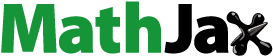
Abstract
Child undernutrition remains a global challenge, with 200 million under-fives suffering from wasting and stunting. Mothers’ access to nutrition and health-related information is critical for improving children’s dietary quality and nutrition outcomes. The use of information and communication technologies (ICT) in low- and middle-income countries is increasing and could improve mothers’ access to this information. This paper examines the effect of mother’s use of ICT on dietary quality and child undernutrition in a lower-middle-income country. The study uses a nationally representative sample of 10,385 children aged 6–59 months extracted from the Kenya Integrated Household Budget Survey 2015–2016. Dietary quality was measured using a child’s dietary diversity score (CDDS), while undernutrition was measured using underweight, wasting, and stunting levels. An instrumental variable approach was used to control for endogeneity of dietary quality and was executed using extended probit and extended ordered probit models. The study found that except for breast milk and dairy products, children of mothers who used ICT had significantly higher consumption of all food groups (P < 0.01). Children whose mothers used ICT had a higher CCDS and nutrient-dense food consumption (P < 0.01). Besides, the study found a significant (p < 0.01) positive effect of mothers’ use of ICT on the quality of children’s diets, with radio having the, most substantial effect. Dietary quality significantly (p < 0.01) and negatively affected the prevalence of being underweight and wasted. Children of mothers using ICT were found to have a significantly (p < 0.05) lower prevalence and severity of underweight and stunting, with the strongest effect resulting from mobile phone use. This research offers novel evidence to policymakers about the importance of incorporating ICT into policies and interventions for disseminating child nutritional and health information to mothers and caregivers in low- and middle-income countries.
1. Introduction
Attaining food and nutritional security is a global development challenge, with increasing human population, urbanization, globalization, inequalities and worsening climate change (FAO, IFAD, UNICEF, WFP, & WHO, Citation2019). International efforts to end all forms of childhood malnutrition include strategies to improve maternal, infant and young children’s nutrition; promote exclusive breastfeeding; universal health coverage; and transform food systems to offer healthy diets and market access (UNICEF, Citation2020). Despite these efforts, 200 million children under the age of five years suffer from wasting and stunting, and about 340 million suffer from hidden hunger, especially deficiencies in vitamins and minerals (UNICEF, Citation2019).
Undernutrition (stunting, underweight and wasting) contributes to the weak cognitive development and poor educational achievement of a child, which leads to low economic productivity and a higher risk of chronic illness and mortality later in life, and is responsible for about 45% of all global deaths of children under five years (WHO and UNICEF, Citation2019, Citation2020). The World Health Assembly in 2012 prioritized the reduction of stunting by 40% and wasting to less than 5% among the global targets for maternal, infant and young child nutrition to be achieved by 2025 (WHO and UNICEF, Citation2019, Citation2020). However, Africa lags behind, with notably high levels of stunting and other forms of undernutrition. Sub-Saharan Africa (SSA) bears the largest burden of child undernutrition globally, with statistics showing that the number of stunted children under five years increased by 6.5 million in West and Central Africa and by 1.4 million in East and South Africa, between 2000 and 2018 (UNICEF, Citation2019).
The determinants of undernutrition include a comprehensive range of environmental and socio-economic factors, dietary quality and safety, infant and child feeding practices, child-level characteristics and political factors (Akombi et al., Citation2017; Khamis et al., Citation2019; Matanda et al., Citation2014; Obasohan et al., Citation2020). Several studies have evaluated the link between dietary diversity and nutritional outcomes (Lowery et al., Citation2019; Temesgen et al., Citation2018). Evidence accentuates that access to information by primary caregivers is critical for good nutrition and general childcare. Hence, improved access to nutrition-related knowledge, especially by mothers and caregivers, is likely to be positively correlated with children’s nutrition outcomes (Fadare et al., Citation2019; Seyyedi et al., Citation2019). However, studies focusing on mothers’ access to childcare information and how this influences the dietary quality and nutritional status of under-fives are rare.
The use of information and communication technologies (ICT) and smartphone applications are promising innovative approaches to improve the nutrition and health outcomes of children in the underserved population in low-resource settings. For example, m-health communication innovations have been found to increase the effectiveness of nutrition interventions aimed at improving the intake of nutrient-rich foods such as fruits and vegetables or decreasing the intake of fat (Olson, Citation2016). Besides, the calculator of inadequate micronutrients (CIMI), a web-based application, has been shown to determine nutrient intake and micronutrient deficiencies in children and mothers in SSA (Desalegn et al., Citation2019). Recent evidence shows that mobile phone-based nutritional interventions overcome malnutrition challenges among preschoolers in developing countries (Seyyedi et al., Citation2019). In most rural and informal settlements in urban areas of SSA, the formal dissemination of nutrition knowledge and information is limited, and the use of ICT could offer great promise for knowledge dissemination.
Evidence suggests that the demand for and use of ICT is rapidly increasing in low- and middle-income countries. The increased use of ICT and digital platforms opens up a channel for two-way communication to share information about health and nutrition between health personnel and the general public (Manju & Kalpana, Citation2021). In these countries, however, there is minimal evidence linking mothers’ use of ICT to their children’s food quality and nutritional outcomes. Our study fills this literature gap by assessing the association of mothers’ ICT use with dietary quality and the undernutrition status of children aged 6–59 months in a lower middle-income country setting. The study employs nationally representative child-level data from Kenya. In this Sub-Saharan African country, undernutrition is still a problem among children under the age of five. The average prevalence of stunting is 26.2%, which is higher than the global average of 22.0%. The prevalence of wasting is 4.2%, which is lower than both global (6.7%) and Africa’s (6.0%) averages (Global Nutrition Report, Citation2021). These statistics show that much had to be done in order to accomplish the UN Sustainable Development Goals of achieving zero hunger and ending all types of malnutrition by 2030 (United Nations Ed., Citation2017). One critical strategy for fostering better nutritional outcomes for Kenyan children is to improve access to nutrition and childcare information by mothers and caregivers, mainly through ICT. This study focuses on mothers’ use of three ICT tools (mobile phones, television and radio) to answer the following questions: (1) Does mothers’ use of ICT effect child’s dietary quality? (2) Does mothers’ use of ICT affect child’s nutritional outcomes?
2. Materials and methods
2.1. Data and sampling design
The study uses data from a nationally representative dataset, the Kenya Integrated Household Budget Survey (KIHBS) 2015/2016, collected by the Kenya National Bureau of Statistics (KNBS). The data was collected from all 47 counties, in rural and urban domains. A three-stage stratified cluster sampling technique was used. Stratification was done using the rural–urban classification of the area of residence in each county, whereby all 47 counties had urban strata, and 45 had rural strata (Nairobi and Mombasa counties did not have rural strata since they are fully urban), making a total of 92 strata. In the first stage, 2400 clusters were sampled with equal probability, of which 988 clusters were in urban areas and 1,412 in rural areas. The second stage involved the selection of 16 households per cluster, from which further subsampling of 10 households was done in the third stage, resulting in a national sample of 24,000 households (Kenya National Bureau of Statistics, Citation2018). The dataset contains information on child-level characteristics, mothers’ characteristics, mothers’ ICT use, feeding practices, household socio-economic characteristics and environmental factors such as sanitation facilities. Republic of Kenya, (Citation2019), which ensures that data collection and dissemination procedures follow statutory requirements and are both legal and ethical. This study considered 11,462 children aged 6–59 months in the sample, but 1,077 children did not have complete information on anthropometric measurements. Hence, our final sample was 10,385 children who had valid and complete information on both dietary quality and anthropometric measurements. We excluded children below six months because their recommended diet is mainly breastmilk (WHO, Citation2021).
2.2. Measurement of key variables
2.2.1. ICT use
ICT use was measured by asking sampled households whether mothers had used ICT tools within the three months preceding the survey (regardless of whether they owned the tools or not). Capturing the use of ICT tools instead of possession of ICT makes the ICT variables exogenous. The tools considered were mobile phone, TV and radio. A dummy variable was created for each ICT tool, equal to one if the mother had used the tool and zero otherwise. The study assumed that the use of ICT tools by mothers would enhance information access and consequently increase nutritional and childcare knowledge that could help to minimize undernutrition in children.
2.2.2. Dietary quality of children
To measure children’s dietary quality, we followed the WHO Infant and Young Children Feeding (IYCF) guidelines (WHO & UNICEF, Citation2019). The study considered seven food groups: (i) grains, roots and tubers, (ii) legumes and nuts, (iii) dairy products, (iv) flesh foods, (v) eggs, (vi) vitamin A-rich fruits and vegetables and (vii) other fruits and vegetables. Breast milk was considered as a food group for children aged 6–23 months. The child diet was analyzed using a 24-h recall interview with the child’s mother or the primary caregiver who provided information on food items and liquids a child consumed during the previous day. If a child consumed a food item in a given food group, a value of one was assigned and a value of zero otherwise. The food scores were summed up to compute a child dietary diversity score (CDDS), for each child.
2.2.3. Child undernutrition
The study applied child anthropometric measurements as indicators of health outcomes: underweight, wasting and stunting, which are recommended for measuring the nutritional status of children (WHO, Citation2006). Hence, three types of Z-scores were computed and used as good pointers for nutritional outcomes. The first is the Weight-for-age Z-score (WAZ), which measured the degree of underweight of a child, and is recommended for examining short-term effects such as illness and inadequate food intake of children (WHO, Citation2006). The second is the Height-for-age Z-score (HAZ) that measures the degree of stunting and is an indicator of past or chronic undernutrition; while the third is the Weight-for-height Z-score (WHZ) that measures the degree of wasting, and identifies children suffering from current and acute undernutrition. The WAZ, HAZ and WHZ are indicators of whether the child’s actual weight or height is equal to the median for their age category and were used to categorize a child’s undernutrition severity. Scores greater than −1 indicate normal (no undernutrition) status and scores between −2 and −1 indicate marginal undernutrition. Scores between −3 and −2 indicate moderate undernutrition, while scores less than −3 indicate severe undernutrition (WHO, Citation2006; WHO & UNICEF, Citation2019).
2.3. Conceptual framework
The study uses the conceptual framework in Figure to show the effect pathways of mothers’ use of ICT on the dietary quality and undernutrition status of young children. Following the available body of literature, we hypothesized that child undernutrition is influenced by many factors, among them, mother’s characteristics, child characteristics and household characteristics (which we refer to as control variables1), dietary quality and a random error (Debela et al., Citation2020; Marinda et al., Citation2018). In addition, recent studies have shown that ICT could play a role in determining nutritional outcomes through access to nutritional education and knowledge that influences feeding practices, lifestyle changes and dietary choices (Billah et al., Citation2022; Fadare et al., Citation2019; Muange & Ngigi, Citation2021).
Figure 1. A representation of mother’s ICT use, child dietary quality and child undernutrition relationships.
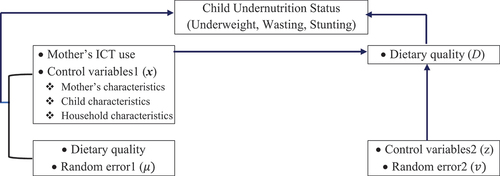
Dietary quality itself is a function of several variables, including those that influence child undernutrition (control variables1) as shown by Smith and Haddad (Citation2015), Machira et al. (Citation2020) and Aboagye et al. (Citation2021), as well as other variables that may not have a direct influence over child undernutrition except through dietary quality (control variables2). The use of ICT has been found to influence dietary quality. It is hypothesized that ICT use by mothers could determine the quality of diets offered to children by giving mothers child nutrition and feeding information.
2.4. Econometric framework
Following the conceptual framework above, we applied the regression approach, to model determinants of undernutrition using the following generic equation:
Table 3. Descriptive statistics for variables used in regression analysis (N = 10,385)
In estimating Equationequation (1)(1)
(1) , we considered
to be potentially endogenous. Studies have shown that dietary quality is influenced by several factors that are also correlated with nutritional and health outcomes (Debela et al., Citation2020; Marinda et al., Citation2018; Muange & Ngigi, Citation2021). Hence, it is highly likely that there are unobserved factors in
(omitted variables) that are correlated with both U and
and failing to account for this endogeneity would produce inconsistent parameter estimates (Wooldridge, Citation2016). We therefore used an instrumental variable (IV) approach in which dietary quality,
, was hypothesized to be influenced by several variables as follows:
We modeled undernutrition status, U, using two approaches: first as a binary variable measuring the presence or absence of undernutrition, and second, as a categorical variable, measuring the severity of undernutrition. As a binary variable, we used U = 1 to indicate that a child was undernourished (underweight, wasted or stunted) and U = 0, otherwise. On the other hand, as a categorical variable, undernutrition severity as defined by the WHO guidelines took four values, with 0 = no undernutrition, 1 = marginal undernutrition, 2 = moderate undernutrition and 3 = severe undernutrition (WHO, Citation2006; WHO & UNICEF, Citation2019). Since this categorization is ranked, U was considered an ordinal variable. For the binary variable approach, a probit model with an endogenous covariate, , of the following form, was used to jointly estimate Equationequations (1)
(1)
(1) and (Equation2
(2)
(2) ):
where is the standard normal cumulative distribution function that restricts the estimated response probabilities to between zero and one. The endogenous variable D was estimated as:
where is the IV for
.
was measured as the count of all sources of energy used by the child’s household including electricity, gas, biogas, firewood, charcoal, paraffin, candles, generator solar and batteries. The variables are hypothesized to exhibit properties discussed by (Wooldridge, Citation2016), among them direct and significant partial correlation with
after netting out effects of exogenous variables in Equationequation (3)
(3)
(3) ; no direct correlation with U except through
; and no correlation with
. Since different foods require different preparation methods, it was reasonably hypothesized that households which use different sources of energy were more likely to prepare more diverse diets for their children than those with fewer energy sources.
EquationEquations (3)(3)
(3) and (Equation4
(4)
(4) ) were estimated simultaneously by maximum likelihood using Stata command eprobit (extended probit) that accommodates both the continuous and binary nature of endogenous covariate, D (StataCorp, Citation2017). Standard errors were clustered at the household level to correct for lack of independence among children from the same household, and heteroscedasticity. Endogeneity of
was assessed using a test of correlation between v and
, which is inbuilt in eprobit. The test has a null hypothesis of cov (v,
) ≠ 0, that is, unobserved factors in Equationequation (3)
(3)
(3) are not correlated with those in Equationequation (4)
(4)
(4) . A rejection of this hypothesis would imply correlation of the two errors and hence endogeneity of
, justifying the use of estimation methods that correct for endogeneity.
For the categorical (ordinal) variable approach, we estimated the following ordered probit model:
which following Hill et al. (Citation2011) would result in response probabilities be given by:
where for Equationequations (5)(5)
(5) and (Equation6
(6)
(6) ),
;
is a random error which is normally distributed with a mean of zero and variance of one;
is the cut-point (threshold) for each undernutrition indicator (j) and status (k) estimated by the model; and other variables and parameters are as earlier defined. Endogeneity of
was corrected by estimating Equationequation (5)
(5)
(5) jointly with Equationequation (4)
(4)
(4) . This estimation was done by maximum likelihood, using Stata command eoprobit (extended ordered probit) with endogenous covariate (StataCorp, Citation2017). Robust standard errors were used, and test for endogeneity of
done using the approach described in Equationequation (4)
(4)
(4) .
3. Results
3.1. Descriptive results
3.1.1. Dietary quality and mothers’ use of ICT
Children’s consumption of different food groups by the status of their mothers’ ICT use is shown in Table . The results show that CDDS was low, averaging at 2.7, but it was 30% higher for children whose mothers used ICT (P < 0.01). The findings indicate that only a paltry 13.7% of children consumed at least one of the nutrient-dense (ND) food groups rich in iron, protein and calcium that include eggs, meat, and legumes and nuts, but consumption of ND foods was higher for children whose mothers used ICT tools (P < 0.01). The dominant food groups consumed by children were grains, roots and tubers, non-Vitamin A fruits and vegetables and Vitamin A fruits and vegetables. The findings show that children of mothers who used ICT had significantly higher consumption of all food groups (P < 0.01) except breast milk and dairy products compared to children whose mothers did not use ICT.
Table 1. Consumption of different food groups and dietary quality, by mothers’ ICT use status
3.1.2. Prevalence and severity of undernutrition
As shown in Table , about 22% of the children were underweight, with 14.4% being moderately underweight and 8.1% severely underweight. The prevalence of moderate and severe underweight reflects acute under-nutrition and the intensity of nutritional constraints. Wasting was also substantial in the sample (14.3%), with 8.5% of children being moderately wasted and 5.2% severely wasted. Approximately, 18.1% of the children were stunted, with 11.4% being moderately stunted, and 6.6% severely stunted. Children consuming nutrient-dense food groups had a significantly lower prevalence of underweight, wasting and stunting than their counterparts who did not consume these food groups (P < 0.01). Similarly, children of mothers who used ICT tools had a significantly lower prevalence of underweight, wasting and stunting (P < 0.01).
Table 2. Prevalence of child undernutrition by dietary quality, ICT use, and other key variables
3.1.3. Child, mothers’ and household characteristics
Descriptive statistics for variables used in regression analysis are shown in Table . About 51% of the sample comprised of boys and the average age of the children was 32 months. The ICT tools mostly used by mothers are mobile phone (84%) and radio (71%), while TV was least used at 33%. About 26% of mothers had no formal education, while only 7% had attained college or university education. Only 59% of the children had access to improved drinking water sources, while 48% of the children were raised in households that use improved toilet facilities. Notably, only 11% of the children were from households with a place for washing hands near their toilet facility.
3.2. Empirical results
Models (1) to (6) in Table and models (7) to (12) in Table present results on the association of mothers’ ICT use with child dietary quality and undernutrition, respectively. The correlation coefficient corr(e.cdds, e.undernutrition) was significantly different from zero except in the stunting models (Table ), implying that dietary quality was indeed endogenous. The number of energy sources as an instrument turned positive and significant coefficients (Table ), implying that our instrument was strongly associated with the instrumented variable, dietary quality, as measured by CDDS.
Table 4. First-stage results of eprobit and eoprobit showing correlation of ICT use with dietary quality
Table 5. Eprobit and eoprobit results showing correlation of ICT use with child undernutrition
3.2.1. Effect of mother’s use of ICT on child’s dietary quality
Table shows the determinants of dietary quality, which correspond to the first-stage regression results of the undernutrition models in Table , and answers the research question 1: does mothers’ use of ICT effect child’s dietary quality? The results show that children whose mothers used TV and radio consumed more diversified diets compared to children whose mothers did not use the ICT tools. However, for mobile phone use, the effect was not significant. This implies that the use of TV and radio by mothers could help them access information on child nutrition and feeding options, consequently enabling them to provide their children with more diversified and better-quality diets. Results for control variables were omitted from Table for brevity.
3.2.2 Effect of mother’s use of ICT on child undernutrition
Table presents results for eprobit and eoprobit with an endogenous covariate that addressed research question 2: does mothers’ use of ICT affect child’s nutritional outcomes? The coefficient of CDDS was negative and significant for the prevalence of underweight and wasting. This implies that the probability of a child being underweight or wasted was significantly lower for children who consumed high-quality diets. Similarly, the significant negative coefficient of CDDS in models (10) and (11) shows that children consuming quality diets had a lower likelihood of falling into more severe underweight or wasting categories compared to their counterparts not fed on nutrient-rich diets. Surprisingly, CDDS coefficients are positive and insignificant or only weakly significant in the stunting models, implying that controlling for other factors, dietary quality is uncorrelated with the stunting status of children. This is because stunting reflects long-term undernutrition; hence, the current level of dietary quality could be irrelevant. It could also be an indication of compensating behavior of mothers by providing stunted kids with more diversified diets to compensate for stunted growth, hoping to catch up with growth milestones.
The use of ICT by mothers significantly affected undernutrition where a negative and significant coefficient of ICT indicates children of mothers who used ICT had a lower likelihood of suffering from undernutrition. Mothers’ use of mobile phone was associated with a lower prevalence of underweight and a reduced likelihood of shifting from well-nourished status to severe levels of underweight and stunting. Children of mothers who watched TV had a lower probability of falling into severe stunting, compared to children whose parents did not use TV. Similarly, children whose parents listened to radio had a lower prevalence of stunting compared to those whose parents did not listen to radio. Considering the coefficients of the use of different ICT tools on undernutrition, use of mobile phone had the strongest association with a low prevalence of underweight and stunting. Results for control variables on drivers of child undernutrition are omitted from Table for brevity.
4. Discussion
This study contributes to the recent debate on the need to integrate ICT tools and digital innovations into strategies and interventions for disseminating child nutritional and health information for overall child well-being. The study leveraged a comprehensive nationally representative child-level dataset to examine the association of mother’s ICT use with dietary quality and undernutrition among children aged 6–59 months. This study provides novel evidence that mothers’ use of ICT significantly improves the nutritional status of their children, even after controlling for the endogeneity of dietary quality.
The study found that mothers’ use of ICT is significantly associated with a higher probability of providing children with diversified and higher-quality diets and a lower likelihood of a child being undernourished. ICT use is likely to increase the probability of acquiring nutrition and health information from health service providers, the internet and food product advertisements, or sharing nutritional knowledge among mothers through various media and ICT platforms (Manju and Kalpana, Citation2021). Besides, mobile phone applications could improve maternal health care and nutritional information, growth monitoring and tackle child malnutrition, hence overcoming the challenge of malnutrition in children (Desalegn et al., Citation2019; Seyyedi et al., Citation2019). Onyeneke et al. (Citation2019) showed that mobile phone ownership increased dietary quality in children in remote communities in Nigeria, while radio and TV increased the quality of diets in children in Ethiopia (Temesgen et al., Citation2018). The increased access to mobile phones and applications in SSA facilitates the mobile-money transfer and access to loans that foster the ability to buy quality food groups for children and access health care. Besides, SMS-based tools and short videos could help health workers provide essential nutrition and healthcare information to mothers, which could translate to better child nutritional outcomes (UNICEF, Citation2021). Billah et al. (Citation2022) showed that using a digital job aid by community health workers for nutrition counselling to pregnant women improved dietary quality and feeding practices among infants and young children in rural Bangladesh. These findings highlight the need to enhance the sustainability of ICT use in lower-income countries. Improving network coverage, stabilizing and reducing the cost of power sources like solar, electricity, and rechargeable batteries, and increasing access to ICT devices can improve the sustainability of ICT use. Besides, establishment of community digital resource centres to act as central points for information dissemination could improve information access for mothers in low-income settings, who may be faced with serious constraints in acquiring and maintaining ICT tools.
The study also found that dietary quality is associated with a lower prevalence of undernutrition, notably underweight and wasting, and a decreased probability of an upward shift towards severe underweight and wasting levels. This finding accentuates the relevance of dietary quality in improving children’s health outcomes as supported by similar studies in Ghana, Tanzania and Burkina Faso (Frempong & Annim, Citation2017; Khamis et al., Citation2019; Sié et al., Citation2018). Smith and Haddad (Citation2015) demonstrated that the quality and quantity of food available are crucial to reducing undernutrition in children. Evidence shows that quality diets and better nutrition impact a child’s mental, social and physical development and improve the immune system, thus reducing vulnerability to and severity of illness and speeding up recovery from illnesses (UNICEF, Citation2019). However, our results did not find a positive role of dietary quality in reducing stunting. Stunting is a long-term consequence of children’s chronic undernutrition, and the cross-sectional data could not establish a temporal relationship between short-term dietary quality and nutritional outcomes. Besides, other non-dietary factors may be more relevant for reducing stunting in some contexts, or the diets given to children omit foods that may be responsible for increased body height. For instance, environmental factors such as improved water supplies and sanitation facilities, were negatively linked with the prevalence and severity of stunting in this study. This implies that better sanitation facilities and clean water could reduce the incidence of stunting among young children.
5. Conclusions
This study examines the associations of mothers’ use of ICT with dietary quality and child undernutrition in a lower middle-income country context using a sample of 10,385 children aged 6–59 months. The main thrust of this study is the use of nationally representative data collected from all 47 counties in the rural and urban domains, which makes the findings generalizable for the country. The study concludes that mothers’ use of ICT, particularly radio and TV, was significantly (p < 0.01) and positively associated with children’s dietary quality, with radio having the, most substantial effect. Moreover, increasing consumption of diversified and nutrient-dense food groups that include eggs, meat, legumes and nuts significantly (p < 0.01) reduced the prevalence and severity of underweight and wasting in children. However, our results did not find a significant association between dietary quality and stunting, meaning that non-dietary factors may be more relevant for reducing stunting, which is an indicator of chronic malnutrition. The use of ICT by mothers was linked to a lower prevalence and severity of undernutrition (p < 0.05). The use of mobile phones was found to have the most vital link to a decreased prevalence and severity of stunting and being underweight. Children of mothers who watched TV had a reduced probability of being severely stunted, while children of mothers who listened to the radio had a lower likelihood of being severely stunted. Our findings imply that ICT-based strategies and interventions hold great potential to improve mothers’ understanding of child nutrition and health, resulting in better child health outcomes. The results also suggest that complementary ICT tools are needed, to enhance communication between healthcare providers and low-literate mothers. Thus, ICT tools should combine text, voice, and visual communication. Future studies could use panel data and assess mothers’ use of specific information disseminated through ICT, both of which were unavailable for this study.
Acknowledgments
This research did not receive any specific grant from funding agencies in the public, commercial, or not-for-profit sectors. The authors are grateful to KNBS for allowing them access to and use of the datasets.
Disclosure statement
No potential conflict of interest was reported by the authors.
Additional information
Funding
Notes on contributors
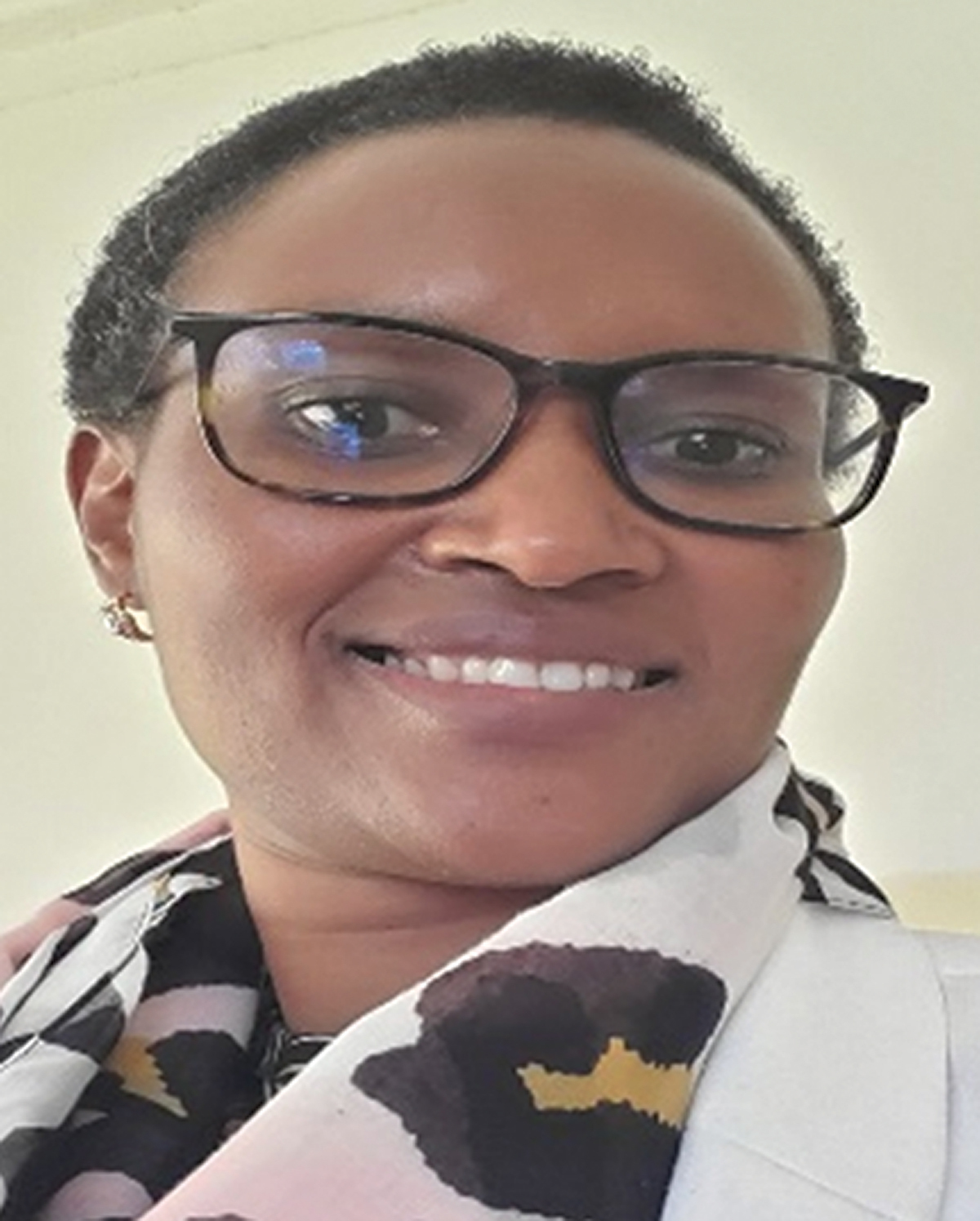
Marther W. Ngigi
Dr Marther W. Ngigi is a researcher in the fields of agricultural economics and market development. Her research experience and interests are in the agricultural market and value chain development, technological change, climate change economics, food security, gender research (youth and women), and factor market dynamics in SSA. She is currently working on ways of engaging youth as agents of change in food systems transformation. Dr Ngigi is a Senior Lecturer at the Department of Agricultural Sciences, Machakos University. She holds a PhD in Agricultural Economics from the University of Bonn, Germany, M.Sc. in Agricultural and Applied Economics and B.Sc. Agribusiness Management from the University of Nairobi, Kenya. Her publications can be found here

Elijah N. Muange
Dr Elijah Muange is an agriculturalist specializing in the economics of agricultural and rural development. He has vast experience in agricultural research and training in Eastern Africa. His experience and interests are in technology research, dissemination and adoption; the role of information dissemination in agriculture; use of social networks and ICT in agricultural information dissemination; the interaction between agriculture, technology and nutrition; agricultural marketing; project evaluations; and impact assessments. Dr. Muange is currently a Lecturer and Chairman at the Department of Agricultural Sciences, Machakos University, where he teaches Agricultural Microeconomics, Production economics, Marketing, International Trade, Research Methods, Statistics, Quantitative Techniques, and Project management. Dr. Muange holds a PhD in Agricultural Sciences (Agricultural Economics & Rural Development) – the University of Gottingen; and MSc in Agricultural & Applied Economics and BSc in Agriculture (Agricultural Economics Major) from the University of Nairobi. He has published widely in peer-reviewed journals here
References
- Aboagye, R. G., Seidu, A.-A., Ahinkorah, B. O., Arthur-Holmes, F., Cadri, A., Dadzie, L. K., Hagan, J. E., Eyawo, O., & Yaya, S. (2021). Dietary diversity and undernutrition in children aged 6–23 months in Sub-Saharan Africa. Nutrients, 13(10), 3431. https://doi.org/10.3390/nu13103431
- Akombi, B. J., Agho, K. E., Hall, J. J., Wali, N., Renzaho, A. M. N., & Merom, D. (2017). Stunting, wasting and underweight in Sub-Saharan Africa: A systematicrReview. International Journal of Environmental Research and Public Health, 14(8), 863. https://doi.org/10.3390/ijerph14080863
- Billah, S. M., Ferdous, T. E., Kelly, P., Raynes‐Greenow, C., Siddique, A. B., Choudhury, N., Ahmed, T., Gillespie, S., Hoddinott, J., Menon, P., Dibley, M. J., & Arifeen, S. E. (2022). Effect of nutrition counselling with a digital job aid on child dietary diversity: Analysis of secondary outcomes from a cluster randomised controlled trial in rural Bangladesh. Maternal & Child Nutrition, 18(1), e13267. https://doi.org/10.1111/mcn.13267
- Debela, B. L., Demmler, K. M., Klasen, S., & Qaim, M. (2020). Supermarket food purchases and child nutrition in Kenya. Global Food Security, 25, 1–14. https://doi.org/10.1016/j.gfs.2019.100341
- Desalegn, B., Riedel, C., Lambert, C., Widmer, S., Negese, T., & Biesalski, H. (2019). Nutrient Intake of 12–59-Months-Old children and women in Ethiopia, and development of Calculator for Inadequate Micronutrient Intake (CIMI) app for Ethiopian Population (P13-008-19). Current Development in Nutrition, 3(Suppl 1), nzz036.P13-008–19. https://doi.org/10.1093/cdn/nzz036.P13-008-19
- Fadare, O., Amare, M., Mavrotas, G., Akerele, D., Ogunniyi, A., & Vellakkal, S. (2019). Mother’s nutrition-related knowledge and child nutrition outcomes: Empirical evidence from Nigeria. PLOS ONE, 14(2), e0212775. https://doi.org/10.1371/journal.pone.0212775
- FAO, IFAD, UNICEF, WFP, & WHO. (2019). The state of food security and nutrition in the world: Safeguarding against economic slowdowns and downturns. FAO.
- Frempong, R. B., & Annim, S. K. (2017). Dietary diversity and child malnutrition in Ghana. Heliyon, 3(5), e00298. https://doi.org/10.1016/j.heliyon.2017.e00298
- Global Nutrition Report. (2021). 2021 Global Nutrition Report: The state of global nutrition. Development Initiatives. https://globalnutritionreport.org/reports/2021-global-nutrition-report/
- Hill, R. C., Griffiths, W. E., & Lim, G. C. (2011). Principles of econometrics. international student version (4th ed.). John Wiley & Sons, Inc.
- Kenya National Bureau of Statistics (Ed.). (2018). Basic report: Based on 2015/16 Kenya integrated household budget survey (KIHBS). Kenya National Bureau of Statistics. https://statistics.knbs.or.ke/nada/index.php/catalog/13/download/58.
- Khamis, A. G., Mwanri, A. W., Ntwenya, J. E., & Kreppel, K. (2019). The influence of dietary diversity on the nutritional status of children between 6 and 23 months of age in Tanzania. BMC Pediatrics, 19(1), 518. https://doi.org/10.1186/s12887-019-1897-5
- Lowery, C., Oddo, V., Hurley, K., Jones-Smith, J., Gittelsohn, J., Ponce, S. D., & Black, M. (2019). Maternal employment and children’s dietary diversity scores in Southwestern Guatemala (P10-039-19). Current Developments in Nutrition, 3(Supplement_1), nzz034.P10-039–19. https://doi.org/10.1093/cdn/nzz034.P10-039-19
- Machira, K., Chirwa, T., & Joe, W. (2020). Dietary consumption and its effect on nutrition outcome among under-five children in rural Malawi. PLOS ONE, 15(9), e0237139. https://doi.org/10.1371/journal.pone.0237139
- Manju, P. G., & Kalpana, C. A. (2021). Digital nutrition consultation among hand-held device users during COVID-19 pandemic. International Journal of Food and Nutritional Sciences, 10(3), 18–22. https://ijfans.org/uploads/paper/b87d10af62ba58d25d0bf9db812d4baa.pdf
- Marinda, P. A., Genschick, S., Khayeka-Wandabwa, C., Kiwanuka-Lubinda, R., Thilsted, S. H., & Wieringa, F. (2018). Dietary diversity determinants and contribution of fish to maternal and under-five nutritional status in Zambia. PLOS ONE, 13(9), e0204009. https://doi.org/10.1371/journal.pone.0204009
- Matanda, D. J., Mittelmark, M. B., & Kigaru, D. M. D. (2014). Child undernutrition in Kenya: Trend analyses from 1993 to 2008–09. BMC Pediatrics, 14(1), 5. https://doi.org/10.1186/1471-2431-14-5
- Muange, E. N., & Ngigi, M. W. (2021). Dietary quality and overnutrition among adults in Kenya: What role does ICT play? Food Security, 13(4), 1013–1028. https://doi.org/10.1007/s12571-021-01174-8
- Obasohan, P. E., Walters, S. J., Jacques, R., & Khatab, K. (2020). A scoping review of the risk factors associated with anaemia among children under five years in Sub-Saharan African countries. International Journal of Environmental Research and Public Health, 17(23), 8829. https://doi.org/10.3390/ijerph17238829
- Olson, C. M. (2016). Behavioral nutrition interventions using e- and m-health communication technologies: A narrative review. Annual Review of Nutrition, 36(1), 647–664. https://doi.org/10.1146/annurev-nutr-071715-050815
- Onyeneke, R. U., Nwajiuba, C. A., Igberi, C. O., Umunna Amadi, M., Anosike, F. C., Oko-Isu, A., Munonye, J., Uwadoka, C., & Adeolu, A. I. (2019). Impacts of caregivers’ nutrition knowledge and food market accessibility on preschool children’s dietary diversity in remote communities in Southeast Nigeria. Sustainability, 11(6), 1688. https://doi.org/10.3390/su11061688
- Republic of Kenya (2019) Statistics Act No. 4 of 2006: Revised Edition 2019 [2006]. Nairobi: National Council for Law Reporting. https://www.knbs.or.ke/wp-content/uploads/2020/12/Statistics-Act-4-of-2006-Revised-Edition-2019.pdf.
- Seyyedi, N., Rahimi, B., Farrokh Eslamlou, H. R., Timpka, T., & Lotfnezhad Afshar, H. (2019). Mobile phone applications to overcome malnutrition among preschoolers: A systematic review. BMC Medical Informatics and Decision Making, 19(1), 83. https://doi.org/10.1186/s12911-019-0803-2
- Sié, A., Tapsoba, C., Dah, C., Ouermi, L., Zabre, P., Bärnighausen, T., Arzika, A. M., Lebas, E., Snyder, B. M., Moe, C., Keenan, J. D., & Oldenburg, C. E. (2018). Dietary diversity and nutritional status among children in rural Burkina Faso. International Health, 10(3), 157–162. https://doi.org/10.1093/inthealth/ihy016
- Smith, L. C., & Haddad, L. (2015). Reducing child undernutrition: Past drivers and priorities for the post-MDG era. World Development, 68, 180–204. https://doi.org/10.1016/j.worlddev.2014.11.014
- StataCorp. (2017). Stata Extended Regression Models Reference Manual Release 15. StataCorp LLC. https://www.stata.com/manuals/erm.pdf
- Temesgen, H., Yeneabat, T., & Teshome, M. (2018). Dietary diversity and associated factors among children aged 6–23 months in Sinan Woreda, Northwest Ethiopia: A cross-sectional study. BMC Nutrition, 4(1), 5. https://doi.org/10.1186/s40795-018-0214-2
- UNICEF. (2019) . Children, food and nutrition: Growing well in a changing world.
- UNICEF. (2020). Nutrition, for every child: UNICEF nutrition strategy 2020–2030. United Nations Children’s Fund.
- UNICEF. (2021). Accelerating results for children with technology and digital innovation. Technology for Development Report. New York: UNICEF.
- United Nations (Ed.). (2017). Transforming our world: The 2030 agenda for sustainable development, A/RES/70/1. United Nations. https://doi.org/10.1891/9780826190123.ap02
- WHO. (2006). WHO child growth standards: Length/height-for-age, weight-for-age, weight-for-length, weight -for-height and body mass index-for-age : Methods and development. World Health Organization. https://apps.who.int/iris/handle/10665/43413
- WHO. (2021). Infant and Young Child Feeding. https://www.who.int/news-room/fact-sheets/detail/infant-and-young-child-feeding
- WHO & UNICEF. (2019). WHO/UNICEF discussion paper: The extension of the 2025 maternal, infant and young child nutrition targets to 2030. ReliefWeb. https://reliefweb.int/report/world/whounicef-discussion-paper-extension-2025-maternal-infant-and-young-child-nutrition
- Wooldridge, J. M. (2016). Introductory econometrics. A modern approach (6th ed.). Cengage Learning. https://www.cengagebrain.co.uk/shop/isbn/9781305270107