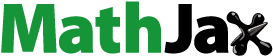
Abstract
The concept of e-Agriculture has become prominent in recent times as a mechanism for the modinization of agriculture especially in developing nations like Ghana. The concept encompasses the creation of a platform for utilizing Information and Communication Technology (ICT)-mediated tools by practitioners such as extensionists and farmers to promote agricultural production and productivity. A key component of e-Agriculture is the use of mobile phones in agriculture. In Ghana, there is little knowledge of the factors driving access and usage of mobile phones by farmers and the associated impact on household crop income. To provide empirical evidence of mobile phone access and usage and the impact on crop income, this study was carried out relying on data from 2,662 respondents. Specifically, the study categorized mobile phone usage into agricultural and non-agricultural use. Multinomial endogenous treatment effect model was employed to assess the drivers of mobile phone usage and the impact of usage on crop income. The results showed that cost of airtime, location of the household, and access to electricity significantly influenced mobile phone usage for both agricultural and non-agricultural purposes. Furthermore, gender, educational status, and livestock ownership significantly influenced mobile phone usage for agricultural purposes. With regard to impact, the results showed that crop income increased with mobile phone usage, with a higher-income effect for agricultural usage. The study therefore recommends that the National Communication Authority (NCA) should partner with telecommunication companies to ensure that mobile network connectivity is made available and accessible to rural communities to promote mobile phone usage. Also, extension agents should adopt the use of mobile phones to render extension services in order to reach more farmers. Meanwhile, reducing the tax on airtime for rural dwellers will go a long way to promote mobile phone usage and increase crop income.
1. Introduction
Agriculture is said to be the “Oxygen” for most developing countries providing jobs and livelihoods for many households (Nwanma, Citation2021). Hence, there is the need for developing countries to ensure that their agricultural sectors continue to grow and expand to meet the needs of the growing populations. A major factor for improving the agricultural sector is the development and application of cutting-edge technologies in production (Canton, Citation2021). This brings to fore the need for smart technologies in agriculture. The issue of smart-agriculture stems from the application of digital agricultural technologies that offer innovative platforms for agricultural service delivery. One such innovation is e-Agriculture that involves, among other things, the use of information and communication technology in agriculture (MoFA, Citation2017). According to Okediran and Ganiyu (Citation2019), e-Agriculture is a field that applies current information and communication methods, innovations, and technologies to increase agricultural productivity and make information available for agricultural purposes which may include agricultural research and production monitoring, among others. In Ghana, the Ministry of Food and Agriculture (MOFA) seeks to modernize the agricultural sector through research and technology development as well as effective extension delivery (MoFA, Citation2017). The information and communication unit of the ministry continues to explore ways of digitizing agricultural service delivery through the use of ICT tools through the framework of what is commonly referred to as e-Agriculture. One of the key components of e-Agriculture that is of great importance in rendering agricultural services to farmers is the mobile phone. The mobile phone helps to provide access to information and offers a platform for communication and training, as well as an avenue for financial inclusion through savings and credit accessibility (Abdul‐rahaman & Abdulai, Citation2021; Hoang, Citation2020).
Awuor et al. (Citation2016) asserted that poverty in most agrarian economies is related to the disconnection between e-Agriculture and the information needs of farmers, adding that the proper application of ICT in agriculture can potentially help increase food security and promote sustainable agriculture. They further argued that e-Agriculture would enhance farmers’ access to relevant and timely information about pre-harvest and post-harvest practices as well as pricing and weather conditions which are necessary for increased productivity. Okediran and Ganiyu (Citation2019) also revealed that e-Agriculture helps to increase farmers’ knowledge about production practices and connects farmers with remunerative markets, hence increasing household incomes and reducing poverty. According to the Food and Agriculture Organization of the United Nations (FAO) and the International Telecommunication Union (ITU), e-Agriculture can propel growth of the economy and improve earnings of poor people through its mediating role in promoting efficiency of production, livelihoods, and agricultural value-chain development (FAO, Citation2017).
In Pakistan, a mobile phone–based e-agricultural system was presented as another option to the traditional extension method, and an evaluative study found that producers favored voice-based content of extension delivery in comparison to text messages (Khan et al., Citation2020). The authors thus concluded that a mobile phone–based system is an efficient tool which is good for extension delivery but must go along with some trainings on digital literacy for optimum results (Khan et al., Citation2020). In another study, Hoang (Citation2020) investigated the factors driving the adoption of ICT tools among Vietnamese vegetable producers using mobile phones as a proxy for usage of ICT tools. He found that the adoption of mobile phones for agricultural marketing was influenced by socioeconomic, demographic, and institutional issues.
In Ghana, the Planting for Food and Jobs (PFJ) programme is a major government initiative that seeks to boost agricultural productivity and provides jobs for farmers through increased crop incomes, among others. Among the strategic pillars of the PFJ is the e-Agriculture component which seeks to add ICT to other thematic areas such as fertilizer subsidy, provision of quality seeds, agricultural extension, and marketing. The purpose of the e-Agriculture concept is to promote efficiency, transparency, and accountability through the provision of fast, reliable, and accurate dissemination of information to stakeholders (MoFA, Citation2017). The ICT tools highlighted in the program include mobile phones and software applications to help offer web portal, text-based, and mobile internet-based services to farmers in order to facilitate easy dissemination of innovations (MoFA, Citation2017). While this initiative is very laudable, there is generally a lack of empirical evidence on the efficacy of this component, including the factors influencing the access and usage of these ICT tools. So far, much of the emphasis has been on the fertilizer subsidy policy and provision of improved seeds to farmers, with little empirical evidence of the impact of the e-Agriculture component.
Nyarko and Kozári (Citation2021) observed that despite technological advancements made in other countries to promote e-Agriculture, access to agricultural and extension information in Ghana remains a challenge to most rural cocoa farmers, ostensibly due to the disconnect between e-Agriculture and the information needs of farmers. This study therefore seeks to advance the frontiers of knowledge on smart agriculture in Ghana focusing on e-Agriculture by investigating the drivers of mobile phone access and usage by farmers and estimating the effect of mobile phone usage on crop income. The results will help inform agricultural extension and advisory service delivery and farm-related services aimed at increasing crop incomes and reaching a global agenda of zero hunger and poverty. The findings will also serve as a guide to policymakers in complementing technology with conventional extension delivery systems to bridge the deficit in extension agent-to-farmer ratio in a cost-effective manner.
2. Data and methods
2.1. Study area and source of data
The research covered two regions of Ghana, namely the Upper West and Central regions. The Upper West Region is situated in the northern sector of Ghana, while the Central region is located in the southern part of the country. Ghana is made up of 16 administrative regions with varied ecological conditions. The Upper West Region is situated in the Guinea savanna, while the Central Region is located mainly in the semi-deciduous rainforest. The Central region has a population of 2,859,821, while the Upper West has a population of about 904,695. The Upper West region (70%) has the highest poverty incidence rate among all the regions in Ghana, with the Central region having 11 districts scoring an average poverty incidence rate of 19.6% and one other district with 5.7% poverty incidence rate (Ghana Statistical Service, Citation2021). Farming is a major economic activity in both regions.
Source: Ghana Statistical Service, Citation2021
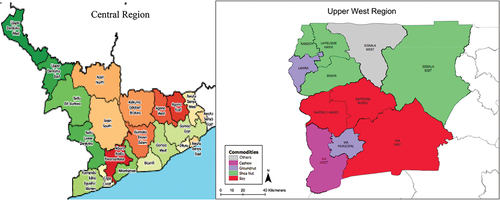
The data used for the study are secondary data which were extracted from a 5-year mNutrition global initiative supported by the Department for International Development (DFID) and collected between 2013 and 2018. The research was conducted by the University of Ghana Institute of Statistical, Social and Economic Research (ISSER) in collaboration with the International Food Policy Research Institute (IFPRI). Household interviews were carried out before and after the intervention. Central and Upper West Regions of Ghana were chosen for quantitative data collection. The mNutrition intervention had two components, made up of the nutrition and agricultural components. At baseline, 3,933 households from 207 communities were interviewed. Participants in the interviews included both primary male and female household members. The project used a semi-structured questionnaire in soliciting data from households. Simple random sampling was used in the selection of communities and households in the research areas. Out of the total sample, 2,662 households were identified as agricultural households and were selected for this study. If at least one household member used a mobile phone, such household was counted as having access to mobile phone. Information on mobile phone usage for agricultural purposes was collected in order to categorize farm households into those who use their phones for agricultural and non-agricultural purposes, respectively.
2.2. Theoretical and empirical framework
The theoretical framework of the study is explained by the utility maximization theory that states that a rational household will choose to use a mobile phone for agricultural purposes based on the utility derived from the mobile phone usage. Hence, a household i may choose to be part of a group of mobile phone users j who use their mobile phones for agricultural purposes, over another group of mobile phone users k who do not use their phones for agricultural purposes, if , where
is the utility derived from being in a particular group. Technically, a household may own a mobile phone but may not use it for agricultural purposes, such as marketing or receiving of advisory services from agricultural extension agents. The interest here is to identify how households who have mobile phone access also use these mobile phones to improve their crop income. For the purposes of this study, access means that a particular household had a mobile phone but did not use it for agricultural purposes, while usage refers to having a mobile phone and using it for agricultural purposes.
Mobile phone access is binary in the sense that a farmer either owns a mobile phone or does not. Similarly, mobile phone usage is binary, implying that the farmer either uses a mobile device for agricultural purposes or not. The dependent variable for mobile phone access and usage is therefore categorical: 0 if a household does not own a mobile phone; 1 if a household owns a mobile phone (but does not use it for agricultural purposes); and 2 if a household owns and uses a mobile phone for agricultural purposes). Analysis of such dependent variables requires a multinomial economic framework that jointly estimates the individual binary equations, using one category as reference point (0).
A typical approach for analyzing the impact of a multinomial dependent variable on a continuous variable such as farm income in the literature is the use of multinomial endogenous treatment effect model (METEM). Farm income is potentially endogenous in the multinomial framework, and the use of METEM has been shown to address this endogeneity issue (Gebremariam & Wünscher, Citation2016). METEM was therefore adopted to analyze the impact of mobile phone usage on farm income.
2.3. Multinomial endogenous treatment effect model
A multinomial endogenous treatment effect model was employed, first, to identify the factors influencing mobile phone access and usage for agricultural purposes and, second, to assess the effects of mobile phone access and usage on household crop income in Ghana.
The model involves a two-step approach where a household chooses to belong to a group of mobile phone users. Following Deb and Trivedi (Citation2006a, Citation2006b), we assume to be a latent variable capturing the group of mobile phone users j (j = 0, 1 … J), instead of belonging to alternative mobile phone user’s group k. Assuming that the subscript i refers to the participants (households), j is the treatment group, and k is the control group, the specification of the latent variable is given below as:
where is a set of exogenous variables that influence the decision to choose to belong to a mobile phone user group and the outcome under consideration,
is the resultant parametric constants,
are the error terms, and
is the latent variable that incorporates the unobserved characteristics common to the household choosing a mobile phone user group and the outcome variable (crop income). Following Deb and Trivedi (Citation2006b), let j = 0 represent no mobile phone access and usage (A0U0) and
. While
is not observed, the group of mobile phone users is observed in the form of a set of binary variables which are represented by a vector,
. Similarly, let
. The probability of treatment is given as:
where g denotes an appropriate multinomial probability distribution. Following Deb and Trivedi (Citation2006b), the study hypothesizes that g has a mixed multinomial logit (MMNL) structure defined as:
In the second stage, we examine the effect of mobile phone usage on the outcome variable, that is, the natural logarithm of household crop income. The expected outcome equation is formulated as follows:
In EquationEquation 4(4)
(4) ,
is the crop income for household
represents exogenous covariates with parameter
.
represents the treatment effects relative to households that did not have access and did not use mobile phones for agricultural purposes. Precisely, the coefficients
indicate the effect of belonging to a mobile phone user group on crop income of farming households. Since
is a function of latent factors
, the dependent variable is influenced by characteristics that are unobserved having the ability to also influence the selection of the dependent variable. It is also worth noting that when the factor-loading settings are set, treatment and outcome are associated through unobservable factor characteristics, where
is positive (negative), there is positive (negative) selection, in other words, as
and
being the associated parameter vectors, respectively (Manda et al., Citation2016). A normal (Gaussian) distribution function was used because the outcome variable is continuous. The Maximum Simulated Likelihood (MSL) approach was used to estimate the model.
In order to ensure robustness of the estimates, there is the need to include at least one instrumental variable as opined by Deb and Trivedi (Citation2006a). In testing for the validity of an instrument, an instrument is valid if it affects the decision of belonging to mobile phone user group’s equations but does not affect the outcome variable among the non-adopting sub-samples (DiFalco & Veronesi, Citation2013; DiFalco et al., Citation2011). Hence, for this study, access to electricity had an effect on the adoption variable (mobile phone users) but had no effect on the outcome variable (crop income). Access to electricity was used as an exclusion variable or instrument because a household with electricity access will have the motivation to have access and use mobile phones unlike those without electricity as this will be a deterring factor for these households to own and use mobile phones.
Gebremariam and Wünscher (Citation2016) and Deb and Trivedi (Citation2006a) also added that, after model estimation, an exogeneity test is carried out on the treatment equation to ascertain if the instrument used in the model specification is appropriate. The data were identified to be endogenous as shown by the significance of lambda in the results in Table . In the estimation, if at least one of the lambdas turns out to be significant, there is an element of endogeneity, which makes the model appropriate for the estimation.
3. Results and discussions
3.1. Summary statistics of household socioeconomic variables
Table shows the distribution of households based on the three identified dependent variables, namely, households who did not own a phone, those who had access to phone but did not use it for agricultural purposes, and households that had access to phones and used their phones for agricultural purposes. Relatively, few households (i.e., about 5%) use their phones for agricultural purposes, while the majority of households (88%) did not use their phones for agricultural purposes. About 7% of households did not own a mobile phone.
Table 1. Distribution of mobile phone access and usage
Table presents the summary statistics of household specific, social, and economic variables postulated to have an influence on e-Agriculture. The results showed that, on average, there were more male household heads accounting for 80% of the total sample. Also, about 98% of the household heads were married. The study found that 57.6% of the household heads had formal education indicating that, on average, over half of the household heads in the study area could read and write. The ability to read and write is expected to aid in mobile phone usage in terms of sending and receiving information on agriculture. The data show that on average, more than half (68.4%) of households in the study area had access to electricity. This is expected to enhance mobile phone access and usage. The study was undertaken in the Upper West and Central Regions with 62.1% of the respondents coming from the Upper West Region.
Table 2. Summary statistics of household socioeconomic variables
The cost of airtime is an important component of mobile phone usage, either for messaging or making and receiving of calls. On average, households in the study area spent GH¢ 31.66 on airtime per month. About 81% of the households owned livestock, which provides an additional income source for farm households in rural areas. Livestock ownership as a source of income can motivate the use of mobile phones for contacting and receiving advice from veterinary and extension officers. On the average, 9.1% of the households used manure on their farms, while 36.7% used inorganic fertilizers. Through mobile phone usage, farm households can be on their farms and contact prospective dealers of manure and inorganic fertilizers, thus saving time and reducing transaction costs. Credit is another important component when it comes to agriculture. On the average, 52.6% of farm households accessed credit for their production activities. The use of mobile phones for credit purposes is gaining prominence in recent times, and this is anticipated to improve agricultural production and farm income. By means of a mobile phone, farm households can easily obtain information about credit, interest rates, and microfinance institutions in their locality thus deepening financial inclusion and credit access.
3.2. Factors influencing mobile phone use for agricultural and non-agricultural purposes
Table provides the estimates of the first stage of the METEM, which reports the determinants of mobile phone access as well as the determinants of mobile access and usage for agricultural purposes. For this study, “mobile phone access” refers to having a mobile phone but not using it for agricultural purposes, while “mobile phone access and usage” refers to owning a mobile phone and using it for agricultural purposes. The study identified that airtime used per month, credit, region, and access to electricity influenced farming households’ access to mobile phones (or non-agricultural usage of phones), while, gender, education, cost of airtime used per month, livestock ownership, region (location), and access to electricity influenced the usage of mobile phones for agricultural purposes.
Table 3. Determinants of mobile phone use for agricultural and non-agricultural purposes
As shown in Table , gender of the household head indicates whether the head of the family as at the time of the interview was male or female. Gender of household head was positive and significant for households’ usage of mobile phones for agricultural purposes. This implies that male household heads have a higher probability of using mobile phones for agricultural purposes compared to females. The reason could be that most farm households in Ghana are characterized by male household heads who control most of the household resources. This is consistent with the findings of Nyamba and Mlozi (Citation2012) which indicated that the gender of a respondent significantly influenced mobile phone ownership in Tanzania. Aminou et al. (Citation2018) and Quandt et al. (Citation2020) also had similar results among maize farmers in Benin and Tanzania, respectively.
Educational status of household head indicates whether a household head had formal education. Educational status was significant and positive in its influence on mobile phone usage for agricultural purposes. This implies that farmers who can read and write have a higher likelihood to use their mobile phones for agricultural activities in line with general expectations. The reason for this is that formal education aids in reading and writing which is essential in the operation of mobile phones for various activities vital for agricultural production. The results agree with Issahaku et al. (Citation2017), who found education to have a positive influence on mobile phone ownership and usage among maize producers in Ghana. Nyamba and Mlozi (Citation2012) found the level of education to have a positive but insignificant effect on mobile phone ownership and usage in Tanzania.
The cost of airtime used per month positively influenced both agricultural and non-agricultural usage of mobile phones at 1% significant level. This result was expected and makes economic sense because, as a person uses the device, there will be the need to buy airtime for making calls, for sending of text messages, or for browsing the internet. Hence, the cost of airtime is anticipated to correlate positively with mobile phone usage. A similar result was obtained by Akinola (Citation2017) among farmers in Nigeria.
Farming households that own livestock showed a significantly higher probability of using their phones for agricultural purposes in Ghana. This is because livestock owners need information on vaccination, treatment, and safety of their animals which may be communicated to them by veterinary officers by means of mobile phones. As indicated by Karimuribo et al. (Citation2016), mobile phones in Tanzania are currently utilized to support livestock production through exchanging information on animal health, livestock market information, and livestock extension and advisory services. Livestock ownership also provides income to rural households, thereby enhancing access and usage of mobile phones.
Access to credit only influenced household access to mobile phones but not its usage for agricultural purposes. This result was not expected but could be due to the fact that one does not necessarily need a mobile phone in order to access credit from financial institutions. Asif et al. (Citation2017) found out that there was no correlation between the use of mobile phones by farmers in getting messages on vegetable cultivation and credit in Bangladesh, which is in line with this study’s finding.
The regional dummy variable was negative and statistically significant for both agricultural and non-agricultural usage of mobile phones at 1% significant level. This implies that households in the Upper West Region were less likely to have access to mobile phones nor use them for agricultural purposes. This coincides with a prior expectation of the study, as people in the southern part of Ghana are more exposed to information and communication technologies as compared to those in the northern part of the country (National Communications Authority & Ghana Statistical Service, Citation2020).
Access to electricity had a significant and positive influence on both agricultural and non-agricultural use of mobile phones at 1% significant level. Thus, households with access to electricity are more likely to have access to (or own) mobile phones as well as use them for agricultural purposes. This is because these households will have electricity to recharge their mobile phones once the batteries run down serving as a motivation to use mobile phones for farming and other purposes. Issahaku et al. (Citation2017) found a similar trend among smallholder maize farmers in Ghana. According to Nyamba and Mlozi (Citation2012), lack of electricity is one of the major constraints impeding ownership and use of mobile phones for agricultural information in Tanzania. Asif et al. (Citation2017) also observed that lack of electricity access was a major constraint to the use of mobile phones in receiving information on vegetable farming in Bangladesh.
3.3. Factors influencing crop income
Crop income as used in this study was operationalized as total net income from crops and crop products produced during the major cropping season. The study used the log form of crop income in the estimation. The results from Table showed that gender had a positive and significant influence on crop income. This implies that male-headed households had a higher likelihood to increase crop income by 9.8% compared to their female counterparts. This suggests that male-headed households are more likely to increase crop income due to the level of control in resources for productions such as land, farm machinery, and new technologies. Oyakhilomen (Citation2014) found gender to have a positive and significant influence on the income of maize farmers in Kaduna, Nigeria. Issahaku et al. (Citation2017), Aminou et al. (Citation2018) and Quandt et al. (Citation2020) all had similar results in their respective studies in Ghana, Benin, and Tanzania among maize farmers.
Table 4. Factors influencing crop income of farm households
Contrary to expectations, education was found to negatively influence crop income. This implies that household heads who can read and write will decrease crop income by 1.7%. The reason for this could be that educated farmers usually engage in non-farm activities which may be along the agricultural value chain that generate more income than that from the farm.
The cost of airtime used per month was significant and positively related to crop income at 1% significant level. This implies that households with higher income are associated with higher spending on airtime. A likely reason for this result is that higher airtime cost implies that farmers use their mobile phones more frequently which may include agricultural usage and therefore may receive more quality agricultural information to positively influence their farm earnings. In addition, these farmers perhaps might have subscribed to “esoko” agricultural services, a mobile telephony organization that provides timely and vital agricultural production and market information to farmers and links them to available markets at a fee via their mobile phone. These farmers utilized that information to increase output as well as used their market information to enable them to sell their output at a higher price at those available markets, hence contributing to higher crop income.
Also, households that own livestock increased their crop income by 5.7%. This makes economic sense as these households could use mobile phones in their livestock transactions such as buying feed, accessing veterinary services, and sales, among others, which is likely to save time and reduce transaction costs. According to Neudert et al. (Citation2020), household incomes (crop income included) correlated positively with land and livestock assets of households in a study in Azerbaijan and Georgia.
Manure application and use of inorganic fertilizer were both significant and positively influenced crop income, both at 1% significant level. This implies that households who may have larger farm sizes and make higher crop income will be more likely to increase manure applications and afford inorganic fertilizer. The reason for this could be that farmers use their devices to purchase manure and inorganic fertilizers at the best possible prices through the internet and other retail outlets and agents thus reducing transaction costs and saving time. According to Teenstra et al. (Citation2016), farm income is the difference between cost savings and benefits and this can be influenced by integrated manure management while saving on synthetic fertilizer.
Households in the Upper West Region increased crop income by 13.4% relative their counterparts in the Central Region. It means that these households witnessed more gains from mobile phone usage than households in the central region, Ceteris Parisbus. This could be as a result of efficient and effective use of mobile phones for agricultural purposes such as contacting prospective buyers of their produce and input dealers, among others.
3.4. Effect of mobile phone access and usage on crop income
The study also identified the effect of mobile phone access and usage on crop income. In other words, we are looking at the income effect of having a mobile phone and using it for non-agricultural purposes vis-à-vis having a mobile phone and using it for agricultural purposes. While farmers who use their mobile phones for agricultural purposes are expected to also use these devices for non-agricultural purposes such as surfing the internet, making telephone calls to friends, and sending friendly chats via WhatsApp, among others, the focus is to identify farmers who put their mobile phones to agricultural use and the associated income benefit.
Results from Table shows that the use of mobile phones for both agricultural and non-agricultural purposes had a significant and positive effect on crop income of farm households in Ghana. In particular, households that used their mobile phones solely for non-agricultural purposes increased crop income by 4.1%, while those that use their mobile phones for agricultural purposes increased their crop income by 7.2%. The positive impact of mobile phone usage on crop income could be attributed to some indirect or unintended benefits, such as assessing beneficial information on agriculture and agribusiness from telecommunication service providers. This could be through bulk messaging requested by projects for farmers in specific regions that creates incentives for investment in the farm.
Table 5. Effect of phone access and usage on crop income
It must, however, be noted that households that used mobile phones for agricultural purposes witnessed an extra 3.1% increase in crop income than those who only used mobile phones for non-agricultural purposes holding other factors constant. The reason for this outcome could be associated with the timely attainment of information from agricultural extension agents (AEAs). This is consistent with the findings of Nyamba and Mlozi (Citation2012) which showed that crop income in Tanzania significantly increased as a result of the use of mobile phones by farmers.
4. Conclusions and recommendations
The purpose of the study was to identify the factors influencing household access to and usage of mobile phones for both agricultural and non-agricultural purposes, as well as the effect of mobile phone usage on crop income of farm households in Ghana. Using data from the Global System for Mobile Communications (GSMA) covering 2,662 respondents and employing the multinomial endogenous treatment effect model, the study found that household usage of mobile phones for non-agricultural purposes (referred to simply as “access”) was significantly influenced by the amount of airtime used per month, access to credit, location, and electricity access. Meanwhile, male-headed households, educational status, amount of airtime used per month, livestock ownership, location, and access to electricity influenced household usage of mobile phones for agricultural purposes. Household crop income was found to be influenced by gender, educational status, amount of airtime used per month, livestock ownership, the use of inorganic fertilizer, manure application, and location.
The study thus recommends that to advance e-Agriculture through the use of mobile phones, government should partner with telecommunication companies to ensure that telecommunication network is made available in all rural communities in the country in order to encourage mobile phone usage. Second, the National Communication Authority (NCA) should partner with Chamber of Telecommunication companies to reduce taxes on airtime to encourage farmers’ use of mobile phones to promote e-Agriculture in Ghana. These measures will contribute to increase farm productivity and crop income of farm households. Meanwhile, extension agents should adapt to the use of mobile phones as part of the mechanisms in rendering extension delivery in order to reach more farmers and increase the extension agent-to-farmer ratio.
Acknowledgments
The authors thank the International Food Policy Research Institute (IFPRI) for making the data for this study available to them to carry out the research.
Disclosure statement
No potential conflict of interest was reported by the authors.
Additional information
Funding
Notes on contributors
Kamaru Abubakari
Kamaru Abubakari has backgrounds in agribusiness, agricultural economics and international development studies. He acquired his undergraduate and postgraduate degrees at the University for Development Studies. As part of his postgraduate studies, he participated in an exchange program at Utrecht University in the Netherlands. He is currently a freelance researcher as he continues to grow in the field of agriculture development, climate change and rural development.
Gilbert Dagunga
Gilbert Dagunga is a Tutor at the St. John Bosco College of Education, Navrongo, Ghana. He holds a master of philosophy degree in agricultural economics from the the University for Development Studies, Ghana. His research interest is in the area of sustainable food systems, rural poverty and economics of agricultural technology.
Benjamin Tetteh Anang
Benjamjn Tetteh Anang has taught and conducted research at the University for Development Studies for several years. His research focuses on agricultural productivity and efficiency analysis, impact of technology adoption, among others. He has published over 50 peered reviewed papers in both local and international academic journals
Mawuli Yevu
Mawuli Yevu is an Agricultural Economist at the CSIR-Savanna Agricultural Research Institute. He received his Bachelor of Science (BSc) and Master of Philosophy (MPhil) (Agricultural Economics) degrees from the University of Ghana. He is currently a member of the Socio-economic Section of CSIR-SARI. His key research interests are in the areas of management of agribusiness and marketing, Soil fertility and Sustainable intensification, innovation technology adoption, efficiency, value-chain development, farming systems research, financial inclusion, gender, and impact evaluation.
Raymond Mwinwan Galyuon
Raymond Mwinwan Galyuon holds a Mphil. Agricultural Extension from the University of Ghana, and a Tutor at the St. John Bosco College of Education, Navrongo, Ghana. He is interested in research related to agricultural innovations and extension, impact assessment measures as well educational pedagogy.
References
- Abdul‐rahaman, A., & Abdulai, A. (2021). Mobile money adoption, input use, and farm output among smallholder rice farmers in Ghana. Agribusiness, 38(1), 1–13. https://doi.org/10.1002/agr.21721
- Akinola, A. A. (2017). Influence of socio-economic factors on farmers’ use of mobile phones for agricultural information in Nigeria. Library Philosophy and Practice, 1688, 1–14.
- Aminou, F. A. A., Houensou, D. A., & Hekponhoue, S. (2018). Effect of mobile phone ownership on agricultural productivity in Benin: The case of maize farmers. Journal of Economics, 6(4), 77–88.
- Asif, A. S., Uddin, M. N., Dev, D. S., & Miah, M. A. M. (2017). Factors affecting mobile phone usage by the farmers in receiving information on vegetable cultivation in Bangladesh. Journal of Agricultural Informatics, 8(2). https://doi.org/10.17700/jai.2017.8.2.376
- Awuor, F., Raburu, G., Onditi, A. A., & Rambim, D. (2016). Building e-agriculture framework in Kenya. Journal of Agricultural Informatics, 7(1), 75–93. https://doi.org/10.17700/jai.2016.7.1.244
- Canton, H. (2021). Food and Agriculture Organization of the United Nations—FAO. In The Europa directory of international organizations 2021 (pp. 297–305). Routledge.
- Deb, P., & Trivedi, P. K. (2006a). Maximum simulated likelihood estimation of a negative binomial regression model with multinomial endogenous treatment. The Stata Journal, 6(2), 246–255. https://doi.org/10.1177/1536867x0600600206
- Deb, P., & Trivedi, P. K. (2006b). Specification and simulated likelihood estimation of a non-normal treatment-outcome model with selection: Application to health care utilization. The Econometrics Journal, 9(2), 307–331. https://doi.org/10.1111/j.1368-423X.2006.00187.x
- DiFalco, S., & Veronesi, M. (2013). How can African agriculture adapt to climate change? A counterfactual analysis from Ethiopia. Land Economics, 89(4), 743–766. https://doi.org/10.3368/le.89.4.743
- DiFalco, S., Veronesi, M., & Yesuf, M. (2011). Does adaptation to climate change provide food security? A micro-perspective from Ethiopia. American Journal of Agricultural Economics, 93(3), 825–842. https://doi.org/10.1093/ajae/aar006
- Food and Agriculture Organization of the United Nations [FAO] and the International Telecommunication Union [ITU] [(2017) E-agriculture strategy guide. http://www.fao.org/asiapacific/resources/e-agriculture.
- Gebremariam, G., & Wünscher, T. (2016). Combining sustainable agricultural practices pays off: evidence on welfare effects from Northern Ghana. Invited Paper Presented at the 5th International Conference of the African Association of Agricultural Economists, September 23-26, 2016, 1–37.
- Ghana Statistical Service. (2021). Population and Housing Census: Provisional Results, September, 1–7. https://statsghana.gov.gh/gssmain/storage/img/infobank/2021PHCProvisionalResultsPressRelease.pdf
- Hoang, H. G. (2020). Determinants of the adoption of mobile phones for fruit marketing by Vietnamese farmers. World Development Perspectives, 17, 100178. https://doi.org/10.1016/j.wdp.2020.100178
- Issahaku, H., Abu, B. M., & Nkegbe, P. K. (2017). Does the use of mobile phones by smallholder maize farmers affect productivity in Ghana? Journal of African Business, 19(3), 302–322. https://doi.org/10.1080/15228916.2017.1416215
- Karimuribo, E. D., Batamuzi, E. K., Massawe, L. B., Silayo, R. S., Mgongo, F. O. K., Kimbita, E., & Wambura, R. M. (2016). Potential use of mobile phones in improving animal health service delivery in underserved rural areas: Experience from Kilosa and Gairo districts in Tanzania. BMC Veterinary Research, 12(1), 1–6. https://doi.org/10.1186/s12917-016-0860-z
- Khan, N. A., Qijie, G., Sertse, S. F., Nabi, M. N., & Khan, P. (2020). Farmers’ use of mobile phone-based farm advisory services in Punjab, Pakistan. Information Development, 36(3), 390–402. https://doi.org/10.1177/0266666919864126
- Manda, J., Alene, A. D., Gardebroek, C., Kassie, M., & Tembo, G. (2016). Adoption and impacts of sustainable agricultural practices on maize yields and incomes: Evidence from rural Zambia. Journal of Agricultural Economics, 67(1), 130–153. https://doi.org/10.1111/1477-9552.12127
- Ministry of Food and Agriculture [MoFA]. (2017). Planting for food and jobs. Strategic plan for implementation (2017-2020). MoFA.
- National Communications Authority & Ghana Statistical Service. (2020). Household survey on ICT in Ghana. Abridged Report, March 2020. Available at https://statsghana.gov.gh/gssmain/fileUpload/pressrelease/Household%20Survey%20on%20ICT%20in%20Ghana%20Abridged%20new%201.pdf.
- Neudert, R., Allahverdiyeva, N., Mammadov, N., Didebulidze, A., & Beckmann, V. (2020). Diversification of livestock-keeping smallholders in mountainous rural regions of Azerbaijan and Georgia. Land, 9(8), 1–23. https://doi.org/10.3390/LAND9080267
- Nwanma, V. (2021) Agriculture as oxygen for African economies, https://dailytrust.com/agriculture-as-oxygen-for-african-economies
- Nyamba, S. Y., & Mlozi, M. R. S. (2012). Factors Influencing the use of mobile phones in communicating agricultural information: A case of Kilolo District, Iringa. Tanzania, 2(7), 558–563.
- Nyarko, D. A., & Kozári, J. (2021). Information and communication technologies (ICTs) usage among agricultural extension officers and its impact on extension delivery in Ghana. Journal of the Saudi Society of Agricultural Sciences, 20(3), 164–172. https://doi.org/10.1016/j.jssas.2021.01.002
- Okediran, O. O., & Ganiyu, R. A. (2019). E-agriculture reviewed: Theories, concepts and trends. FUOYE Journal of Engineering and Technology, 4(1), 125–130. https://doi.org/10.46792/fuoyejet.v4i1.366
- Oyakhilomen, O. (2014). Gender influence on the income of maize farmers in Giwa Local Government area of Kaduna State. Russian Journal of Agricultural and Socio-Economic Sciences, 25(1), 14–18. https://doi.org/10.18551/rjoas.2014-01.03
- Quandt, A., Salerno, J. D., Neff, J. C., Baird, T. D., Herrick, J. E., McCabe, J. T., Xu, E., & Hartter, J. (2020). Mobile phone use is associated with higher smallholder agricultural productivity in Tanzania, East Africa. PloS One, 15(8), e0237337. https://doi.org/10.1371/journal.pone.0237337
- Teenstra, E., Andeweg, K., & Vellinga, T. (2016). Manure helps feed the world: Integrated manure management demonstrates manure is a valuable resource, livestock research. Wageningen University and Research.