A., Sheik-ali, A., A, I., Jama, O. M., Abdirahman, A., & Mohamed, G. (2022b). Assessing the effects of climate change and political instability on sorghum production : Empirical evidence from Somalia. Journal of Cleaner Production, 360(April), 131893. https://doi.org/10.1016/j.jclepro.2022.131893
- Warsame, A. A., Sheik-Ali, I. A., Ali, A. O., & Sarkodie, S. A. (2021). Climate change and crop production nexus in Somalia: An empirical evidence from ARDL technique. Environmental Science and Pollution Research, 1–13. https://doi.org/10.1007/s11356-020-11739-3
- Warsame, A. A., Sheik-Ali, I. A., Barre, G. M., & Ahmed, A. (2022c). Examining the effects of climate change and political instability on maize production in Somalia. Environmental Science and Pollution Research, 1–14. https://doi.org/10.1007/s11356-022-22227-1
- Warsame, A. A., Sheik-Ali, I. A., Hassan, A. A., & Sarkodie, S. A. (2022a). Extreme climatic effects hamper livestock production in Somalia. Environmental Science and Pollution Research, 1–13. https://doi.org/10.1007/s11356-021-18114-w
- Warsame, A. A., Sheik-Ali, I. A., Mohamed, J., & Sarkodie, S. A. (2022c). Renewables and institutional quality mitigate environmental degradation in Somalia. Renewable Energy, 194, 1184–1191. https://doi.org/10.1016/j.renene.2022.05.109
- Wei, W., & Wang, Z. (2021). Impact of industrial air pollution on agricultural production. Atmosphere, 12(5), 639. https://doi.org/10.3390/atmos12050639
- Wheeler, D. (2011). Quantifying vulnerability to climate change : Implications for adaptation assistance working Paper 240 January 2011. In Center for global development. https://www.cgdev.org/sites/default/files/1424759_file_Wheeler_Quantifying_Vulnerability_FINAL.pdf
- World Bank. (2022). data.worldbank.org. Retrieved from https://data.worldbank.org/indicator/AG.LND.ARBL.HA?locations=SO
- Yurtkuran, S. (2021). The effect of agriculture, renewable energy production, and globalization on CO2 emissions in Turkey: A bootstrap ARDL approach. Renewable Energy, 171, 1236–1245. https://doi.org/10.1016/j.renene.2021.03.009
- Zandi, G., & Haseeb, M. (2019). The importance of green energy consumption and agriculture in reducing environmental degradation: Evidence from sub-Saharan African countries. International Journal of Financial Research, 10(5), 215–227. https://doi.org/10.5430/ijfr.v10n5p215