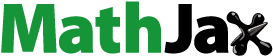
Abstract
With global climatic shifts and increase in greenhouse gases (GHG) emissions, agriculture is a contributor, but it is largely affected by the effects, particularly affecting the smallholder farmers. Climate-smart agricultural (CSA) practices provide the best solution for adapting to climate change and variability. Moreover, some CSA practices act as carbon sinks, thereby helping reduce GHG build-up in the atmosphere. These practices may include conservation agriculture, agroforestry, water conservation, use of tolerant varieties and breeds, as well as the use of risk insurance. Despite the highlighted benefits, the uptake of CSA practices among smallholder sorghum farmers in Busia county is still low. From the foregoing, this study sought to evaluate factors affecting the level of uptake of CSA practices among smallholder sorghum farmers in Busia County. With the aid of a semi-structured questionnaire, primary data were collected from 423 smallholder sorghum farmers selected through multistage sampling technique. Data were analysed using the standard Poisson regression model. The average number of CSA practices adopted by sorghum farmers in the past one year was two, with a minimum of zero and a maximum of thirteen. Age of the farmer, farming experience, ownership and the proportion of land under farming, access to credit, frequency of receiving agrometeorological information, trust in the information, number of farmers’ trainings attended, and exposure to climate shocks, significantly influenced CSA practices uptake level. Findings give insights on provision of agrometeorological information and trainings as possible ways to encourage the uptake of CSA practices at the small farm level.
1. Introduction
Climate change is a threat to food security that many regions face, with countries in Sub-Saharan Africa (SSA), Kenya included, being among the most affected due to inadequate capacity to adapt to climate change and variability (Wekesa et al., Citation2018). Climate change and variability shifts climatic patterns, making it increasingly unpredictable and hence difficult for farmers to plan their production. Smallholder farmers, particularly in the arid and semi-arid land (ASALs) using the traditional agricultural production system, are the most affected by climate variability due to their overdependence on rain-fed agriculture, compounded with limited adaptive capacity (Kalele et al., Citation2021). Climate change shocks; erratic rains, unpredictable rainfall patterns, rising temperature, frequent droughts, increased snowing, and thawing are currently more common, further affecting the agricultural sector, especially smallholder farmers (Mogaka et al., Citation2021).
Considering the prevailing environmental challenges, coupled with climate change and global emission of greenhouse gas (GHG), CSA practices are highly touted as potential and efficient solutions to these problems to sustain effective food production. CSA practices build smallholder farmers’ adaptive capacity for resilience to climate change, thus protecting their source of livelihood and ensuring food security (Wekesa et al., Citation2018). These practices involve effective management and use of natural resources and adopting sustainable and effective farming methods and technologies that ensure optimum production, processing, and marketability of smallholder farmers’ produce. For instance, improved breeds, use of organic manure, and improved postharvest handling improve the quality of products, leading to higher marketability and improved prices for the produce. Agroforestry reduces smallholder farmers’ vulnerability to climate change by acting as carbon sinks and protecting soil from erosion, contributing to sustainable agricultural production (Beyene et al., Citation2019). Organic farming and mulching enhance soil structure, soil moisture conservation, and carbon sequestration. Moreover, intercropping ensures efficient land utilisation, reducing the possibility of total crop failure due to climate variability (Mogaka et al., Citation2021). Other CSA practices include integrated pest management, cover cropping, integrated crop-animal agriculture, traditional organic farming, crop rotation, and diversification (Mazhar et al., Citation2021).
Farmers’ choice of CSA practices depends on their socio-economic characteristics (perception, awareness, affordability, ease of implementation, etc.), land characteristics/topography, and institutional factors (Kalele et al., Citation2021; Mutunga et al., Citation2018). Literature reveals extensive studies on factors influencing the choice of CSA practices among farmers (Talanow et al., Citation2021). For instance, age of farming decision-maker, size of land under farming, land tenure, and distance from the farm to the market significantly influenced the choice of CSA practice by farmers (Beyene et al., Citation2019). Additionally, education level, household income, farming experience, and access to extension services were among the factors influencing adoption of CSA practices (Mogaka et al., Citation2021). The adoption levels of CSA practices vary across regions and farmers’ productive capacity, ranging from small- to large-scale producers.
CSA practices offers an efficient, reliable, and sustainable means to achieve resilience and reduce environmental degradation (Food and Agriculture Organization, Citation2010). CSA builds resilience to climate variability and reduces greenhouse gases, which promotes sustainable increase in agricultural productivity and incomes (Food and Agriculture Organization, Citation2013). According to Sapkota et al. (Citation2015), Conservation Agriculture (CA)-based system reduces production cost by up to 23% while maintaining or yielding more than the conventional system. A study by Khatri-Chhetri et al. (Citation2016) found that greater yields were achieved by farmers after implementation of CSA practices in rice and wheat crops with the use of improved seeds, zero tillage, and laser land levelling, increasing total production by 19%, 6%, and 610% respectively. There was also substantial reduction in input costs – by up to 41% – under the zero tillage practice. CSA prioritizes mitigating climate variability in order to improve food security (Lipper et al., Citation2014).
Effects of climate variability threaten nations worldwide, with poor countries more vulnerable because of overdependence on rain-fed agriculture, low adaptive capacity, and heavy reliance on agriculture, which is vulnerable to climate variability. In East Africa, agricultural sector has been adversely affected by climate variability, and the effect is projected to get worse in future (Aryal et al., Citation2021). In Kenya, the evidence of climate variability is unmistakable, with increased extreme events – temperatures rising throughout the country and rainfall becoming irregular and unpredictable. These unprecedented events have led to heavy economical losses to the country, with evidence indicating damages equivalent to 2.4% of the Gross Domestic Product (GDP) resulting from drought between 1999 and 2000 (GOK, Citation2010).
Like the rest of the country, Busia County has experienced the effects of climate variability. Climate variability in the county is quite evident, with serious effects on crops and livestock production (GOK, Citation2010) leading to higher poverty levels (64.2%) than the national average of 45.9% (Lukano, Citation2013). According to A. S. Mugure (Citation2017), majority of farmers in Busia County have noticed long-term changes in temperature and rainfall and the county continues to suffer low agricultural productivity due to declining soil fertility, and extreme climate events, with historical data indicating that drought frequency has increased from every 10 years to every 2–5 years. This has led to loss of quality and quantity of natural biodiversity, soil erosion, and flooding. These are experienced due to varying rainfall patterns, affecting both land preparation and food production, leading to lower yields across all crops (Wekesa et al., Citation2018). Consequently, the county government of Busia, in collaboration with other organizations like ICRAF and NEMA, is working to promote the implementation of CSA strategies to cope with climate variability.
Despite farmers being resilient to climate change variability, many, especially smallholder producers, lag behind in adapting to CSA practices effectively. Their CSA adoption levels (number of practices adopted by farmers) remain relatively low. This could be attributed to economic challenges (inflation) and social imbalances, as mostly it is the women who farm the land, but do not have the ownership rights to the land; hence are constrained in the implementation of some CSA practices. In addition, low institutional support makes them vulnerable to climate change. Therefore, smallholder farmers might adopt none, one, or more CSA practices, based on their ability. Moreover, there is inadequate knowledge of how smallholder sorghum farmers in Busia county perceive CSA practices for adoption, due to the shock of climate change and variability.
In this regard, this study seeks to address the highlighted knowledge gap by assessing factors affecting the level of uptake of CSA practices among smallholder sorghum farmers in Busia County. Farmers’ choice of CSA practices affects their planning, investment, adoption, and implementation for optimum results on their farms. The study results could inform relevant policy formulation bodies to enhance smallholder sorghum farmers’ resilience to climate change.
2. Materials and methods
2.1. Study area and sampling design
The study was conducted in Busia County, which lies between latitude 0º and 0º 45 North and longitude 34º 25 East, and covers an area of approximately 1,695 km squared (KNBS, Citation2013). Busia County is on the western side of Kenya. It has a mean temperature of about 21–27 degrees Celsius and an annual rainfall of about 750-2000 mm (Wanjala, Citation2018). The area receives bimodal rainfall patterns; the long rains come between March and May (First season) and the short rains between August and October (Second season). The county is at an altitude of 1,140–1,500 meters above sea level; it is therefore suitable for crop farming. The county experiences long rain season, which is at its peak between late March and late May, while the short rains fall between August and October. The county has prolonged dry spells, with scattered rains from December to February. Some of the main crops farmed in Busia county include cassava, sorghum, maize, beans, groundnuts, sugar cane, vegetables, roots, and tubers. Livestock kept include goats, sheep, cattle, and poultry, while fish farming is also practised in some areas of the County (Ng’endo et al., Citation2018).
Busia county is among the high sorghum production counties in Kenya, and is farmed mainly by smallholder farmers, with land sizes measuring between 1–2 acres. The county is among the regions highly affected by climate change, making the choice of sorghum as a drought-resistant crop among farmers in the area; the area under production increased by 71% between 2012–2014 (Githinji et al., Citation2020). Busia county has seven (7) sub-counties. However, the county has experienced environmental degradation due to soil erosion and flooding, which pose threat to food production potential. Fluctuating rainfall patterns also affect agricultural production patterns, leading to lower yields. There is a remarkable decline in water volumes in rivers, 23 wells, pans, and springs, with the average distance to watering point averaging at 1.5 km (Wekesa et al., Citation2018)
The study used a multistage sampling technique; first, Busia county was selected consciously because it is vulnerable to climate change and variability. In the second stage, the four sub-counties (Teso South, Teso North, Matayos, and Samia) were intentionally chosen among the seven sub-counties because they are the main sorghum producing areas within the county (BCIDP, Citation2018; Nyongesa et al., Citation2017). In the third and last stage, sorghum farmers were selected using a systematic sampling procedure in each sub-county, where households were picked from a list of sorghum farmers; this list was generated during a pre-visit. Sorghum farmers were prequalified using land size criteria (smallholder farmers) and the period for which they have been practicing sorghum farming.
Since the exact population of sorghum farmers is unknown, the desired sample size was derived from Cochran (Citation2007) approach, as shown in EquationEquation 1(1)
(1) .
where; n = sample size; Z= confidence level (α = 0.05); p = proportion of the population containing the major interest; while q = 1-p; and E= allowable error. Since the proportion of the population is not known, p = 0.5, q = 1–0.5 = 0.5, Z = 1.96 and E (allowable error) = 0.05 because the study allows a 95% confidence level.
The sample size in EquationEquation 2(2)
(2) was upwardly adjusted by 10% to ensure that the minimum required sample is retained, even after dropping some of the uncooperative respondents or because of erors found during the data cleaning stage; therefore, the total sample of interest was 423 sorghum farming households. The 423 households were distributed across the four selected sub-counties, proportionate to the population size per sub-county, using the list of farmers from sub-county agricultural offices, as shown in Table ;
Table 1. Sampling size distribution per sub-county
The study collected primary data from smallholder sorghum farmers using a semi-structured questionnaire administered by well-trained enumerators. A pilot study was done to ascertain the feasibility of the digitised data collection tool, which was installed in mobile phones as an open data kit (ODK) application. The collected data was then entered into STATA software for analysis.
2.2. Econometric estimation of factors influencing the level of uptake of climate-smart agricultural practices
In this study, the uptake level of CSA practices was measured as the number of climate-smart farming practices applied by smallholder sorghum farmers to prevent climate shocks in the past one (1) year. Using the Poisson regression model, the study modelled the determinants of the uptake of CSA practices, since the number of climate-smart farming practices was a count outcome. Following Cameron and Trivedi (Citation2013), the observed number of CSA practices by the smallholder sorghum farmers, , conditioned by a vector of covariates Xi, is a count variable that can be specified as follows:
where, parameter is the conditional mean number of events for each explanatory variable. The parameter
is given by
where, is a
parameter vector, with the
parameter being the coefficient for the intercept and
are coefficients associated with
regressors.
The Poisson model was appropriate in estimating the association between the level of uptake of CSA practices by smallholder sorghum farmers and regressors. The Poisson model assumes that the count outcome variable’s conditional variance equals the conditional mean (Cameron & Trivedi, Citation2013). Extensions of the Poisson, such as the over-dispersed Poisson, negative binomial, and two-stage (hurdle) or zero-inflated models, could have been adopted appropriately in case the assumptions regarding the distribution of the data (in particular, when mean equals the variance) were not plausible. The assumption that the mean equals the variance is expressed as follows:
where is the level of uptake of CSA practices by the smallholder sorghum farmers (non-negative integer), Xi is a vector of the level of uptake of CSA practices by the smallholder sorghum farmers, and
is the conditional mean of the level of uptake of CSA practices.
The level of uptake of CSA practices by the smallholder sorghum farmers is a choice that is conditioned by individual preferences and other factors. As a result, it is expected that the number of times a smallholder sorghum farmer adopts CSA practices differ substantively.
The motives behind the uptake of CSA practices and the number of CSA practices to apply are highly complex, since it varies with the type of climatic shock. It is quite challenging to rationalize why one variable affects the uptake of CSA practices but not the level of uptake of CSA practices and vice versa. Therefore, the study assumes that all explanatory variables used in the equations for adoption and level of uptake of CSA practices are identical.
The variables to be included in the Poisson model to establish the determinants of the level of uptake of CSA practices were considered in correspondence with previous studies (Antwi-Agyei et al., Citation2021; Gbegeh & Akubuilo, Citation2013; Gbetibouo, Citation2009; Nuani et al., Citation2022; Teklewold et al., Citation2019).
3. Results and discussion
3.1. Descriptive results
Results in Table show descriptive and summary statistics of the survey. Majority of the respondents had an average of 49 years of age, with approximately nine years of education. Many sorghum farmers had spent almost half of their lifetime in farming, evidenced by an average of 22 years of farming experience. This is nearly a half of their average age. On average, many respondents had received at least 2 and 3 extension visits and agrometeorological trainings in the past 12 months. About 96% of smallholder farmers owned their farmland, with roughly 58% having access to credit. Busia is a vulnerable county to climate change; an absolute majority (91%) of the sorghum farmers reported to have experienced at least one of the climate change shocks; frequent droughts, frequent floods, or frequent erratic hailstorms. The distance from the farm to the nearest farm input and output (agricultural produce) market was approximately 3 km, therefore accessible to many smallholder sorghum farmers. Regarding the effect of climate change and adaptation mechanisms employed by farmers, a minor percentage of the farming households (38%) trusted the agrometeorological information services received from a wider climate change information dissemination pathway.
Table 2. Description of variables and descriptive statistics
The average number of CSA practices adopted by sorghum farmers in the past one year was two (Table ). The yearly maximum CSA practices used by smallholder sorghum farmers was 13, while the minimum was zero, implying that the variance in adoption levels could result from a lack of awareness and affordability of these practices, which agrees with Kalele et al. (Citation2021).
Table 3. Number of Climate-Smart Agricultural practices (CSA) applied in the past year
3.2. Determinants of level of uptake of climate-smart agricultural practices
Two count models were estimated consecutively to identify the one that best explains the determinants of the level of CSA practices among smallholder sorghum farmers in Busia county. The standard Poisson model was estimated first and the results are presented in Table .
Table 4. Standard poisson results on determinants of level of uptake of climate-smart agricultural practices
To establish the feasibility and applicability of the standard poison, a test for goodness of fit and a test for over-dispersion was done. The mean deviance and the Pearson chi-square ratio (the deviance and Pearson chi-square values divided by their degrees of freedom) were used to assess the degree of fit of the standard Poisson model, as shown below;
Deviance/df = 198.2907/405 = 0.4896
Pearson/df = 265.9239/405 = 0.6566
Where df refers to the degrees of freedom (405 in this case). These results for deviance (0.4896) and Pearson (0.6566) show that both ratios are significantly smaller than 1. Therefore, there is no evidence of the over-dispersion problem in the data. The NBR model was estimated for comparison and subsequent decision-making on the appropriate model (Table ). The NBR model results revealed that the likelihood ratio test was not significant, since alpha was less than zero (1.8e-05), implying the NBR model was not a significant improvement over the standard Poisson model. Moreover, the data did not have an over-dispersion problem, as revealed by the test for the existence of this problem in the model. The standard Poisson model fits the data well.
Using the estimates from the standard Poisson model (Table ), several socio-economic, institutional, and land characteristics variables significantly influenced the CSA practices uptake level among smallholder sorghum farmers of Busia County. Out of the seventeen independent variables, nine significantly influenced the number of CSA practices adopted by farmers. Four variables (age, proportion of land under farming, ownership of farmland, and access to credit facilities) negatively and significantly influenced the CSA counts adopted. The remaining five covariates (farming experience in years, number of agrometeorological information received, training counts attended by farmers, exposure to climate shocks, and if farmers trusted the agrometeorological information received) positively and significantly influenced the number of CSA practices employed by sorghum farmers in the past one year.
Age of the decision-maker had a significantly negative effect on the number of CSA practices used by sorghum farmers. Younger farmers are strong and energetic, and likely to adopt many CSA practices. On the contrary, ageing is connected to a reduction in physical ability, increased risk-aversion, and short-term planning. Older farmers are likely less proactive, and lack interest or strength (physical/mental ability) to learn new farming techniques; therefore, they would go for farming techniques they are familiar with or easier to implement. These findings are in line with the findings of (Macharia et al., Citation2014), who noted that older farmers are risk-averse, explaining the low potential of adopting multiple CSA practices. Similarly, Musafiri et al. (Citation2020) found that older farmers tend to trust traditional methods of agricultural practices more than other types, and probably miss out on the new agricultural innovations. This is likely to influence them to adopt fewer CSA practices. Contrarily, Challa and Tilahun (Citation2014) found out that age of farmers positively influenced the probability of adoption of climate variability-related technologies. This was associated with farming experience, which improves skills for better farming.
Increased farming experience is more likely to influence the number of CSA adopted by farmers. Possibly, many years of farming endows farmers with the relevant technical know-how of adapting to different environmental, weather, and climatic changes; therefore, they are likely to choose many CSA practices for various enterprises, depending on their perceived benefits. These findings corroborate with Mogaka et al. (Citation2021), who concluded that older farmers adopt multiple CSA practices, which is attributed to more skills and farming knowledge accrued over time.
Higher proportion of land allocated to farming was less likely to influence the number of CSA practices used by sorghum farmers. Implementation of CSA practices is laborious and costly. Despite farmers allocating a large proportion of their land to farming/agricultural production, the lack of enough capital to implement multiple CSA practices discourages their implementation. This is in line with the findings of (Autio et al., Citation2021), who found out that farmers are likely to choose fewer farming techniques that can be implemented within their budget constraint. In contrast, a study by Abegunde et al. (Citation2020) found farmers with larger farmlands were likely to adopt more CSA practices due to the availability of enough space to accommodate the adoption of multiple technologies.
Land ownership by title was less likely to influence the adoption of many CSA practices among sorghum farmers. The study was restricted to smallholder farmers, implying that majority had less than 3 acres of land allocated to farming. Therefore, the small size of the owned land under farming in the study area limits the number of CSA practices adopted by farmers. The survey revealed that majority of the farmers had very small land allocated for farming (less than one care); therefore, the more the number of farmers with small farmland, the fewer the uptake levels of CSA practices. Additionally, implementation of CSA needs resources beyond just owning the rights to land; these resources may not be available, hence curtailing the adoption of CSA. As against this, A. Mugure et al. (Citation2013) found that land ownership positively influenced the adoption of multiple CSA practices, to a larger extent due to land tenure security. This finding needs further research.
Access to credit facilities negatively affected the number of CSA practices implemented by farmers. Increased access to credit facilities increases business activity, which does not necessarily improve farm productivity. Literature on the adoption of multiple CSA practices reveals that the nature of the CSA practice or technology (cost of implementation), prevailing local circumstances, and farmers’ characteristics, such as honesty and integrity, would determine whether they put the extended credit into good use or probably divert it into unintended purposes (Bryan et al., Citation2013). If the latter is the case, they are more likely to adopt fewer CSA practices, even after getting the necessary credit facilities. Other farmers prefer using the credit extended to them on CSA practices that assure them high returns on investment; therefore they choose only a limited number of technologies to adopt (Wood et al., Citation2014). This is in contrast to the findings by Wekesa et al. (Citation2018), who indicated that farmers who received credit in the previous farming season were more likely to implement some CSA practices. However, he also found that credit access may discourage uptake of some CSA practices, especially if the credit is diverted to other uses.
The more the times a farmer receives agrometeorological information in a year, the more likely they adopt multiple CSA practices. Information is power; therefore, farming households that receive more agro-based information are likely to adopt many CSA practices to mitigate climate change (Musafiri et al., Citation2020). For instance, CSA practices have varying short-term and long-term benefits, depending on the type o CSA practice. Moreover, these practices can complement each other or act as a substitute. Farmers with agrometeorological information likely have the skills and knowledge to plan their farming activities by using multiple CSA practices that would lead to optimum productivity, which agrees with Qazlbash et al. (Citation2021).
The number of agricultural trainings received by a farmer in a year positively influenced the CSA practices uptake levels. Training farmers about new farming technologies promotes knowledge sharing and increases the awareness of CSA practices; therefore, it is more likely to enhance their adoption rate among smallholder farmers. This is in line with the theory of diffusion and adoption of innovations, which stipulates that the more the farmers are made aware of the existing and new farming technologies and their accompanying benefits, the more they adopt them (Rogers, Citation2010). Similarly, Ouédraogo et al. (Citation2019) found that more training on adopting CSA practices such as increased productivity, soil improvement, ability to increase income, and environmental conservation informed their enhanced uptake levels. This is in contrast with the findings of a study in Zambia by Arslan et al. (Citation2015), which indicated that extension agents were involved in a lot of activities that include delivering inputs and administering credit; hence farmers may question their skills, impacting their trust and the eventual decline in implementation.
Farmers who had been previously exposed to climate shocks were more likely to adopt multiple CSA practices. Climate change and variability have a significant bearing on the adoption strategies of CSA practices that are resilient to farmers, to cushion them against such effects/occurrences currently and in future. The smallholder farmers who received threats of climate shocks (erratic rains, increased temperatures, short rains, floods, and infestation of pests and diseases) were more likely to adopt many CSA practices such as crop diversification, planting drought-resistant crops, and mulching (Tanti et al., Citation2022). The choice of CSA practices and their uptake levels depend on the nature of shocks the farmer is exposed to – at the more the shocks, the more the practices adopted.
Trust in agrometeorological information services had a positive and significant effect on the uptake levels of CSA practices. The agro-based information received by farmers ought to be demand-driven, cost-effective, reliable, timely, accessible, easy to implement, and efficient. In this regard, the received information would help build trust among farmers. It enables them to plan efficiently, and effectively time their production; land preparation, planting, seed variety selection, weeding, fertiliser application, and harvesting (Djido et al., Citation2021). Discussions with the respondents revealed that agrometeorological information received by farmers had some payoffs. It guided them to choose CSA practices that had optimum results and trust them, similar to the findings by Ouedraogo et al. (Citation2018). However, they argued that information received on long-term CSA practices was more trusted than for the short-term CSA practices, as it helps compare the previous production with the current production, and forecast and plan for the future.
4. Conclusions and recommendations
Using the standard Poisson regression model, this study evaluated the level of uptake of CSA practices among smallholder sorghum farmers of Busia county, Kenya. Findings revealed that there is a generally low uptake of CSA practices due to the small sizes of the farming land owned by farmers in this study area and the age of farmers, with older farmers having a low uptake of CSA practices.
Access to more agrometeorological information improved the level of adoption of CSA practices, which is attributed to enhanced knowledge acquired from the information received by farmers. Similarly, farmers’ training promotes knowledge sharing, which equips farmers with the necessary skills to select efficient CSA practices that could assure them optimum benefits.
There is need for an agricultural policy that seeks to enhance smallholder farmers’ awareness of CSA practices, as also the various methods of appraising and selecting the farming technologies with high returns and the uptake level of these practices. This is likely to increase diversification in production, ultimately resulting in improved farmers’ welfare and contributing to food security through increased productivity. The study recommends regular enhanced trainings to equip farmers with necessary skills such as project appraisal using cost-benefit analysis, enabling them to invest in profitable CSA practices. Moreover, improved agrometeorological dissemination pathways are necessary for farmers to acquire the necessary skills and strategies to blend cost-effective technologies of both modern and traditional agricultural practices. For further research, this study recommends a similar future research that could consider farmers’ choice of CSA practices, based on their willingness to adopt them, which might affect their behavioural choices for these practices.
Authors’ contributions
G.O.A. formulated the research investigation under the supervision of E.O.G., and K.W.S. undertook raw data collection, data analysis and draft manuscript compilation. E.O.G. and K.W.S carried out research scrutiny and scientific validation. The manuscript results are the effort of all the authors, who have read and agreed to the published version of the manuscript.
Ethical approval
“All procedures performed in studies involving human participants were in accordance with the ethical standards of the institutional and/or national research committee and with the 1964 Helsinki declaration and its later amendments or comparable ethical standards”.
Acknowledgments
The authors acknowledge and are grateful for the research grant extended to the first author by the Kenya Climate-Smart Agriculture Project (KCSAP). The authors also extend gratitude to Egerton University for facilitating their training and the county agricultural officers in Busia county for linking the researchers with the farmers. Special thanks also go to the enumerators for a job well done and the respondents for their corporation during the study.
Disclosure statement
No potential conflict of interest was reported by the author(s).
Additional information
Funding
Notes on contributors
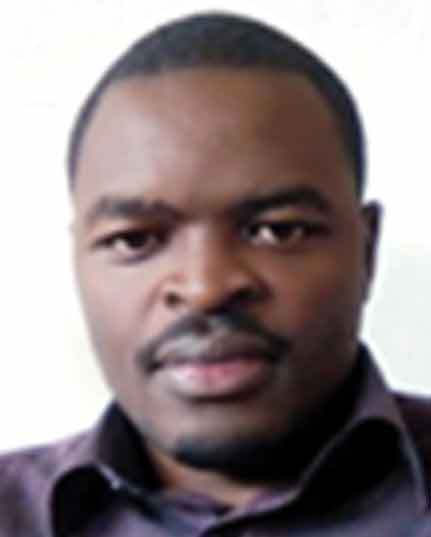
Godfrey Obwina Atsiaya
Godfrey Obwina Atsiaya is a PhD Candidate in Agricultural economics at Egerton University. He has over five years of experience in agriculture-based research, with areas of interest spanning from, climate-smart technologies, role of market innovation in inclusive and sustainable smallholder agriculture, transitioning agri-food systems in sub-Sahara Africa, agri-value chains development, sustainable rural development and livelihoods assessment. Atsiaya has done extensive work on climate variability adaptation, mitigation and adoption of technologies by smallholder farmers.
Eric Obedy Gido
Eric Obedy Gido is a Lecturer in the Department of Agricultural Economics and Agribusiness Management from Egerton University. He holds a PhD in Agricultural Economics and specializes in production Economics and Agricultural Markets.
Kenneth Waluse Sibiko
Kenneth Waluse Sibiko is a Postdoctoral Socialscientist at The Alliance of Bioversity International and CIAT. He holds a PhD in Agribusiness Management and specializes in consumer behaviour and willingness to pay.
References
- Abegunde, V. O., Sibanda, M., & Obi, A. (2020). Determinants of the adoption of climate-smart agricultural practices by smallholder farming households in King Cetshwayo District municipality, South Africa. Sustainability, 12(1), 195. https://doi.org/10.3390/su12010195
- Antwi-Agyei, P., Amanor, K., Hogarh, J. N., & Dougill, A. J. (2021). Predictors of access to and willingness to pay for climate information services in north-eastern Ghana: A gendered perspective. Environmental Development, 37, 100580. https://doi.org/10.1016/j.envdev.2020.100580
- Arslan, A., McCarthy, N., Lipper, L., Asfaw, S., Cattaneo, A., & Kokwe, M. (2015). Climate smart agriculture? Assessing the adaptation implications in Zambia. Journal of Agricultural Economics, 66(3), 753–13.
- Aryal, J. P., Sapkota, T. B., Rahut, D. B., Marenya, P., & Stirling, C. M. (2021). Climate risks and adaptation strategies of farmers in East Africa and South Asia. Scientific Reports, 11(1), 10489. https://doi.org/10.1038/s41598-021-89391-1
- Autio, A., Johansson, T., Motaroki, L., Minoia, P., & Pellikka, P. (2021). Constraints for adopting climate-smart agricultural practices among smallholder farmers in Southeast Kenya. Agricultural Systems, 194, 103284. https://doi.org/10.1016/j.agsy.2021.103284
- BCIDP. (2018). Busia County Integrated Development Plan 2018-2022.
- Beyene, A. D., Mekonnen, A., Randall, B., & Deribe, R. (2019). Household level determinants of agroforestry practices adoption in Rural Ethiopia. Forests, Trees and Livelihoods, 28(3), 194–213. https://doi.org/10.1080/14728028.2019.1620137
- Bryan, E., Ringler, C., Okoba, B., Roncoli, C., Silvestri, S., & Herrero, M. (2013). Adapting agriculture to climate change in Kenya: Household strategies and determinants. Journal of Environmental Management, 114, 26–35. https://doi.org/10.1016/j.jenvman.2012.10.036
- Cameron, A. C., & Trivedi, P. K. (2013). Regression analysis of count data (Vol. 53). Cambridge university press.
- Challa, M., & Tilahun, U. (2014). Determinants and impacts of modern agricultural technology adoption in West Wollega: The case of Gulliso District. Journal of Biology, Agriculture and Healthcare, 4(20), 2224–3208.
- Cochran, W. G. (2007). Sampling techniques. John Wiley and Sons.
- Djido, A., Zougmoré, R. B., Houessionon, P., Ouédraogo, M., Ouédraogo, I., & Seynabou Diouf, N. (2021). To what extent do weather and climate information services drive the adoption of climate-smart agriculture practices in Ghana? Climate Risk Management, 32, 100309. https://doi.org/10.1016/j.crm.2021.100309
- Food and Agriculture Organization. (2010). Climate smart agriculture: Policies, practices and financing for food security, adaptation and mitigation. FAO. https://www.fao.org/3/i1881e/i1881e00.pdf
- Food and Agriculture Organization. (2013). Multiple dimensions of food security. The state of food insecurity in the world. FAO. https://www.fao.org/publications/sofi/2013/en/
- Gbegeh, B. D., & Akubuilo, C. J. C. (2013). Adoption of selected improved agricultural technologies by farmers in Rivers State, Nigeria. International Journal of Innovative Research and Development, 2(1), 330–346.
- Gbetibouo, G. A. (2009). Understanding farmers’ perceptions and adaptations to climate change and variability: The case of the limpopo basin, South Africa (Vol. 849). Intl Food Policy Res Inst.
- Githinji, E. K., Irungu, L. W., Ndegwa, P. N., Machani, M. G., Amito, R. O., Kemei, B. J., Murima, P. N., Ombui, G. M., Wanjoya, A. K., Mbogo, C. M., & Mathenge, E. M. (2020). Impact of insecticide resistance on P. falciparum vectors’ biting, feeding, and resting behaviour in selected clusters in Teso North and South subcounties in Busia County, Western Kenya. Journal of Parasitology Research, 2020, 1–11. https://doi.org/10.1155/2020/9423682
- GOK. (2010). National climate change response strategy. Ministry of Environment and Mineral Resources.
- Kalele, D. N., Ogara, W. O., Oludhe, C., & Onono, J. O. (2021). Climate change impacts and relevance of smallholder farmers’ response in arid and semi-arid lands in Kenya. Scientific African, 12, e00814. https://doi.org/10.1016/j.sciaf.2021.e00814
- Khatri-Chhetri, A., Aryal, J. P., Sapkota, T. B., & Khurana, R. (2016). Economic benefits of climate smart agricultural practices to smallholders’ farmers in the Indo-Gangetic Plains of India. Current Science, 110(7), 1251–1256.
- KNBS. (2013). Exploring Kenya’s inequality; pulling apart or pulling together. Kenya National Bureau of Statistics.
- Lipper, L., Thornton, P., Campbell, B. M., Baedeker, T., Braimoh, A., Bwalya, M., Caron, P., Catteneo, A., Garrity, D., Henry, K., Hottle, R., Jackson, L., Jarvis, A., Kossam, F., Mann, W., McCarthy, N., Meybeck, A., Neufeld, H., Remington, T. … Torquebiau, E. F. (2014). Climate smart agriculture for food security. Nature and Climate Change, 4(12), 1068–1072. https://doi.org/10.1038/nclimate2437
- Lukano, L. S. (2013). Busia County integrated development plan, 2013–2017, ministry of planning and county development. County Government of Busia.
- Macharia, J., Mugwe, J., Mucheru, M. M., & Mugendi, D. (2014). Socioeconomic factors influencing levels of knowledge in soil fertility management in the central highlands of Kenya. Journal of Agricultural Science and Technology B, 4(9), 701–711.
- Mazhar, R., Ghafoor, A., Xuehao, B., & Wei, Z. (2021). Fostering sustainable agriculture: Do institutional factors impact the adoption of multiple climate-smart agricultural practices among new entry organic farmers in Pakistan? Journal of Cleaner Production, 283, 124620. https://doi.org/10.1016/j.jclepro.2020.124620
- Mogaka, B. O., Bett, H. K., & Ng’ang’a, S. K. (2021). Socioeconomic factors influencing the choice of climate-smart soil practices among farmers in western Kenya. Journal of Agriculture and Food Research, 5, 100168. https://doi.org/10.1016/j.jafr.2021.100168
- Mugure, A. S. (2017). Effects of climate change on rural livelihoods in Busia County. Kenya International Journal of Agriculture and Earth Science, 3(8), 2489.
- Mugure, A., Oino, P. G., & Sorre, B. M. (2013). Land ownership and its impact on adoption of agroforestry practices among rural households in Kenya: A case of Busia County. International Journal of Innovation and Applied Studies, 4(3), 552–559.
- Musafiri, C. M., Macharia, J. M., Ng’etich, O. K., Kiboi, M. N., Okeyo, J., Shisanya, C. A., Okwuosa, E. A., Mugendi, D. N., & Ngetich, F. K. (2020). Farming systems’ typologies analysis to inform agricultural greenhouse gas emissions potential from smallholder rain-fed farms in Kenya. Scientific African, 8, e00458. https://doi.org/10.1016/j.sciaf.2020.e00458
- Mutunga, E. J., Ndungu, C. K., & Muendo, P. (2018). Factors influencing smallholder farmers’ adaptation to climate variability in kitui county, Kenya. International Journal of Environmental Sciences, 8(5). IJESNR.MS.ID.555746
- Ng’endo, M., Bhagwat, S., & Keding, G. B. (2018). Challenges and opportunities for market integration to improve food security among smallholder farming households in Western Kenya. The International Journal of Sociology of Agriculture and Food, 24(2), 229–252.
- Nuani, F. O., Gido, E. O., Ayuya, O. I., & Musyoka, M. P. (2022). Consumer preference for selected roots and tubers among urban households. International Journal of Vegetable Science, 8(1), 1–14. https://doi.org/10.1080/23311932.2022.2093047
- Nyongesa, D., Esilaba, A. O., Emongor, R., Bikketi, E., & Were, K. (2017). Assessment of gender and innovations in climate-smart agriculture for food and nutrition security in Kenya: A case of kalii watershed. International Journal of Agricultural Resources, Governance and Ecology, 13(2), 109–137. https://doi.org/10.1504/IJARGE.2017.086434
- Ouedraogo, I., Diouf, N. S., Ouédraogo, M., Ndiaye, O., & Zougmoré, R. B. (2018). Closing the gap between climate information producers and users: Assessment of needs and uptake in Senegal. Climate, 6(1), 13. https://doi.org/10.3390/cli6010013
- Ouédraogo, M., Houessionon, P., Zougmoré, R. B., & Partey, S. T. (2019). Uptake of climate-smart agricultural technologies and practices: Actual and potential adoption rates in the climate-smart village site of mali. Sustainability, 11(17), 4710. https://doi.org/10.3390/su11174710
- Qazlbash, S. K., Zubair, M., Manzoor, S. A., Haq, A. U., & Baloch, M. S. (2021). Socioeconomic determinants of climate change adaptations in the flood-prone rural community of Indus Basin, Pakistan. Environmental Development, 37, 100603. https://doi.org/10.1016/j.envdev.2020.100603
- Rogers, E. M. (2010). Diffusion of innovations (4th Edition ed.). Simon and Schuster.
- Sapkota, T. B., Jat, M. L., Aryal, J. P., Jat, R. K., & Khatri-Chhetri, A. (2015). Climate change adaptation, greenhouse gas mitigation and economic profitability of conservation agriculture: Some examples from cereal systems of Indo-Gangetic Plains. Journal of Integrative Agriculture, 14(8), 1524–1533. https://doi.org/10.1016/S2095-3119(15)61093-0
- Talanow, K., Topp, E. N., Loos, J., & Martín-López, B. (2021). Farmers’ perceptions of climate change and adaptation strategies in South Africa’s Western Cape. Journal of Rural Studies, 81, 203–219. https://doi.org/10.1016/j.jrurstud.2020.10.026
- Tanti, P. C., Jena, P. R., Aryal, J. P., & Rahut, D. B. (2022). Role of institutional factors in climate‐smart technology adoption in agriculture: Evidence from an Eastern Indian state. Environmental Challenges, 7, 100498. https://doi.org/10.1016/j.envc.2022.100498
- Teklewold, H., Mekonnen, A., & Kohlin, G. (2019). Climate change adaptation: A study of multiple climate-smart practices in the Nile Basin of Ethiopia. Climate and Development, 11(2), 180–192. https://doi.org/10.1080/17565529.2018.1442801
- Wanjala, W. P. (2018). Path and stability analyses of yield and yield components [ PhD Thesis]. School of Agriculture and Enterprise Development, Kenyatta University.
- Wekesa, B. M., Ayuya, O. I., & Lagat, J. K. (2018). Effect of climate-smart agricultural practices on household food security in smallholder production systems: Micro-level evidence from Kenya. Agriculture and Food Security, 7(1), 80. https://doi.org/10.1186/s40066-018-0230-0
- Wood, S. A., Jina, A. S., Jain, M., Kristjanson, P., & DeFries, R. S. (2014). Smallholder farmer cropping decisions related to climate variability across multiple regions. Global Environmental Change, 25, 163–172. https://doi.org/10.1016/j.gloenvcha.2013.12.011
Appendix
Table A1. NBR model results on determinants of level of uptake of Climate-Smart Agricultural practices